Deep learning for enhanced porosity detection in AZ91 magnesium alloys using windowed perception and aggregated sensing
Abstract
In this study, we innovatively proposed a deep learning model architecture to address the industry challenges in the detection of porosity in magnesium alloys. Magnesium alloys, known for their lightweight and high-strength characteristics, are extensively utilized in aerospace, automotive, and biomedical fields. However, the absorption of hydrogen during the production process leads to the formation of pores, which not only reduce the material’s strength and durability but may also cause premature failure of the material. The formation of pores typically occurs during the solidification stage of magnesium alloys, where hydrogen dissolved in the molten metal is released upon cooling, forming tiny gas pores. The presence of these gas pores significantly affects the mechanical properties of the material, potentially leading to crack initiation and propagation under high stress. Therefore, accurate detection and quantification of pores are crucial for enhancing the quality control of magnesium alloys. Our developed model integrates window-shaped perception blocks with convolutional neural networks, enhanced by aggregated sensing layers (ASLs) on long-range connections. Extensive training on real samples demonstrated that our model outperforms mainstream algorithms such as U-Net and TransUNet across various evaluation metrics, particularly in fine target detection tasks under complex scenarios. Specifically, our model achieved a Dice coefficient of 74.77% and an Intersection over Union index of 71.00%, significantly surpassing other models. Moreover, the method also demonstrated superior accuracy in pore edge prediction, effectively mitigating issues of oversegmentation and undersegmentation, especially for small and irregular pores. An ablation study further confirmed the effectiveness of each component, with the ASL module showing particular strength in feature extraction and reducing upsampling loss. In summary, this research highlights the significant potential of deep learning technology in material defect detection and provides an efficient, automated solution for practical production, contributing to advancements in materials science and industrial quality control.
Keywords
INTRODUCTION
Magnesium alloys, known for their lightweight and high-strength properties[1], are widely used in aerospace, automotive, and biomedical industries[2-6]. However, hydrogen absorption during production often leads to the formation of pores[7], which severely affect the mechanical properties of the material. These pores not only reduce the material’s strength and durability but also may cause premature failure during service. The formation of pores typically occurs during the solidification of magnesium alloys, where hydrogen dissolved in the molten metal is released upon cooling, forming tiny gas pockets. The presence of these pores significantly influences the mechanical properties of the material, potentially leading to crack initiation and propagation under high stress. Therefore, accurate detection and quantification of pores are crucial for enhancing the quality control of magnesium alloys. Traditional detection methods, such as X-ray[8] and ultrasonic testing, are inefficient and rely heavily on operator experience, leading to unstable results. Therefore, there is an urgent need to develop automated and efficient detection methods to improve product quality. This study combines machine learning[9] and deep learning[10,11] to develop an improved model. The model incorporates a window-shaped (WS) perception blocks and a convolutional neural network (CNN) module, and adds an aggregated sensing layer (ASL) on the long skip connections at each level. This design retains global information while enhancing the capture of local features, thereby improving segmentation accuracy. Experimental results show that the model outperforms other mainstream segmentation methods, such as U-Net and TransUNet, in multiple evaluation metrics, including Dice coefficient and Intersection over Union (IoU), particularly in the accuracy of pore edge prediction. The method makes significant contributions to the field of material science by providing a more precise and efficient means of defect detection[12-15].
In recent years, thanks to the rapid development of computer vision and deep learning technologies, automated image segmentation methods have been successfully applied in many industrial inspection tasks. The core of image segmentation technology is to precisely separate different regions within an image for better analysis and processing. Traditional image segmentation methods, such as edge detection and region growing, perform well in certain scenarios but are less robust to complex textures and noise. To solve these problems, fully convolutional networks (FCN) and other deep learning techniques have emerged, significantly improving the accuracy and efficiency of image segmentation. As the field of computer vision has rapidly developed, image segmentation technology has become an important component of artificial intelligence research. From early edge-based traditional methods to advanced algorithms based on deep learning in recent years, image segmentation technology has made significant progress. FCN, first proposed by Jonathan Long, Evgeniy Shelhamer, and Trevor Darrell in 2015, revolutionarily discarded the fully connected (FC) layers in traditional CNNs and adopted a fully convolutional approach to process input data[16,17], enabling the handling of images of arbitrary size. Since its introduction, FCN has spawned many variants, such as U-Net, DeepLab, and Mask Region-CNN (R-CNN)[18-20], all of which have achieved excellent results in various applications. These models continuously drive the development of image segmentation technology and are widely used in medical image analysis, autonomous driving, robotics, and other fields[21-23]. In recent years, image segmentation algorithms based on Transformer architectures have gained widespread attention and application in multiple domains due to their excellent scalability and deep semantic understanding capabilities. TransUNet, as an innovative framework that integrates the advantages of Transformer and the classic U-Net model[24-26], has shown significant advantages in tasks emphasizing semantic understanding[27,28]. Particularly in applications requiring a broad receptive field, TransUNet achieves dynamic adjustment of the receptive field by integrating Transformer components at the lower levels of the model, greatly enhancing the model’s ability to capture global information. However, when dealing with specific domain tasks such as the microscopic images of magnesium alloys, the potential limitations of TransUNet gradually become apparent. Such tasks often require the model to possess highly accurate local information extraction capabilities to accurately identify and analyze the subtle differences in the material’s microstructure. Although the ConvBlock in TransUNet[29] can effectively extract basic features of the image and perform initial downsampling, its expressiveness is insufficient for complex and dense local details.
