Webinar
Contents
Host
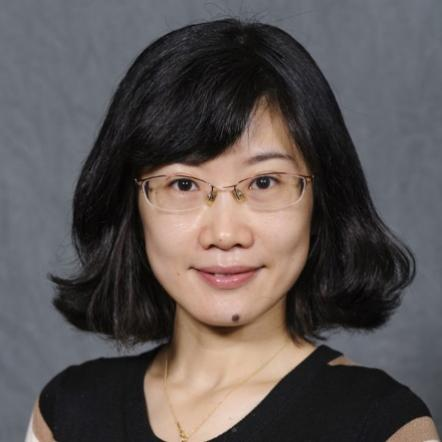
Prof. Lin Li
School for Engineering of Matter, Transport and Energy, Arizona State University, Tempe, AZ, USA.
Dr. Lin Li is an associate professor in the School for Engineering of Matter, Transport and Energy (SEMTE) at Arizona State University (ASU). She holds a Bachelor's degree from Zhejiang University, a Ph.D. degree from The Ohio State University, and worked as a postdoctoral associate at the Massachusetts Institute of Technology. Prior to joining ASU, she was a faculty member at The University of Alabama. Her research interests are in the field of multiscale material mechanics modeling for materials in extreme environments, advanced manufacturing and energy sustainability. Dr. Li is the recipient of the Ralph E. Powe Junior Faculty Enhancement Award and the Air Force Summer Faculty Fellowship. Dr. Li serves as a Youth Editor Board Member of Journal of Materials Informatics.
Speaker
Prof. Yongming Liu
Mechanical and Aerospace Engineering, Arizona State University, Tempe, AZ, USA.
Yongming Liu is a Professor in mechanical and aerospace engineering at Arizona State University and is the founding director of the Center for Complex System Safety – a joint center of Arizona State University, University of Arizona, and Northern Arizona University. He completed his Ph.D. at Vanderbilt University in 2006 and obtained his bachelor's and Master's degrees from Tongji University in China in 1999 and 2002, respectively. Dr. Liu's research interests include scientific machine learning, probabilistic methods, fatigue and fracture, imaging-based experiments, and computation and simulation. He was the recipient of the Air Force Young Investigator Award in 2011. He has worked with various governmental agencies and industrial partners for his research, including NASA, NSF, DOE, DOT, and DOD. He is currently leading a NASA University Leadership Initiative project on information fusion for prognostics and health management, which focuses on developing probabilistic learning methods with extensive data resources for air transportation safety assurance.
Abstract
Machine Learning (ML) has recently been explored in almost every engineering discipline. Classical ML is known to have poor prediction capability as a purely data-driven approach since no physics knowledge is included. This talk presents the concept of physics-guided machine learning (PGML). The PGML methodology encodes the underlying physics of engineering problems into machine learning models and fuses information from abstracted knowledge and observed data together. Three physics-encoding methods are presented to cover the most common engineering problems: encoding in network architecture, encoding in input features, and encoding in output functions. This talk will focus on the network architecture design for PGML. Two types of physics encoding are illustrated. The first type of physics is expressed in terms of rigorous governing equations, such as differential equations. The second type of physics is expressed as phenomenological knowledge, such as trending or range constraints. The first type of physics encoding is illustrated using the convolutional neural networks (CNN)-based method for partial differential equations (PDEs), e.g., computational mechanics for materials. Finite Element Analysis NETwork (FEA-Net) is proposed to solve the mechanics and diffusion problems. FEA-Net is further augmented with learning-based multigrid simulation and solver design for ultra-efficient computing. Following this, another physics embedding is illustrated by encoding prior knowledge for material fatigue analysis. A probabilistic physics-guided neural network architecture is proposed to incorporate known derivative trending constraints. Fatigue life prediction for additively manufactured titanium alloy is demonstrated with varying manufacturing process parameters and X-ray tomography images. The reported studies show several major advantages of physics-guided machine learning: superior prediction/extrapolation capability; significant reduction of training sample requirements; fast computation, especially for high-dimensional and non-homogeneous problems; and interpretable and trustworthy learning results. Several limitations and future research directions are discussed based on the findings from the current study.
Presentation
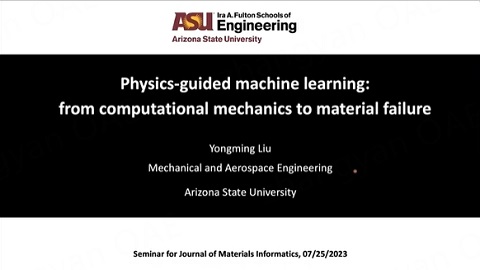
Prof. Yongming Liu
Topic: Physics-Guided Machine Learning: from Computational Mechanics to Material Failure
NaN
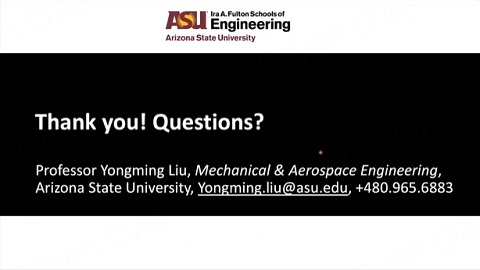
Topic: Free discussion
NaN
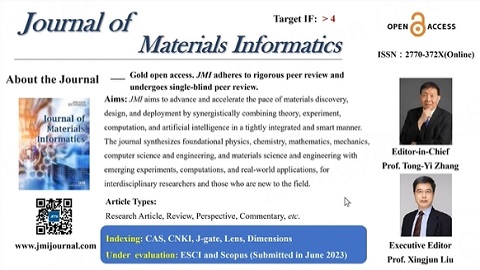
Topic: JMI introduction
NaN