A bendamustine resistance gene signature in diffuse large B-cell lymphoma and multiple myeloma
Abstract
Aim: Bendamustine is primarily used for treatment of indolent lymphomas but has shown efficacy in some patients with diffuse large B-cell lymphoma (DLBCL) and multiple myeloma (MM). Molecular-based patient stratification for identification of resistant patients, who will benefit from alternative treatments, is important. The aim of this study was to develop a resistance gene signature (REGS) from bendamustine dose-response assays in cultures of DLBCL and MM cell lines, enabling prediction of bendamustine response in DLBCL and MM patients.
Methods: Bendamustine response was determined in 14 DLBCL and 11 MM cell lines. Using baseline gene expression profiles and degree of growth inhibition after bendamustine exposure, a bendamustine REGS was developed and examined for the risk stratification potential in DLBCL (n = 971) and MM (n = 1,126) patients divided into prognostic subtypes.
Results: Bendamustine resistance significantly correlated with resistance to cyclophosphamide in DLBCL and melphalan in MM cell lines. The bendamustine REGS showed significantly lower bendamustine resistance probabilities in DLBCL patients with GCB subtype tumors and in tumors of the differentiation dependent centrocyte and plasmablast subtypes. In MM patients, pre-BII classified tumors displayed high bendamustine resistance probabilities and the plasma cell subtype had lower bendamustine resistance probability than memory cells. Furthermore, tumors belonging to the 4p14, MAF, and D2 TC subclasses consistently displayed high bendamustine resistance probabilities.
Conclusion: Significant differences in predicted response to bendamustine were found in molecular subtypes of DLBCL and MM, encouraging validation in prospective bendamustine-treated cohorts with available gene expression profiles and follow-up data.
Keywords
Introduction
Bendamustine is a bi-functional drug used for treatment of chronic lymphocytic leukemia (CLL) and rituximab-refractory indolent non-Hodgkin’s lymphoma (NHL)[1]. It contains three structural components: (1) a nitrogen mustard (2-chloroethylamine group) which confers the alkylating function; (2) a benzimidazole ring (purine analog) giving it the antimetabolite function; and (3) a butyric acid group that confers water solubility[2]. The mechanisms of action of bendamustine include DNA cross-linking, activation of DNA damage response leading to apoptosis, inhibition of mitotic checkpoints, and induction of mitotic catastrophe - a form of non-apoptotic cell death[3]. Bendamustine is known to be well-tolerated and has also been shown to be effective as a single agent in heavily pretreated, high-grade NHL patients[4].
The most common type of NHL is diffuse large B-cell lymphoma (DLBCL)[5]. DLBCL is an aggressive form of NHL and the standard treatment is immuno-chemotherapy consisting of rituximab, cyclophosphamide, doxorubicin, vincristine, and prednisone (R-CHOP). Unfortunately, up to one third of patients eventually develop refractory disease or relapse (R/R) in which case they are treated with high dose salvage therapy and autologous stem cell transplantations (ASCT)[6]. Not all diagnostic patients are eligible for full-dose R-CHOP due to toxicities which might lead to dose reduction, substitution, or discontinuation of one or more of the drugs[7]. Moreover, a substantial fraction of R/R patients are ineligible for ASCT due to comorbidities and age, and for these no standard salvage therapy exist[6,8,9]. Thus, effective alternative therapies with manageable toxicities are needed. In that context, bendamustine in combination with rituximab therapy has been suggested due to long-term remission for some patients together with low toxicity[8,10,11]. Several clinical studies have tested the effect of combined bendamustine and rituximab treatment in DLBCL patients and reported an acceptable toxicity profile with modest activity, meaning only a subset of the patients respond [Supplementary Table 1][4,12-18]. Thus, strategies for patient stratification based on bendamustine response are needed.
Multiple myeloma (MM) accounts for up to 10% of all hematological malignancies[19] and develops from post-germinal, antibody-secreting plasma cells that have undergone somatic hypermutation before they infiltrate the bone marrow[20]. Over the past decades, the survival of MM patients has improved drastically, mainly attributed by advances in novel therapies[21,22]. Melphalan combined with prednisone was the first successful therapy in achieving relatively high response rates in MM and has remained the gold standard for several decades. Of note, a phase III study has later revealed a generally superior outcome when using bendamustine in combination with prednisone in comparison to melphalan combined with prednisone, and it was thus approved as first-line therapy for MM patients not eligible for ASCT[23]. In R/R MM patients, the overall response to bendamustine monotherapy is 30%-55%[24] and higher when combined with other chemotherapy agents [Supplementary Table 1][23,25-27]. As with DLBCL, bendamustine combination treatment has been shown to be safe, effective, and well-tolerated in both diagnostic and R/R MM patients[24,28,29].
Various prognostic molecular classifications have been developed based on gene expression profiles (GEPs) of DLBCL and MM cell lines and patient tumor samples. According to the 2016 revision of the World Health Organization classification of lymphoid malignancies, the standard molecular subclassification of DLBCL is activated B-cell-like (ABC) and germinal center B-cell-like (GCB) DLBCL which has proven to have prognostic impact favoring the GCB-DLBCL subtypes[30,31]. MM can be subclassified based on the translocation and cyclin D (TC) classification which distinguishes eight subtypes based on immunoglobin translocations, activation of cyclin D genes, and chromosomal ploidy[32]. A more refined cell-of-origin (COO) based classification system, the “B-cell associated gene signature” (BAGS), also exist for DLBCL and MM where primary cancer cells at the time of diagnosis are associated to normal B-cell subset phenotypes from tonsils or bone marrow, respectively. DLBCL patients are classified into one of the following subtypes: naïve, centrocyte, centroblast, memory B-cell, or plasmablast[33], and MM patients into: Pre-BI, Pre-BII, immature, naïve, memory, or plasma cell[34]. BAGS provide for both DLBCL and MM independent and significant prognostic information as compared to ABC/GCB and TC, and for DLBCL when compared to the clinical international prognostic index. In addition, the BAGS subtypes have distinct and specific responses to chemotherapeutic agents used in a routine clinical setting for treatment of DLBCL and MM[33,34]. Machine learning models have previously been developed to predict response to components of the R-CHOP regimen in DLBCL and melphalan in MM. These models were successfully generated based on dose-response studies and resistance gene signatures (REGS) in human B-cell cancer cell lines (HBCCL)[35-37].
