Local environment interaction-based machine learning framework for predicting molecular adsorption energy
Abstract
Machine learning (ML) models in materials science are mainly developed for predicting global properties, such as formation energy, band gap, and elastic modulus. Thus, these models usually fall short in describing local characteristics, such as molecular adsorption on surfaces. Here, we introduce a local environment interaction-based ML framework that contains a modified graph-based Voronoi tessellation geometrical representation, improved fingerprint feature engineering, and traditional ML and advanced deep learning (DL) algorithms. The precise characterization can be extracted using this framework for representing local information of adsorption of molecules on a surface. Using both traditional ML and advanced DL algorithms, we demonstrate remarkable prediction accuracy and robustness on 0D, two-dimensional (2D), and three-dimensional (3D) catalysts. Furthermore, it is found that the employment of this approach reduces data requirements and augments computational speed, specifically for DL algorithms. This work provides an effective and universal ML framework for various applications of molecular adsorption from catalysis, sensors, carbon capture, and energy storage to drug delivery, signifying a novel and promising avenue in the field of materials informatics. The implementation code in this work is available at https://github.com/mpeshel/LEI-framework_LERN.
Keywords
INTRODUCTION
Machine learning (ML) has found widespread applications in the field of materials science and engineering[1,2]. Researchers such as Behler and Parrinello have utilized atomic neural networks to learn total energy, which has been instrumental in developing interatomic potentials[3]. Moreover, ML techniques, such as SchNet[4], Crystal Graph Convolutional Neural Network (CGCNN)[5], and Atomistic Line Graph Neural Network (ALIGNN)[6], have been employed to establish relationships between atomic structures and their properties. These methods have been used to predict up to 50 different characteristics of crystals and molecular materials, including formation energy and electronic band gaps. Additionally, deep learning (DL) techniques have been leveraged in various applications to identify chemically feasible spaces. For instance, Bayesian optimization methods, in conjunction with MEGNet, have been employed as energy evaluators for direct structure relaxation[7]. To further enhance the performance, BOWSR incorporates band symmetry relaxation alongside Bayesian optimization[8]. Accurate characterization of localized physicochemical properties is of paramount importance in numerous scientific and engineering disciplines. Ranging from electrochemical catalysis, sensors, carbon capture, and energy storage and conversion to drug delivery, exploring and exploiting the intricacies of local environments are at the heart of many frontier investigations. For instance, adsorption is a pervasive surface phenomenon in areas such as electrocatalysis, with its understanding rooted in foundational theories such as bonding and adsorption thermodynamics[9]. Crucially, the influence of neighboring atoms on adsorption sites must be fully accounted for, as factors including atomic electronic structures, spatial constraints, surface stoichiometry, and surface defects can all impinge on the behavior of adsorbates on surfaces[10].
Recently, DL models have found applications in the catalysis realm, as demonstrated by various end-to-end graph neural network models developed in the Open Catalyst Project (OCP) challenge, encompassing equivariant Euclidean Neural Networks (e3nn), Spherical Channel Network (SCN), and Equivariant Spherical Channel Network (eSCN), among others[11–17]. While these models showcase stellar performance on the data-rich OC20 database[18], their computational complexity often leads to overfitting on smaller datasets [19–22]. Specifically, unlike single metal materials, multi-metal alloy catalysts exhibit excellent physical and chemical properties in the field of nanoparticles[23]. However, for this type of complex adsorption systems such as large organic molecules and certain transition metal oxides, accurate Density Functional Theory (DFT) computations are challenging, resulting in a paucity of reliable data[24,25]. Currently, graph neural networks based on global information are designed to capture the topology of the data, making them well-suited for processing small- to medium-scale molecular and crystalline materials, where local connectivity does not increase significantly with system size. However, for large systems, the number of edges and nodes in the graph network increases dramatically, resulting in significant performance degradation. Furthermore, it is difficult for the graph network to distinguish which information is critical. Therefore, extracting local information is critical to the adsorption energy. The structural diversity of large nanoparticles or two-dimensional (2D) materials is higher, and it is difficult for graph networks to handle the complexity caused by structural differences. In addition, implementing effective boundary condition processing in graph