In-situ real-time monitoring of muscle energetics with soft neural-mechanical wearable sensing
Abstract
Skeletal muscles, as the primary actuators for voluntary limb motions, achieve motion dexterity and endurance at the cost of majority of metabolic energy. Muscle energetics provide a powerful framework for examining motion skills, serving as fundamental mechanisms for converting metabolic energy into effective work to optimize motion performance through muscle synergy. However, existing energy sensing methods are sensitive to physiological, psychological, and environmental disturbances, making it challenging to monitor the energetic dynamics of muscle synergy. Inspired by the characteristics of muscle excitation and contraction, this study proposes a neural-mechanical sensing method to perceive muscle work by integrating the myoelectric and capacitive measurements that are indicative of muscle forces and contraction displacements. The proposed sensing method is validated through the weight lifting tests, comparing results against the dynamic analysis and muscle oxygen consumption. To the best of our knowledge, this research is the first to achieve in-situ real-time wearable sensing of muscle work. It is expected to pave a practical way to study muscle energetics that is beneficial to sports science, rehabilitation medicine and robotics engineering.
Keywords
INTRODUCTION
Skeletal muscles play a critical role in executing voluntary limb motions, consuming the majority of the metabolic energy to perform mechanical work[1]. While muscle strength training can enhance motion capabilities by providing large impulses and strong endurance[2,3], it does not guarantee improved dexterity in motion performance[4]. For instance, athletes with the strongest muscles may not excel in swimming if they lack the necessary skills. Similarly, an elderly person with weaker muscles may maintain a more stable jogging pace than a strong adult immediately after a stroke. Powered exoskeletons designed for weight bearing and gait assistance could lead to an increase of 26% in heart rate and an increase of 39% in oxygen consumption[5]. In these examples, the work performed by individual muscles may counteract one another due to inadequate coordination, neural disorders, or poor human-machine interactions, resulting in suboptimal performance of overall human body motions. Even a simple action such as level walking requires precise muscle work at the right joint and timing to achieve forward movement with each stride[6]. In other words, muscle energetics offers a powerful tool for examining motion skills, functioning as a fundamental mechanism for converting metabolic cost into effective work, both spatially and temporally, to optimize motion performance through muscle synergy. Therefore, quantifying individual muscle work is crucial for understanding the fundamentals of musculoskeletal biomechanics to restore motion capability and improve motion efficiency.
Muscle work can be evaluated through physiological methods involving biochemical measurements and mechanical approaches using dynamic analysis. Metabolic energy analyzers, which assess human motion efficiency based on oxygen consumption and carbon dioxide concentration[7], provide a practical metric for evaluating assistive performance of wearable robots[8]. However, typical gas analyses suffer from slow responses of over 1 s and significant delays due to breathing, making them inadequate for real-time applications, especially during rapid human movements[9]. Furthermore, exoskeletons have been shown to reduce metabolic cost by 7.2%, although the standard deviation of the measured metabolic cost can be as high as ±2.6% (accounting for 36.1% of the 7.2% reduction). In addition, obtaining accurate data requires multiple long-duration measurements, making real-time monitoring challenging[10]. The large standard deviation in overall metabolic measurement can be attributed to various influencing factors, such as physiological conditions, mental states, and environmental disturbance. For instance, every 1 °C increase in fever leads to an approximately 9% increase in oxygen consumption[11]; intense mental work results in an extra 10% consumption of local glucose[12]; and the body needs to increase heat generation by about 7% in mildly cold environments[13]. The near-infrared spectroscopy (NIRS) offers another way to assess local muscle metabolism by detecting hemoglobin concentration[14,15]. It is worth noting that fluctuations of oxygen saturation due to blood circulation can hinder quantitative analysis that requires complicated processing algorithms[16]. Dynamic analysis is a straightforward approach to quantify muscle work with the contraction force and deformation displacement. The isotonic testing system[17,18], which tracks the joint torque and angular velocity, provides a standard assessment for the maximal muscle group power. However, this bulky equipment is limited to testing single joint rotations, making it unsuitable for general motion studies. Detailed comparison is summarized in Supplementary Table 1.
Soft wearable sensors provide a non-invasive approach to monitor neuromuscular activation and biomechanical dynamics through electromyography (EMG) and muscle contractions. While EMG relates to muscle force magnitude[19], the scalar signals cannot infer the force direction, preventing differentiation between concentric (positive) and eccentric (negative) mechanical work. Furthermore, isometric contractions produce non-zero EMG despite generating no external mechanical work. Hybrid sensing strategies integrating EMG and inertial measuring units (IMUs)[20,21] attempt to estimate muscle force or even muscle work by inferring contraction displacements from joint kinematics[22]. However, such methods suffer from limitations including model complexity, algorithm errors and heavy computations, leading to inaccuracies in resolving individual muscle deformation and work output [Supplementary Table 2]. Emerging modalities, including capacitive sensing[23], piezoelectric sensing[24], ultrasound imaging[25], resistive sensing[26] and electrical impedance tomography[27], are capable of augmenting EMG with muscle kinematics for the possibility of energy sensing, among which the capacitive strain sensors possess the good dynamic durability, low lag, and high linearity.
