Precision radiation oncology in head and neck cancer: beyond physical precision - a narrative review
Abstract
Radiotherapy is an integral part of the management of head and neck cancers, both in radical and adjuvant settings. Traditionally, similar radiation dose and fractionation schedules have been used based on tumor stage with variable outcomes indicating “one size does not fit all”. In the era of precision medicine, though we have achieved physical precision with technological advancements, we have yet to attain biologic precision. In the current review, we have highlighted the different aspects of precision oncology such as hypoxia targeting, radiomics and radiogenomics, radiobiologic targeting, and big data. The review also discusses various potential therapeutic targets and approaches in head and neck cancer management that might help to increase radiosensitization, which in turn increase survival, and quality of life. This can be incorporated into the armamentarium of radiation oncology in all the phases of radiation planning, from diagnosis to treatment to the prognosis and management of long-term side effects. Biologic precision can be applied in the clinic to provide individualized, personalized treatment in the future.
Keywords
INTRODUCTION
Since the discovery of X-rays by Wilhelm Roentgen in 1895, the clinical use of radiotherapy has evolved significantly[1]. The introduction of computed tomography (CT) scan in the 1980s has helped in visualizing the tumor 3-dimensionally (3D) instead of bony landmark 2-dimension (2D) and thus effective planning of radiotherapy in head and neck cancer (HNC). Since then, there have been progressive technological advancements in radiation oncology with the advent of intensity-modulated radiotherapy (IMRT) in the 1990s, image-guided radiotherapy (IGRT), stereotactic radiotherapy (SRT), particle radiotherapy, and adaptive radiotherapy. All these high-precision techniques are aimed at improving the therapeutic ratio, targeted delivery of very high doses, and reducing doses to the organs at risk (OAR), thus improving the outcome and providing better quality of life in HNC patients.
Even though anatomic or physical precision was achieved in the 21st century, still the whole world is preaching precision oncology in radiotherapy. Despite the advances, there continues to be a significant failure which may be due to unpredictable biological behavior of similar tumors in different individuals. Patients with the same stage and disease at the same site respond differently to the same treatment modality, reaffirming “one size does not fit all”. Hence, requiring more to be integrated into the treatment approach than what is presently practiced. This has attracted the investigators to think and go beyond physical precision and attempt to incorporate biological precision. Precision oncology holds the promise of tailoring the spectrum of cancer care from diagnosis to risk assessment, predicting the outcome, and personalization of cancer care.
In this narrative review, we aim to highlight the different aspects of precision oncology in the present era, such as targeting hypoxia, incorporation of imaging as data and predictive models, radiobiologic targeting, and finally “Big Data” in achieving personalized precision therapy.
HYPOXIA TARGETING
Locoregional failure (LRF) is the major cause of disease progression and death in HNC patients. Hypoxia is one of the important tumor micro-environmental components associated with radioresistance, tumor progression, and metastasis, which poses a great clinical challenge as well as an attractive target for therapeutic manipulation in HNC. The impact of hypoxia has been known since the 1950s and there is extensive evidence of its impact on clinical outcomes[2,3].
Although the mechanism of hypoxia-induced radioresistance has been described in different models, it is more complex than what is understood. The normal stem cell niche not only has the capability to tolerate and survive hypoxia but also requires hypoxia for survival[4-6]. The tumor cells are generally considered to be more hypoxic than normal stem cells[7,8]. The same mechanism of survival of cancer stem cells can be replicated from normal cells. This is by the mechanism of “radical competition”. The low oxygen tension protects the stem cell DNA damage from free oxygen radicals and the DNA radicals are reduced by hydrogen donation from the thiol compound, thus leading to survival and radiation protection[9]. There are several hypoxic-induced responsive mechanisms, which not only decrease the effectiveness of therapeutic radiation but also negatively influence the outcomes of chemotherapeutics and radiosensitizers. These mechanisms/molecules include hypoxia-inducible factor (HIF)-1α based mechanisms, hypoxia-induced reprogramming of energy metabolism, heat shock transcription factor 1(HSF1)-mediated heat stress response, and heat shock proteins (HSPs), endoplasmic reticulum stress, glucose-regulated proteins (GRPs), hypoxia-induced autophagy, hypoxia-induced formation of the radioresistant cancer stem cells (CSC) phenotype, hypoxia-Responsive microRNAs, long non-coding RNAs and circular RNAs and hypoxia-induced exosome generation. The hypoxic areas are more prone to develop into Epithelial Mesenchymal Transition (EMT), where mobile invasive cancer stem cells invade the vessels and migrate to other organs, leading to distant metastasis. It is the site where cancer cells increase their invasiveness, motility, potential for metastases, stemness, and chemo-radio resistance. Hypoxia-adapted cancer cells are resistant to low as well as high linear energy transfer (LET) radiation due to certain phenotypes with specific regulation[10].
Despite the mechanism and effect of hypoxia being understood, the quantification of tumor hypoxia (TH) poses a diagnostic challenge for therapeutic manipulation. Different methods like polarographic technique, bioreductive agents, determination of HIF-1α, positron emission tomography (PET-CT) with hypoxic tracers (18F-labelled misonidazole (FMISO), fluoroazomycin arabinoside (FAZA), blood oxygen level-dependent magnetic resonance imaging (BOLD-MRI) have been used for determining tumor hypoxia. However, there is no standard, most acceptable method of determining hypoxia, leading to decreased reliability in its determination. MicroRNAs (miRNAs) are short, endogenous non-coding RNAs found to be involved in the regulation of many biological processes such as cellular proliferation, development, cell death, metabolism, and oncogenesis. These miRNAs may help to determine hypoxia[11]. Of the 11 different miRNAs dysregulated in HNC, miR-210 is the only miRNA consistently induced and regulated by hypoxia and hence can be used as a predictor of hypoxia[12,13].
Another novel approach to detecting hypoxia and predicting response to hypoxic modification is hypoxic gene signature profiling. Toustrup et al. developed a 15-gene hypoxic classifier for predicting the impact of hypoxic modification, which has been validated in the Danish Head and Neck Cancer Group (DAHANCA) 5 study cohort[14,15]. Another study of 26-gene hypoxia signatures in laryngeal cancer patients reported improved benefits with accelerated radiotherapy with carbogen and nicotinamide (ARCON) in tumors with higher hypoxia scores than those with lower scores[16]. Recently, P4HA1 has been proven to be a single gene surrogate of hypoxic signature in oral squamous cell carcinoma[17]. Different hypoxia markers can be detected in biopsy or resected specimens. The markers can be either endogenous such as HIF-1α, carbonic anhydrase IX (CAIX), glucose transporter 1 (GLUT-1), and vascular endothelial growth factor (VEGF) or exogenous such as pimonidazole and EF5, overexpression of which is associated with poor response/treatment failure and thus poor prognosis in HNC patients.