networks is also a challenge. The Adsorbate Chemical Environment-based Graph Convolution Neural Network (ACE-GCN) endeavors to convert each adsorbent surface to the configuration is initially split into subgraphs to explicitly account for the local chemical and structural environment of the adsorbent[26]. This approach suffers from weak interpretability and captures interactions in complex molecular systems. The segmentation of subgraphs has limitations and uncertainties, making it difficult to generalize to more diverse systems such as 2D materials. Employing ML approaches boasts advantages such as heightened interpretability, reduced data dependency, flexibility in designing and selecting features tailored to specific problems, and capabilities in prediction interpretation and error analysis[27–32]. Earlier studies proposed numerous feature engineering descriptors to enhance the prediction of ML models for adsorption energy, encompassing atomic number, ionization energy, electronegativity, ionic radius, and inter-atomic interactions[33–35]. These descriptors reported are equally pertinent to the field of electrocatalysis[36]. Yet, adequately representing metal compound adsorption sites remains a major challenge. Tran and Ulissi introduced using geometric fingerprints to depict the local region around each atom, but their atomic state descriptions were restricted to rudimentary elemental properties[37]. In addition, the local average electronegativity and generalized coordination numbers[38] of neighboring atoms are also considered effective features for calculating adsorption energy[39]. Based on this, a Coordination-activity diagram is developed to compute the adsorption energy by calculating the nearest neighbor[40]. Zhou et al. predicted the effective barrier of metal oxides by constructing a bulk-phase topology-derived tetrahedral descriptors[41] for the quantitative description of active sites. Li et al. proposed a method to extract local environment information based on simple intercepts[42]. The intercept setting of this technique usually relies on intuition and is difficult to standardize. It is only improved during pooling, and it is challenging to completely capture the local information required for adsorption energy calculation. Feature engineering based on central environments has demonstrated efficacy in describing local environments[43], but it still faces issues with generality, proving not universally applicable to surfaces[44].
In this work, we embark on exploring a novel approach by introducing a local environment interaction-based ML framework (LEI-framework) that extracts both local geometric and chemical features which can be integrated into either traditional ML or advanced DL algorithms [Figure 1]. We apply this framework to various complex systems [0D nanoparticles, 2D materials, and three-dimensional (3D) materials] and compare it with other state-of-the-art ML models. It is found that our approach outperforms others in predicting hydrogen adsorption energy on surfaces. Moreover, upon various ML models and deep neural networks, our LEI-framework significantly reduces computer cost. This work paves the way for new possibilities in understanding and manipulating the complexity of molecular adsorption systems. Such precision translates into high applicability, such as catalysis and sensor technologies.
Figure 1. The general ML framework of the local environment interaction. It contains three modules: (1) a modified graph-based Voronoi tessellation geometric representation; (2) improved fingerprint feature engineering; and (3) traditional ML and advanced neural network algorithms. Given the generality of the descriptor extraction method, it can be input into either traditional ML algorithms as weighted features or neural networks as graphs. Thus, this framework provides a highly interpretable and lightweight manner, retaining the advantages of traditional machine learning algorithms. ML: Machine learing.
MATERIALS AND METHODS
The concept of local environment, for ML-based prediction of adsorption energy, is primarily rooted in the analysis and feature extraction of the surrounding environment of the adsorbate. We first locate the position of the adsorbate within the 3D structure and then sequentially extract the atoms neighboring the adsorbate at each layer. Through feature engineering of the surrounding atoms at each layer, we obtain descriptors that describe the local environment, which can be used to input ML or neural network algorithms. This approach allows us to capture the essential information about the immediate surroundings of the adsorbate and characterize its local environment effectively. In this model, we use mean absolute error as the loss function and Adam as the optimization algorithm to train the network.
Database
This work relies on two essential databases, including in both 2D and 3D materials, each serving a distinct purpose. The 2D material database contains a substantial collection of 2,472 DFT calculations for hydrogen adsorption energy on 2D materials[45]. These calculations are performed on surfaces obtained from our developed 2Dmatpedia database[46], which currently encompasses over 10,000 distinct 2D materials.
