Intraoperative imaging techniques for robotic-assisted partial nephrectomy: where do we stand?
Abstract
Robot-assisted partial nephrectomy is currently the gold standard treatment for localized selected cT1 and cT2 renal tumors. This narrative review aims to analyze the technologies employed in this procedure to increase the precision and accuracy of the surgeon, in order to obtain adequate oncological radicality, negative surgical margins, and good preservation of renal function. In this scenario, new technologies are developing, from three-dimensional reconstructions to artificial intelligence up to the new concept of metaverse.
Keywords
INTRODUCTION
Partial nephrectomy (PN) has emerged as the standard of care for treating selected cT1 and cT2 renal cell tumors, with oncological outcomes comparable to radical nephrectomy and maximal preservation of renal function[1]. Robot-assisted partial nephrectomy (RAPN) has become a widespread nephron-sparing surgery (NSS) modality due to its technical advantages and shortened learning curve compared to laparoscopic partial nephrectomy (LPN) while achieving comparable perioperative outcomes[2]. In performing NSS, the urologist aims to achieve the Trifecta, defined by three criteria: negative surgical margins (NSM), a limited warm ischemia time (WIT), and the absence of complications[3]. However, sometimes NSS can be challenging, resulting in a complete resection of the tumor. Marszalek et al. have highlighted a rate of patients with positive surgical margins (PSM) after NSS ranges from 0% to 7%, and in the case of single or bilateral renal cell carcinoma (RCC), it rises to 18%[4]. Intraoperative imaging can help perform a cancer-safe partial nephrectomy by allowing a better localization of the tumor, differentiating it from normal renal tissue. Imaging is essential to guide the surgeon and provide a complete study of the anatomy of the patient. To date, conventional two-dimensional (2D) imaging does not allow an optimal evaluation. However, several technological advances have emerged in recent years. In this narrative review, current and emerging imaging technologies that can aid in performing a RAPN will be explored.
Literature search strategy
A literature review was performed by analyzing different databases (PubMed, MEDLINE, Research Gate, and Google Scholar) using the following keywords: “RAPN”, “intraoperative imaging”, “renal cancer”, “3-D imaging”, “robotic surgery”, “augmented reality”, and “artificial intelligence”. First, articles from the last decade were selected and evaluated based on their titles and abstracts. Then, some older articles were included based on their relevance to the topic. All study designs and publication types (reviews, narratives, or trials) were considered. Finally, the bibliographic references of the various selected articles were also analyzed.
INTRAOPERATIVE IMAGING TECHNIQUES
Ultrasonography
Intraoperative ultrasound (IOUS) has long been used as a real-time intraoperative imaging technique during PN, as it helps, with a simple and effective approach, to identify endophytic tumors, evaluate tumor characteristics, delineate tumor border and renal perfusion during clamping, with a simple and effective approach[5-7]. The frequency normally used for the laparoscopic or robotic probe is between 7.5 and 10 Mhz, enabling the detection of tumors up to 3-4 mm in diameter[8]. During RAPN, both the assistant and the surgeon can control the laparoscopic ultrasound probe. Usually, the probe control by the console of the surgeon allows for greater autonomy and accuracy[5].
Doppler mode and contrast-enhanced ultrasound (CEUS) allow for better visualization of renal tumors[9]. Hyams et al. presented a prospective evaluation of using the laparoscopic Doppler ultrasound (LDU) to accelerate hilar dissection during RAPN[10]. Their study demonstrates a reduction in hilar dissection time, enhanced detection of accessory vessels (AV) compared to preoperative imaging, adjustments in operational management based on AV detection, and a change in arterial clamp management.
CEUS is extremely useful in identifying renal tumors. One of the latest generation contrast agents is SonoVue (Bracco, Milan, Italy), containing stabilized microbubbles of sulfur hexachloride gas[11]. Additionally, it appears to be non-nephrotoxic, being eliminated by the lungs, and can be used in patients with impaired renal function. The recommended dose for renal imaging is 1-2.4 mL and can be repeated if necessary. The intraoperative CEUS technique uses two types of images: a conventional 2D image (brightness mode), and a contrast-enhanced mode (a contrast-specific image) that shows the deflection produced by the distribution bubble space[8].