Targeting hypoxia may lead to improved outcomes in HNC. Different strategies have been developed for targeting hypoxia, including vascular endothelial growth factor (VEGF) inhibitors, hyperthermia, hyperbaric oxygenation (HBO), erythropoietin stimulating agents, agents targeting tumor blood flow (pentoxifylline), use of bioreductive drugs, hypoxic cytotoxins, ARCON therapy and PR-104, CP-506. However, none of the methods have been widely accepted for clinical use as the basic idea of these methods is improving oxygen delivery[18,19]. However, there are some clinically useful and applicable strategies to target hypoxia, which are shown in Table 1. Phase II trials on ARCON reported it as the most promising therapy in improving tumor control of bladder and HN cancer[20,21]. A phase III randomized study showed that ARCON improves locoregional control and disease-free survival (DFS) in HNC patients with low expression of epidermal growth factor receptor (EGFR)[21-23]. Among the radiosensitizers, nimorazole is the only agent that has been adopted for clinical use, and that too only in Denmark. The confirmatory study, DAHANCA 29-EORTC 1219, done outside Denmark, to prove the efficacy of nimorazole in stage III-IV laryngeal, hypopharyngeal or p16-negative oropharyngeal SCC was not successful. The trial was closed prematurely due to the low conditional power associated with the hypothesized treatment effect. The 2-year locoregional control probability was not clinically different either in the entire population or in the subgroup of hypoxic-gene-positive patients[24]. However, DAHANCA 30, an ongoing non-inferiority trial, has gone one step further to evaluate the impact of hypoxia profile guided hypoxic modification with nimorazole in head and neck cancers (Clinicaltrials.gov Identifier: NCT02661152). Similarly, DAHANCA 33 is evaluating functional image-guided dose-escalated radiotherapy in patients with hypoxic squamous Cell carcinoma of the head and neck (Clinical trial.gov Identifier: NCT02976051). So, hypoxia can be used as a potential therapeutic target in improving the outcomes through hypoxia modification in HNC patients treated with radiotherapy in the future. Recently, several molecular strategies have been proposed for hypoxia modification through direct or indirect modification of HIF-1α expression[25]. S-2-amino-3-(4′-N, N,-bis[2-chloroethyl] amino) phenyl propionic acid N-oxide dihydrochloride (PX-478) is one of the molecules assessed in phase I trials for direct modification of HIF-1α expression through the inhibition of glucose metabolism[26,27]. Phosphatidylinositol 3-kinase (PI3K)/protein kinase B (Akt)/mammalian target of rapamycin (mTOR) signaling axis and Janus kinase/signal transducer and activator of transcription (STAT) signaling pathway can be a target for hypoxia therapy through indirect regulation of HIF-1α[28]. In head and neck malignancies, the phosphorylation and concurrent HIF-1α expression induce STAT3 activation. This activation can be inhibited by STAT3 inhibitor static, leading to suppression of tumor and enhanced tumor radio-sensitivity[29]. A DAHANCA 29 EORTC 1219 study data fails to show any significant benefits of Nimorazole in the whole population, or in the sub-group with hypoxic gene signature[24].
Selective strategies to target hypoxia in head and neck carcinoma
Strategy | Targeted mechanism/molecular target | Clinical trials [n] | Clinical outcome | Toxicity |
HBOT (Hyperbaric Oxygen Therapy) | Physical increase in O2 supply by enhanced amount of dissolved O2 | Benette et al.[31] Meta-Analysis [19 Clinical trials] | Reduced risk of death at 1 year and 5 year (RR = 0.83) Decrease local recurrence (RR = 0.66) | 1. Claustrophobia 2. Increased risk of severe radiation tissue injury (RT + HBOT) 3. Risk of oxygen toxicity seizures |
TPZ (Tirapazemine) | DNA strands breaks; HAP | Rischin et al.[32] (TROG 02.02, Head START) | No difference in failure-free survival/time to locoregional failure | More hematologic and GI toxicities in TPZ arm |
ARCON | Carbogen (95% O2; 5% CO2) breathing can reduce chronic hypoxia Accelerated radiotherapy can counteract tumor cell repopulation Nicotinamide can decrease acute hypoxia | Kaanders et al.[21] [215 patients] | 1. High local and regional control rates 2. For T3-T4 tumors, LC rate 80% for larynx 60% for hypopharynx, 87% for oropharynx, 29% for oral cavity 3. Regional Control rate 100% for N0, 93% for N1, 74% for N2 disease | 1. Acceptable acute and late morbidity 2. Grade 4 morbidities developed in 5% of patients |
Janssens et al.[23] [345 patients] | Improved 5-year LRC (79% vs. 53%; DFS (68% vs. 45%; P = 0.04) in patients with low pretreatment hemoglobin levels | 1. Acceptable toxicities 2. Patients' stratification is required; especially useful for anemic patients | ||
Nimorazole | Oxygen mimetic | Toustrup et al.[33] (DAHANCA 5; phase III) [323 patients] | Improved 5year LRC (49% vs. 18%; DSS (48% vs. 30%; P = 0.04) in more head and neck cancer with hypoxia | Minor nausea and vomiting |
Nelfinavir | Decreased HIF-1α and VEGF levels, suppresses angiogenesis, and suppresses Akt signaling | Hoover et al.[34] [15 patients] | No meaningful improvement in patients with recurrent ACC | 1. Grade 3 or higher medical adverse events or toxicities experienced by 33% of the patients requiring dose modification 2. Radio-sensitizing effect |
Despite the aforesaid strengths and potential use of hypoxia targeting, several caveats and limitations still remain. To effectively target hypoxia, several requirements must be met, including the use of expensive dedicated equipment, repeated biopsies, multiple injections at tumoral sites, exogenous drug administration, and the implantation of particulate paramagnetic materials. We need to validate the usefulness of these various biomarkers in large clinical trials[30]. There are clinical trials which study those selective Strategies to target hypoxia in Head and Neck Carcinoma such as using HBOT (Hyperbaric Oxygen Therapy)[31], tirapazemine[32], ARCON (Accelerated Radiotherapy with carbogen and Nicotinamide)[21,23], Nimorazole[33] and Nelfinavir[34] [Table 1].