In recent years, several studies have explored the application of machine learning techniques for pore detection in magnesium alloys. For instance, Anand et al. used PoreNet model[30] to identify pore defects. Compared to PoreNet model, our deep learning approach handles image data more directly and efficiently, automatically learning features from images, thus improving accuracy and efficiency. Bosse et al. proposed a CNN-based method for pore detection[31]. While their method performs well on specific datasets, our approach enhances detection capabilities for complex backgrounds and irregular pores by incorporating WS Perception Blocks and ASLs. Brown and Green combined deep learning with traditional image processing techniques to improve detection accuracy. However, our fully deep learning-based method better adapts to different types and shapes of pores while maintaining real-time processing capabilities. Through these comparisons, we highlight the unique advantages of our work: superior feature extraction and multi-scale information integration, particularly in handling complex backgrounds and irregular pore shapes with higher accuracy and robustness.
To address the aforementioned challenges, this study proposes a series of targeted optimization strategies. Considering the unique needs of the magnesium alloy microscopic image analysis task, this study innovatively adjusts the Transformer architecture to a cross-perception mode. This design allows the model to focus on capturing and analyzing subtle changes within local regions while maintaining the ability to understand global information, thus achieving a deeper and finer understanding of the magnesium alloy microstructure. Additionally, to enhance the model’s ability to capture local information, a local information supplement module (ASL) is introduced into the skip connections (Skip Connection) of the first three stages (Stage). This module draws on the characteristics of Low-Level tasks and is built based on a residual learning framework, aiming to optimize feature representation through a multi-kernel adaptive selection attention mechanism. Specifically, different types of convolutional components perform multi-scale feature extraction, and feedforward neural networks calculate attention scores for each feature map. Finally, based on these scores as weights, different sources of feature maps are weighted and fused, prompting the network to focus more on key local details. This design not only enriches the model’s representation capability but also improves its robustness and accuracy in handling complex images. In summary, by optimizing the TransUNet framework, this study aims to develop a new generation of image segmentation algorithms that can converge quickly and accurately capture local features, better serving advanced analysis tasks in fields such as materials science.
MATERIALS AND METHODS
Experimental process
Metallographic analysis of AZ91 magnesium alloy
Figure 1 illustrates the process of metallographic analysis of materials. The left image shows a porous structure of AZ91 magnesium alloy, with specific regions indicated by arrows selected for detailed metallographic analysis. The middle image displays the observed microstructure of the selected region through a microscope, including different phases and grains. The right image is recorded by the camera of the microscope, using polarized light to reveal the internal microstructural features of the material through different colors and contrasts.
Dataset introduction and preprocessing
The dataset used in this experiment was provided by the Additive Manufacturing Research Institute of the University of Shanghai for Science and Technology, consisting of 23 optical microscope images of AZ91 magnesium alloy. After selection and processing, 15 images were used, divided into a training set and validation set at a ratio of 10:5. To improve the generalization and robustness of the model, comprehensive data preprocessing was performed on the original images. First, all input images were standardized to ensure pixel values were within the same range, enhancing training efficiency and stability. Specifically, the pixel values were normalized to the range [0, 1]. Additionally, to increase the diversity of training samples and prevent overfitting, various data augmentation techniques were employed, including random rotation, random flipping, random cropping, and brightness adjustment. These data augmentation methods not only improve the model’s generalization ability but also effectively enhance the accuracy of the image segmentation task.
Figure 2 shows the process of preprocessed AZ91 magnesium alloy microscopic images, which underwent machine segmentation followed by manual segmentation. Preprocessing steps included standardizing the images (ensuring pixel values were within the same range to improve training efficiency and stability) and data augmentation (such as random rotation, random flipping, random cropping, and brightness adjustment to increase sample diversity and prevent overfitting). Preprocessing ensures the quality and consistency of images input into the model, aiding in efficient and stable model training and enhancing the model’s generalization ability. As seen in the figure, machine segmentation can accurately identify most porosity defects, but some details remain unrecognizable. Manual segmentation supplements and refines the machine segmentation results, especially for small or blurred-edge porosity defects, further improving segmentation accuracy.
Figure 2. The image was preprocessed and then subjected to machine segmentation followed by manual segmentation.
Figure 3 shows the refinement of machine segmentation results through manual segmentation, particularly addressing details overlooked by machine segmentation. For small or poorly defined porosity defects, machine segmentation may not fully accurately identify them, necessitating manual correction to ensure the accuracy of the final segmentation results. Human intervention compensates for the shortcomings of machine learning models, especially in handling complex or irregular defects, providing higher precision segmentation results.
Figure 3. Manual refinement was performed to fill in the details overlooked by the machine segmentation.
Figures 2 and 3 collectively illustrate the comprehensive workflow of image preprocessing, machine segmentation, and manual segmentation as outlined in this study. The inclusion of manual segmentation serves as a critical refinement step, wherein human intervention is employed to enhance the segmentation outcomes, particularly for minute or poorly defined porosity defects. This approach is especially pertinent when dealing with the intricate micrographs of magnesium alloys, offering an efficient and robust solution for image segmentation tasks within the realm of materials science[32]. By integrating both machine and manual segmentation methodologies, not only is the accuracy of segmentation markedly improved, but it also establishes a solid foundation for subsequent material analysis and quality control processes.