The 3D materials dataset comprises a remarkable 47,279 DFT-calculated adsorption energy values. These calculations were conducted using the Generalized Adsorption Simulator for Python[37,47]. The dataset includes 21,269 adsorption energies concerning hydrogen atoms, which are the central focus of this study. Additionally, 26,010 adsorption energies pertain to other atoms. This extensive dataset covers a wide range of 52 chemical elements and 1,952 bulk materials, thereby enhancing its relevance and applicability. Furthermore, it is enriched with 9,102 symmetrically distinct surfaces and 29,843 distinct coordination environments, all carefully characterized based on the surface and the adsorbate neighbors.
The utilization of these comprehensive and diverse databases ensures that the findings of our study are both robust and pertinent, paving the way for significant contributions to the field. The data distribution is largely normal and is, therefore, deemed suitable for ML methods. For details, please refer to [Supplementary S1]. The training set comprises 80% of the dataset, while the test and validation sets account for 10% each.
Structure representation
This study uses a graph-based representation of structural properties mostly based on local properties. These properties are determined by looking at the differences in elemental properties between an atom and its neighboring atoms. Specifically, the local property difference for each atom is calculated by taking the face-weighted average of the absolute differences in elemental properties between that atom and each of its neighboring atoms. Voronoi tessellation (VT)[48], also known as Voronoi diagram or Voronoi partitioning, is a mathematical method used to divide a space into a number of regions based on distance to a specific set of points known as Voronoi sites. Each point in the given space is associated with the closest Voronoi site, creating a Voronoi cell around each site. These cells together form a tessellation that covers the entire space without overlap or gaps. In addition, the study obtains data from the Open Quantum Materials Database (OQMD)[49], which includes Specific Volume, Band Gap Energy, Magnetic Moment (per atom), and Space Group Number of 0 K Ground States. By analyzing a total of 22 different elemental properties, the study calculates the mean, mean absolute deviation, maximum, and minimum values of the local property differences for each atom, which are used to create the elemental properties.
The VT method offers several advantages, including freedom from parameter tuning, transferability, and reproducibility. The infinite vertices issue is addressed without introducing any human input parameters, which ensures the accuracy and integrity of the calculations. Furthermore, this approach is applicable to general hydrogen surface adsorption problems and is independent of the symmetry and composition of the adsorbent surface. However, when applying the original VT technique to the surface adsorption system, the adsorbate is considered in connection with the atom on the surface, even at infinity [Figure 2]. This poses a critical issue when using the method to determine the first nearest neighbors of the adsorbate since such infinite interactions are unphysical. To address this problem, we modify the VT method by introducing a pseudo-surface above the actual surface by reflecting each site about the adsorbate [Figure 2]. The VT operation is then carried out to extract the nearest neighbors of the adsorbate. It is worth noting that VT identifies the nearest neighbors of the adsorbate in both the actual and pseudo surfaces. However, only the molecule-like structures containing the adsorbate and those on the actual adsorbent surface will be considered in subsequent calculations. In practice, a
Figure 2. (A) Illustration of the infinite vertices problem when applying Voronoi tessellation to surface adsorption systems. The solid black and blue points represent slab atoms and the adsorbate (proton), respectively; (B) Illustration of the modified Voronoi tessellation. The pseudo surface (depicted by the black circles) is constructed by reflecting the actual adsorbent surface (represented by the solid black and orange points) about the adsorbate (indicated by the solid blue point). The highlighted regions encompass the molecule-like structure, which includes the proton and its nearest neighbors on the actual surface, representing the adsorption system.
To prove the efficiency of our modified VT method in complex neural networks, we apply it to optimize the original graph structure of CGCNN into a Voronoi structure input. As depicted in [Supplementary S2], the modfied CGCNN achieves superior convergence performance compared to the original CGCNN that uses the conventional graph input. The faster training speed of modified CGCNN is especially important for large datasets because DL algorithms often require long training cycles due to the large number of hidden layers in neural networks.