In this study, we hypothesize that bendamustine dose-response assays and GEP of cell lines can be used to find gene expression patterns in which the expression of a group of genes predicts the cellular response to bendamustine. The predicted response can be applied to samples from DLBCL and MM patients to identify the subset of patients that will be sensitive or resistant to treatment. To test this, we developed a REGS from DLBCL and MM cell lines based on GEP and bendamustine dose-response assays, and subsequently investigated their stratification potential of DLBCL and MM patients based on molecular profiles.
Methods
Cell lines and identity confirmation
HBCCLs consisting of 14 DLBCL cell lines (DB, FARAGE, HBL-1, MC-116, NU-DHL-1, NU-DUL-1, OCI-Ly3, OCI-Ly7, OCI-Ly8, RIVA, SU-DHL-5, SU-DHL-8, SU-DHL-10, and U-2932) and 11 MM cell lines (AMO-1, KMS-11, KMS-12-BM, KMS-12-PE, LP-1, MOLP-2, MOLP-8, NCI-H929, OPM-2, RPMI-8226, and U266) were used. Culturing and DNA barcoding for verification of cell line identities were performed as previously described[36,38]. The cells were cultured at 37 °C in a humidified atmosphere of 95% air and 5% CO2 with the appropriate medium, serum, and supplements [Table 1].
Cell line | Disease | Culture media | ABC/GCB or TC classification | BAGS |
---|---|---|---|---|
DB | DLBCL | RPMI1640, 20%FBS | GCB | Centrocyte |
FARAGE | DLBCL | RPMI1640, 10%FBS | GCB | Centrocyte |
HBL-1 | DLBCL | RPMI1640, 10%FBS | ABC | Centrocyte |
MC-116 | DLBCL | RPMI1640, 15%FBS | GCB | Centrocyte |
NU-DHL-1 | DLBCL | RPMI1640, 10%FBS | ABC | Memory |
NU-DUL-1 | DLBCL | RPMI1640, 15%FBS | UC | Naïve |
OCI-Ly3 | DLBCL | IMDM, 20%HS* | ABC | Centrocyte |
OCI-Ly7 | DLBCL | RPMI1640, 10%FBS | GCB | Centroblast |
OCI-Ly8 | DLBCL | RPMI1640, 10%FBS | UC | Centroblast |
RIVA | DLBCL | RPMI1640, 10%FBS | ABC | Centrocyte |
SU-DHL-10 | DLBCL | RPMI1640, 10%FBS | GCB | Centrocyte |
SU-DHL-5 | DLBCL | RPMI1640, 20%FBS | GCB | Centrocyte |
SU-DHL-8 | DLBCL | RPMI1640, 10%FBS | GCB | Centroblast |
U-2932 | DLBCL | RPMI1640, 10%FBS | GCB | Centrocyte |
AMO-1 | MM | RPMI1640, 20%FBS | D2 | Plasma cell |
KMS-11 | MM | RPMI1640, 10%FBS | 4p16 | Plasma cell |
KMS-12-BM | MM | RPMI1640, 20%FBS | 4p16 | Plasma cell |
KMS-12-PE | MM | RPMI1640, 20%FBS | 4p16 | Unclassified |
LP-1 | MM | IMDM, 10%FBS | 4p16 | Plasma cell |
MOLP-2 | MM | RPMI1640, 20%FBS | D2 | NA |
MOLP-8 | MM | RPMI1640, 20%FBS | 4p16 | Plasma cell |
NCI-H929 | MM | RPMI1640, 10%FCS** | 4p16 | Plasma cell |
OPM-2 | MM | RPMI1640, 10%FBS | 4p16 | Plasma cell |
RPMI-8226 | MM | RPMI1640, 10%FCS | MAF | Naïve |
U266 | MM | RPMI1640, 10%FBS | 4p16 | Unclassified |
Systematic dose-response assay
Prior to the dose-response experiments, the optimal seeding concentration for each cell line was determined as the highest concentration at which the cells grew at an exponential rate throughout the experiment without drugs added. The effect of bendamustine on viable proliferating cells was measured for the 25 HBCCL. Cells were seeded in 120 µL culture media per well in a 96 well plate 24 h before 16 bendamustine concentrations of two-fold increments were added. The highest bendamustine concentration used was 500 µg/mL in isotonic saltwater. Viability was measured immediately after addition of bendamustine (0 h plate) and after 48 h of drug exposure (48 h plate) by addition of CellTiter reagent (CellTiter 96 Aqueous One Solution Reagent, Promega, USA) and subsequent absorbance measurement at 492 nm using FLUOstar Optima (BMG LABTECH, Germany). Isotonic saltwater was used as controls alongside bendamustine. Border effects were circumvented by only including non-border wells for analysis for each experiment, and experiments were performed in triplicates. Time independent summary statistics were obtained as previously described[36].
Gene expression profiling datasets for cell lines and clinical cohorts
GEPs for each untreated cell line using the Affymetrix Human Genome U133 Plus 2.0 arrays were used. These were generated by purification of RNA from cells, cDNA conversion, biotin-labelling, and lastly hybridization to the GeneChip. The .CEL files for all cell lines are deposited with GEO accessions GSE53798[35], GSE99634[34], and GSE22759[40]. In DLBCL, three individual, diagnostic patient cohorts with available GEP datasets from tumors were used to test the bendamustine REGS, and GEP data were pre-processed as previously described[41]. These encompass the IDRC dataset (International DLBCL Rituximab-CHOP Consortium Program Study, n = 467 GEO accession: GSE31312)[42], the LLMPP dataset (Lymphoma/Leukemia Molecular Profiling Project, with data divided based on subsequent treatment into LLMPPCHOP: n = 181 and LLMPPRCHOP: n = 233, GEO accession: GSE10846)[43], and the MDFCI dataset (Mayo-Dana-Farber Cancer Institute, n = 90, GEO accession: GSE34171)[44]. Similarly, the following three separate and previously described[34] MM patient cohorts were used to test the bendamustine REGS: HOVON-65 dataset (n = 320, GEO accession: GSE19784)[45], MRC Myeloma IX dataset (n = 247, GEO accession: GSE15695, Platform: GPL570)[46], and UAMS dataset (University of Arkansas for Medical Sciences, n = 559, GEO accession: GSE24080)[47].
Statistical analysis
For all statistical analyses R version 4.0.2 was used[48]. An Rmarkdown document detailing the analysis is available as Supplementary File 1.
Correlations between bendamustine, cyclophosphamide, doxorubicin, vincristine, and melphalan response were determined by assessing the pairwise Pearson’s correlation of the area under the positive parts of the dose-response curves (AUC0). In this aspect, previously generated summary statistics for the other drugs, used in the treatment of hematological cancers, were obtained in addition to the bendamustine dose-response assays[35,40]. A generalized pairs plot was created using the R package GGally[49].