It is clear from the above that there is currently no reliable method to quantitatively measure individual muscle work by effectively coupling neural and mechanical signals in a simple and accurate way. Only recently have their simultaneous measurements at the same location on a muscle become feasible; however, these novel bi-modal sensing methods identify the latency of the neural and mechanical signals as a significant limitation in the application of motion pattern recognition[28,29]. To tackle the challenging problem of in-situ real-time muscle work sensing, our study introduces a generalized energy framework for muscle work assessment by integrating neural drive (EMG-derived force estimation)[30] and mechanical displacement (capacitive sensing)[23] into a unified paradigm. Central to this idea is a neural-mechanical sensor featuring a shared-electrode mechatronic architecture that enables simultaneous in-situ capture of muscle deformations and electrophysiological activities. While the numerical integration strategy of dual-mode signals to minimizes latency artifacts between modalities and resolves zero-work issue during muscle isometric contractions. The sensor employs copper calcium titanate (CCTO)[31] for high-sensitivity capacitive strain detection and nano silver powder[32] for optimized myoelectric signal acquisition. This co-located sensing strategy eliminates latency artifacts between modalities, ensuring temporally aligned signal fusion that bypasses traditional postprocessing delays during non-working muscle states. To our knowledge, this work represents the first demonstration of in-situ real-time wearable sensing of muscle work. It is expected that the proposed method paves a practical way for muscle work monitoring that is beneficial to sports science, rehabilitation medicine and robotics engineering.
EXPERIMENTAL
Fabrication of the neural-mechanical sensor
The sensor was constructed by first laminating a 0.1 mm-thick copper/nickel-coated polyester conductive fabric (20 mm diameter, conductivity approximately 7.4 × 105 S/m) with a 0.2 mm polyvinyl chloride (PVC) encapsulation layer, followed by screen-printing a flexible silver nanoparticle electrode [NSPP composite: nano silver/polydimethylsiloxane (PDMS)/kerosene 8:2:1 mass ratio, cured at 160 °C for 3 h] onto a 0.2 mm PDMS substrate to achieve a 0.1 mm conductive layer (conductivity about 6.5 × 106 S/m), interconnected via silver-welded joints (7 mm × 6 mm) to copper wires. A 3 mm-thick CCTO and polyurethane sponge (CCTPS) dielectric layer was integrated by immersing a 60 par per inch (PPI) polyurethane sponge (20 mm diameter) in an ultrasonically homogenized CCTO/3-amino-propyl-triethoxysilane (APTES) suspension (mass ratio 35:10:75:1), dried at 60 °C for 8 h to enhance particle adhesion. Finally, a double-sided adhesive patterned with 2 mm apertures (3 mm spacing) filled with conductive cream was applied to optimize skin-electrode contact impedance.
Weight lifting with single muscle
The protocol focused on controlled weight lifting by a target muscle (e.g., biceps brachii, BIC) to quantify its mechanical work output [Supplementary Note 1]. Participants stabilized their upper arm on a 115 mm vertical support, lifting a 1.25 kg mass until the forearm reached the vertical orientation (γ = 90°) perpendicular to the table surface, then lowering it back to the initial resting position (γ = θ - 180°). This motion facilitated BIC-specific concentric (lifting) and eccentric (lowering) contractions, with equivalent mechanical work during elevation due to the identical vertical displacement of the mass. For active trials (fast and slow speeds), the BIC performed work to rotate the forearm from a stationary position (zero initial velocity) to a stationary state (zero final velocity), while passive trials (gravity-driven release) eliminated active muscle tension, resulting in a non-zero terminal velocity as the mass accelerated under unopposed gravitational force.
Weight lifting with multiple muscles
The second weight-lifting test was designed to assess mechanical work performed with multiple upper-limb muscles. Participants leaned forward with their upper arm angled at θ relative to the torso and aligned parallel to the table. To minimize compensatory motion of the upper body, the left hand stabilized the right shoulder. The forearm executed a forward lift of weight from the initial resting state (γ = -π/2) to γ = -θ, a phase dominated by mechanical work from the BIC thus termed the BIC phase. Subsequently, the weight was lifted backward from γ = -π/2 to γ = -π + θ, a transitioned to the triceps brachii (TRI) dominance, referred to as the TRI phase.