Feature engineering of local environment interaction
We first improved the crystallography neural network[33]. The atomic radius is a feature that better describes in vitro steric effects [35]. However, atomic radii may also change due to changes in the environment. In the present work, we use the atomic number instead of this feature as it is simple and deterministic. Pauling electronegativity has been shown to be a good feature of electron affinity[50]. To account for steric and ambient electron effects, the coordination number has been identified to be a successful feature[51]. Crude estimates of the properties have proved successful and can improve predictive power, so we use the average adsorption energy as a description. Additionally, we included the atom distance to the adsorbate H, a parameter directly related to the adsorption energy magnitude in adsorption. Finally, we added the valence number which is calculated as the average of the elements within all the layers.
For the neighboring elements at each adsorption site, we utilized six elemental properties as follows: the atomic number of the element (Z), the Pauling electronegativity of the element (
Generally, including two layers of nearest neighbors is regarded as effective in capturing the local information of atoms[37,42]. However, in the experiment, we found that only considering two layers of atoms has poor accuracy in some cases, especially for nanoparticles or rough surfaces, because when the adsorption site is located at the corner, it contains too few neighbor atoms. Therefore, we propose considering incorporating the third neighbor layer. Experimentally, in surface adsorption, this method can adequately contain the information needed to calculate the adsorption energy. Our final result demonstrates that only six descriptors for each layer can make the model reach the highest accuracy.
Applying local environment interaction for convolutional networks
In the latest adsorption energy calculations, graph neural networks are typically employed. Our descriptors of the LEI-framework can also be transformed into a
where each
For an input with three layers and eleven elements, the input X can be represented as:
Here,
where
where
The residual function
where
Our framework possesses two key features to ensure the robustness and high performance of the model in adsorption tasks. Firstly, we adopt the mean absolute error as the loss function and train the network with the Adam optimization algorithm, ensuring the robustness and adaptability of the model. This choice not only adapts to adsorption tasks with different complex structures but also ensures that the model performs exceptionally well in various data scenarios. Secondly, we use the ResNet-34 model as our backbone network. ResNet-34 consists of 33 convolutional layers, providing depth and capability to the model, enabling it to be applied to larger databases, thereby enhancing the model accuracy and robustness. ResNet-34 is a widely used DL framework with proven outstanding performance in numerous fields. Our choice also provides robust support for adsorption tasks. Overall, by constructing a convolutional network based on LEI-framework, we integrate the high-importance features of the three nearest neighbor atoms surrounding the adsorbate and learn the relationships between elements of each layer. Then, we learn from the data of a large dataset based on the convolutional network. Introducing ResNet reduces the learning difficulty of the model and enhances its generalizability.
Regression result evaluation
To train the model, several regression outcome evaluation methods are employed. The true and predicted values in the experiment are denoted as:
True value:
Predicted value:
Performance metrics for all methods used in this article include Median Absolute Error (MDAE), Mean Absolute Error (MAE), Root Mean Squared Error (RMSE), and Mean Absolute Relative Percent Difference (MARPD). The median absolute error is particularly interesting because it is robust to outliers, with its unit being eV. The median absolute error estimated over
MARPD is used because it provides normalized measures of accuracy that may be more interpretable for those unfamiliar with adsorption energy measurements in eV, as determined by
RESULTS AND DISCUSSION
In this work, we propose a comprehensive and universal framework for predicting molecular adsorption energy on surfaces, which involves three key steps [Figure 1]. Firstly, we transform the original material structure into a graph-based 2D Voronoi diagram and extract improved fingerprint information. Next, we further optimize the descriptors using ML techniques. Finally, we utilize the powerful performance of DL to construct, train, and predict. This approach enables us to capture important descriptors of the adsorption process and achieve high prediction accuracy using either traditional ML or advanced DL algorithms.