RADIOMICS
HNC poses a major challenge in radiation oncology due to the anatomic location of a target, surrounding critical normal structure and the wide heterogeneity in the tumor as well as the radio sensitivity. HNC, like all other solid cancers, have significant temporal and spatial heterogeneity within tumors of the same site and stage, which may be the key to varying responses to the same treatment. The heterogeneity is attributed to the different subpopulations of cells within the tumor with differential growth kinetics, microvasculature, expression of biomarkers, immunological characteristics, genetic profile, and tumor microenvironment[35,36]. This heterogeneity leads to phenotypic heterogeneity, which can likely cause treatment resistance, progression, and metastasis[37]. Usually, biopsies provide genetic and phenotypic information, but with several limitations. Biopsy samples collected represent a specific subpopulation of cells within the tumor taken at a specific time point, and this procedure involves an invasive technique. It does not provide adequate pathophysiological and genetic information on the entire tumor. Conversely, radiomics, an emerging field of precision oncology, is a non-invasive representation of the virtual biopsy of the entire tumor in all 3 dimensions, providing integrative information on genetic alteration and phenotypic characteristics[38]. Thus, radiomics will help us with the implementation of personalized treatment. Biomedical imaging such as CT, Magnetic Resonance Imaging (MRI) and PET has the potential for unraveling the genotypic characteristics hidden behind the phenotypic expression and predicting the outcome[39-42]. Radiomics helps in the extraction of high throughput quantitative tumor heterogeneity data from these images, such as intensity, shape, texture, and wavelet, using a data-characterization algorithm and thus supporting and enhancing the clinical decision-making process[40,43].
Radiomics has its place in different steps of HNC, from diagnosis and prognosis to therapy response assessment/prediction [Table 2]. Texture analysis can accurately describe the tumor phenotype in HNC. CT radiomics can distinguish between human papillomavirus (HPV) positive and negative oropharyngeal cancer (OPC) without the need for immune histochemistry and in-situ hybridization[44]. Even it can distinguish between HPV-positive and negative non-oropharyngeal cancer[45]. PET CT can also be reliably used as another non-invasive modality for classifying HNC based on HPV status[46]. Radiomics can provide insight into the tumor microenvironment. Tumor resistance to radiation is the worst nightmare of the radiation oncologist and hypoxia is one major culprit for the resistance. PET-labeled nitroimidazole compounds can precisely locate the spatial distribution of hypoxic sub-volumes within the tumor and thus provide an opportunity to escalate doses to those specific sub-volumes and a promise for better local control[47-50]. Accurate prognostic and predictive models in radiomics are vital steps in widening its application to clinical oncology.
Selected studies on applicability of radiomics in radiotherapy planning of head and neck cancer
Step | Study [n] | No of patients (n) | Sites | Features studied | Outcome prediction |
Pretreatment | |||||
HPV Status OPC | Buch et al.[44] | 40 | Oropharyngeal cancer | Extracted 42 texture features 13 histogram features, 5 gray-level co-occurrence matrix (GLCM) features, 11 gray-level run-length (GLRL) features, 4 gray-level gradient matrix (GLGM) features, and 9 Law's features | Significant difference in histogram parameters median (P = 0.006), entropy (P = 0.016), squamous cell carcinoma entropy (P = 0.043) based on HPV status |
Non-OPC | Fujita et al.[45] | 46 | Oral cavity, Larynx, Hypopharynx (non- OPC) | 42 texture features extracted | Significant differences between (HPV)-positive and HPV-negative non-oropharyngeal carcinoma (non-OPC) for 5 histogram features(P ≤ 0.03), 3 GLCM features(P ≤ 0.02), 1 GLRL features (P = 0.009), 2 GLGM features(P ≤ 0.02) and 5 law features (P ≤ 0.04) |
Extra-nodal extension | Kann et al.[61] | 200# Lymph nodes | HN | ENE on pretreatment computed tomography (CT) imaging is identified by A deep learning algorithm | The algorithm achieved AUC of 0.9, outperforms the radiologists AUC |
Distant Metastasis | Zhou et al.[62] | - | HN | Multifaceted Radiomics (M-Radiomics) Multiple base classifiers to build model | Outperforms current radiomic models in predicting distant metastasis |
Prognosis | Aerts et al.[43] | 1,019* | HN | Prognostic radiomic signature, capturing intra-tumor heterogeneity | These features are associated with underlying gene expression patterns. The routinely used imaging can improve decision support in cancer treatment at a low cost |
Radiotherapy planning | |||||
Target volumes Delineation | Lu et al.[63] | 40 | Nasopharynx | 21 FO intensity features, 10 shape features 57 SO and HO textural features | Acceptable reproducibility and stability across manual and various automated segmentation techniques. Discretization generated larger effects on features than segmentation in both tracers. Features extracted from [11C] choline were more robust than [18F] FDG for segmentation |
Organ at risk (OAR) Delineation 21 OAR | Nikolov et al.[64] | 513 | HN | 21 computed tomography scans from clinical practice, each with 21 organs at risk segmented by 2 independent experts Surface Dice similarity coefficient, a new metric for the comparison of organ delineation | Deep learning model’s segmentations and those of radiographers did not show any clinically meaningful difference It will improve the efficiency, consistency, and safety of radiotherapy pathways |
Intra-treatment | |||||
Daily CBCT and pCT | Bagher-Ebadian et al.[65] | 18 | Oropharynx | 165 Textural features extracted, smoothing, sharpening of the images and noise to evaluate changes in features | Interchangeability of pCT and CBCT for investigating radiomics features as possible biomarkers for outcome |
Treatment Verification | Park et al.[66] | 20 + 20 Fluence Map of VMAT | HN+Prostate | Six textural features like angular second moment, inverse difference moment, contrast, variance, correlation, and entropy were calculated for fluence maps of VMAT | Contrast and variance showed considerable correlations (P < 0.003) with the plan deliverability Can be used as an indicator for degree of modulation of VMAT plans and is better than the conventional modulation indices |
Treatment Delivery | Bogowicz et al.[67] | 40 | HN | From Two volumes including GTV-Recurrence and GTV-Control, we had extracted FO, SO and HO features. Bi-regional model was built. Local radiomics was implemented | The AUC of local radiomics for detection of noncontrolled sub-volumes in the training and validation sets was 0.66 (95%CI: 0.56-0.75) and 0.70 (95%CI: 0.53-0.86), respectively Local radiomics can detect sub-volumes with decreased radiosensitivity and dose escalation |
Adaptive planning | Guidi et al.[68] | 40 1,200 MVCT | HN | Support vector machine (SVM) and cluster analysis have identified cases where there are dose and volume discrepancies between fractions in certain treatment sessions | Whenever the patient needs a new dosimetric scan to adapt the volumes in head and neck cancer patients, these automated tools for adaptive treatment help to raise an alert |
Post-treatment | |||||
Survival | Parmar et al.[54] | 101 (Training cohort) 95 (Validation cohort) | HN | 440 features extracted. Different feature selection and classification methods were used, such as Minimum redundancy maximum relevance (MRMR), Mutual information feature selection (MIFS), Conditional infomax feature extraction (CIFE) | MRMR, MIFS, CIFE had high prognostic performance and stability. Identified prognostic and reliable machine-learning methods for the prediction of overall survival of head and neck cancer patients |
Post treatment Toxicity (Xerostomia) | van Dijk et al.[59] | 249 | HN | Patient-specific characteristics, based on CT image biomarkers (IBMs) including geometric, CT intensity and textural characteristics of the parotid and submandibular glands | Prediction of Moderate-to-severe xerostomia and sticky saliva at 12 months was improved by including IBMs representing heterogeneity and density of the salivary glands |
Different PET-CT and CT radiomic studies have been able to predict the risk of failure in different models. In a large study, Aerts et al. extracted 440 radiomic features in four phenotypes (tumor image intensity, texture, shape and wavelet formation) from the CT database of 1,019 patients of different cancers, including HNC, which showed a strong prognostic association of tumor heterogeneity and the underlying gene signature[43]. This radiomic signature was well validated externally in another cohort of 542 oropharyngeal cancer patients[51]. Besides the CT scan, it has been shown that texture analysis of PET-CT is a better predictor of outcome than the mere standardized uptake values (SUV)[52]. Radiomic machine learning classifiers have predicted the risk of local failure and distant metastasis in HNC[53,54]. This provides insights into treatment intensification or de-intensification on an individual basis, allowing for tailoring the treatment. Advanced tumor shape signature radiomics has also been shown to be a predictor of local recurrence in locally advanced HNC, which has also been validated in a cohort of 86 patients from TCGA databases[55].