RESULTS AND DISCUSSION
Muscle work sensing with neural-mechanical sensor
Motor neurons transmit action potentials along nerve fibers to generate the EMG in muscles [Figure 1A], which further causes muscle contractions, limb motions and energy consumption. Inspired by the excitation and contraction signatures of muscles, the neural-mechanical sensor is designed by integrating a capacitive sensing component and an EMG electrode. The sensor is composed of the PVC film, conductive fabric electrode, CCTPS dielectric layer, flexible silver nanoparticle electrodes, and conducting layer [Figure 1B]. The conductive fabric and flexible silver nanoparticle electrodes together with the CCTPS dielectric layer form the capacitance Cs. The conductive fabric electrode is encapsulated by PVC films to prevent a short circuit between the two electrodes. The flexible silver nanoparticle electrode is used to monitor muscle EMG in terms of the voltage U with reference to the commercial gel electrode. The conducting layer, a double-sided adhesive foam with a planar array of circular holes (PACH) containing conductive cream, provides low and stable contact impedance between the flexible silver nanoparticle electrode and human skin. PDMS was chosen for its unique mechanoelectrical stability, overcoming limitations of hydrogels (ionic drift/dehydration) and Ecoflex (interfacial delamination due to < 100 kPa modulus). Its 1-2 MPa modulus balances mechanical support and elasticity, enabling durable signal fidelity across 30%-100% strain ranges during dynamic monitoring. Silver nanoparticles overcome the limitations of liquid metals (processing challenges) and carbon nanotubes (humidity sensitivity and non-screen-printable properties), achieving high conductivity [signal-to-noise ratio (SNR) > 46 dB] and flexible compatibility when compounded with PDMS. CCTO, with a dielectric constant three times that of barium titanate (BaTiO3) and 30 times that of strontium titanate (SrTiO3), enhances capacitive sensitivity when integrated into polyurethane sponge while retaining flexibility (compression resilience > 98%) and thermal stability (250 °C). These two materials synergistically achieve the integration of high sensitivity and wearable reliability. In practice, the neural-mechanical sensor is secured in place and preloaded using an adhesive tape made of non-stretchable acetate cloth. The sensor prototype has a diameter of 24.5 mm and a thickness of 4.5 mm [Figure 1C]. The capacitive deformation signal is acquired via an AD7746 analog-to-digital converter (21-bit resolution, ±4 pF range, expandable to 0-21 pF), while the EMG signal is processed through an AD8422ARMZ instrumentation amplifier (±3,300 μV range, 16-bit resolution), sampled at
Figure 1. Neural-mechanical sensor. (A) Comparison between deformation and EMG during voluntary muscle contraction; (B) Schematic of the neural-mechanical sensor; (C) Appearance of the sensor prototype with labeled dimensions; (D) Experiment of weight lifting. The volunteer subject performed the weight lifting with muscle concentric, isometric, and eccentric contractions; (E) Responses of the measured EMG and Cs under different muscle contractions for testing muscle fatigue; (F) Illustration of Ew calculation. The Ew is calculated with integration of the RMS of EMG and Cs for different muscle contraction modes during the fatigue testing. EMG: Electromyography; RMS: root mean square.
Since the muscle work can be estimated by the inner product of muscle contraction forces and deformation displacements, its equivalent index can be formulated as the area under the relation curve between UR and Cs [Figure 1F]
with the assumptions: (1) The displacement of muscle contraction ΔL is linear with change of the measured Cs in ΔL = k1ΔCs; (2) the muscle force F is linear with the root mean square (RMS) of EMG, namely UR, processed with a sliding window via F = k2UR; and (3) the muscle strain energy determined by Es = ∫F(t)dL can be expressed as
where K = k1k2, and L and F are evaluated by the kinematic and dynamic analysis in Supplementary Note 1.
For a musculoskeletal system consisting of n muscles, it performs the total mechanical work
where the strain energy Es(i) for each muscle transforms into mechanical work and dissipative energy with the efficiency of 0 < ηi < 1 for i = 1, 2, …, n. Substitution of Equations (2) into (3) gives rise to
where ηi and Ki vary with different muscles. The above illustrates the overall mechanical work for the musculoskeletal system as an algebraic summation of each muscle work.
To validate the muscle work sensing model, the overall work performed by the musculoskeletal system is quantified with the weight lifting test [Figure 1D] in terms of the mechanical energy of the weight m
where g is the gravitational acceleration, L12 is the forearm length, γ is the angle between the forearm and the horizontal X-axis, and ω is the angular velocity of forearm rotation.
Calculation of Ew with UR and Cs using Equation (1) is illustrated for different modes of muscle motions in the weight lifting test. The measured BIC went through concentric, isometric, and eccentric contractions when the tested subject lifted a 6-kg weight to a position where the forearm was parallel to the ground, and held still before releasing to the initial state [Figure 1D]. The neural-mechanical sensor simultaneously captures both EMG and muscle deformation [Figure 1E]. During concentric and eccentric contractions, the measured EMG occurred and vanished earlier than the muscle deformation, and the mean power frequency (MPF) of the EMG gradually decreases while the measured capacitive deformation stays stable during isometric contraction with fatigue [Supplementary Figure 1]. Figure 1F shows the change in the area under the Ew curve in the lifting condition. When the muscle undergoes isometric contractions, Ew remains approximately constant with little change of the area spanned by UR and Cs [Figure 1F]. It further shows isometric contractions (purple) yield far less cumulative Ew over 50 s than brief, high-intensity concentric/eccentric contractions (lasting for couple seconds). The EMG-to-deformation latency (20-50 ms) is negligible compared to typical muscle contraction duration (> 1 s). During this brief delay, no deformation occurs despite initial EMG surges in concentric contractions [Supplementary Figure 1A], whereas deformation persists after EMG stops during passive stretching [Supplementary Figure 1B]. These transient differences have minimal impact on integration of muscle work (Ew) due to smooth strain progression and limited EMG fluctuations. Given the positive UR, the sign of Ew increment is the same as that of Cs change for different muscle contractions. As the muscle performs positive work to lift the weight, Ew increases with UR and Cs. When the muscle undergoes isometric contractions, Ew remains approximately constant with little changes of UR and Cs. After a prolonged isometric contraction, the fatigue muscle was unable to maintain enough tension and started to be passively stretched by the weight descending. In this way, the area Ew slightly decreases [Supplementary Figure 1D] with the dramatic vanishing of the measured EMG as visualized by the blue curve for the eccentric contraction [Figure 1F].