Testing local environment approach with traditional machine learning models
Traditional ML algorithms have high interpretability and can output weights for descriptors, so they are often used to improve descriptors[52]. In this ML Section, to test the performance of our local environment interaction-based approach, we applied three widely-used algorithms, namely the Gradient Boosting (GB)[53], K-Nearest Neighbors (KNN)[54], and Random Forest (RF)[55]. In addition, for some systems with specific requirements, we provide a simple path to use the RF algorithm to determine the feature importance in the training process to fine-tune the model, refer to [Supplementary S3] for specific results upon further analysis.
Table 1 summarizes the training results. The random forest model incorporating the descriptors of the LEI-framework produces the lowest MAE value of 0.13 eV. The RF algorithm outperforms the other two ML methods thanks to its ability to integrate decision trees and capture complex inter-atomic relationships. In addition, the ML models using the improved VT-based fingerprint descriptors [Figure 1, module 2] outperform those using conventional VT-based descriptors [Figure 1, module 1]. This is because the conventional VT method cannot incorporate layered chemical information about adsorbed hydrogen atoms into the model. As a result, the descriptors of the LEI-framework showed reliable performance in predicting the H adsorption energy of catalytical processes with significantly lower error rates.
Performance comparison of machine learning models using different descriptors [Figure 1]
Model | Algorithm | MDAE | MAE | RMSE | MARPD |
Using conventional VT-based descriptors | GB | 0.20 | 0.28 | 0.42 | 108% |
KNN | 0.20 | 0.29 | 0.44 | 105% | |
RF | 0.19 | 0.27 | 0.41 | 103% | |
Using improved VT-based fingerprint descriptors | GB | 0.16 | 0.20 | 0.27 | 89% |
KNN | 0.11 | 0.17 | 0.25 | 72% | |
RF | 0.08 | 0.14 | 0.22 | 61% |
Testing local environment approach with advanced deep learning models
To check whether our approach can also apply for DL models and whether advanced DL models have higher performance than traditional ML models, we introduce a ResNet[56] into our model. The ResNet has excellent tunability and fast training speed, allowing for greater versatility in applying our model to a wider range of adsorbates and scenarios. Additionally, its strong generalization performance facilitates easy portability of our model to other fields. Considering the local environment descriptor input as a list type [Figure 1], which was previously represented by a
The detailed structure is shown in Table 2.
The structure of local environment ResNet
Layer name | Output size | 34-Layer |
Conv1 | ||
Conv2_x | ||
Conv3_x | ||
Conv4_x | ||
Conv5_x | ||
AdaptiveAvgPool |
The prediction results of the LERN model are shown in Figure 3. The Residual plot is beneficial for analyzing the distribution of prediction errors. In the scatter plot on the left, the x-axis and y-axis represent the LERN training process and the residuals between predicted and actual values on both the training and test sets, respectively. The overall residual regression line is also calculated and displayed in the plot. The red and blue parts represent the training and test sets, respectively. It can be observed that the LERN prediction results are highly consistent with the actual values on both the training and test sets, as evidenced by the regression lines of the residuals with slopes close to zero. On the right, the overall distribution of residuals on the training and test sets is displayed. The results show that the distribution of residuals on both the training and test sets is close to normal distribution, indicating that the model is well-suited for learning from this type of data. Moreover, the distributions of residuals on the training and test sets are very similar, indicating that the model does not suffer from obvious overfitting. Therefore, the LERN prediction results are highly consistent with the actual DFT calculation results within the allowable error range, suggesting that LERN has DFT-level accuracy.
Figure 3. Residual plot of LERN models. The left-hand side is a scatter plot, where the x-axis is the predicted value of the model on the training and test sets, the y-axis is the corresponding predicted value minus the true value, and the middle corresponds to its regression line, respectively. On the right-hand side are the residual distributions for the training and test sets, respectively. LERN: Local environment input into ResNet.
To benchmark the performance of our proposed model in a materials database, we use other state-of-the-art neural networks. We compare our LERN with the original CGCNN, SchNet[57], AliGNN[6], and our Modified CGCNN. To elaborate further, the robustness of LERN in dealing with outliers is a highly desirable trait in ML models. Outliers are data points that deviate significantly from the normal distribution of the dataset, and they can occur due to various reasons such as measurement errors or anomalous samples. The presence of outliers can negatively affect the performance of a model, especially if it is not designed to handle them properly.