An important and most challenging issue in head and neck radiotherapy is the critical location of normal organs. Radiomics has the potential to detect the physiologic and functional alteration in normal organs during radiotherapy. In a texture analysis study of nasopharyngeal cancer, Scalco et al. have shown a correlation between the dosimetric parameters and the structural changes in the parotid gland[56]. They have also shown that the early textural changes in the parotid gland can predict the final shrinkage with an accuracy of about 71.4%[57]. A similar study by Pota et al. has predicted parotid shrinkage and 12-month xerostomia from CT radiomics[58]. van Dijk et al. suggested that the mid-treatment changes in the parotid gland are better predictors of late xerostomia as quantified by delta radiomic features[59]. Thus, for the implementation of personalized treatment, radiomics has a critical role to play in different stages of radiotherapy planning, from pretreatment patient section to intratreatment and post-treatment radiomics, which is beyond the scope of this review. However, radiomics-guided radiotherapy (RGRT) may lead to more effective radiation therapy and its usage in radiotherapy is increasing over the years[60]. The radiomics has been applied using different parameters in pretreatment[43-45,61,62], during radiotherapy planning[63,64], during treatment[65-68] and even in posttreatment setting[54] [Table 3].
Selected studies on applicability of big data in head and neck cancer management
Study | No. of patients | Primary | Major findings |
Robertson et al.[87] | 684 | Head and Neck cancer | Data-mining algorithm confirmed the following well-known OAR-dose/outcome relationships: • dysphagia/larynx, • voice changes/larynx, • esophagitis/esophagus, • xerostomia/parotid glands • mucositis/oral mucosa |
Cavalieri et al.[89] | 1,537 | Stage III‐IV HNSCC | Represents the HNSCC largest available repository. It will allow for: • Developing/validating a decision support system integrating multiscale data to explore through classical and machine learning models their prognostic role |
Resteghini et al.[94] | - | Head and Neck cancer | Computational strategies derived from big data science hold the promise • Identifying new prognostic and predictive factors • Discovering potential therapeutics • Identifying new molecular mechanisms driving head and neck cancer pathogenesis |
Hybrid machine learning radiomics also helps in detecting molecular subtypes of Low-Grade Glioma (LGGs) using 726 raw features on MRI[69]. Radiomics-based models like eXtreme Gradient Boosting (XGBoost) help to detect molecular prognostic factors such as 1p/19q codeletion status on MRI[70].
The quantitative approach using radiomics has been widely investigated as a non-invasive and objective imaging biomarker in cancer patients; however, Because of a lack of standardization and validation of image acquisition protocols, features segmentation, extraction, processing, and data analysis, it is not applied as clinical routine practice. In the future, radiomics might be a reliable application in oncologic imaging for each type of cancer[71].
Artificial intelligence (AI), which uses deep machine learning to create “algorithms”, has helped us to study image texture analysis, which is ultimately a part of radiomics. AI will help to facilitate pattern recognition in images, detection in biomarker data, and integration with non-imaging variables, which will flourish the field of radiomics. Though radiomics has greater potential in the field of precision oncology, there are several unmet challenges to be addressed. These include processing and reproducibility of large amounts of high-quality imaging data, application in the clinic and integration of genomics data. But these radiomics features do have some limitations due to their sensitivity to acquisition modes and reconstruction parameters. Hence, all of them are not recommended for use. We should investigate the topic such as the impact of harmonization and standardization on the quantification and predictive values of radiomic features. This still remains a challenging aspect[72].
BIOLOGIC ADAPTATION AND TARGETED THERAPY
Head and neck radiotherapy poses a great challenge subjecting to variation in the planned dose and dose delivered due to the ongoing anatomic changes related to tumor shrinkage and weight loss through the course of treatment delivery. With the advent of IGRT, adaptive radiotherapy (ART) has been the proposed solution to account for these changes with yet-to-be-proven benefits, given the subjective variations in the practice and indications of ART. ART would probably play a prominent role in controlling doses to the OAR without compromising the doses to the target volumes; however, there is significant uncertainty regarding the time of adaptation and is currently subject to physician discretion[73].
Although ART has been practiced for a long time and seems a logical step during radiotherapy based on volumetric (physical) changes, biological adaptation would play a more important role in precision oncology. Several trials have looked at trying to address the biological differences between oropharyngeal tumors based on HPV status and adapt the treatment accordingly. Different methods of dose de-escalation were evaluated in an attempt to treat this favorable group of relatively younger patients to reduce toxicity without compromising treatment-related outcomes. The different strategies include reduction in all the components of triple modality treatment[74].
Besides treatment adaptation based on HPV status, radiobiologic tumor targeting is a new and emerging concept in precision oncology. Genome-based biologic targeting is being used in medical oncology to quantify the benefits of specific agents in specific groups of the population. Genome-adjusted radiation dose (GARD) is a relatively recent concept in radiation oncology, similar to genome-based targeting in medical oncology. Scott et al. used the gene-expression-based radiation-sensitivity index and the linear quadratic model to derive the GARD[75]. The postulation was that high GARD values predict a higher therapeutic effect with radiotherapy, which would relate to clinical outcomes. GARD is a genome-based model developed to predict the optimal radiation dose for a particular tumor using the linear quadratic model and radiosensitivity index; hence, the dose and fractionation schedule can be planned for each individual in the future.