Experimental environment and configuration
To ensure the reliability and reproducibility of the experiments, this study used high-performance hardware and advanced software tools for image segmentation tasks, as shown in Table 1. The specific experimental environment and configuration are as follows: The experimental platform was equipped with four NVIDIA GeForce RTX3090 GPUs, an Intel(R) Xeon(R) Platinum 8280L CPU @ 2.60GHz, and the Linux operating system. The training framework used Pytorch for deep learning, facilitating debugging and model modification, and Tensorboard for monitoring and visualizing the training process, providing an intuitive display of various metrics during training, helping us better understand and optimize the model. In this study, to ensure effective training and optimization of the model, we set a total of 10,000 training epochs, with a batch size of 4, an initial learning rate of 0.00001, polynomial decay, a minimum learning rate of 0.000001, and momentum parameters (0.9, 0.999). To further enhance the model’s learning efficiency and generalization ability, we used the Adam optimization algorithm, with a weight decay coefficient (L2 regularization term) set to 0.005.
Training parameter settings
Parameter name | Epochs | Batch size | Initial learning rate | Learning rate decay method | Minimum learning rate | Momentum parameters | Optimization algorithm | Weight decay coefficient |
Setting value | 10,000 | 4 | 0.00001 | Polynomial decay | 0.000001 | (0.9, 0.999) | Adam | 0.005 |
Figure 4 depicts the trend of the training loss function over 10,000 training epochs. The x-axis represents the training epoch, and the y-axis represents the corresponding training loss value. It can be clearly seen that as the number of training epochs increases, the training loss continues to decrease and eventually reaches a relatively stable level. This indicates that the proposed model not only effectively learns the intrinsic patterns of the data on the training set but also exhibits good convergence, proving the effectiveness of the model design and training strategy[33].
Evaluation metrics
In the field of machine learning and computer vision, especially in image segmentation, object detection, and classification tasks, a series of evaluation metrics[34] are frequently used to measure model performance. In this study, we primarily used IoU, Accuracy (ACC), F1-Score (F-Score), Precision, Recall, and Dice Similarity Coefficient (Dice). IoU is a metric used to evaluate the overlap between predicted and ground truth bounding boxes. It is calculated by dividing the area of intersection by the area of union. IoU ranges from 0 to 1, with higher values indicating more accurate predictions. Accuracy refers to the proportion of correctly predicted samples among all samples. F1-Score is the harmonic mean of Precision and Recall, balancing the relationship between the two. F1-Score ranges from 0 to 1, with higher values being better. Precision is the proportion of actual positive samples among those predicted as positive. High precision indicates high confidence in the positive predictions made by the model. Recall is the proportion of actual positive samples correctly predicted as positive. High recall indicates that the model can capture more actual positive samples. Dice Coefficient is similar to IoU, measuring the similarity between two sets. It is defined as twice the number of intersecting elements divided by the sum of the elements in both sets. These metrics each have their focus and may have different importance in different application scenarios. Typically, we combine multiple metrics to comprehensively evaluate a model’s performance, which are defined by
Where true positive (TP) represents the number of samples actually positive and predicted as positive, true negative (TN) indicates the number of samples actually negative and predicted as negative, false positive (FP) denotes the number of samples actually negative but incorrectly predicted as positive, and false negative (FN) stands for the number of samples actually positive but incorrectly predicted as negative.
Network overview
This study proposes an innovative deep learning framework aimed at improving the efficiency and accuracy of medical image segmentation. As shown in Figure 5, the framework integrates CNNs with improved WS Perception Blocks and introduces ASL modules on long skip connections. The specific process is as follows: input images are first processed by CNNs to generate basic hidden features. These features are then linearly projected into the WS Perception Block module, which includes multi-layer perceptron (MLP), layer normalization (LN), and a unique cross-shaped window self-attention mechanism, capable of capturing complex dependencies between features. The processed features are downsampled at three different resolution levels (1/2, 1/4, 1/8) and combined with corresponding convolution operations through long skip connections. At each level of the long skip connections, ASL modules are added, using spatial and channel-level attention mechanisms to further enhance feature expression, selectively integrating key features while ignoring other unimportant features, thereby reducing theoretical complexity and improving performance. During the decoding process, features are upsampled step-by-step and fused with multi-scale features, ultimately generating segmentation results by the segmentation head.
WS perception block as encoder
The WS perception block constructs an efficient and powerful image processing framework, specifically designed to capture multi-level, multi-scale features in images. The architecture consists of four main stages, each progressively performing deep feature extraction on the input image, gradually reducing spatial resolution while increasing the number of feature channels, thereby achieving a coarse-to-fine feature representation process. Specifically, Stage 1 receives raw RGB images as input, and after processing through one or more WS perception blocks, outputs feature maps with dimensions reduced by four times and channel numbers of C. Subsequent stages (Stages 2 to 4) continue to process the output from the previous stage, halving the spatial resolution and doubling the feature dimensions each time, until the final high-dimensional feature representation is obtained. As shown in Figure 6, the WS Perception Block, as the core component of this architecture, cleverly combines Lateral Self-Attention, LN, and MLP. Among them, the Lateral Self-Attention module significantly enhances the model’s ability to capture long-distance dependencies while maintaining lower computational costs through a unique window division strategy. LN is applied at the input and output endpoints of each Block, helping to stabilize the training process and accelerate convergence. The MLP further introduces non-linear mapping, enhancing the model’s expressive power. The WS Perception Block performs cross-window self-attention by executing self-attention in horizontal and vertical stripes. The specific formulas are as follows:
(a) Horizontal and vertical stripe self-attention formulas: The WS perception block performs cross-window self-attention by executing self-attention in horizontal and vertical stripes. For horizontal stripe self-attention, the input feature X ∈ RH × W × C is divided into stripes of equal width and undergoes self-attention calculation after linear projection to K heads. The output of horizontal stripe self-attention is:
where Yki = Attention (XiWkQ, XiWkK, XiWkV), Xi ∈ R(sw*W)*C, and sw is the stripe width. The formula for vertical stripe self-attention is similar, with the output denoted as V-AttentionK(X).