Mechatronic design and performance specification
Before leaping into neural-mechanical coupling, electrical and mechanical performance for the patchable electrodes were investigated for different designs in terms of contact impedance, SNR, sensitivity, and fatigue resistance. To achieve a low and stable contact impedance for EMG sensing with a high SNR, the flexible silver nanoparticle electrode was fabricated with a PDMS film printed with the mixed material that consisted of nanosilver powder dispersed in PDMS liquid with industrial kerosene (NSPP). The measured contact impedance of the sensor on the human skin decreases with the excitation frequency of the inductance-capacitance-resistance (LCR) digital bridge and is on average 46% lower than the contact impedance of commercial gel electrodes in the range within 1 kHz [Figure 2A]. This is attributed to the excellent conductivity and high conformability of the conductive gel and nano silver powder in the flexible silver nanoparticle electrode. In a 12 kg weight lifting test, SNR of the EMG collected on the BIC was
Figure 2. Mechatronic design and performance characterization of the sensor. (A) Comparison of the neural-mechanical sensor and commercial gel electrode. Impedances are analyzed for the two electrodes adhered to the skin on the BIC; (B) EMG characterization for the two electrodes. The measured EMG captured by the neural-mechanical sensor and commercial gel electrode were compared in terms of SNRs; (C) Effect of muscle deformations on the impedance of the skin-electrode interface; (D) Impedance variation with cyclic compression. The maximum impedance change was only 1.05% with a cyclic loading for 750 times; (E) Mechanical properties of the polyurethane sponges. In comparison of polyurethane sponges with different pore sizes, the pore density of 60 PPI exhibited the highest sensitivity in the compression test; (F) Effects of CCTO on the sensitivity of muscle deformation measurements. The sensitivity of the sensor with CCTO is three times higher than that without CCTO; (G) Cyclic compression test. During 14,000 cycles of compression, the measured capacitive deformation exhibited a drift of approximate 0.85%; (H) SNR of the capacitive sensing for a tender touch. BIC: Biceps brachii; EMG: electromyography; SNRs: signal-to-noise ratios; PPI: par per inch; CCTO: copper calcium titanate.
To investigate the stability of electrode contact impedance when the muscle deforms and compresses the neural-mechanical sensor, compression tests (the compression machine ZQ-990) were conducted on pig skin. When the CCTPS dielectric layer of neural-mechanical sensor was compressed by 1.8 mm using a custom-made formaldehyde fixture, the impedance change of the skin-electrode interface is 0.111 kΩ (red line) and 0.043 kΩ (black line) for cases with conductive cream alone and a double-sided foam adhesive with PACH, respectively [Figure 2C]. The double-sided foam adhesive with PACH reduces the contact impedance variation by holding the conductive cream in place without leaking from the skin-electrode interface. In addition, the maximum impedance change is only 1.05% with a cyclic loading for 750 times [Figure 2D]. This means that during cyclic muscle deformations, the changes in the contact impedance at the skin-electrode interface are small enough that there is no significant distortion in the EMG data captured by the neural-mechanical sensor [Supplementary Figure 2].
Sensitivity of the capacitive measurements is characterized by comparing six polyurethane sponges (3.1 ± 0.15 mm in thickness) of 10 to 60 PPI. The 60 PPI design has the highest sensitivity with the steepest slope for the loading curve [Figure 2E]. The CCTPS dielectric layer exhibits high dielectric performance with the CCTO attached on the porous inner surface of the polyurethane foam. The sensor sensitivity for the design with CCTO is larger than 7 and three times of that for the case without CCTO [Figure 2F]. In addition, the measured capacitance change exhibits a linear relationship of the compression displacement with the square of the correlation coefficient (R2) greater than 0.93 for both loading and unloading at different step sizes of 0.2, 0.3 and 0.45 mm. The fatigue performance was investigated with a compression test of more than 14,000 cycles, during which the drift of 0.083 pF was observed throughout the process (approximately 0.85% of the amplitude change of 9.736 pF in Figure 2G). Furthermore, the SNR of muscle deformation sensing is estimated as 23.26 dB for a tender touch [Figure 2H].
Coupling muscle deformation and EMG
The neural-mechanical sensor, by coupling the bipolar plate capacitor with EMG electrodes, simultaneously measures muscle deformations and EMG in-situ. To analyze neural and mechanical motions of a muscle, lifting tests were conducted with various muscle contraction modes and speeds. As shown in the single muscle lift [Figure 3A].