The radiosensitivity index was developed as a molecular marker of cellular survival at 2 Gy[76]. This has been systematically validated as a predictor of clinical outcomes in HNC[77]. This multigene expression model of intrinsic radiosensitivity successfully proved to improve 2-year locoregional control (LRC) in the predicted radiosensitive group (86% vs. 61%, P = 0.05). Hence, the radiosensitive index could be used to segregate those patients who would benefit from radiotherapy vs. those who would not. Another study by Scott et al. demonstrated a wide variation in the radiosensitive index in HNC between the most sensitive and most resistant tumors, which indicates that the one-size-fits-all strategy is no longer applicable in radiation oncology and the radiation dose needs to be tailored in the era of precision oncology[75]. Another aspect of radiobiologic targeted therapy is combining the molecular-targeted agents with radiotherapy. Currently, the two targets for targeted therapy are epidermal growth factor receptor (EGFR) and vascular endothelial growth factor receptor (VEGFR)[78]. Combining the EGFR monoclonal antibody, cetuximab, with radiation showed improved survival in HNC and has been approved by the Food and Drug Administration (FDA). VEGFR tyrosine kinase inhibitor (TKI), vandetanib, has been shown to enhance the antitumor efficacy of radiotherapy and restore the radiosensitivity of HNC cells by increasing the apoptosis and decreasing the microvessel density in HNC cells in preclinical studies[79,80]. A phase II trial is ongoing in advanced HNC to test the efficacy of vandetanib[81]. Similarly, sunitinib, sorafenib and linifanib have shown efficacy in preclinical studies and need to be tested in the future[81-83]. Restoring the p53 function is also another attractive area of manipulation in HNC[84] and several studies are ongoing. Several of these molecular-targeted therapies are under active investigation and the results may improve the effectiveness of current therapeutic strategies.
BIG DATA
Implementation of precision oncology needs the highest quality, evidence-based guidelines for application in patients. The more specific the approach, it decreases the pool of “like patients” to be tested for specific therapies. “Big Data” plays a major role in generating a pool of “like patients”. Big Data is defined as a moving semi-quantitative target, and in computer memory terms, the total amount of data is in terms of terabytes or petabytes or more[85]. The patient data studied is much larger. Different sources of Big Data include the Surveillance, Epidemiology and End Results (SEER) database, the American College of Surgeon’s National Cancer Database, other national cancer databases and different large completed clinical trials[86]. Most clinicians make decisions based on outcomes of available randomized control trials (RCTs), and patients and relatives are informed about the relative effectiveness of specific modalities over others based on the trial results. However, it may not always be possible to conduct large RCTs to answer clinical questions in a timely manner. Therefore, Big Data can be utilized for retrospective data analysis with large patient cohorts and at a faster rate. Data from large sources of Big Data can be generalized to the general population and can benefit the patients early and much before RCT proves the benefit. The benefit may be in terms of treatment adoption, improved efficacy, and fewer logistic issues. Big Data can overcome the problem of a smaller number of “like patients” when testing specific targeted therapy in these groups of patients. The result would be treating a smaller number of patients with specific therapy and sparing a large cohort of patients from unnecessary, ineffective treatments.
Big Data can be utilized for personalizing treatment plans for individual patients in radiation oncology. The quality and efficiency of treatment planning can be improved by previous patient treatment plans. Initiatives have been taken for collating large-scale data from dose-volume histograms for correlating and predicting toxicity[87,88]. Big Data provides greater hope for predicting normal tissue toxicities beyond the normal tissue complication probability (NTCP) models. To use multiparametric variables for predicting prognosis and guiding the optimal choice of treatment, the international European Consortium has built Bigdata to Decide (BD2Decide) project (“Big Data and Models for Personalized Head and Neck Cancer Decision (BD2Decide) Support; NCT02832102). This represents the largest available HNSCC repository of 1,537 patients with transcriptomic and radiomic data available for 1,284 (83%) and 1,239 (80%) of the patients. For patients who were enrolled prospectively, data were collected using quality of life questionnaires (QLQ) such as EORTC QLQ 30, EORTC HN 35, and EQ-5D-5L[89]. Big Data also plays a major role in basic and clinical research; the findings of Big Data research will reach the clinic in the coming 5-10 years[90]. The BD4QoL (Big Data for Quality of Life in Head and Neck Cancer), an ongoing study, aims to reduce and anticipate the proportion of HNC survivors experiencing a clinically meaningful reduction of QoL (Clinicaltrials.gov Identifier: NCT05315570). Though big data is not being routinely used in HNC research, specific applications like radiomics and genomics have leapt in recent years.
The features of data sets such as complexity, volume, velocity variety, and veracity determine the value of big data. We need to integrate these sources in order to improve biomedical research, patient care and monitoring the quality of care[91]. Big data is playing a big role in different steps of HNC radiation planning such as, OAR-dose/outcome relationships, identifying new prognostic and predictive factors and discovering potential therapeutics [Table 3].
Big Data requires the collection of large amounts of data from the patient at each patient encounter. It requires consent from the patients and their family, and a large effort to collect the data correctly from the patients and make them understand the need for it. This may also affect the patient-physician relationship as it puts their health information system in the public domain. These are the major challenges for big data[92]. These procedures call for different aspects of addressing ethical questions regarding patient information.
The large database has design and analysis issues such as selection bias, immortal time bias and several confounding factors during data collection[93]. Although Big Data poses some challenges to uniform data collection, it will provide a greater advantage of delivering better care for patients on an individual basis, extending patients’ life expectancy and enhancing the quality of their years. The radiation oncologist will be able to use clinical, imaging, dosimetric, and genomic analyses for treatment recommendations, leading to a knowledge-guided radiotherapy approach and may become an indispensable component of precision oncology in the years to come.
CONCLUSION
Patients with the same TNM stage often have variable responses to the same therapy, which can be attributed to the large heterogeneity within the tumors of similar histology and even within a single tumor. Incorporation of precision and personalized medicine into clinical practice would be of great promise in improving the outcomes of HNC. Though there are lots of limitations and challenges in implementation, there is still a need to move beyond mere physical precision and adapt to biological precision with the application of hypoxia modification, radiomics, genomics, biologic treatment adaptation and Big Data whenever feasible.
DECLARATIONS
Acknowledgement
We are grateful to Yasam Venkata Ramesh, PhD, for his invaluable help in the language and draft editing of this manuscript.
Authors’ contributions
Contributions to conception and design of the study: Swain M, Ghosh-Laskar S, Patil R
Literature search: Swain M, Patil R
Manuscript preparation: Swain M, Ghosh-Laskar S, Patil R, BudrukkarA, Agarwal JP
Manuscript editing: Swain M, Ghosh-Laskar S, Patil R, BudrukkarA, Agarwal JP
Availability of data and materials
Not applicable.