In contrast [Figure 4], the orange points represent the distribution of outliers for each model. Of these, LERN has only 86 outliers, while the other models all have around 110. This suggests that LERN has demonstrated an impressive ability to handle outliers effectively, which is a crucial advantage in real-world applications where data quality is often suboptimal. By being insensitive to outliers, LERN can deliver reliable predictions even in the presence of noisy data. This is particularly relevant in materials science, where experimental data can be scarce, noisy, or incomplete, making it challenging to develop accurate models.
Figure 4. (A) H adsorbed on 3D materials (B) and (C) performance comparison of LERN with other representative models on 3D materials. op*: Outlier percentage. 3D: Three-dimensional; LERN: local environment input into ResNet.
Moreover, the performance of LERN on limited training data samples is noteworthy. Discrete errors often arise from inadequate training data or inherent low similarity in the training set, and the error distribution of LERN is more concentrated, indicating higher prediction accuracy and consistency. The ability to learn from a small amount of data is an important aspect of ML, as it allows for the development of models that can be trained with fewer computational resources and time. This is particularly important in fields such as materials science, where experiments can be both expensive and time-consuming. The ability of LERN to effectively learn from limited data suggests that it has the potential to significantly accelerate the discovery and design of new materials. As depicted in Figure 4, LERN surpasses other models in predicting the adsorption energy of Hydrogen Evolution Reaction (HER). During our training, we discovered that, akin to Modified CGCNN, the accuracy of LERN remains stable even with fewer iterations and smaller sample sizes, whereas other models show significant deterioration under low data conditions. This finding reiterates that the descriptors of the LEI-framework are more fitting for limited data scenarios, reflecting the current state of most catalytic databases. Such high data efficiency can be attributed to the ability of the model to extract vital local distance information and atomic properties from the structure, embedding system knowledge and input-output correlations. LERN can rapidly concentrate on the features surrounding the adsorption site using the training dataset, whereas other models must laboriously learn all atomic correlations without the benefit of system knowledge.
To demonstrate the generality of our LERN model, we apply it on a molecular adsorption dataset based on 2D materials, which still shows stable performance superiority [Figure 5]. This is because our improved VT-based feature engineering of the model only focuses on local information, independent of the material scale and thickness. The model also performs well on small datasets due to the introduction of a deep residual structure and the sharing of parameters. Using a dataset of 1,283 hydrogen adsorption sites[45] from the 2Dmatpedia database[46], we refined 272 HER sites with the LERN. Out of these, seven have been previously reported in experiments, 69 have been noted in other computational studies, and the rest 196 have never been reported before.
Figure 5. (A) H adsorbed on 2D materials (B) and (C) Performance comparison of LERN with other representative models on 2D materials. op*: Outlier percentage. 2D: LERN: local environment input into ResNet.
The results presented above demonstrate that the model exhibits robust performance across molecules of varying sizes. It is particularly noteworthy for addressing the longstanding challenge in the materials science domain of applying DL techniques to large molecules or 2D materials. As illustrated in Figure 6, our model maintains exceptional precision for metallic nanoparticles containing around 80 atoms. Beyond that, our model can be further generalized to surfaces. In comparison, the benchmark model only achieves commendable results on crystalline materials. Moreover, the training time of LERN is faster than that of all other neural networks by an order of magnitude. Notably, all the aforementioned models were trained on a single RTX3080. When iterating 200 times, with the exception of LERN, the training time for all models exceeded two hours, while LERN required only approximately 26 min. This is considerably faster than other conventional graph neural networks while the accuracy is greatly optimized. The above results show that our model solves the current challenges of graph neural networks faced with the computational complexity and information capture problems present in complex systems. At the same time, because we only focus on the local characteristic information of the adsorption site, our model can be adapted to systems with wider system diversity. It helps develop catalysis research in different dimensions.