(b) WS perception block self-attention formula: The WS perception block divides the multi-heads into two groups, one for horizontal stripe self-attention and the other for vertical stripe self-attention, and concatenates the outputs of the two groups:
Where WO ∈ RC*C is a common projection matrix.
Where
(c) Computational complexity analysis: The computational complexity of WS self-attention is:
where sw is the stripe width, which can be adjusted according to the network depth.
(d) Definition of WS Perception Block: Finally, the WS Perception Block is defined as:
ASL module
As illustrated in Figures 7-9, the ASL module represents an advanced iteration built upon the solid foundation of squeeze and excitation network (SENet)[35,36]. It is designed to further enhance the feature representation capabilities of CNNs by integrating channel-wise features with global information, thereby optimizing the network’s learning process for image features. Consequently, we have integrated this module into skip connections to improve the model’s segmentation performance.
Figure 8. The structure of a neural network where each neuron is connected to all input units, achieving a linear combination of the input data. The outputs of multiple neurons are then concatenated through a Concatenation Layer to form a more complex feature representation.
Figure 9. The excitation layer processes the input features using a Sigmoid activation function, and channel-wise multiplication combines the Sigmoid-activated features with the input feature maps through an element-wise product, ultimately generating the output feature maps.
The ASL module not only inherits the core concept of SENet - re-calibrating channel features to boost model performance - but also introduces a novel multi-branch FC layer structure for further optimization of feature representation. Within the ASL module, input features first pass through a Global Average Pooling layer to extract channel-level statistics. These channel-level features are then fed into a multi-branch FC layer structure, which consists of several branches of FC layers of identical size, each capable of independently learning global feature representations.
Following the multi-branch FC layers, an excitation operation generates channel-level weights. These weights are used to re-calibrate the original input features, emphasizing those that are more relevant to the classification task while suppressing less important ones. Thanks to the richer global information provided by the multi-branch FC layers, the ASL module can produce more accurate and effective channel weights. The features processed by the ASL module are subsequently fed into subsequent convolutional layers for further learning and classification.
Due to its ability to adaptively adjust channel weights and incorporate global information to optimize feature representation, the ASL module significantly enhances model performance without substantially increasing the number of parameters. Experimental results demonstrate that models incorporating the ASL module achieve notable improvements in accuracy and generalization capability on multiple image classification datasets compared to baseline models such as ResNet.
Decoder
The decoder part mainly achieves this through cascaded upsampling units (CUP). CUP consists of multiple upsampling steps used to decode hidden features and output the final segmentation mask. After reshaping the sequence of hidden features, multiple upsampling blocks are used to reach full resolution from × to H × W. Each upsampling block consists of a 2× upsampling operator, a 3 × 3 convolution layer, and a ReLU layer in sequence[37].
Loss function
When handling the segmentation task of magnesium alloy microscopic images, using BCE loss[38] might cause the model to overly focus on background pixels and ignore small target regions. Therefore, combining Dice loss helps the model better learn the features of small target regions. Simultaneously, by adjusting the weight parameter αα in the composite loss function, the model can ensure correct segmentation of background regions while improving the detection accuracy of small target regions, as given in
RESULTS AND DISCUSSION
Comparison experiments
In this study, we conducted a comprehensive comparison of the proposed improved segmentation method against several state-of-the-art segmentation techniques specifically for the task of segmenting AZ91 magnesium alloy. The comparative methods included U-Net, Attention U-Net (AttU-Net), and TransUNet. The quantitative analysis results are summarized in Table 2, where the best performance in each metric is highlighted in bold. Our findings demonstrate that the proposed segmentation method outperforms the other models across all evaluation metrics, achieving a Dice coefficient of 74.77% and an IoU index of 71.00%, which are notably higher than those of the competing models.
Quantitative analysis results of different methods
Method | IoU | Acc | F1 | Recall | Dice |
TransUNet | 67.46% | 44.42% | 64.68% | 58.31% | 69.75% |
Unet | 63.42% | 35.69% | 56.95% | 51.77% | 63.12% |
AttU-Net | 62.34% | 33.73% | 54.66% | 50.30% | 61.16% |
This work | 71% | 53.60% | 70.54% | 65.20% | 74.77% |
As illustrated in Figure 10A and B, experimental results clearly demonstrate that the proposed model outperforms mainstream algorithms such as U-Net and TransUNet across multiple evaluation metrics. Specifically, the Dice coefficient reached 74.77%, and the IoU index was 71.00%, significantly surpassing other models. Moreover, the proposed model excelled in the accuracy of pore edge prediction, with the Dice coefficient and IoU improving by 5.02% and 3.54%, respectively.
Visualization of pore segmentation results from different methods
In this study, we selected the most representative four visualizations out of fifteen to illustrate our findings. As shown in Figure 11, the proposed method excels in the pore segmentation task of AZ91 magnesium alloy, delivering both stable and optimal segmentation outcomes, along with accurate predictions of pore boundaries. These results substantiate the efficacy and superiority of our approach, particularly in the automatic pore segmentation of AZ91 magnesium alloy micrographs, thereby affirming its technological leadership. Figure 12 presents the input images alongside their corresponding generated labels, establishing a foundation for subsequent research and learning.