IOUS enables us to characterize an intraparenchymal and complex lesion according to the nephrometric score[12], identify the distal portion of a venous thrombus[13], and perform a more careful evaluation in cases of multifocality[14] and associated renal cysts[15]. It can also help reduce the operative time and the WIT because it improves the delimitation of the mass, especially when there is particularly dense perirenal adipose tissue[8]. CEUS, by providing real-time imaging of the renal vasculature, can further reduce the WIT, favoring the selective clamping process of the arteries that supply blood to the segment of the kidney containing the tumor[16]. This allows for avoiding global ischemia and further decreasing the loss of nephrons.
Fluorescence technology
Intraoperative fluorescence imaging employs special fluorescent dyes emitting light in the near-infrared (NIR) range (700-1,000 nm) and a high-resolution fluorescence camera system to detect NIR light, which is invisible to the human eye[17]. The NIR fluorescent dye most used in urological surgery is indocyanine green (ICG)[18]. ICG can be used to allow the identification of major renal artery branches and perform selective and super-selective clamping, reducing WIT and the portion of renal parenchyma subject to ischemia[19,20], leading to preservation of postoperative upper estimated glomerular filtration rate (eGFR)[21,22]. The limit value of WIT, during PN, to avoid damage to renal function, is commonly believed to be 20-25 min. However, according to some authors, a WIT > 30 min may still not affect renal function, especially in patients with both functioning kidneys after on-clamp PN[23]. On the other hand, the off-clamp (OC) approach would seem to best preserve postoperative functionality despite a greater risk of intraoperative bleeding with reduced vision and greater surgical difficulty for the operator[24]. ICG also allows the differentiation of healthy kidney tissue from tumor tissue, as its binding to a transmembrane protein known as bilitranslocase would allow ICG to spread within cells and into the proximal convoluted tubules of healthy tissue but not into the tumor [Figure 1]. This particular distribution of ICG would make fluorescent normal renal parenchyma under NIR light and hypofluorescent tumor tissue[18]. The success rate in this differentiation varies between 73% and 100% of cases[19]. This variability would also seem to be linked to the lack of standardization of the ICG dosage: in fact, an underdosage can be associated with hypofluorescence of the normal parenchyma, and an overdosage can make the tumor fluorescent. In general, an ICG dose of 0.25-0.50 mL can ensure good differential fluorescence[25].
Figure 1. RAPN using NIR fluorescent imaging with ICG. Renal tumor is seen under (A) white light and under (B) NIRF imaging with ICG. It appears hypofluorescent, adjacent to bright green normal renal parenchyma. RAPN: Robot-assisted partial nephrectomy; NIR: near-infrared; ICG: indocyanine green; NIRF: near-infrared fluorescence.
Simone et al. proposed a technique, “green light riding”, to simplify challenging RAPN based on ICG super-selective transarterial tumor marking[26]. They selected ten consecutive patients with total endophytic renal masses and performed purely OC RAPN following super-selective transarterial delivery of ICG-lipiodol mixture.
In this regard, in recent times, thanks to the widespread diffusion of robotic surgery and the high surgical expertise acquired, the indications for RAPN have expanded, also including particularly complex cases, for example, totally endophytic renal tumors (TERTs)[27]. These tumors are surrounded by healthy renal parenchyma without altering the external profile of the kidney. As highlighted by Tuderti et al., IOUS could prove to be a useful tool, allowing the identification of the mass and the definition of its depth and margins before proceeding to resection[28]. In particular, the preoperative marking of the endophytic mass by super-selective transarterial administration of the lipiodol-ICG mixture and the continuous real-time switching to NIR fluorescent imaging and conventional white light allows for improving the vision of a surgeon during enucleation, minimizing lesions of the healthy subject kidney parenchyma and offering a shorter operating time.
Other emerging approaches consist of using a fluorescent dye conjugated to a specific tumor molecule, such as OTL38 (On Target Laboratories LLC., West Lafayette, IN, USA), a folate-targeted, intraoperative fluorescence agent, highly expressed in the healthy kidney tissue, as opposed to tumor tissue[29]. Another target under evaluation is carbonic anhydrase IX, which is highly expressed in clear cell RCC (ccRCC) but not in normal renal tissue. In this case, girentuximab, a monoclonal antibody, can be used for targeting carbonic anhydrase IX[30]. Recently, a combination of radio-guided and fluorescence-guided surgery has been proposed. Hekman et al. performed a study regarding ex vivo perfusion of renal tumors, evaluating the feasibility and reproducibility of dual imaging modalities using ¹¹¹I-girentuximab IRDye800CW[31]. The synergy of the two techniques would allow for more precise intraoperative imaging of the tumor, but further studies are needed to support its validity.
WHERE DO WE STAND?