Figure 3. The measured EMG, capacitive deformation and muscle work for different muscle contractions. (A) Weight lifting test with single muscle. The volunteer lifted a weight with muscle CC and released it with EC at different speeds. Each column of plots corresponds to different muscle motions: slow CC - slow EC, fast CC - fast EC, slow CC - passive EC, and fast CC - passive EC. The muscle was under tension during slow and fast movements, and was without tension during passive movement; (B) Measurements of EMG and Cs during muscle contractions. RMS of EMG is also calculated for different contractions; (C) Combination of the Cs and the RMS of EMG for different muscle contractions; (D) Illustration of Ew for weight lifting tasks. EMG: Electromyography; CC: concentric contraction; EC: eccentric contraction; RMS: root mean square.
Within one motion cycle, the muscle eccentric contraction facilitated by the gravity produced a weaker EMG than the concentric contraction. In the muscle concentric contraction, slow lifting of 9.3 s produced a weak EMG with its RMS of 203 μV and a large capacitive change of 0.113 pF, while the fast lifting of 1.4 s had a strong EMG with RMS of 262 μV and a small capacitive change of 0.076 pF (the first two plots in Figure 3B). Considering the positive correlation between the RMS of EMG and muscle force levels
For eccentric contractions with muscle tension, the blue area is approximately equal to the red one as the muscle performed negative work and Ew returns to the baseline near zero (the first and second plots in Figure 3C and D). The integration with Equation (1) provides approximately the same maximum Ew for concentric muscle contractions at different speeds (around 17 μV·pF in Figure 3D), because a similar amount of muscle work was performed to raise the weight to the same height. In this way, Ew increases with positive muscle work and decreases with negative muscle work. On the other hand, for eccentric contractions without muscle tension, the muscle performed little work during the passive stretching, and little decrement is observed in Ew due to the small blue area (the third and fourth plots in Figure 3D).
Muscle work estimation
Assumptions (1-3) are validated by investigation of Ew via comparison with the change of mechanical energy Em of the lifted weight and the muscle oxygen saturation SmO2 captured by the NIRS technique (MOXY-3). NIRS was used to measure changes in oxygenated/deoxygenated hemoglobin concentrations at the BIC belly via the principle of diffuse reflection, employing dual-wavelength light sources (730 and
Figure 4. The work of a single muscle. (A and B) Relationship between the measured capacitive deformation and muscle contraction displacement. The change of Cs is consistent with the variation in muscle contraction displacement with the linear regression of R2 = 0.96; (C and D) Relationship between the muscle contraction force and RMS of EMG. The RMS of EMG matches the variation of muscle contraction force with the linear regression of R2 = 0.91; (E) Comparison of muscle work Ew, mechanical energy Em of the weight, and muscle oxygenation SmO2; (F) Linear regression between Em and Ew with R2 = 0.95; (G) Characterization of the measured Cs and EMG during multiple cycles of weight lifting with one single muscle; (H) Validation of muscle work during repeated weight lifting with one single muscle. The high-pass filtered Ew is consistent with Ewmith the linear regression of R2 = 0.95. RMS: Root mean square; EMG: electromyography.
Assumptions (1-3) are further validated for the case involving multiple muscles of the BIC and TRI where
Figure 5. The work performed by multiple muscles. (A) Weight lifting with both BIC and TRI muscles. The BIC phase refers to the period when BIC dominates in positive work performance, and the TRI phase denotes the period when TRI contributes to the major part of positive work; (B and C) Measurements of Cs and EMG on the BIC and TRI muscles throughout the lifting process; (D and E) Comparison of ΔCs and the muscle contraction displacement for BIC and TRI. The regression of ΔCs and muscle contraction displacement was characterized by R2 = 0.98 and 0.85 for BIC and TRI, respectively, validating the capacitive sensing for muscle deformations during collaborative muscle motions; (F and G) Comparison of the RMS of EMG and the muscle contraction forces for BIC and TRI. The regression of RMS of EMG and muscle contraction force was characterized by R2 = 0.98 and 0.91 for BIC and TRI, respectively, validating the feasibility of muscle force sensing with EMG during collaborative muscle motions; (H and I) Curves plotted with ΔCs and the RMS of EMG measured on the BIC and TRI; (J and K) Comparison of Em and Ew (= Ew-BIC + Ew-TRI) with their linear regression of R2 = 0.92, where
Discussion of experimental findings
Previous studies have captured muscle excitation-contraction characteristics with local coupling mechatronic interfaces to identify motion characteristics for human-robot interaction. Muscle strains and EMG signals were coupled to delineate the gait phase cycles of the lower limbs[29]. The finger flexion speed and the gripping strength were qualitatively monitored by the muscle strain rate and the EMG amplitude, respectively[28]. Product of the muscle forces and deformations can lead to an estimation of the effective work performed by the muscle. However, the prerequisite of muscle work sensing for coupled force and deformation quantification has not been well studied and established yet, because there exist spatiotemporal differences between action potentials and myofiber contraction[33] rendering challenges of in-situ measurement and signal synchronization for numerical integration.