Figure 11. Comparison of the segmentation results of different methods. The images (A) to (D) are the results obtained using the AttU-Net method. Images (E) to (H) show the segmentation outcomes from TransUNet. The UNet method’s results are displayed in images (I) to (L). Finally, images (M) to (P) present the segmentation results from this work.
Figure 12. Input images and their corresponding generated labels. Images (A) to (D) are the input images, while images (E) to (H) are the generated labels.
Figure 13 illustrates the segmentation outcomes from integrating different modules into the TransUNet architecture. Figure 13A-D show the results after incorporating the WS module into TransUNet, while Figure 13E-H depict the outcomes with the addition of the ASL module. Figure 13I-L display the combined effect of integrating both WS and ASL modules. Figure 14 provides a detailed comparison of segmentation results for detecting pore defects in AZ91 magnesium alloys using four methods: Att-UNet, TransUNet, UNet, and the proposed method. Each method exhibits distinct strengths and limitations in segmentation effectiveness.
Figure 13. Segmentation results with different module integrations into the TransUNet network structure. Images (A) to (D) display the results when the WS module is added to TransUNet. Images (E) to (H) show the outcomes with the ASL module integrated into TransUNet. Finally, images (I) to (L) present the results when both the WS and ASL modules are combined in TransUNet. WS: Window-shaped; ASL: aggregated sensing layer.
Ablation study
To systematically evaluate the impact of each component of the proposed model on the performance of pore segmentation in magnesium alloy micrographs, we conducted a detailed ablation study. The experimental results are shown in Table 3, with the best results in each column highlighted in bold. We used the standard TransUNet as the baseline model and gradually introduced different components.
Ablation study results
Method | IoU | Acc | F1 | Recall | Dice |
TransUNet | 67.46% | 44.42% | 64.68% | 58.31% | 69.75% |
WS | 68.87% | 47.38% | 67.12% | 60.54% | 71.83% |
ASL | 70.08% | 50.67% | 69.10% | 63% | 73.53% |
This work | 71% | 53.60% | 70.54% | 65.20% | 74.77% |
First, we replaced the Transformer layer in the CNN-Transformer hybrid model with a WS Perception Block, resulting in improvements of 2.08% in Dice and 1.41% in IoU. Next, we added the ASL module on top of this, further improving Dice from 73.53% to 74.77% and IoU from 70.08% to 71.0%.
Figure 10A illustrates the comparative outcomes of different segmentation approaches on the porosity defect detection task in AZ91 magnesium alloys. As depicted, our proposed method exhibits superior accuracy in both the segmentation of pore regions and the precision of edge detection compared to existing methods such as TransUNet, U-Net, and AttU-Net. Specifically, our approach more accurately delineates the boundaries of pores, effectively mitigating issues of oversegmentation and undersegmentation, especially when dealing with complex pore morphologies. Moreover, the proposed method excels in background segmentation, significantly reducing FPs. These results underscore the superior performance of our model in handling the porosity defect detection task in AZ91 magnesium alloys, offering an efficient and reliable solution for image segmentation in materials science.
Figure 10B highlights the comparative performance of different segmentation methods in terms of pore edge prediction accuracy. The data show that our proposed method achieves a marked advantage in capturing the contours of pore edges, minimizing edge blurring and fragmentation, particularly for small and irregular pores. In contrast, other methods such as TransUNet, U-Net, and AttU-Net exhibit varying degrees of error in edge prediction, leading to less refined segmentation outcomes. The enhanced performance of our method can be attributed to the introduction of the WS Perception Block and the ASL module, which significantly improve the capture of local features, thereby achieving higher accuracy and robustness in edge prediction.
To elaborate, while traditional U-Net performs well in many segmentation tasks, it has limitations in handling complex scenarios. AttU-Net builds upon U-Net by incorporating an attention mechanism, enabling the model to dynamically weigh the importance of different regions, thus focusing more on salient feature areas and improving segmentation accuracy. However, the addition of the attention mechanism increases computational complexity, potentially affecting the efficiency of real-time applications and yielding inconsistent results across different datasets. TransUNet integrates Transformer architecture into the conventional U-Net framework, combining the strong local feature extraction capabilities of CNNs with the long-range dependency modeling of Transformers, thereby enhancing the model’s understanding of global information. The WS Perception Block used in our method introduces a hierarchical spatial window mechanism, dividing the input image into multiple local windows, within which tokens undergo self-attention calculations, significantly reducing computational overhead. Additionally, through a cross-window connection mechanism, the WS Perception Block progressively expands the receptive field across layers, capturing broader contextual information while maintaining local computational efficiency. Furthermore, the ASL module employs multi-branch FC layers to enhance classification accuracy with minimal increase in model parameters, thereby contributing to the overall effectiveness of the proposed segmentation approach.