Pathological intraoperative imaging
The goal of the urologist in performing a RAPN is certainly to achieve oncological radicality, trying to preserve renal function as much as possible and obtain NSM[3]. For these reasons, research also focuses on new intraoperative pathological imaging techniques, such as ex vivo confocal microscopy, fluorescence confocal endomicroscopy, and optical coherence tomography (OCT). Intraoperative frozen section remains, to date, the main technique for the pathological examination of surgical margins.
Confocal microscopy is a modality of light sectioning, which captures 2D images of tissue and reconstructs a three-dimensional (3D) image. The confocal laser makes it possible to identify cellular structures with an accuracy almost comparable to traditional histological techniques[32]. To date, confocal microscopy finds application in various clinical fields and above all oncology. Given its detailed resolution, it could be useful in evaluating surgical margins in renal tumors and be used as an optical biopsy[33], thus reducing the high costs, delays, and potential morbidity associated with frozen sections[34]. It is certainly a promising modality that would allow an accurate diagnosis of the surgical margin. However, further studies are needed to validate the use of confocal microscopy instead of frozen sections.
Fluorescence confocal microscopy (FMC) is a technology that uses two different wavelengths of lasers and allows real-time microscopic examination of excised tissues and a visualization of cells and structures with histologic resolution. This technique was applied to prostate tissue[35], and then it was also used in the context of renal biopsy showing a high diagnostic accuracy[36]. Recently, it could be a feasible and reproducible method for the intraoperative assessment of urethral and ureteral surgical margins during radical cystectomy[37].
OCT is an imaging technique that allows real-time cross-sectional imaging of tissue with a resolution ten times higher than that achievable with ultrasound, using infrared light. Linehan et al. demonstrated that OCT was most successful in distinguishing angiomyolipoma and transitional cell carcinoma from normal parenchyma, while clear cell tumors had a heterogeneous appearance on OCT, which precluded reliable differentiation from normal parenchyma and between renal carcinoma subtypes[38]. Hekman et al. have shown its ability to differentiate between benign and malignant renal tissue[39]. The next step will certainly be to evaluate these technologies in vivo and evaluate their effective potential in renal surgery.
3D reconstruction and augmented reality
In the field of urology, 3D virtual reality (VR) is gaining attention, especially in developing models for planning and simulating complex surgical procedures, such as conservative renal surgery[40]. To generate 3D kidney models, computed tomography (CT) and magnetic resonance imaging (MRI) can be used[41,42]. CT represents the imaging technique of choice for renal tumors. MRI is a valid alternative but is currently employed in cases of CT-indeterminate renal tumors or impaired renal function[43]. A clear understanding of the anatomy, location, and size of the tumor, along with the relationship of the renal tumor to the normal parenchyma, collecting system, and vascular structures, is critical to achieving a successful surgical outcome in RAPN. Additionally, 3D imaging has made it possible to obtain a better evaluation of these aspects by the surgeon, compared to the original 2D imaging, thanks also to the possibility of manipulating the anatomical structures at 360° along all axes, for better evaluating intraparenchymal anatomy and tumor extent[44]. Bertolo et al., in their study, demonstrated the reliability of 3D VR in the preoperative planning of complex renal tumors amenable to conservative surgery, especially for the more accurate management of the renal peduncle compared to standard 2D imaging-based planning[45]. As regards the prediction of the surgical complexity of a renal tumor, several nephrometric systems have been developed in recent years, such as the PADUA and RENAL score[46,47]. Several recent studies have focused on a quantitative analysis of nephrometry scores based on 3D imaging, finding a higher level of accuracy than 2D-based scoring systems, especially in predicting major intra- and perioperative complications[48,49]. Huang et al. have developed a scoring system termed ROADS that provides a standardized and quantitative 3D anatomical classification to stratify renal sinus tumors and guide surgical strategy[50]. A high ROADS score has been correlated with greater surgical complexity, such as longer operative and ischemia times and higher estimated blood loss and complication rates. Another important aspect is the relationship between postoperative renal function and the volume of the resected cortical margin, calculated using the 3D reconstruction technique. The margin volume of the healthy cortex calculated with the reconstruction technique was found to be an independent risk factor for impaired postoperative renal function[51]. Using precise 3D volumetric analysis, Meyer et al. demonstrated that ischemia time, tumor size, and endophytic/exophytic characteristics of a renal mass are the most important determinants of renal parenchymal volume loss[52]. Furthermore, 3D reconstruction techniques could provide an important aid for the super-selective clamping of renal artery branches during RAPN for complex renal masses, allowing for reducing renal damage caused by ischemia[53].