Financial support and sponsorship
None.
Conflicts of interest
All authors declared that there are no conflicts of interest.
Ethical approval and consent to participate
Not applicable.
Consent for publication
Not applicable.
Copyright
© The Author(s) 2023.
REFERENCES
1. Röntgen, Ueber eine neue art von Strahlen, Wurzburg, 1896. Available from: https://www.sothebys.com/buy/e1f1e6ca-ae21-4a47-b628-71aea6ab12e6/lots/feb988bf-f908-49c1-bfea-4687612640df [Last accessed on 28 Aug 2023].
2. Gray LH, Conger AD, Ebert M, Hornsey S, Scott OC. The concentration of oxygen dissolved in tissues at the time of irradiation as a factor in radiotherapy. Br J Radiol 1953;26:638-48.
3. Vaupel P, Mayer A. Hypoxia in cancer: significance and impact on clinical outcome. Cancer Metastasis Rev 2007;26:225-39.
4. Ejtehadifar M, Shamsasenjan K, Movassaghpour A, et al. The effect of hypoxia on mesenchymal stem cell biology. Adv Pharm Bull 2015;5:141-9.
5. Hawkins KE, Sharp TV, McKay TR. The role of hypoxia in stem cell potency and differentiation. Regen Med 2013;8:771-82.
6. Imanirad P, Dzierzak E. Hypoxia and HIFs in regulating the development of the hematopoietic system. Blood Cells Mol Dis 2013;51:256-63.
7. Höckel M, Vaupel P. Tumor hypoxia: definitions and current clinical, biologic, and molecular aspects. J Natl Cancer Inst 2001;93:266-76.
8. Vaupel P, Kallinowski F, Okunieff P. Blood flow, oxygen and nutrient supply, and metabolic microenvironment of human tumors: a review. Cancer Res 1989;49:6449-65.
9. Biologic basis of radiation oncology. 2015. Available from: https://clinicalgate.com/biologic-basis-of-radiation-oncology/ [Last accessed on 28 Aug 2023].
10. Kabakov AE, Yakimova AO. Hypoxia-induced cancer cell responses driving radioresistance of hypoxic tumors: approaches to targeting and radiosensitizing. Cancers 2021;13:1102.
11. Ameres SL, Zamore PD. Diversifying microRNA sequence and function. Nat Rev Mol Cell Biol 2013;14:475-88.
12. Chen D, Cabay RJ, Jin Y, et al. MicroRNA deregulations in head and neck squamous cell carcinomas. J Oral Maxillofac Res 2013;4:e2.
14. Toustrup K, Sørensen BS, Alsner J, Overgaard J. Hypoxia gene expression signatures as prognostic and predictive markers in head and neck radiotherapy. Semin Radiat Oncol 2012;22:119-27.
15. Toustrup K, Sørensen BS, Nordsmark M, et al. Development of a hypoxia gene expression classifier with predictive impact for hypoxic modification of radiotherapy in head and neck cancer. Cancer Res 2011;71:5923-31.
16. Eustace A, Mani N, Span PN, et al. A 26-gene hypoxia signature predicts benefit from hypoxia-modifying therapy in laryngeal cancer but not bladder cancer. Clin Cancer Res 2013;19:4879-88.
17. Kappler M, Kotrba J, Kaune T, et al. P4HA1: a single-gene surrogate of hypoxia signatures in oral squamous cell carcinoma patients. Clin Transl Radiat Oncol 2017;5:6-11.
18. Kaanders JH, Bussink J, van der Kogel AJ. Clinical studies of hypoxia modification in radiotherapy. Semin Radiat Oncol 2004;14:233-40.
19. Vaupel P. Tumor microenvironmental physiology and its implications for radiation oncology. Semin Radiat Oncol 2004;14:198-206.
20. Hoskin PJ, Rojas AM, Phillips H, Saunders MI. Acute and late morbidity in the treatment of advanced bladder carcinoma with accelerated radiotherapy, carbogen, and nicotinamide. Cancer 2005;103:2287-97.
21. Kaanders JH, Pop LA, Marres HA, et al. ARCON: experience in 215 patients with advanced head-and-neck cancer. Int J Radiat Oncol Biol Phys 2002;52:769-78.
22. Nijkamp MM, Span PN, Terhaard CH, et al. Epidermal growth factor receptor expression in laryngeal cancer predicts the effect of hypoxia modification as an additive to accelerated radiotherapy in a randomised controlled trial. Eur J Cancer 2013;49:3202-9.
23. Janssens GO, Rademakers SE, Terhaard CH, et al. Improved recurrence-free survival with ARCON for anemic patients with laryngeal cancer. Clin Cancer Res 2014;20:1345-54.
24. Grégoire V, Tao Y, Kaanders J, et al. OC-0278 Accelerated CH-RT with/without nimorazole for p16- HNSCC: the randomized DAHANCA 29-EORTC 1219 trial. Radiother Oncol 2021;161:S187-8.
26. Welsh S, Williams R, Kirkpatrick L, Paine-Murrieta G, Powis G. Antitumor activity and pharmacodynamic properties of PX-478, an inhibitor of hypoxia-inducible factor-1alpha. Mol Cancer Ther 2004;3:233-44.
27. Xia Y, Choi HK, Lee K. Recent advances in hypoxia-inducible factor (HIF)-1 inhibitors. Eur J Med Chem 2012;49:24-40.
28. Aebersold DM, Burri P, Beer KT, et al. Expression of hypoxia-inducible factor-1alpha: a novel predictive and prognostic parameter in the radiotherapy of oropharyngeal cancer. Cancer Res 2001;61:2911-6.
29. Adachi M, Cui C, Dodge CT, Bhayani MK, Lai SY. Targeting STAT3 inhibits growth and enhances radiosensitivity in head and neck squamous cell carcinoma. Oral Oncol 2012;48:1220-6.
30. Le QT, Courter D. Clinical biomarkers for hypoxia targeting. Cancer Metastasis Rev 2008;27:351-62.
31. Bennett MH, Feldmeier J, Smee R, Milross C. Hyperbaric oxygenation for tumour sensitisation to radiotherapy. Cochrane Database Syst Rev 2018;4:CD005007.
32. Rischin D, Peters LJ, O’Sullivan B, et al. Tirapazamine, cisplatin, and radiation versus cisplatin and radiation for advanced squamous cell carcinoma of the head and neck (TROG 02.02, HeadSTART): a phase III trial of the Trans-Tasman Radiation Oncology Group. J Clin Oncol 2010;28:2989-95.