The experimental evidence indicates that the standalone inclusion of the WS module notably enhances segmentation performance, especially in capturing fine edges of pore defects. Further incorporation of the ASL module leads to an improvement in segmentation accuracy, demonstrating greater robustness when handling complex backgrounds and noise. Att-UNet achieves higher segmentation precision in the green-marked areas by accurately capturing key features in the image, yet it suffers from some missegmentation issues in the red-marked regions, particularly in delineating clear segmentation boundaries in complex backgrounds. TransUNet leverages the global context processing capability of Transformers to achieve good segmentation on large-scale images; however, it performs less effectively on small targets in the red-marked regions and has a larger number of model parameters, which increases training difficulty. The UNet structure is simple and easy to implement, making it suitable for various image segmentation tasks, but its segmentation accuracy may decline when dealing with complex backgrounds or detail-rich images in the red-marked areas. In contrast, the proposed method maintains computational efficiency while enhancing segmentation accuracy, especially in handling images with complex backgrounds. The WS block, through its unique cross-shaped window mechanism, effectively captures local features, leading to clearer and more precise pore defect boundaries, which is critical for applications requiring high-resolution segmentation. The ASL module further refines feature representation by selectively integrating crucial features and suppressing irrelevant ones, thereby increasing robustness and accuracy in high-noise or complex texture regions. Finally, Figure 14 showcases the segmentation results of our method combining the WS block and ASL module. This combination significantly boosts overall segmentation accuracy, enabling the high-precision capture of fine edges of pore defects while preserving high detail in background regions. Even in challenging scenarios, such as overlapping structures, the boundaries of pore defects are clearly distinguishable.
The ablation study results indicate that each component significantly enhances segmentation performance, with the ASL module particularly excelling in feature extraction and reducing upsampling loss. Therefore, the complete proposed model achieves optimal segmentation performance, providing strong support for pore segmentation tasks in magnesium alloy micrographs.
The ablation study results indicate that each component significantly enhances segmentation performance, with the ASL module particularly excelling in feature extraction and reducing upsampling loss. Therefore, the complete proposed model achieves optimal segmentation performance, providing strong support for pore segmentation tasks in magnesium alloy micrographs.
The proposed method has significant potential for industrial applications, particularly in the aerospace, automotive, and biomedical industries, where the quality control of magnesium alloys is critical. Traditional inspection methods are often inefficient, costly, and limited in detecting small or low-contrast defects. Our automated and efficient segmentation approach provides a cost-effective solution for improving product quality. The model’s ability to accurately detect and segment porosity defects can help manufacturers identify potential issues early in the production process, reducing the risk of material failure and enhancing the overall reliability of the final product. Moreover, the model’s adaptability to different types of materials and microstructures opens up new possibilities for its application in various fields of materials science and industrial quality control. Integrating our model into existing production lines can streamline quality assurance processes, leading to faster and more reliable inspections.
While our proposed model demonstrates significant advantages over existing methods, there are still areas for improvement. One limitation is the current reliance on manual refinement for certain complex or irregular defects, which can be time-consuming and labor-intensive. Future work will focus on developing fully automated solutions that can handle these cases without human intervention. Additionally, we plan to explore the integration of additional sensing modalities, such as X-ray and ultrasonic data[39-42], to further enhance the model’s performance. Another area of interest is the optimization of the model structure to improve its efficiency and scalability, particularly for large-scale industrial applications. Finally, we aim to extend the application of our method to other types of materials and defect detection tasks, contributing to advancements in materials science and industrial quality control.
CONCLUSIONS
In this study, we successfully developed a novel deep learning-based image segmentation method aimed at addressing the challenge of detecting bubble defects in AZ91 magnesium alloys. By integrating a WS Perception Block and a CNN module, and innovatively adding an ASL module at each level of long skip connections, our model not only retains global information but also significantly enhances the capture of local features, thereby greatly improving segmentation accuracy. Experimental results clearly show that compared to mainstream segmentation methods such as U-Net and TransUNet, our model exhibits significant advantages in key evaluation metrics such as Dice coefficient and IoU, particularly in the accuracy of pore edge prediction. Additionally, we optimized strategies by adjusting the Transformer architecture to a cross-shaped perception mode and introducing a local information supplementation module, optimizing feature representation through multi-kernel adaptive selection attention mechanisms. By improving the loss function, combining Dice loss and BCE loss, and adjusting the weight parameters of the combined loss function, we significantly improved the detection accuracy of small target areas while ensuring correct segmentation of background regions. To enhance the model’s generalization and robustness, we performed extensive data preprocessing, including normalization and various data augmentation techniques such as random rotation, flipping, cropping, and brightness adjustment. When evaluating model performance, we used multiple evaluation metrics to comprehensively and accurately measure the model’s performance. Finally, through comparative experiments and ablation studies, we further confirmed the comprehensive superiority of our model over other advanced segmentation methods and the significant impact of each component on segmentation performance. This research not only provides an efficient and automated solution for detecting bubble defects in AZ91 magnesium alloys but also offers valuable references for similar image segmentation tasks in materials science, demonstrating the effectiveness and broad application potential of our model in practical applications.
DECLARATIONS
Authors’ contributions
Conceptualization, data curation, formal analysis, investigation, methodology, software, validation, writing - original draft, writing - review and editing: An, M.
Theoretical guidance, data analysis, manuscript review and scientific content advice: Zheng, Z.
Investigation, writing - review and editing: Yue, X.
Formal analysis, supervision, writing - original draft, writing - review and editing: Wang, J.
Data curation, resources, supervision: Xing, C.
Availability of data and materials
The data that support the findings of this study are available from the corresponding author upon reasonable request.
Financial support and sponsorship
The authors are grateful for the financial support provided by the University of Shanghai for Science and Technology Teaching Achievement Award Cultivation Project (JXCG-202414). Additionally, we would like to acknowledge the support and assistance provided by the Analysis and Testing Center of the University of Shanghai for Science and Technology.
Conflicts of interest
All authors declared that there are no conflicts of interest.