Augmented reality (AR) consists of the superimposition of acquired images acquired from the intraoperative or preoperative field on real images or videos of a patient in real time[54]. First, to perform an AR procedure, it is necessary to acquire a 3D surgical model reconstruction, starting from standard images. Subsequently, the image must be registered on the real anatomy, also obtaining the tracking of the surgical instruments, the movements of the target organs, and the adjacent anatomical structures[55]. Registration is the process by which different sets of data are aligned into a single coordinate system such that the positions of the corresponding points match in the best way possible[56]. However, the superimposition of the images requires very high accuracy and precision. The most important aspects to be evaluated in the image superimposition in AR are the movements of the organs and the deformation of the tissues[56]. A possible solution to this problem may be using organ detectors to correct their breathing-related movements over time. However, another important problem remains, represented by tissue deformation. Surgical dissection, tumor characteristics, bleeding, arterial clamping, respiration, and pneumoperitoneum are the main factors implicated in tissue deformation. To date, this deformation can only be partially calculated by current computer models[57], but at present, this problem can be overcome by using real-time intraoperative imaging instead of preoperative imaging for image overlay.
One possibility is represented by IOUS superimposition[58], although it could be complex for the surgeon to perform a resection at the same time as using the ultrasound probe, as this is in close contact with the surface of the kidney. Intraoperative cone beam CT (CBCT) with intraoperative contrast represents another real-time imaging alternative[56]. Also, in this case, the possible interference of the CBCT C-arm with the robot arms could represent a significant problem, requiring the temporary disconnection of the robot and the use of a hybrid operating room and dedicated imaging technology.
Artificial intelligence
Artificial intelligence (AI) is defined as the ability of machines to perform tasks and solve problems for which they were not explicitly programmed. In recent years, the interest in AI has increased significantly as it contributes to improving diagnostic and therapeutic accuracy in clinical practice. Currently, it finds application in the diagnosis, management, perioperative care, and follow-up of RCC. However, it is crucial that healthcare professionals develop a basic understanding of the algorithms and decision-making process of the applied AI method, often perceived as difficult to understand, to implement its use in daily practice[59].
The goal of AI is to build a machine capable of perceiving its environment and performing activities, trying to obtain the maximum probability of success. This process makes use of different subcategories of AI such as machine learning (ML), artificial neural networks (ANN) and deep learning (DL), natural language processing (NLP), computer vision, predictive analytics, genetic informatics, expert systems, visual recognition, and speech processing[60]. ML and DL algorithms based on CT texture analysis have been applied to differentiate renal masses[61], predict nuclear grade, identify genetic mutations, and predict prognosis, recurrence risk, and overall survival (OS)[62]. Kocak et al. applied ML techniques to identify the nuclear grade of ccRCC and compare the results with those obtained with percutaneous biopsy[63]. They found that the algorithm could differentiate the nuclear grades in 85.1% of ccRCC cases. Ding et al. conducted a study using AI to achieve higher accuracy in classifying the degree of ccRCC[64]. Other researchers have focused on tumor gene expression to predict OS and prognosis[65]. Kocak et al., in another study, applied algorithms based on ANN and ML to detect polybromo-1 (PBRM1) mutations, common in ccRCC, allowing for identifying up to 88% of ccRCCs with PBRM1 mutation status[66].
In regards to robotic surgery, AI has mostly been used for proficiency assessment in robot-assisted prostate surgery. Recently, some studies have also focused on RAPN. Nakawala et al. proposed in their study the combined use of DL and knowledge management tools for surgical workflow recognition of RAPN[67]. AI would help the surgeon dissect the hilum during RAPN by detecting the slight movements of tissue surfaces related to the pulsation of hidden blood vessels[68].
CONCLUSIONS
RAPN is a complex surgery for which various techniques have been developed to allow better intraoperative visualization of the tumor, ensure more accurate vascular dissection, and facilitate overall tumor resection, with an increasingly precise evaluation of the surgical margins. Ultrasound and fluorescence imaging are techniques widely used to assist the surgeon in RAPN. IGC is particularly advantageous for differentiating healthy tissue from tumor tissue, especially in the case of exophytic versus endophytic tumors, due to the shallow penetration depth of light into the tissue. This limitation could be exceeded using the mode combination of radiolabel with fluorescence imaging. However, further studies are needed in this field to demonstrate this real benefit. Each technique has its advantages and limitations. An adequate preoperative evaluation of the patient is certainly essential, which will allow for identifying the most useful imaging technique to support the surgeon during RAPN, guaranteeing an adequate resection of the tumor tissue, and minimizing the risk of PSM.