The bendamustine REGS is based on regularized multivariate regression analysis with cross-validated tuning parameters and can assign probability of resistance to a specific drug utilizing GEP and dose-response data from cell lines[38]. For the development of the bendamustine REGS, we followed a previously described approach[35]. Briefly, the HBCCL and clinical cohort microarray data were background corrected and Robust Multichip Average (RMA) pre-processed using the Bioconductor package frma[50]. Cell lines were split into tertiles according to their AUC0 values separately for each disease to avoid comparison of diseases and assigned values of sensitive, intermediate, or resistant. Sensitive and resistant cell lines were treated as binary outcomes and GEPs as predictors in a logistic regression model with an elastic net penalty using the R package glmnet[51]. Optimal parameters were obtained using leave-one-out cross-validation, with misclassification error as the loss function.
Cell lines and patient samples from cohorts were assigned to DLBCL or MM BAGS classes using the BAGS classifiers[33,34]. Assignment to a BAGS group was determined by the highest predicted probability across the classes, setting the 15% of patients with lowest classification probability in each cohort as unclassified. Kaplan-Meier curves of DLBCL patients were trichotomized based on predicted bendamustine response including p-values from a log-rank test generated using the R package survminer[52].
Results
Bendamustine dose-response assay in a panel of 25 human B-cell cancer cell lines
Using the relative growth inhibition for a drug, it is possible to generate cell line specific dose-response curves[38]. However, faster growing cells generally appear more sensitive to chemotherapy drugs when using a fixed timepoint[36], thus to account for this bias under the assumption of exponential growth, the growth rate from treated cells was normalized using untreated cells at both 0h and 48h time points as reference. This allows for a time independent determination of growth inhibition where growth rates are accounted for and hereby the bendamustine response among HBCCL panel can be ranked and compared. The four summary statistics from the drug response experiments are: 50% growth inhibition compared to untreated cells (GI50), total growth inhibition (TGI), the concentration at which the cell population is halved after 48 h (LC48), and AUC0 (the area under the curve above 0) [Table 2].
Summary statistics and T0 from bendamustine dose-response assays consisting of: Doubling time for individual cell lines (T0) in hours, concentration to obtain GI50, TGI, 50% cell decay at 48 h (LC48), and area under the positive part of the dose-response curve (AUC0)
Cell line | T0 | GI50 | TGI | LC48 | AUC0 |
---|---|---|---|---|---|
AMO-1 | 30 (29;31) | 1.84 (1.83;1.84) | 1.91 (1.91;1.92) | 2.01 (2.00;2.02) | 350.77 (344.73;357.66) |
DB | 40 (39;41) | 1.83 (1.83;1.84) | 1.96 (1.95;1.97) | 2.10 (2.10;2.10) | 344.57 (338.43;347.85) |
FARAGE | 48 (46;50) | 0.99 (0.96;1.01) | 1.37 (1.34;1.39) | 1.58 (1.57;1.59) | 269.60 (258.59;273.48) |
HBL-1 | 32 (31;32) | 1.46 (1.44;1.47) | 1.63 (1.62;1.64) | 1.81 (1.80;1.82) | 313.61 (308.36;316.95) |
KMS-11 | 47 (45;49) | 1.82 (1.81;1.82) | 1.85 (1.85;1.85) | 1.91 (1.91;1.92) | 340.53 (330.90;348.95) |
KMS-12-BM | 38 (37;40) | 1.30 (1.28;1.33) | 1.53 (1.52;1.53) | 1.62 (1.61;1.63) | 287.42 (279.99;292.87) |
KMS-12-PE | 45 (43;47) | 1.80 (1.80;1.80) | 1.81 (1.81;1.82) | 1.83 (1.82;1.84) | 346.87 (336.46;354.13) |
LP-1 | 37 (35;38) | 2.07 (2.03;2.10) | 2.12 (2.10;2.13) | 2.16 (2.12;2.17) | 351.59 (334.09;361.07) |
MC-116 | 59 (55;62) | 1.56 (1.52;1.57) | 1.75 (1.71;1.78) | 1.81 (1.81;1.81) | 288.97 (274.71;294.63) |
MOLP-2 | 129 (119;141) | 0.99 (0.94;1.01) | 1.20 (1.16;1.22) | 1.80 (1.77;1.80) | 250.41 (237.85;261.82) |
MOLP-8 | 32 (31;33) | 1.27 (1.25;1.28) | 1.50 (1.47;1.52) | 1.75 (1.72;1.78) | 297.54 (293.42;302.04) |
NCI-H929 | 43 (42;45) | 1.80 (1.80;1.80) | 1.83 (1.80;1.83) | 1.88 (1.81;1.89) | 340.65 (326.74;346.13) |
NU-DHL-1 | 34 (33;35) | 0.96 (0.93;0.99) | 1.28 (1.27;1.29) | 1.52 (1.51;1.52) | 267.75 (261.68;269.21) |
NU-DUL-1 | 43 (42;44) | 0.60 (0.60;0.61) | 0.73 (0.72;0.74) | 0.96 (0.95;0.97) | 228.13 (226.01;235.19) |
OCI-Ly3 | 26 (25;26) | 1.35 (1.33;1.36) | 1.61 (1.57;1.64) | 1.88 (1.87;1.89) | 310.70 (304.25;314.61) |
OCI-Ly7 | 44 (42;46) | 1.54 (1.53;1.55) | 1.69 (1.67;1.71) | 1.83 (1.82;1.84) | 269.17 (262.78;278.12) |
OCI-Ly8 | 23 (23;24) | 1.52 (1.49;1.54) | 1.83 (1.82;1.83) | 1.92 (1.91;1.93) | 315.94 (309.48;320.47) |
OPM-2 | 69 (66;73) | 1.82 (1.81;1.83) | 1.88 (1.87;1.89) | 2.06 (2.05;2.07) | 342.42 (329.41;350.07) |
RIVA | 40 (38;41) | 1.34 (1.32;1.38) | 1.56 (1.56;1.57) | 1.73 (1.72;1.74) | 286.65 (275.47;294.00) |
RPMI-8226 | 43 (41;46) | 2.10 (2.10;2.11) | 2.10 (2.10;2.14) | 2.11 (2.11;2.21) | 378.98 (369.63;382.48) |
SU-DHL-10 | 31 (30;32) | 1.83 (1.83;1.83) | 1.87 (1.87;1.88) | 1.93 (1.92;1.93) | 351.16 (343.86;357.56) |
SU-DHL-5 | 35 (34;37) | 0.44 (0.43;0.45) | 0.62 (0.62;0.63) | 0.78 (0.78;0.79) | 219.79 (213.34;223.48) |
SU-DHL-8 | 58 (56;61) | 1.05 (1.03;1.07) | 1.24 (1.22;1.26) | 1.54 (1.52;1.56) | 281.88 (269.61;283.06) |
U266 | 58 (56;60) | 1.80 (1.75;1.80) | 1.88 (1.88;1.89) | 2.09 (2.08;2.10) | 333.48 (326.50;337.13) |
U2932 | 46 (43;47) | 1.16 (1.13;1.19) | 1.37 (1.35;1.39) | 1.68 (1.67;1.70) | 288.84 (280.70;293.88) |
Increasing concentrations of bendamustine induced growth inhibition at varying degrees for both DLBCL and MM cell lines [Figure 1A]. Cell decay can be seen in the bottom half of the dose-response curves which show half times (e.g., a value of -1/48 is the concentration at which the cell population is halved after 48 h). Overall, DLBCL cell lines were less resistant to bendamustine than MM cell lines [Figure 1B and C] with AUC0 ranging from 219.79 (95%CI: 213.3; 223.5) to 351.16 (CI: 343.9; 357.6) in DLBCL and 250.41 (CI: 237.9; 261.8) to 378.98 (CI: 369.6; 382.5) in MM cell lines. The most sensitive cells were SU-DHL-5 and MOLP-2 in DLBCL and MM, respectively, and the most resistant were SU-DHL-10 and RPMI-8226, respectively.