Ethical approval and consent to participate
Not applicable.
Consent for publication
Not applicable.
Copyright
© The Author(s) 2025.
REFERENCES
1. Zhang, Y.; Bai, S.; Jiang, B.; Li, K.; Dong, Z.; Pan, F. Modeling the correlation between texture characteristics and tensile properties of AZ31 magnesium alloy based on the artificial neural networks. J. Mater. Res. Technol. 2023, 24, 5286-97.
2. Bai, J.; Yang, Y.; Wen, C.; et al. Applications of magnesium alloys for aerospace: a review. J. Magnes. Alloys. 2023, 11, 3609-19.
3. Tian, P.; Liu, X. Surface modification of biodegradable magnesium and its alloys for biomedical applications. Regen. Biomater. 2015, 2, 135-51.
4. Yue, X.; Shang, J.; Zhang, M.; Hur, B.; Ma, X. Additive manufacturing of high porosity magnesium scaffolds with lattice structure and random structure. Mater. Sci. Eng. A. 2022, 859, 144167.
5. Abazari, S.; Shamsipur, A.; Bakhsheshi-Rad, H. R.; et al. Magnesium-based nanocomposites: a review from mechanical, creep and fatigue properties. J. Magnes. Alloys. 2023, 11, 2655-87.
6. Wang, S.; Pan, H.; Xie, D.; et al. Grain refinement and strength enhancement in Mg wrought alloys: a review. J. Magnes. Alloys. 2023, 11, 4128-45.
7. V, K.; Kumar, B. N.; Kumar, S. S.; M, V. Magnesium role in additive manufacturing of biomedical implants - challenges and opportunities. Addit. Manuf. 2022, 55, 102802.
8. Katunin, A.; Wronkowicz-Katunin, A.; Dragan, K. Impact damage evaluation in composite structures based on fusion of results of ultrasonic testing and X-ray computed tomography. Sensors 2020, 20, 1867.
9. Fu, Y.; Downey, A. R.; Yuan, L.; Zhang, T.; Pratt, A.; Balogun, Y. Machine learning algorithms for defect detection in metal laser-based additive manufacturing: a review. J. Manuf. Process. 2022, 75, 693-710.
10. Minaee, S.; Boykov, Y.; Porikli, F.; Plaza, A.; Kehtarnavaz, N.; Terzopoulos, D. Image segmentation using deep learning: a survey. IEEE. Trans. Pattern. Anal. Mach. Intell. 2022, 44, 3523-42.
11. Li, K.; Ma, R.; Qin, Y.; et al. A review of the multi-dimensional application of machine learning to improve the integrated intelligence of laser powder bed fusion. J. Mater. Proc. Technol. 2023, 318, 118032.
12. Gao, G.; Xu, G.; Yu, Y.; Xie, J.; Yang, J.; Yue, D. MSCFNet: a lightweight network with multi-scale context fusion for real-time semantic segmentation. IEEE. Trans. Intell. Transport. Syst. 2022, 23, 25489-99.
13. Hong, D.; Yao, J.; Meng, D.; Xu, Z.; Chanussot, J. Multimodal GANs: toward crossmodal hyperspectral–multispectral image segmentation. IEEE. Trans. Geosci. Remote. Sensing. 2021, 59, 5103-13.
14. Wang, L.; Li, R.; Zhang, C.; et al. UNetFormer: a UNet-like transformer for efficient semantic segmentation of remote sensing urban scene imagery. ISPRS. J. Photogramm. Remote. Sens. 2022, 190, 196-214.
15. Lu, W.; Zhang, Z.; Nguyen, M. A lightweight CNN–transformer network with laplacian loss for low-altitude UAV imagery semantic segmentation. IEEE. Trans. Geosci. Remote. Sensing. 2024, 62, 1-20.
16. Wu, J.; Liu, B.; Zhang, H.; He, S.; Yang, Q. Fault detection based on fully convolutional networks (FCN). JMSE. 2021, 9, 259.
17. Papadeas, I.; Tsochatzidis, L.; Amanatiadis, A.; Pratikakis, I. Real-time semantic image segmentation with deep learning for autonomous driving: a survey. Appl. Sci. 2021, 11, 8802.
18. Chu, P.; Li, Z.; Lammers, K.; Lu, R.; Liu, X. Deep learning-based apple detection using a suppression mask R-CNN. Pattern. Recognit. Lett. 2021, 147, 206-11.
19. Yang, J.; Tu, J.; Zhang, X.; Yu, S.; Zheng, X. TSE DeepLab: an efficient visual transformer for medical image segmentation. Biomed. Signal. Process. Control. 2023, 80, 104376.
20. Lin, K.; Zhao, H.; Lv, J.; et al. Face detection and segmentation based on improved mask R-CNN. Discrete. Dyn. Nat. Soc. 2020, 2020, 1-11.
21. Qiong, L.; Chaofan, L.; Jinnan, T.; Liping, C.; Jianxiang, S. Medical image segmentation based on frequency domain decomposition SVD linear attention. Sci. Rep. 2025, 15, 2833.
22. Banjanovic-Mehmedovic, L.; Husaković, A.; Gurdic, R. A.; Prlja, N.; Karabegovi, I. Advancements in robotic intelligence: the role of computer vision, DRL, transformers and LLMs. 2024.
23. Kolides, A.; Nawaz, A.; Rathor, A.; et al. Artificial intelligence foundation and pre-trained models: fundamentals, applications, opportunities, and social impacts. Simul. Model. Pract. Theory. 2023, 126, 102754.