In recent years, 3D reconstructions and their application in robotic surgery, particularly regarding RAPN, have become increasingly widespread. Next-generation 3D models reproduce anatomy and assist surgeons in decision-making and intraoperative navigation. The integration of 3D models with robotic procedures and the use of AR make it possible to improve surgical precision in treating the pathology, with increasingly greater personalization of the procedures[69].
The application of AI in surgery is the most interesting research field. It is being explored to create systems that can suggest treatment options to clinicians and provide greater operational insights. This is currently one of the main trends in scientific research applied to minimally invasive robotic surgery. However, its implementation in clinical practice remains limited due to a lack of reproducibility, well-defined quality control and regulation, and the need for external validation. Further studies are certainly required to maximize the potential of this technology at the service of the surgeon and the patient. We will reach the so-called “extended reality”, which includes VR, AR, and mixed reality (MR), new applications that will allow interactions with hybrid digital/real environments[70].
In the coming years, integrating extended reality and AI into a 5G wireless network will take doctors into the “metaverse”[71], a VR where 3D models, holograms, AR, radiomics, and genomics can improve healthcare, thanks to the sharing of information enhanced by these new technologies.
DECLARATIONS
Acknowledgments
The author thanks Prof. Michele Marchioni and Prof. Luigi Schips for their precious support.
Authors’ contributions
The author contributed solely to the article.
Availability of data and materials
Not applicable.
Financial support and sponsorship
None.
Conflicts of interest
Not applicable.
Ethical approval and consent to participate
Not applicable.
Consent for publication
Not applicable.
Copyright
© The Author(s) 2024.
REFERENCES
1. Ljungberg B, Albiges L, Abu-Ghanem Y, et al. European Association of Urology Guidelines on renal cell carcinoma: the 2022 update. Eur Urol 2022;82:399-410.
2. Benway BM, Bhayani SB, Rogers CG, et al. Robot assisted partial nephrectomy versus laparoscopic partial nephrectomy for renal tumors: a multi-institutional analysis of perioperative outcomes. J Urol 2009;182:866-72.
3. Kim DK, Kim LH, Raheem AA, et al. Comparison of trifecta and pentafecta outcomes between T1a and T1b renal masses following robot-assisted partial nephrectomy (RAPN) with minimum one year follow up: can RAPN for T1b renal masses be feasible? PLoS One 2016;11:e0151738.
4. Marszalek M, Carini M, Chlosta P, et al. Positive surgical margins after nephron-sparing surgery. Eur Urol 2012;61:757-63.
5. Kaczmarek BF, Sukumar S, Petros F, et al. Robotic ultrasound probe for tumor identification in robotic partial nephrectomy: initial series and outcomes. Int J Urol 2013;20:172-6.
6. Zhang Y, Ouyang W, Wu B, et al. Robot-assisted partial nephrectomy with a standard laparoscopic ultrasound probe in treating endophytic renal tumor. Asian J Surg 2020;43:423-7.
7. Puliatti S, Eissa A, Checcucci E, et al. New imaging technologies for robotic kidney cancer surgery. Asian J Urol 2022;9:253-62.
8. Di Cosmo G, Verzotti E, Silvestri T, et al. Intraoperative ultrasound in robot-assisted partial nephrectomy: state of the art. Arch Ital Urol Androl 2018;90:195-8.
9. Correas JM, Anglicheau D, Joly D, Gennisson JL, Tanter M, Hélénon O. Ultrasound-based imaging methods of the kidney-recent developments. Kidney Int 2016;90:1199-210.
10. Hyams ES, Perlmutter M, Stifelman MD. A prospective evaluation of the utility of laparoscopic Doppler technology during minimally invasive partial nephrectomy. Urology 2011;77:617-20.
12. Rosiello G, Piazza P, Puliatti S, et al. Simplified PADUA renal (SPARE) nephrometry score validation and long-term outcomes after robot-assisted partial nephrectomy. Urol Oncol 2022;40:65.e1-9.
13. Hsu TH, Jeffrey RB Jr, Chon C, Presti JC Jr. Laparoscopic radical nephrectomy incorporating intraoperative ultrasonography for renal cell carcinoma with renal vein tumor thrombus. Urology 2003;61:1246-8.