Figure 1. Dose-response curves for the bendamustine screens in DLBCL and MM cell lines. A: dose-response curves for the 14 DLBCL cell lines (DB, FARAGE, HBL-1, MC-116, NU-DHL-1, NU-DUL-1, OCI-Ly3, OCI-Ly7, OCI-Ly8, RIVA, SU-DHL-5, SU-DHL-8, SU-DHL-10, and U-2932) and 11 MM cell lines (AMO-1, KMS-11, KMS-12-BM, KMS-12-PE, LP-1, MOLP-2, MOLP-8, NCI-H929, OPM-2, RPMI-8226, and U266); B: ranked 50% growth inhibition (GI50) corrected for individual growth rates of the cell lines with DLBCL cell lines to the left and MM cell lines to the right; C: ranked area under the positive part of the curve (AUC0) values corrected for individual growth rates of the cell lines with DLBCL cell lines to the left and MM cell lines to the right. DLBCL: diffuse large B-cell lymphoma; MM: multiple myeloma
Interestingly, the six MM cell lines, U266, KMS-12-PE, NCI-H929, KMS-11, OPM-2, and AMO-1 displayed very similar GI50 and AUC0 values with GI50 ranging from 1.80 (CI: 1.75; 1.80) to 1.84 (CI: 1.83; 1.84) and AUC0 ranging from 333.5 (CI: 326.50; 337.13) to 350.8 (CI: 344.7; 357.7) [Figure 1B and C]. Characteristically, these six cell lines belong to the plasma cell or unclassified BAGS-MM subtypes, and five of the cell lines belong to the TC 4p16 class whereas AMO-1 is TC D2 [Table 1]. RPMI-8226 is noticeably more resistant than the rest of the MM cell lines and has a unique profile as it is BAGS classified as naïve with a MAF TC subclass. For DLBCL, most cell lines were either BAGS classified as centroblasts or centrocytes.
Assessment of correlations in response to other hematological drugs
To investigate co-occurrence of resistance and sensitivity among the HBCCL towards various drugs used in the treatment of hematological cancers, we assessed the pairwise Pearson’s correlation between AUC0 values for bendamustine from this study and the cornerstone drugs of standard DLBCL and MM treatment: cyclophosphamide, doxorubicin, vincristine, and melphalan obtained from previous dose-response assays [Supplementary Table 2][35,40]. A significant and positive correlation was regarded as an indication of shared resistance mechanisms between two compared drugs.
In DLBCL cells, the highest and only significant correlation coefficient was found between bendamustine and cyclophosphamide treatment (R = 0.747, P < 0.05) [Figure 2]. Moderate and non-significant correlations in response were also observed for bendamustine compared to doxorubicin and vincristine, both of which displayed the lowest correlation among all the drugs in DLBCL cell lines (R = 0.44 for both). Melphalan dose-response data was not included for DLBCL cell lines since there were too few treated cell lines for comparison, and it is not widely used in the treatment of DLBCL. In MM, correlation coefficients between the drugs were overall lower [Figure 2]. The strongest, albeit non-significant, correlation for bendamustine was found in comparison to melphalan (R = 0.49) and cyclophosphamide (R = 0.43) - two other alkylating agents. Interestingly, these two drugs displayed the overall strongest and most significant correlation in MM (R = 0.659, P < 0.05) [Figure 2]. The lowest drug response correlation in MM was observed for vincristine, which exerts its mechanism of action during the M-phase in contrast to the other four drugs. In addition, there was a very low correlation between bendamustine and doxorubicin response in MM cell lines (R = 0.085). In summary, a stronger correlation in response between agents with the similar mechanisms of action was observed, indicating that there are inherent response patterns to similar drugs in the HBCCL.
Figure 2. Correlations between drug response in HBCCL. A generalized pairs plot, showing correlations in response between two drugs in DLBCL and MM cell lines. Distributions and plots are based on values for area under the positive part of the curve (AUC0) which are displayed on the axes, and the shaded area shows the 95% confidence interval. Data for melphalan in DLBCL was not included since there were too few melphalan treated cell lines available. Corr: correlation. •P < 0.1, *P < 0.05, **P < 0.01, ***P < 0.001. DLBCL: diffuse large B-cell lymphoma; MM: multiple myeloma; HBCCL: human B-cell cancer cell lines
Stratification of clinical datasets based on predicted bendamustine response
A combination of GEPs from untreated cells in the HBCCL panel and outcome from the bendamustine dose-response assays was used to develop the bendamustine REGS. The minimum misclassification error in the cross-validation was 0.33. The final prediction model trained on the complete dataset, using the optimal parameters obtained from the cross-validation, generated a bendamustine REGS with 13 genes (CCND2, EVI2A, HLA-DRA, SERPINF1, HMGN3, COX7A2, CD52, JCHAIN, HSPA1A, MPEG1, PRKAR2B, CLEC2B and QPCT) [Supplementary Table 3]. Both DLBCL and MM tumors display molecular heterogeneity whereby cells from these diseases can be subclassified into different subtypes showing different prognosis after treatment[53,54]. Thus, we evaluated whether there was a difference in the predicted bendamustine response using the bendamustine REGS on GEPs restricted to DLBCL and MM patients and on different subtypes hereof [Supplementary Table 4].