Inspired by the working principle of muscle motions, the coupled neural-mechanical sensing method has been developed to capture individual muscle work. It offers the advantages of (1) in-situ (2) real-time monitoring of muscle work and (3) quantitative neural-mechanical coupling of muscle forces and contraction deformations. It achieves the SNRs of 46.8 dB and 23.26 dB respectively for the deformation and EMG sensing [Figure 2B and H]. The robustness was justified by the negligible drift of 0.85% in capacitive measurements during 14,000 cycles of loading [Figure 2G]. Combining with the lift test [Supplementary Figure 7], the proposed method has been experimentally validated by comparing against the local physiological measurements of oxygenation SmO2 [Figure 4E] and the overall dynamic analysis of mechanical energy [Figure 4F].
The coupled neural-mechanical sensing is able to fully capture muscle kinematics and dynamics. The capacitive sensing can discriminate the muscle motion modes of concentric, eccentric and isometric contractions with the increasing, decreasing and constant measurements, respectively. On the other hand, EMG sensing can identify active and passive motions by the existence of muscle tensions caused by action potentials [Figure 3B]. In this way, an active concentric contraction produces positive work, and an active eccentric contraction gives rise to negative work, while other motions such as isometric contractions or passive deformations do not exert effective work [Figure 3D].
The proposed method also provides an alternative way to study muscle synergy from the energetic viewpoint. A biological joint is typically driven by antagonistic motions among muscle groups. Common myoelectric signals could tell the neural state of a muscle such as activation, fatigue or at rest[34]; however, they could hardly quantify the effective contribution of each muscle’s to overall joint motions. Figure 5J illustrates workload-specific neuromuscular activation patterns in the BIC (Ew-BIC, red line) and TRI (Ew-TRI, blue line), where the BIC generated symmetrical mechanical work during its active phase but showed minimal engagement in the TRI phase, while the TRI exhibited reciprocal behavior. Both muscles achieved the maximum external work output (approximately 9 μV·pF), consistent with similar lifting height during their respective phases. The calculated total multi-muscle work (Ew, green line) strongly correlated (R2 = 0.92) with the mechanical energy change (Em, black line) derived from IMU measurements. The coupled sensing method represents a significant breakthrough in the energy monitoring of muscle synergy, as compared with all existing techniques [Supplementary Table 1]. In addition, energetics of muscle synergy provide a practical metric to evaluate the performance of wearable robots and locomotion capabilities of human bodies on the muscle energy level instead of overall metabolic cost, which may in turn provide a detailed guideline to improve motion skills[35,36], innovate robot designs[6,37] and optimize feedback control[38].
While the proposed neural-mechanical sensing method, which integrates myoelectric and capacitive measurements to quantify muscle work through voltage and capacitance rather than joules, demonstrates feasibility through its correlation with the mechanical energy expenditure during weight lifting, it represents one of many potential approaches for analyzing muscle synergy energetics. Existing alternatives for assessing muscle forces include phonomyogram[39,40] and force myography[41,42], while techniques such as ultrasound imaging[25], resistive sensing[26] and electrical impedance tomography[27] capture muscle deformations. The current framework could thus be enhanced by integrating these complementary modalities, either for force/displacement sensing or both. In this work, upper-limb single-joint motions serve as a foundational demonstration of the coupled sensing paradigm. Future studies will extend this to complex and realistic scenarios, such as distributed sensor networks monitoring multi-joint dynamic movements of running and jumping with high energy expenditure, as well as long-term monitoring requiring robustness to sweat and temperature variations. Scaling to multi-joint and lower-limb applications, such as rehabilitation engineering, sports science and human-centered robotics, will demand adaptive sensor fusion architectures and advanced coupling strategies for force-displacement sensing, particularly under extreme dynamic conditions such as heavy loads, sustained activity or intense impulses, necessitating further methodological refinements.
CONCLUSIONS
In conclusion, the coupled neural-mechanical sensing method has been developed with the muscle excitation-contraction signatures. Unlike existing coupled sensing limited to motion pattern recognition, the proposed method enables quantitative estimation of individual muscle work through explicit closed-form integration, facilitated by the in-situ real-time soft wearable sensing approach. This study has established a foundational framework for muscle work monitoring, with transformative potential for rehabilitation engineering, sports science and human-centered robotics.
DECLARATIONS
Authors’ contributions
Conceived the concept, designed the research, and supervised the project: Guo, J.; Xiong, C.
Formulated the method, designed the experiments and analyzed the results: Guo, J.; Tong, Y.
Developed the capacitive sensing component: Guo, C.; Liu, Y.
Developed the coupled electrode: Tong, Y.; Yin, H.
Conducted the experiments: Tong, Y.; Guo, C.; Liu, Z.
Wrote and revised the manuscript: Guo, J.; Tong, Y.; Guo, C.
Discussed and commented on the manuscript: Li, Z.; Wu, H.
Availability of data and materials
Detailed experimental materials and methods are described in this article and its Supplementary Materials. Additional raw data supporting the research findings can be obtained from the corresponding author upon reasonable request.