14. Campbell SC, Fichtner J, Novick AC, et al. Intraoperative evaluation of renal cell carcinoma: a prospective study of the role of ultrasonography and histopathological frozen sections. J Urol 1996;155:1191-5.
15. Polascik TJ, Meng MV, Epstein JI, Marshall FF. Intraoperative sonography for the evaluation and management of renal tumors: experience with 100 patients. J Urol 1995;154:1676-80.
16. Alenezi AN, Karim O. Role of intra-operative contrast-enhanced ultrasound (CEUS) in robotic-assisted nephron-sparing surgery. J Robot Surg 2015;9:1-10.
17. Keereweer S, Van Driel PBAA, Snoeks TJA, et al. Optical image-guided cancer surgery: challenges and limitations. Clin Cancer Res 2013;19:3745-54.
18. Gadus L, Kocarek J, Chmelik F, Matejkova M, Heracek J. Robotic partial nephrectomy with indocyanine green fluorescence navigation. Contrast Media Mol Imaging 2020;2020:1287530.
19. Bjurlin MA, McClintock TR, Stifelman MD. Near-infrared fluorescence imaging with intraoperative administration of indocyanine green for robotic partial nephrectomy. Curr Urol Rep 2015;16:20.
20. Diana P, Buffi NM, Lughezzani G, et al. The role of intraoperative indocyanine green in robot-assisted partial nephrectomy: results from a large, multi-institutional series. Eur Urol 2020;78:743-9.
21. McClintock TR, Bjurlin MA, Wysock JS, et al. Can selective arterial clamping with fluorescence imaging preserve kidney function during robotic partial nephrectomy? Urology 2014;84:327-32.
22. Mattevi D, Luciani LG, Mantovani W, et al. Fluorescence-guided selective arterial clamping during RAPN provides better early functional outcomes based on renal scan compared to standard clamping. J Robot Surg 2019;13:391-6.
23. Abdel Raheem A, Alowidah I, Capitanio U, et al. Warm ischemia time length during on-clamp partial nephrectomy: does it really matter? Minerva Urol Nephrol 2022;74:194-202.
24. Simone G, Ferriero M, Papalia R, Costantini M, Guaglianone S, Gallucci M. Zero-ischemia minimally invasive partial nephrectomy. Curr Urol Rep 2013;14:465-70.
25. Sentell KT, Ferroni MC, Abaza R. Near-infrared fluorescence imaging for intraoperative margin assessment during robot-assisted partial nephrectomy. BJU Int 2020;126:259-64.
26. Simone G, Tuderti G, Anceschi U, et al. “Ride the green light”: indocyanine green-marked off-clamp robotic partial nephrectomy for totally endophytic renal masses. Eur Urol 2019;75:1008-14.
27. Autorino R, Khalifeh A, Laydner H, et al. Robot-assisted partial nephrectomy (RAPN) for completely endophytic renal masses: a single institution experience. BJU Int 2014;113:762-8.
28. Tuderti G, Brassetti A, Mastroianni R, et al. Expanding the limits of nephron-sparing surgery: surgical technique and mid-term outcomes of purely off-clamp robotic partial nephrectomy for totally endophytic renal tumors. Int J Urol 2022;29:282-8.
29. Sulek JE, Steward JE, Bahler CD, et al. Folate-targeted intraoperative fluorescence, OTL38, in robotic-assisted laparoscopic partial nephrectomy. Scand J Urol 2021;55:331-6.
30. Povoski SP, Hall NC, Murrey DA Jr, et al. Multimodal imaging and detection strategy with 124 I-labeled chimeric monoclonal antibody cG250 for accurate localization and confirmation of extent of disease during laparoscopic and open surgical resection of clear cell renal cell carcinoma. Surg Innov 2013;20:59-69.
31. Hekman MC, Boerman OC, de Weijert M, et al. Targeted dual-modality imaging in renal cell carcinoma: an ex vivo kidney perfusion study. Clin Cancer Res 2016;22:4634-42.
32. Phung MC, Rouse AR, Pangilinan J, et al. Investigation of confocal microscopy for differentiation of renal cell carcinoma versus benign tissue. Can an optical biopsy be performed? Asian J Urol 2020;7:363-8.
33. Su LM, Kuo J, Allan RW, et al. Fiber-optic confocal laser endomicroscopy of small renal masses: toward real-time optical diagnostic biopsy. J Urol 2016;195:486-92.
34. Gordetsky J, Gorin MA, Canner J, et al. Frozen section during partial nephrectomy: does it predict positive margins? BJU Int 2015;116:868-72.