In DLBCL, the bendamustine REGS was tested in four independent, diagnostic DLBCL cohorts. As there was no significant difference in bendamustine resistance probabilities between the four cohorts [Supplementary Figure 1A], they were merged into one large cohort for further analyses. Subclassification into ABC/GCB subtypes showed there was a significant difference in predicted bendamustine response (P < 0.01), where ABC patients displayed the highest bendamustine resistance probability in contrast to GCB patients [Figure 3A]. When the merged DLBCL cohorts was divided into BAGS subtypes, a significant difference between the molecular subtypes was observed (P < 0.01) despite the large variations in resistance probability. The DLBCL patients with reminiscent transcriptional expression patterns matching centrocyte and plasmablasts displayed lower probabilities of bendamustine resistance in comparison to the naïve, centroblast, memory, and unclassified subtypes [Figure 3B]. Analysis of the BAGS subtypes separately for patients belonging to the ABC and GCB subtypes showed significant differences in predicted response to bendamustine in molecular subtypes reflecting the degree of cellular differentiation in both groups [Supplementary Figure 1C and D]. Of notice, tumors of the memory subtype in ABC-DLBCL patients displayed high probabilities of bendamustine resistance, whereas they were predicted to be the least bendamustine resistant subtype along with plasmablasts in the GCB-DLBCL patients.
Figure 3. Bendamustine resistance probability in COO subtypes of patient cohorts. A: bendamustine REGS in ABC/GCB subtypes of DLBCL; B: bendamustine resistance probabilities using the bendamustine REGS in BAGS-DLBCL subtypes; C: bendamustine resistance probability using the REGS in BAGS-MM subtypes separately for each dataset; D: bendamustine resistance probability using the REGS in TC subclasses separately for each dataset. Significant differences between subtypes were tested using Kruskal-Wallis tests. DLBCL: diffuse large B-cell lymphoma; MM: multiple myeloma; BAGS: B-cell associated gene signature; REGS: resistance gene signature; COO: cell-of-origin; ABC: activated B-cell-like; GCB: germinal center B-cell-like
For the MM cohorts there was a significant difference in the distribution of bendamustine resistance probabilities between the three datasets used [Supplementary Figure 1B]. Thus, subsequent comparison to the BAGS classification was performed in each individual dataset [Figure 3C]. When the bendamustine REGS was applied to the MM datasets, we did not find any significant difference between BAGS subtypes, however, the plasma cell subtype had the lowest bendamustine resistance probability in the MyelomaIX and UAMS datasets and was less resistant than the memory subtype in general. Additionally, the Pre-BII subtype had a high bendamustine resistance probability in all datasets [Figure 3C, Supplementary Figure 1E]. Significant differences between the TC subclasses were found in all three datasets, where the highest bendamustine resistance probability was consistently found for the TC classes: 4p14, MAF, and D2 [Figure 3D].
We did not find any bendamustine treated DLBCL cohorts usable for retrospective validation of the prediction model. However, as there was a significant correlation between bendamustine and cyclophosphamide response in the HBCCL in vitro, the predictive value of the bendamustine REGS was evaluated in patients treated with cyclophosphamide containing drug regimens. The LLMPP clinical DLBCL dataset, which is split into CHOP and R-CHOP treated patients, was used, and patients were trichotomized into sensitive, intermediate, and resistant groups based on predicted bendamustine response. Bendamustine resistance probabilities using the bendamustine REGS were significantly associated with overall survival in the CHOP treated cohort (P < 0.01), but not in the R-CHOP treated cohort [Supplementary Figure 2A and B]. However, when the bendamustine REGS and a previously generated cyclophosphamide REGS was compared in patients within each of the clinical cohorts, no significant correlation was found between the two REGSs [Supplementary Figure 3].
In summary, in clinical samples (DLBCL: n = 971, MM: n = 1,126) with individually assigned bendamustine resistance probabilities, we found that the ABC subtype was the most resistant in DLBCL, and the centrocyte and plasmablast subtypes were predicted to be the least resistant in the DLBCL patient cohorts. Bendamustine resistance probabilities in memory subtype tumors depended on ABC/GCB subclassification. In MM, the Pre-BII subtype had a high bendamustine resistance probability along with TC classes 4p14, MAF, and D2. Thus, molecular and COO based subclassification of patient cohorts can stratify patients based on bendamustine resistance probabilities.
Discussion
Bendamustine is a good choice for treatment of elderly, frail, comorbid, or transplant ineligible DLBCL and MM patients, however, a fraction of these patients do not respond with sufficient efficacy[24,55,56], urging the development of patient stratification tools for better prediction of responders and non-responders. Both DLBCL and MM display great molecular heterogeneity[53,54] which might play a role in the response to bendamustine. The aim of the current study was to develop bioinformatics algorithms, enabling the prediction of bendamustine response in DLBCL and MM patients based on a combination of in vitro bendamustine dose-response assays in DLBCL and MM cell lines and their baseline GEPs. Within the HBCCL panel, response to bendamustine did not correlate with subtypes of BAGS nor with ABC/GCB subtypes for DLBCL. For MM, most of the cell lines were TC classified as 4p16 whereas the most bendamustine resistant cell line, RPMI-8226, was classified as MAF.
Bendamustine contains both a 2-chloroethylamine group also found in cyclophosphamide, melphalan, and chlorambucil, which confers the alkylating properties and a purine-like benzimidazole ring, which is found in nucleoside analogs, giving it an antimetabolite function[2]. Significant correlation between response to bendamustine and cyclophosphamide was observed in DLBCL cell lines and between melphalan and cyclophosphamide in MM cell lines. In addition, there was a moderate correlation between bendamustine response with cyclophosphamide and melphalan in MM cell lines. Higher coefficients of correlation suggest that the agents have similar mechanisms of action which is to be expected as these are all alkylating agents. Strong correlations have previously been found between cyclophosphamide, melphalan, and chlorambucil in the NCI-60 pan cancer cell panel, however, correlation between these agents and bendamustine was not as strong[3]. As expected, the similar mechanisms of action support the cross-resistance observed between bendamustine and chlorambucil and also to the nucleoside analog, fludarabine, in cells from CLL patients[57].