33. Toustrup K, Sørensen BS, Lassen P, Wiuf C, Alsner J, Overgaard J. Gene expression classifier predicts for hypoxic modification of radiotherapy with nimorazole in squamous cell carcinomas of the head and neck. Radiother Oncol 2012;102:122-9.
34. Hoover AC, Milhem MM, Anderson CM, et al. Efficacy of nelfinavir as monotherapy in refractory adenoid cystic carcinoma: results of a phase II clinical trial. Head Neck 2015;37:722-6.
35. Fisher R, Pusztai L, Swanton C. Cancer heterogeneity: implications for targeted therapeutics. Br J Cancer 2013;108:479-85.
36. Heppner GH, Miller BE. Tumor heterogeneity: biological implications and therapeutic consequences. Cancer Metastasis Rev 1983;2:5-23.
38. Gerlinger M, Rowan AJ, Horswell S, et al. Intratumor heterogeneity and branched evolution revealed by multiregion sequencing. N Engl J Med 2012;366:883-92.
39. Gillies RJ, Kinahan PE, Hricak H. Radiomics: images are more than pictures, they are data. Radiology 2016;278:563-77.
40. Lambin P, Rios-Velazquez E, Leijenaar R, et al. Radiomics: extracting more information from medical images using advanced feature analysis. Eur J Cancer 2012;48:441-6.
41. Yang X, Knopp MV. Quantifying tumor vascular heterogeneity with dynamic contrast-enhanced magnetic resonance imaging: a review. J Biomed Biotechnol 2011;2011:732848.
42. Basu S, Kwee TC, Gatenby R, Saboury B, Torigian DA, Alavi A. Evolving role of molecular imaging with PET in detecting and characterizing heterogeneity of cancer tissue at the primary and metastatic sites, a plausible explanation for failed attempts to cure malignant disorders. Eur J Nucl Med Mol Imaging 2011;38:987-91.
43. Aerts HJ, Velazquez ER, Leijenaar RT, et al. Decoding tumour phenotype by noninvasive imaging using a quantitative radiomics approach. Nat Commun 2014;5:4006.
44. Buch K, Fujita A, Li B, Kawashima Y, Qureshi MM, Sakai O. Using texture analysis to determine human papillomavirus status of oropharyngeal squamous cell carcinomas on CT. AJNR Am J Neuroradiol 2015;36:1343-8.
45. Fujita A, Buch K, Li B, Kawashima Y, Qureshi MM, Sakai O. Difference between HPV-positive and HPV-negative non-oropharyngeal head and neck cancer: texture analysis features on CT. J Comput Assist Tomogr 2016;40:43-7.
46. Vallieres M, Kumar A, Sultanem K, El Naqa I. FDG-PET image-derived features can determine hpv status in head-and-neck cancer. Int J Radiat Oncol Biol Phys 2013;87:S467.
47. Horsman MR, Mortensen LS, Petersen JB, Busk M, Overgaard J. Imaging hypoxia to improve radiotherapy outcome. Nat Rev Clin Oncol 2012;9:674-87.
48. Servagi-Vernat S, Differding S, Sterpin E, et al. Hypoxia-guided adaptive radiation dose escalation in head and neck carcinoma: a planning study. Acta Oncol 2015;54:1008-16.
49. Thorwarth D, Alber M. Implementation of hypoxia imaging into treatment planning and delivery. Radiother Oncol 2010;97:172-5.
50. Jeong J, Setton JS, Lee NY, Oh JH, Deasy JO. Estimate of the impact of FDG-avidity on the dose required for head and neck radiotherapy local control. Radiother Oncol 2014;111:340-7.
51. Leijenaar RT, Carvalho S, Hoebers FJ, et al. External validation of a prognostic CT-based radiomic signature in oropharyngeal squamous cell carcinoma. Acta Oncol 2015;54:1423-9.
52. Hatt M, Majdoub M, Vallières M, et al. 18F-FDG PET uptake characterization through texture analysis: investigating the complementary nature of heterogeneity and functional tumor volume in a multi-cancer site patient cohort. J Nucl Med 2015;56:38-44.
53. Zhang B, He X, Ouyang F, et al. Radiomic machine-learning classifiers for prognostic biomarkers of advanced nasopharyngeal carcinoma. Cancer Lett 2017;403:21-7.
54. Parmar C, Grossmann P, Rietveld D, Rietbergen MM, Lambin P, Aerts HJ. Radiomic machine-learning classifiers for prognostic biomarkers of head and neck cancer. Front Oncol 2015;5:272.
55. Reuz S, Limkin E, Ou D, et al. A radiomic signature based on advanced tumor shape parameters (Spiculated-ness) to predict the outcome of locally advanced (LA) HNSCC patients. J Clin Oncol 2016;34:11571.
56. Scalco E, Fiorino C, Cattaneo GM, Sanguineti G, Rizzo G. Texture analysis for the assessment of structural changes in parotid glands induced by radiotherapy. Radiother Oncol 2013;109:384-7.
57. Scalco E, Moriconi S, Rizzo G. Texture analysis to assess structural modifications induced by radiotherapy. Annu Int Conf IEEE Eng Med Biol Soc 2015;2015:5219-22.
58. Pota M, Scalco E, Sanguineti G, et al. Early prediction of radiotherapy-induced parotid shrinkage and toxicity based on CT radiomics and fuzzy classification. Artif Intell Med 2017;81:41-53.
59. van Dijk LV, Langendijk JA, Zhai TT, et al. Delta-radiomics features during radiotherapy improve the prediction of late xerostomia. Sci Rep 2019;9:12483.
60. Abdollahi H, Chin E, Clark H, et al. Radiomics-guided radiation therapy: opportunities and challenges. Phys Med Biol 2022;67:12TR02.
61. Kann BH, Hicks DF, Payabvash S, et al. Multi-institutional validation of deep learning for pretreatment identification of extranodal extension in head and neck squamous cell carcinoma. J Clin Oncol 2020;38:1304-11.
62. Zhou Z, Wang K, Folkert M, et al. Multifaceted radiomics for distant metastasis prediction in head & neck cancer. Phys Med Biol 2020;65:155009.
63. Lu L, Lv W, Jiang J, et al. Robustness of radiomic features in [11C]choline and [18F]FDG PET/CT imaging of nasopharyngeal carcinoma: impact of segmentation and discretization. Mol Imaging Biol 2016;18:935-45.
64. Nikolov S, Blackwell S, Zverovitch A, et al. Clinically applicable segmentation of head and neck anatomy for radiotherapy: deep learning algorithm development and validation study. J Med Internet Res 2021;23:e26151.
65. Bagher-Ebadian H, Siddiqui F, Liu C, Movsas B, Chetty IJ. On the impact of smoothing and noise on robustness of CT and CBCT radiomics features for patients with head and neck cancers. Med Phys 2017;44:1755-70.