35. Puliatti S, Bertoni L, Pirola GM, et al. Ex vivo fluorescence confocal microscopy: the first application for real-time pathological examination of prostatic tissue. BJU Int 2019;124:469-76.
36. Mir MC, Bancalari B, Calatrava A, et al. Ex-vivo confocal fluorescence microscopy for rapid evaluation of renal core biopsy. Minerva Urol Nefrol 2020;72:109-13.
37. Prata F, Anceschi U, Taffon C, et al. Real-time urethral and ureteral assessment during radical cystectomy using ex-vivo optical imaging: a novel technique for the evaluation of fresh unfixed surgical margins. Curr Oncol 2023;30:3421-31.
38. Linehan JA, Bracamonte ER, Hariri LP, et al. Feasibility of optical coherence tomography imaging to characterize renal neoplasms: limitations in resolution and depth of penetration. BJU Int 2011;108:1820-4.
39. Hekman MCH, Rijpkema M, Langenhuijsen JF, Boerman OC, Oosterwijk E, Mulders PFA. Intraoperative imaging techniques to support complete tumor resection in partial nephrectomy. Eur Urol Focus 2018;4:960-8.
40. Esperto F, Prata F, Autrán-Gómez AM, et al. New technologies for kidney surgery planning 3D, impression, augmented reality 3D, reconstruction: current realities and expectations. Curr Urol Rep 2021;22:35.
42. Papalia R, Panebianco V, Mastroianni R, et al. Accuracy of magnetic resonance imaging to identify pseudocapsule invasion in renal tumors. World J Urol 2020;38:407-15.
43. Kim JH, Sun HY, Hwang J, et al. Diagnostic accuracy of contrast-enhanced computed tomography and contrast-enhanced magnetic resonance imaging of small renal masses in real practice: sensitivity and specificity according to subjective radiologic interpretation. World J Surg Oncol 2016;14:260.
44. Moldovanu CG, Lebovici A, Buruian MM. A systematic review of the clinical value and applications of three-dimensional virtual reconstructions in renal tumors. Med Pharm Rep 2022;95:11-23.
45. Bertolo R, Autorino R, Fiori C, et al. Expanding the indications of robotic partial nephrectomy for highly complex renal tumors: urologists’ perception of the impact of hyperaccuracy three-dimensional reconstruction. J Laparoendosc Adv Surg Tech A 2019;29:233-9.
46. Porpiglia F, Amparore D, Checcucci E, et al. Three-dimensional virtual imaging of renal tumours: a new tool to improve the accuracy of nephrometry scores. BJU Int 2019;124:945-54.
47. Tuderti G, Mastroianni R, Anceschi U, et al. Assessing the trade-off between the safety and effectiveness of off-clamp robotic partial nephrectomy for renal masses with a high RENAL score: a propensity score-matched comparison of perioperative and functional outcomes in a multicenter analysis. Eur Urol Focus 2023;9:1037-43.
48. Liu J, Liu J, Wang S, et al. Three-dimensional nephrometry scoring system: a precise scoring system to evaluate complexity of renal tumors suitable for partial nephrectomy. PeerJ 2020;8:e8637.
49. Yoshitomi KK, Komai Y, Yamamoto T, et al. Improving accuracy, reliability, and efficiency of the RENAL nephrometry score with 3D reconstructed virtual imaging. Urology 2022;164:286-92.
50. Huang Q, Gu L, Zhu J, et al. A three-dimensional, anatomy-based nephrometry score to guide nephron-sparing surgery for renal sinus tumors. Cancer 2020;126:2062-72.
51. Mitsui Y, Sadahira T, Araki M, et al. The 3-D volumetric measurement including resected specimen for predicting renal function afterrobot-assisted partial nephrectomy. Urology 2019;125:104-10.
52. Meyer A, Woldu SL, Weinberg AC, et al. Predicting renal parenchymal loss after nephron sparing surgery. J Urol 2015;194:658-63.
53. Porpiglia F, Amparore D, Checcucci E, et al. for ESUT Research Group. Current use of three-dimensional model technology in urology: a road map for personalised surgical planning. Eur Urol Focus 2018;4:652-6.
54. Tang SL, Kwoh CK, Teo MY, Sing NW, Ling KV. Augmented reality systems for medical applications. IEEE Eng Med Biol Mag 1998;17:49-58.
55. Checcucci E, Amparore D, Fiori C, et al. 3D imaging applications for robotic urologic surgery: an ESUT YAUWP review. World J Urol 2020;38:869-81.