Bendamustine has synergistic effects in DLBCL and MM cell lines when combined with various nucleoside analogs and other alkylating agents such as cyclophosphamide, chlorambucil, and melphalan, mainly due to its purine analog-like properties[58]. Synergistic effects with nucleoside analogs, but not with chlorambucil, was also shown in CLL cell lines[57]. These results indicate that bendamustine can be combined with other alkylating agents and purine analogs due to its bi-functional property and unique mechanisms of action. Moreover, synergy might not be necessary to achieve curative drug combinations exemplified by the antagonistic interaction seen by vincristine in combination with cyclophosphamide and doxorubicin at higher concentrations in DLBCL cell lines, where the combinations were still able to induce cell death due to non-overlapping resistance mechanisms[59].
Bendamustine REGS in patients assigned to the phenotypic BAGS groups showed that patients belonging to the centrocyte and plasmablast subtypes in DLBCL, and the plasma cell subtype in the MyelomaIX and UAMS MM cohorts were predicted to be less resistant to bendamustine than the rest. Additionally, the bendamustine resistance probability was lower in the GCB-DLBCL subtype than in the ABC-DLBCL subtype. Thus, in a clinical setting, patients with a reminiscent transcriptional profile of these subtypes are suggested to be more sensitive towards bendamustine.
The use of malignant HBCCLs in vitro have limitations when compared to in vivo models, including scarce information on the tumor microenvironment and interaction with the immune system, lack of pharmacokinetic information (e.g., metabolism) regarding the drugs before reaching the tumor, and lack of consideration for intra- and inter-patient clonal heterogeneity of tumors. Despite these limitations, malignant cancer cell lines, like the HBCCLs, serve as strong pre-clinical models for disease specific pharmacogenomic studies (e.g., discovery of resistance genes, new drug targets, combinatorial effects, and cross-resistance) as they harbor many of the same mutations and genomic alterations found in malignant B-cells from DLBCL and MM[60,61]. In addition, the HBCCLs were originally developed from patients and display many of the cytogenic characteristics of DLBCL and MM which contribute to oncogenesis and possibly response to treatments[61]. The translational advantage of the HBCCL is the number of DLBCL and MM tumor specific cell lines in contrast to other cell panels such as NCI-60, which only contains two DLBCL and MM like cell lines.
The prognostic potential in patient stratification using the cell line based bendamustine REGS should be validated in large, independent, and prospective randomized clinical cohorts treated with or without bendamustine in combination with standard treatment regimens to assess its clinical potential. At the current time and to our knowledge, no bendamustine treated DLBCL and MM validation cohorts with available GEP data and follow-up exist. We have previously validated a melphalan REGS, where high probabilities of resistance were associated with inferior clinical outcome in the HOVON-65 dataset[37]. Furthermore, a combined REGS developed from cyclophosphamide, doxorubicin, and vincristine treated cell lines also showed prognostic value in DLBCL[35].
In addition to the correlation between cyclophosphamide and bendamustine response in vitro, significant differences were found in the overall survival between patients trichotomized based on predicted bendamustine resistance in the CHOP treated cohort. This could suggest that the bendamustine REGS is a pseudo marker for response to treatment with alkylating agents sharing similar mechanisms of action with bendamustine in DLBCL. It is, however, worth noting that the DLBCL patients from the clinical datasets were subjected to a combination treatment containing either four or five drugs with different mechanisms of actions, some of which both interact with each other and the surrounding tumor microenvironment affecting the clinical outcome[59,62]. Additionally, the bendamustine resistance probabilities did not correlate with the cyclophosphamide resistance probabilities in patients. Thus, a bendamustine treated cohort is needed for validation of the bendamustine REGS. Strong and validated predictors of treatment outcome based on clear molecular signatures have a great potential in the clinical setting for the identification of resistant patients who will benefit more from alternative treatments. However, many biological and statistical considerations are needed prior to their development and clinical application.
In conclusion, the use of disease-specific cell lines is a strong tool for prognostic classification of patient cohorts as they can be used to create REGSs which can be further applied to clinical samples and used in risk stratification, either alone or in combination with other molecular subclassification models. Here, we evaluated the REGS for bendamustine in COO based subtypes in DLBCL and MM. For DLBCL, we found that the GCB subtype and the differentiation dependent BAGS subtypes of centrocyte, plasmablasts are predicted to have lower bendamustine resistance along with the plasma cell subtype in MM. However, these findings need to be validated in large, independent, prospective patient cohorts with bendamustine-treated patients and with available GEP and follow-up.
Declarations
AcknowledgmentsThe authors thank Louise Hvilshøj Madsen and Helle Høholt for their technical assistance.
Authors’ contributionsConception or design: Issa II, Brøndum RF, Dybkær K
Data acquisition, analysis and interpretation: All authors
Manuscript preparation: All authors
Final approval for publication: All authors
Availability of data and materialsThe datasets used in this article can be accessed through the National Center for Biotechnology Information Gene Expression Omnibus (https://www.ncbi.nlm.nih.gov/geo).
Financial support and sponsorshipThis work was supported by a grant from The Danish Cancer Society (Grant ID: R130-A8346-15-S38) and the Karen Elise Jensen Foundation.
Conflicts of interestAll authors declared that there are no conflicts of interest.
Ethical approval and consent to participateNot applicable.
Consent for publicationNot applicable.
Copyright© The Author(s) 2021.
REFERENCES
1. Gandhi V, Burger JA. Bendamustine in B-cell malignancies: the new 46-year-old kid on the block. Clin Cancer Res 2009;15:7456-61.
2. Forero-Torres A, Saleh MN. Bendamustine in non-hodgkin lymphoma: the double-agent that came from the cold war. Clin Lymphoma Myeloma 2007;8:S13-7.
3. Leoni LM, Bailey B, Reifert J, et al. Bendamustine (Treanda) displays a distinct pattern of cytotoxicity and unique mechanistic features compared with other alkylating agents. Clin Cancer Res 2008;14:309-17.
4. Weidmann E, Kim SZ, Rost A, et al. Bendamustine is effective in relapsed or refractory aggressive non-Hodgkin’s lymphoma. Ann Oncol 2002;13:1285-9.
5. Miller KD, Nogueira L, Mariotto AB, et al. Cancer treatment and survivorship statistics, 2019. CA Cancer J Clin 2019;69:363-85.
7. Fields PA, Linch DC. Treatment of the elderly patient with diffuse large B cell lymphoma. Br J Haematol 2012;157:159-70.