24. Han, D.; Pan, X.; Han, Y.; Song, S.; Huang, G. Flatten transformer: vision transformer using focused linear attention. In: 2023 IEEE/CVF International Conference on Computer Vision (ICCV), Paris, France. Oct 01-06, 2023. IEEE, 2023; pp. 5961-71.
25. Chen, J.; Mei, J.; Li, X.; et al. 3D TransUNet: advancing medical image segmentation through vision transformers. arXiv2023, arXiv:2310.07781. Available online: https://doi.org/10.48550/arXiv.2310.07781. (accessed on 12 Mar 2025)
26. Ozcan, A.; Tosun, Ö.; Donmez, E.; Sanwal, M. Enhanced-TransUNet for ultrasound segmentation of thyroid nodules. Biomed. Signal. Process. Control. 2024, 95, 106472.
27. Jain, J.; Li, J.; Chiu, M.; Hassani, A.; Orlov, N.; Shi, H. OneFormer: one transformer to rule universal image segmentation. arXiv2022, arXiv:2211.06220. Available online: https://doi.org/10.48550/arXiv.2211.06220. (accessed on 12 Mar 2025)
28. Chen, J.; Mei, J.; Li, X.; et al. TransUNet: rethinking the U-Net architecture design for medical image segmentation through the lens of transformers. Med. Image. Anal. 2024, 97, 103280.
29. Chen, J.; Lu, Y.; Yu, Q.; er,. TransUnet: transformers make strong encoders for medical image segmentation. arXiv2021, arXiv:2102.04306. Available online: https://doi.org/10.48550/arXiv.2102.04306. (accessed on 12 Mar 2025)
30. Anand, V.; Kanhangad, V. PoreNet: CNN-based pore descriptor for high-resolution fingerprint recognition. IEEE. Sensors. J. 2020, 20, 9305-13.
31. Al-Zaidawi, S. M. K.; Bosse, S. A pore classification system for the detection of additive manufacturing defects combining machine learning and numerical image analysis. Eng. Proc. 2023, 58, 122.
32. Budd, S.; Robinson, E. C.; Kainz, B. A survey on active learning and human-in-the-loop deep learning for medical image analysis. Med. Image. Anal. 2021, 71, 102062.
33. Zheng, S.; Song, Y.; Leung, T.; Goodfellow, I. Improving the robustness of deep neural networks via stability training. In: 2016 IEEE Conference on Computer Vision and Pattern Recognition (CVPR), Las Vegas, USA. Jun 27-30, 2016. IEEE, 2016; pp. 4480-8.
34. Michaelis, C.; Mitzkus, B.; Geirhos, R. Benchmarking robustness in object detection: autonomous driving when winter is coming. arXiv2019, arXiv:1907.07484. Available online: https://doi.org/10.48550/arXiv.1907.07484. (accessed on 12 Mar 2025)
35. Farrukh, Y. A.; Wali, S.; Khan, I.; Bastian, N. D. SeNet-I: an approach for detecting network intrusions through serialized network traffic images. Eng. Appl. Artif. Intell. 2023, 126, 107169.
36. Huang, Y.; Shi, P.; He, H.; He, H.; Zhao, B. Senet: spatial information enhancement for semantic segmentation neural networks. Vis. Comput. 2024, 40, 3427-40.
37. Zhao, Y.; Jiang, Y.; Huang, L.; Xia, K. SEF-UNet: advancing abdominal multi-organ segmentation with SEFormer and depthwise cascaded upsampling. PeerJ. Comput. Sci. 2024, 10, e2238.
38. Cai, Z.; Liu, S.; Wang, G.; Ge, Z.; Zhang, X.; Huang, D. Align-DETR: enhancing end-to-end object detection with aligned loss. arXiv2023, arXiv:2304.07527. Available online: https://doi.org/10.48550/arXiv.2304.07527. (accessed on 12 Mar 2025)
39. Nong, X.; Luo, X.; Lin, S.; Ruan, Y.; Ye, X. Multimodal deep neural network-based sensor data anomaly diagnosis method for structural health monitoring. Buildings 2023, 13, 1976.
40. Alammar, Z.; Alzubaidi, L.; Zhang, J.; Li, Y.; Lafta, W.; Gu, Y. Deep transfer learning with enhanced feature fusion for detection of abnormalities in X-ray images. Cancers 2023, 15, 4007.
41. Tang, Q.; Liang, J.; Zhu, F. A comparative review on multi-modal sensors fusion based on deep learning. Signal. Process. 2023, 213, 109165.
Cite This Article

How to Cite
Download Citation
Export Citation File:
Type of Import
Tips on Downloading Citation
Citation Manager File Format
Type of Import
Direct Import: When the Direct Import option is selected (the default state), a dialogue box will give you the option to Save or Open the downloaded citation data. Choosing Open will either launch your citation manager or give you a choice of applications with which to use the metadata. The Save option saves the file locally for later use.
Indirect Import: When the Indirect Import option is selected, the metadata is displayed and may be copied and pasted as needed.
About This Article
Copyright
Data & Comments
Data
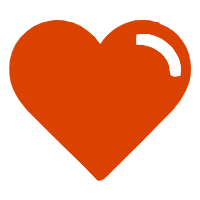
Comments
Comments must be written in English. Spam, offensive content, impersonation, and private information will not be permitted. If any comment is reported and identified as inappropriate content by OAE staff, the comment will be removed without notice. If you have any queries or need any help, please contact us at [email protected].