66. Park SY, Kim IH, Ye SJ, Carlson J, Park JM. Texture analysis on the fluence map to evaluate the degree of modulation for volumetric modulated arc therapy. Med Phys 2014;41:111718.
67. Bogowicz M, Pavic M, Riesterer O, et al. Targeting treatment resistance in head and neck squamous cell carcinoma - proof of concept for CT radiomics-based identification of resistant sub-volumes. Front Oncol 2021;11:664304.
68. Guidi G, Maffei N, Vecchi C, et al. A support vector machine tool for adaptive tomotherapy treatments: prediction of head and neck patients criticalities. Phys Med 2015;31:442-51.
69. Lam LHT, Do DT, Diep DTN, et al. Molecular subtype classification of low-grade gliomas using magnetic resonance imaging-based radiomics and machine learning. NMR Biomed 2022;35:e4792.
70. Kha QH, Le VH, Hung TNK, Le NQK. Development and validation of an efficient MRI radiomics signature for improving the predictive performance of 1p/19q Co-deletion in lower-grade gliomas. Cancers 2021;13:5398.
71. Caruso D, Polici M, Zerunian M, et al. Radiomics in oncology, part 1: technical principles and gastrointestinal application in CT and MRI. Cancers 2021;13:2522.
73. Brouwer CL, Steenbakkers RJ, Langendijk JA, Sijtsema NM. Identifying patients who may benefit from adaptive radiotherapy: Does the literature on anatomic and dosimetric changes in head and neck organs at risk during radiotherapy provide information to help? Radiother Oncol 2015;115:285-94.
74. Laskar SG, Swain M. HPV positive oropharyngeal cancer and treatment deintensification: how pertinent is it? J Cancer Res Ther 2015;11:6-9.
75. Scott JG, Berglund A, Schell MJ, et al. A genome-based model for adjusting radiotherapy dose (GARD): a retrospective, cohort-based study. Lancet Oncol 2017;18:202-11.
76. Torres-Roca JF, Eschrich S, Zhao H, et al. Prediction of radiation sensitivity using a gene expression classifier. Cancer Res 2005;65:7169-76.
77. Eschrich SA, Pramana J, Zhang H, et al. A gene expression model of intrinsic tumor radiosensitivity: prediction of response and prognosis after chemoradiation. Int J Radiat Oncol Biol Phys 2009;75:489-96.
78. Du Y, Peyser ND, Grandis JR. Integration of molecular targeted therapy with radiation in head and neck cancer. Pharmacol Ther 2014;142:88-98.
79. Gustafson DL, Frederick B, Merz AL, Raben D. Dose scheduling of the dual VEGFR and EGFR tyrosine kinase inhibitor vandetanib (ZD6474, Zactima) in combination with radiotherapy in EGFR-positive and EGFR-null human head and neck tumor xenografts. Cancer Chemother Pharmacol 2008;61:179-88.
80. Sano D, Matsumoto F, Valdecanas DR, et al. Vandetanib restores head and neck squamous cell carcinoma cells’ sensitivity to cisplatin and radiation in vivo and in vitro. Clin Cancer Res 2011;17:1815-27.
81. Hsu HW, Wall NR, Hsueh CT, et al. Combination antiangiogenic therapy and radiation in head and neck cancers. Oral Oncol 2014;50:19-26.
82. Yadav A, Kumar B, Teknos TN, Kumar P. Sorafenib enhances the antitumor effects of chemoradiation treatment by downregulating ERCC-1 and XRCC-1 DNA repair proteins. Mol Cancer Ther 2011;10:1241-51.
83. Bozec A, Sudaka A, Toussan N, Fischel JL, Etienne-Grimaldi MC, Milano G. Combination of sunitinib, cetuximab and irradiation in an orthotopic head and neck cancer model. Ann Oncol 2009;20:1703-7.
84. de Bakker T, Journe F, Descamps G, et al. Restoring p53 function in head and neck squamous cell carcinoma to improve treatments. Front Oncol 2021;11:799993.
85. Chen RC, Gabriel PE, Kavanagh BD, McNutt TR. How will big data impact clinical decision making and precision medicine in radiation therapy? Int J Radiat Oncol Biol Phys 2016;95:880-4.
86. Jagsi R, Bekelman JE, Chen A, et al. Considerations for observational research using large data sets in radiation oncology. Int J Radiat Oncol Biol Phys 2014;90:11-24.
87. Robertson SP, Quon H, Kiess AP, et al. A data-mining framework for large scale analysis of dose-outcome relationships in a database of irradiated head and neck cancer patients. Med Phys 2015;42:4329-37.
88. Marungo F, Robertson S, Quon H, et al. Creating a data science platform for developing complication risk models for personalized treatment planning in radiation oncology. In: 2015 48th Hawaii International Conference on System Sciences. Kauai, HI, USA; 5-8 January 2015; pp. 3132-40.
89. Cavalieri S, De Cecco L, Brakenhoff RH, et al. Development of a multiomics database for personalized prognostic forecasting in head and neck cancer: the big data to decide EU project. Head Neck 2021;43:601-12.
90. Rosenstein BS, Capala J, Efstathiou JA, et al. How will big data improve clinical and basic research in radiation therapy? Int J Radiat Oncol Biol Phys 2016;95:895-904.
91. Willems SM, Abeln S, Feenstra KA, et al. The potential use of big data in oncology. Oral Oncol 2019;98:8-12.
92. Huser V, Cimino JJ. Impending challenges for the use of big data. Int J Radiat Oncol Biol Phys 2016;95:890-4.
93. Jairam V, Park HS. Strengths and limitations of large databases in lung cancer radiation oncology research. Transl Lung Cancer Res 2019;8:S172-83.
Cite This Article

How to Cite
Download Citation
Export Citation File:
Type of Import
Tips on Downloading Citation
Citation Manager File Format
Type of Import
Direct Import: When the Direct Import option is selected (the default state), a dialogue box will give you the option to Save or Open the downloaded citation data. Choosing Open will either launch your citation manager or give you a choice of applications with which to use the metadata. The Save option saves the file locally for later use.
Indirect Import: When the Indirect Import option is selected, the metadata is displayed and may be copied and pasted as needed.
About This Article
Copyright
Data & Comments
Data
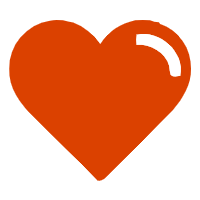
Comments
Comments must be written in English. Spam, offensive content, impersonation, and private information will not be permitted. If any comment is reported and identified as inappropriate content by OAE staff, the comment will be removed without notice. If you have any queries or need any help, please contact us at [email protected].