8. Arcari A, Chiappella A, Spina M, et al. Safety and efficacy of rituximab plus bendamustine in relapsed or refractory diffuse large B-cell lymphoma patients: an Italian retrospective multicenter study. Leuk Lymphoma 2016;57:1823-30.
9. Jantunen E, Canals C, Rambaldi A, et al. Autologous stem cell transplantation in elderly patients (>=60 years) with diffuse large B-cell lymphoma: an analysis based on data in the European blood and marrow transplantation registry. Haematologica 2008;93:1837-42.
10. Merchionne F, Quintana G, Gaudio F, et al. Bendamustine plus rituximab for relapsed or refractory diffuse large B cell lymphoma: a retrospective analysis. Leuk Res 2014;38:1446-50.
11. Hong JY, Yoon DH, Suh C, et al. Bendamustine plus rituximab for relapsed or refractory diffuse large B cell lymphoma: a multicenter retrospective analysis. Ann Hematol 2018;97:1437-43.
12. Weidmann E, Neumann A, Fauth F, et al. Phase II study of bendamustine in combination with rituximab as first-line treatment in patients 80 years or older with aggressive B-cell lymphomas. Ann Oncol 2011;22:1839-44.
13. Ohmachi K, Niitsu N, Uchida T, et al. Multicenter phase II study of bendamustine plus rituximab in patients with relapsed or refractory diffuse large B-cell lymphoma. J Clin Oncol 2013;31:2103-9.
14. Vacirca JL, Acs PI, Tabbara IA, et al. Bendamustine combined with rituximab for patients with relapsed or refractory diffuse large B cell lymphoma. Ann Hematol 2014;93:403-9.
15. Park SI, Grover NS, Olajide O, et al. A phase II trial of bendamustine in combination with rituximab in older patients with previously untreated diffuse large B-cell lymphoma. Br J Haematol 2016;175:281-9.
16. Hitz F, Zucca E, Pabst T, et al. Rituximab, bendamustine and lenalidomide in patients with aggressive B-cell lymphoma not eligible for anthracycline-based therapy or intensive salvage chemotherapy - SAKK 38/08. Br J Haematol 2016;174:255-63.
17. Storti S, Spina M, Pesce EA, et al. Rituximab plus bendamustine as front-line treatment in frail elderly (>70 years) patients with diffuse large B-cell non-Hodgkin lymphoma: a phase II multicenter study of the Fondazione Italiana Linfomi. Haematologica 2018;103:1345-50.
18. Sehn LH, Herrera AF, Flowers CR, et al. Polatuzumab vedotin in relapsed or refractory diffuse large B-cell lymphoma. J Clin Oncol 2020;38:155-65.
20. Matsui W, Huff CA, Wang Q, et al. Characterization of clonogenic multiple myeloma cells. Blood 2004;103:2332-6.
21. Nunnelee J, Zhao Q, Benson DM, et al. Improvement in survival of multiple myeloma patients: a long-term institutional experience. Blood 2019;134:4573.
22. Thorsteinsdottir S, Dickman PW, Landgren O, et al. Dramatically improved survival in multiple myeloma patients in the recent decade: results from a Swedish population-based study. Haematologica 2018;103:e412-5.
23. Pönisch W, Mitrou PS, Merkle K, et al. Treatment of bendamustine and prednisone in patients with newly diagnosed multiple myeloma results in superior complete response rate, prolonged time to treatment failure and improved quality of life compared to treatment with Melphalan and Prednisone-a r. J Cancer Res Clin Oncol 2006;132:205-12.
24. Gentile M, Vigna E, Recchia AG, et al. Bendamustine in multiple myeloma. Eur J Haematol 2015;95:377-88.
25. Offidani M, Corvatta L, Maracci L, et al. Efficacy and tolerability of bendamustine, bortezomib and dexamethasone in patients with relapsed-refractory multiple myeloma: a phase II study. Blood Cancer J 2013;3:e162-9.
26. Mateos MV, Oriol A, Rosinol L, et al. Bendamustine, bortezomib and prednisone for the treatment of newly diagnosed multiple myeloma patients: results of a prospective phase 2 Spanish/Pethema trial. Haematologica 2015;100:1096-102.
27. Mey UJM, Brugger W, Schwarb H, et al. Bendamustine, lenalidomide and dexamethasone (BRd) has high activity as 2nd-line therapy for relapsed and refractory multiple myeloma - a phase II trial. Br J Haematol 2017;176:770-82.
28. Ludwig H, Kasparu H, Leitgeb C, et al. Bendamustine-bortezomib-dexamethasone is an active and well-tolerated regimen in patients with relapsed or refractory multiple myeloma. Blood 2014;123:985-91.
29. Michael M, Bruns I, Bölke E, et al. Bendamustine in patients with relapsed or refractory multiple myeloma. Eur J Med Res 2010;15:13.
30. Swerdlow SH, Campo E, Pileri SA, et al. The 2016 revision of the World Health Organization classification of lymphoid neoplasms. Blood 2016;127:2375-90.
31. Alizadeh AA, Alizadeh AA, Eisen MB, et al. Distinct types of diffuse large B-cell lymphoma identified by gene expression profiling. Nature 2000;403:503-11.
32. Bergsagel PL, Kuehl WM, Zhan F, et al. Cyclin D dysregulation: an early and unifying pathogenic event in multiple myeloma. Blood 2005;106:296-303.
33. Dybkær K, Bøgsted M, Falgreen S, et al. Diffuse large B-cell lymphoma classification system that associates normal B-cell subset phenotypes with prognosis. J Clin Oncol 2015;33:1379-88.
34. Bødker JS, Brøndum RF, Schmitz A, et al. A multiple myeloma classification system that associates normal B-cell subset phenotypes with prognosis. Blood Adv 2018;2:2400-11.
35. Falgreen S, Dybkær K, Young KH, et al. Predicting response to multidrug regimens in cancer patients using cell line experiments and regularised regression models. BMC Cancer 2015;15:1-15.
36. Falgreen S, Laursen MB, Bødker JS, et al. Exposure time independent summary statistics for assessment of drug dependent cell line growth inhibition. BMC Bioinformatics 2014;15:168.
37. Bøgsted M, Bilgrau AE, Wardell CP, et al. Proof of the concept to use a malignant B cell line drug screen strategy for identification and weight of melphalan resistance genes in multiple myeloma. In: Wallace GR, editor. PLoS One 2013. p. e83252.
38. Laursen MB, Falgreen S, Bødker JS, et al. Human B-cell cancer cell lines as a preclinical model for studies of drug effect in diffuse large B-cell lymphoma and multiple myeloma. Exp Hematol 2014;42:927-38.