56. Hughes-Hallett A, Mayer EK, Marcus HJ, et al. Augmented reality partial nephrectomy: examining the current status and future perspectives. Urology 2014;83:266-73.
57. Altamar HO, Ong RE, Glisson CL, et al. Kidney deformation and intraprocedural registration: a study of elements of image-guided kidney surgery. J Endourol 2011;25:511-7.
58. Hughes-Hallett A, Pratt P, Mayer E, et al. Intraoperative ultrasound overlay in robot-assisted partial nephrectomy: first clinical experience. Eur Urol 2014;65:671-2.
59. Kowalewski KF, Egen L, Fischetti CE, et al; Young Academic Urologists (YAU)-Urotechnology-Group. Artificial intelligence for renal cancer: from imaging to histology and beyond. Asian J Urol 2022;9:243-52.
60. Hameed BMZ, S Dhavileswarapu AVL, Raza SZ, et al. Artificial intelligence and its impact on urological diseases and management: a comprehensive review of the literature. J Clin Med 2021;10:1864.
61. Feng Z, Rong P, Cao P, et al. Machine learning-based quantitative texture analysis of CT images of small renal masses: differentiation of angiomyolipoma without visible fat from renal cell carcinoma. Eur Radiol 2018;28:1625-33.
62. Shah M, Naik N, Somani BK, Hameed BMZ. Artificial intelligence (AI) in urology-current use and future directions: an iTRUE study. Turk J Urol 2020;46:S27-39.
63. Kocak B, Yardimci AH, Bektas CT, et al. Textural differences between renal cell carcinoma subtypes: machine learning-based quantitative computed tomography texture analysis with independent external validation. Eur J Radiol 2018;107:149-57.
64. Ding J, Xing Z, Jiang Z, et al. CT-based radiomic model predicts high grade of clear cell renal cell carcinoma. Eur J Radiol 2018;103:51-6.
65. Li P, Ren H, Zhang Y, Zhou Z. Fifteen-gene expression based model predicts the survival of clear cell renal cell carcinoma. Medicine 2018;97:e11839.
66. Kocak B, Durmaz ES, Ates E, Ulusan MB. Radiogenomics in clear cell renal cell carcinoma: machine learning-based high-dimensional quantitative CT texture analysis in predicting PBRM1 mutation status. AJR Am J Roentgenol 2019;212:W55-63.
67. Nakawala H, Bianchi R, Pescatori LE, De Cobelli O, Ferrigno G, De Momi E. “Deep-Onto” network for surgical workflow and context recognition. Int J Comput Assist Radiol Surg 2019;14:685-96.
68. Amir-Khalili A, Hamarneh G, Peyrat JM, et al. Automatic segmentation of occluded vasculature via pulsatile motion analysis in endoscopic robot-assisted partial nephrectomy video. Med Image Anal 2015;25:103-10.
69. Amparore D, Piramide F, De Cillis S, et al; Renal Cancer Working Group of the Young Academic Urologists (YAU) and European Association of Urology (EAU). Robotic partial nephrectomy in 3D virtual reconstructions era: is the paradigm changed? World J Urol 2022;40:659-70.
70. Veneziano D, Amparore D, Cacciamani G, Porpiglia F; Uro-technology; SoMe Working Group of the Young Academic Urologists Working Party of the European Association of Urology; European Section of Uro-technology. Climbing over the barriers of current imaging technology in urology. Eur Urol 2020;77:142-3.
Cite This Article
How to Cite
Download Citation
Export Citation File:
Type of Import
Tips on Downloading Citation
Citation Manager File Format
Type of Import
Direct Import: When the Direct Import option is selected (the default state), a dialogue box will give you the option to Save or Open the downloaded citation data. Choosing Open will either launch your citation manager or give you a choice of applications with which to use the metadata. The Save option saves the file locally for later use.
Indirect Import: When the Indirect Import option is selected, the metadata is displayed and may be copied and pasted as needed.
About This Article
Special Issue
Copyright
Data & Comments
Data
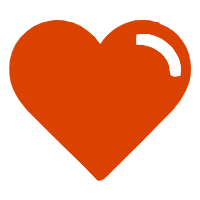
Comments
Comments must be written in English. Spam, offensive content, impersonation, and private information will not be permitted. If any comment is reported and identified as inappropriate content by OAE staff, the comment will be removed without notice. If you have any queries or need any help, please contact us at [email protected].