Financial support and sponsorship
The authors gratefully acknowledge the financial support from the National Natural Science Foundation of China (51875221, U22A20249, 52188102, 92248304, 52027806).
Conflicts of interest
All authors declared that there are no conflicts of interest.
Ethical approval and consent to participate
Approved by the Ethics Committee of Tongji Medical College, Huazhong University of Science and Technology (Approval No. S212), this study adheres to the Declaration of Helsinki. All participants provided informed consent, with ethical oversight maintained for protocol execution, data security, and adverse event reporting throughout the research.
Consent for publication
Not applicable.
Copyright
© The Author(s) 2025.
Supplementary Materials
REFERENCES
1. Hargreaves, M.; Spriet, L. L. Skeletal muscle energy metabolism during exercise. Nat. Metab. 2020, 2, 817-28.
2. Gorostiaga, E. M.; Granados, C.; Ibáñez, J.; Izquierdo, M. Differences in physical fitness and throwing velocity among elite and amateur male handball players. Int. J. Sports. Med. 2005, 26, 225-32.
3. Granados, C.; Izquierdo, M.; Ibáñez, J.; Ruesta, M.; Gorostiaga, E. M. Are there any differences in physical fitness and throwing velocity between national and international elite female handball players? J. Strength. Cond. Res. 2013, 27, 723-32.
4. Budgett, R. Fatigue and underperformance in athletes: the overtraining syndrome. Br. J. Sports. Med. 1998, 32, 107-10.
6. Collins, S. H.; Wiggin, M. B.; Sawicki, G. S. Reducing the energy cost of human walking using an unpowered exoskeleton. Nature 2015, 522, 212-5.
7. Shepertycky, M.; Burton, S.; Dickson, A.; Liu, Y. F.; Li, Q. Removing energy with an exoskeleton reduces the metabolic cost of walking. Science 2021, 372, 957-60.
8. Meng, Q.; Zeng, Q.; Xie, Q.; et al. Flexible lower limb exoskeleton systems: a review. NeuroRehabilitation 2022, 50, 367-90.
9. Bohm, S.; Mersmann, F.; Santuz, A.; Arampatzis, A. The force-length-velocity potential of the human soleus muscle is related to the energetic cost of running. Proc. Biol. Sci. 2019, 286, 20192560.
10. Tang, X.; Wang, X.; Ji, X.; et al. A wearable lower limb exoskeleton: reducing the energy cost of human movement. Micromachines 2022, 13, 900.
11. Schrock, J. M.; Snodgrass, J. J.; Sugiyama, L. S. Lassitude: the emotion of being sick. Evol. Hum. Behav. 2020, 41, 44-57.
13. Dauncey, M. J. Influence of mild cold on 24 h energy expenditure, resting metabolism and diet-induced thermogenesis. Br. J. Nutr. 1981, 45, 257-67.
14. Scheeren, T. W.; Schober, P.; Schwarte, L. A. Monitoring tissue oxygenation by near infrared spectroscopy (NIRS): background and current applications. J. Clin. Monit. Comput. 2012, 26, 279-87.
15. Binzoni, T.; Cooper, C. E.; Wittekind, A. L.; et al. A new method to measure local oxygen consumption in human skeletal muscle during dynamic exercise using near-infrared spectroscopy. Physiol. Meas. 2010, 31, 1257-69.
16. Lucero, A. A.; Addae, G.; Lawrence, W.; et al. Reliability of muscle blood flow and oxygen consumption response from exercise using near-infrared spectroscopy. Exp. Physiol. 2018, 103, 90-100.
17. Schmitz, R. J.; Westwood, K. C. Knee extensor electromyographic activity-to-work ratio is greater with isotonic than isokinetic contractions. J. Athl. Train. 2001, 36, 384-7.
18. Kojima, T. Force-velocity relationship of human elbow flexors in voluntary isotonic contraction under heavy loads. Int. J. Sports. Med. 1991, 12, 208-13.
19. Shin, D.; Kim, J.; Koike, Y. A myokinetic arm model for estimating joint torque and stiffness from EMG signals during maintained posture. J. Neurophysiol. 2009, 101, 387-401.
20. Lopes, J.; Simão, M.; Mendes, N.; Safeea, M.; Afonso, J.; Neto, P. Hand/arm gesture segmentation by motion using IMU and EMG sensing. Procedia. Manuf. 2017, 11, 107-13.
21. Jiang, S.; Lv, B.; Guo, W.; et al. Feasibility of wrist-worn, real-time hand, and surface gesture recognition via sEMG and IMU sensing. IEEE. Trans. Ind. Inf. 2018, 14, 3376-85.
22. Stanev, D.; Filip, K.; Bitzas, D.; et al. Real-time musculoskeletal kinematics and dynamics analysis using marker- and IMU-based solutions in rehabilitation. Sensors 2021, 21, 1804.
23. Guo, J.; Guo, C.; Zhou, J.; Duan, K.; Wang, Q. Flexible capacitive sensing and ultrasound calibration for skeletal muscle deformations. Soft. Robot. 2023, 10, 601-11.