39. Due H, Brøndum RF, Young KH, Bøgsted M, Dybkær K. MicroRNAs associated to single drug components of R-CHOP identifies diffuse large B-cell lymphoma patients with poor outcome and adds prognostic value to the international prognostic index. BMC Cancer 2020;20:237.
40. Boegsted M, Holst JM, Fogd K, et al. Generation of a predictive melphalan resistance index by drug screen of B-cell cancer cell lines. In: Cheriyath V, editor. PLoS One 2011. p. e19322.
41. Michaelsen TY, Richter J, Brøndum RF, et al. A B-cell-associated gene signature classification of diffuse large B-cell lymphoma by NanoString technology. Blood Adv 2018;2:1542-6.
42. Visco C, Li Y, Xu-Monette ZY, et al. Comprehensive gene expression profiling and immunohistochemical studies support application of immunophenotypic algorithm for molecular subtype classification in diffuse large B-cell lymphoma: a report from the International DLBCL Rituximab-CHOP Consortiu. Leukemia 2012;26:2103-13.
43. Lenz G, Wright G, Dave SS, et al. Stromal gene signatures in large-B-cell lymphomas. N Engl J Med 2008;359:2313-23.
44. Monti S, Chapuy B, Takeyama K, et al. Integrative analysis reveals an outcome-associated and targetable pattern of p53 and cell cycle deregulation in diffuse large B cell lymphoma. Cancer Cell 2012;22:359-72.
45. Broyl A, Hose D, Lokhorst H, et al. Gene expression profiling for molecular classification of multiple myeloma in newly diagnosed patients. Blood 2010;116:2543-53.
46. Dickens NJ, Walker BA, Leone PE, et al. Homozygous deletion mapping in myeloma samples identifies genes and an expression signature relevant to pathogenesis and outcome. Clin Cancer Res 2010;16:1856-64.
47. Shi L, Campbell G, Jones WD, et al. The MicroArray Quality Control (MAQC)-II study of common practices for the development and validation of microarray-based predictive models. Nat Biotechnol 2010;28:827-38.
48. R Core Team. R: a language and environment for statistical computing. [Internet]. Vienna, Austria: 2020.
49. Emerson JW, Green WA, Schloerke B, et al. The generalized pairs plot. J Comput Graph Stat 2013;22:79-91.
50. McCall MN, Bolstad BM, Irizarry RA. Frozen robust multiarray analysis (fRMA). Biostatistics 2010;11:242-53.
51. Friedman J, Hastie T, Tibshirani R. Regularization paths for generalized linear models via coordinate descent. J Stat Softw 2010;33:7-10.
52. Kassambara A, Kosinski M, Biecek P, Fabian S. Survminer: drawing survival curves using “ggplot2” version 0.4.8. CRAN. 2020; (version 0.4.8). Available from: https://rdrr.io/cran/survminer/. [Last accessed on 12 Nov 2020].
53. Zhang J, Grubor V, Love CL, et al. Genetic heterogeneity of diffuse large B-cell lymphoma. Proc Natl Acad Sci 2013;110:1398-403.
55. Bogeljić Patekar M. Bendamustine: an old drug in the new era for patients with non-hodgkin lymphomas and chronic lymphocytic leukemia. Acta Clin Croat 2018;57:542-53.
56. Zeremski V, Jentsch-Ullrich K, Kahl C, et al. Is bendamustine-rituximab a reasonable treatment in selected older patients with diffuse large B cell lymphoma? Results from a multicentre, retrospective study. Ann Hematol 2019;98:2729-37.
57. Kost SEF, Bouchard EDJ, LaBossière É, et al. Cross-resistance and synergy with bendamustine in chronic lymphocytic leukemia. Leuk Res 2016;50:63-71.
58. Hiraoka N, Kikuchi J, Yamauchi T, et al. Purine analog-like properties of bendamustine underlie rapid activation of DNA damage response and synergistic effects with pyrimidine analogues in lymphoid malignancies. In: Akagi T, editor. PLoS One 2014. p. e90675.
59. Palmer AC, Chidley C, Sorger PK. A curative combination cancer therapy achieves high fractional cell killing through low cross-resistance and drug additivity. Elife 2019;8:189-91.
60. Niu N, Wang L. In vitro human cell line models to predict clinical response to anticancer drugs. Pharmacogenomics 2015;16:273-85.
61. Quentmeier H, Pommerenke C, Dirks WG, et al. The LL-100 panel: 100 cell lines for blood cancer studies. Sci Rep 2019;9:1-14.
Cite This Article
Export citation file: BibTeX | EndNote | RIS
OAE Style
Issa II, Brøndum RF, Due H, Schmidt L, Bøgsted M, Dybkær K. A bendamustine resistance gene signature in diffuse large B-cell lymphoma and multiple myeloma. Cancer Drug Resist 2021;4:208-22. http://dx.doi.org/10.20517/cdr.2020.76
AMA Style
Issa II, Brøndum RF, Due H, Schmidt L, Bøgsted M, Dybkær K. A bendamustine resistance gene signature in diffuse large B-cell lymphoma and multiple myeloma. Cancer Drug Resistance. 2021; 4(1): 208-22. http://dx.doi.org/10.20517/cdr.2020.76
Chicago/Turabian Style
Issa Ismail Issa, Rasmus Froberg Brøndum, Hanne Due, Linnéa Schmidt, Martin Bøgsted, Karen Dybkær. 2021. "A bendamustine resistance gene signature in diffuse large B-cell lymphoma and multiple myeloma" Cancer Drug Resistance. 4, no.1: 208-22. http://dx.doi.org/10.20517/cdr.2020.76
ACS Style
Issa, II.; Brøndum RF.; Due H.; Schmidt L.; Bøgsted M.; Dybkær K. A bendamustine resistance gene signature in diffuse large B-cell lymphoma and multiple myeloma. Cancer Drug Resist. 2021, 4, 208-22. http://dx.doi.org/10.20517/cdr.2020.76
About This Article
Copyright
Data & Comments
Data
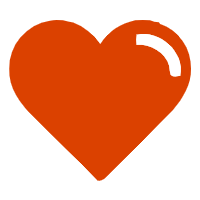
Comments
Comments must be written in English. Spam, offensive content, impersonation, and private information will not be permitted. If any comment is reported and identified as inappropriate content by OAE staff, the comment will be removed without notice. If you have any queries or need any help, please contact us at support@oaepublish.com.