24. Kim, N.; Lee, J. M.; Moradnia, M.; et al. Biocompatible composite thin-film wearable piezoelectric pressure sensor for monitoring of physiological and muscle motions. Soft. Sci. 2022, 2, 8.
25. Yang, X.; Sun, X.; Zhou, D.; Li, Y.; Liu, H. Towards wearable A-mode ultrasound sensing for real-time finger motion recognition. IEEE. Trans. Neural. Syst. Rehabil. Eng. 2018, 26, 1199-208.
26. Wong, T. H.; Yiu, C. K.; Zhou, J.; et al. Tattoo-like epidermal electronics as skin sensors for human machine interfaces. Soft. Sci. 2021, 1, 10.
27. Sun, B.; Baidillah, M. R.; Darma, P. N.; Shirai, T.; Narita, K.; Takei, M. Evaluation of the effectiveness of electrical muscle stimulation on human calf muscles via frequency difference electrical impedance tomography. Physiol. Meas. 2021, 42, 035008.
28. Cai, P.; Wan, C.; Pan, L.; et al. Locally coupled electromechanical interfaces based on cytoadhesion-inspired hybrids to identify muscular excitation-contraction signatures. Nat. Commun. 2020, 11, 2183.
29. Wang, T.; Zhao, Y.; Wang, Q. A wearable co-located neural-mechanical signal sensing device for simultaneous bimodal muscular activity detection. IEEE. Trans. Biomed. Eng. 2023, 70, 3401-12.
30. Roberts, T. J.; Gabaldón, A. M. Interpreting muscle function from EMG: lessons learned from direct measurements of muscle force. Integr. Comp. Biol. 2008, 48, 312-20.
31. Chhetry, A.; Sharma, S.; Yoon, H.; Ko, S.; Park, J. Y. Enhanced sensitivity of capacitive pressure and strain sensor based on CaCu3Ti4O12 wrapped hybrid sponge for wearable applications. Adv. Funct. Mater. 2020, 30, 1910020.
32. Zhu, K.; Guo, W.; Yang, G.; Li, Z.; Wu, H. High-fidelity recording of EMG signals by multichannel on-skin electrode arrays from target muscles for effective human–machine interfaces. ACS. Appl. Electron. Mater. 2021, 3, 1350-8.
33. Böl, M.; Weikert, R.; Weichert, C. A coupled electromechanical model for the excitation-dependent contraction of skeletal muscle. J. Mech. Behav. Biomed. Mater. 2011, 4, 1299-310.
34. Marco, G.; Alberto, B.; Taian, V. Surface EMG and muscle fatigue: multi-channel approaches to the study of myoelectric manifestations of muscle fatigue. Physiol. Meas. 2017, 38, R27-60.
35. Michalsik, L. B.; Aagaard, P.; Madsen, K. Locomotion characteristics and match-induced impairments in physical performance in male elite team handball players. Int. J. Sports. Med. 2013, 34, 590-9.
36. Póvoas, S. C.; Seabra, A. F.; Ascensão, A. A.; Magalhães, J.; Soares, J. M.; Rebelo, A. N. Physical and physiological demands of elite team handball. J. Strength. Cond. Res. 2012, 26, 3365-75.
37. Cherry, M. S.; Kota, S.; Young, A.; Ferris, D. P. Running with an elastic lower limb exoskeleton. J. Appl. Biomech. 2016, 32, 269-77.
38. Fleischer, C.; Hommel, G. A human-exoskeleton interface utilizing electromyography. IEEE. Trans. Robot. 2008, 24, 872-82.
39. Al-Mulla, M. R.; Sepulveda, F.; Colley, M. A review of non-invasive techniques to detect and predict localised muscle fatigue. Sensors 2011, 11, 3545-94.
40. Petitjean, M.; Maton, B.; Cnockaert, J. C. Evaluation of human dynamic contraction by phonomyography. J. Appl. Physiol. 1992, 73, 2567-73.
41. Zou, P.; Wang, Y.; Cai, H.; et al. Wearable iontronic FMG for classification of muscular locomotion. IEEE. J. Biomed. Health. Inform. 2022, 26, 2854-63.
Cite This Article
How to Cite
Download Citation
Export Citation File:
Type of Import
Tips on Downloading Citation
Citation Manager File Format
Type of Import
Direct Import: When the Direct Import option is selected (the default state), a dialogue box will give you the option to Save or Open the downloaded citation data. Choosing Open will either launch your citation manager or give you a choice of applications with which to use the metadata. The Save option saves the file locally for later use.
Indirect Import: When the Indirect Import option is selected, the metadata is displayed and may be copied and pasted as needed.
About This Article
Copyright
Data & Comments
Data
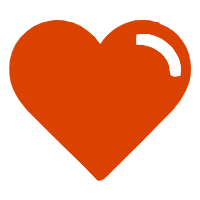
Comments
Comments must be written in English. Spam, offensive content, impersonation, and private information will not be permitted. If any comment is reported and identified as inappropriate content by OAE staff, the comment will be removed without notice. If you have any queries or need any help, please contact us at [email protected].