Figure 6. (A) and (B) The H adsorption sites on a sample of nanoparticles and 2D materials, respectively; (C) The comparison of the adsorption energy prediction results of LERN with the DFT calculation and the benchmark (ALiGNN) results. 2D: Two-dimensional; LERN: local environment input into ResNet; DFT: Density Functional Theory; ALiGNN: Atomistic Line Graph Neural Network.
CONCLUSIONS
In summary, we have spearheaded a novel and universal ML framework utilizing the local environment interaction to enhance feature input for effectively predicting molecular adsorption energy. Within this framework, descriptors are constructed by modified graph-based VT representation (geometric information) and improved fingerprint feature engineering (chemical information). These descriptors can be input into conventional ML models as weighted features or deep neural networks as a graph form. We took conventional ML, CGCNN, and ResNet as examples across diverse systems, including 0D, 2D and 3D catalysts, to demonstrate the robustness and generalization of this framework. Such local environment interaction-based descriptors make a lightweight advantage over other models, showcasing a significant boost in computational speed. This universal and robust LEI-framework can be expanded to broader applications, such as catalysis, sensors, and drug design.
DECLARATIONS
Authors’ contributions
Made substantial contributions to the conception and design of the study and performed data analysis and interpretation: Li Y, Wu Y, Han Y, Shen L
Conducted data acquisition and contributed to the feature engineering part and model training: Lyu Q, Wu H, Zhang X
Availability of data and materials
The source code of our work is freely accessible at https://github.com/mpeshel/LEI-framework_LERN.
Financial support and sponsorship
This work was supported by Singapore MOE Tier 1 (No. A-8001194-00-00) and Singapore MOE Tier 2 (No. A-8001872-00-00).
Conflicts of interest
All authors declared that there are no conflicts of interest.
Ethical approval and consent to participate
Not applicable.
Consent for publication
Not applicable.
Copyright
© The Author(s) 2024.
REFERENCES
1. Sha W, Guo Y, Yuan Q, et al. Artificial intelligence to power the future of materials science and engineering. Adv Intell Syst 2020;2:1900143.
2. Choudhary K, DeCost B, Chen C, et al. Recent advances and applications of deep learning methods in materials science. npj Comput Mater 2022;8:59.
3. Behler J, Parrinello M. Generalized neural-network representation of high-dimensional potential-energy surfaces. Phys Rev Lett 2007;98:146401.
4. Schütt KT, Kindermans PJ, Sauceda HE, Chmiela S, Tkatchenko A, Müller KR. SchNet: a continuous-filter convolutional neural network for modeling quantum interactions. In: Proceedings of the 31st International Conference on Neural Information Processing Systems. Curran Associates Inc.; 2017. pp. 992-1002. Available from: https://dl.acm.org/doi/abs/10.5555/3294771.3294866. [Last accessed on 28 Mar 2024].
5. Xie T, Grossman JC. Crystal graph convolutional neural networks for an accurate and interpretable prediction of material properties. Phys Rev Lett 2018;120:145301.
6. Choudhary K, DeCost B. Atomistic line graph neural network for improved materials property predictions. npj Comput Mater 2021;7:185.
7. Chen C, Ye W, Zuo Y, Zheng C, Ong SP. Graph networks as a universal machine learning framework for molecules and crystals. Chem Mater 2019;31:3564-72.
8. Zuo Y, Qin M, Chen C, et al. Accelerating materials discovery with Bayesian optimization and graph deep learning. Mater Today 2021;51:126-35.
9. Conway BE, Tilak BV. Interfacial processes involving electrocatalytic evolution and oxidation of H2, and the role of chemisorbed H. Electrochim Acta 2002;47:3571-94.
10. Loffreda D. Theoretical insight of adsorption thermodynamics of multifunctional molecules on metal surfaces. Surf Sci 2006;600:2103-12.
11. Thomas N, Smidt T, Kearnes S, et al. Tensor field networks: rotation- and translation-equivariant neural networks for 3D point clouds. arXiv.[Preprint] 18 May 2018 [accessed on 2024 Mar 28]. Available from: https://arxiv.org/abs/1802.08219.
12. Batzner S, Musaelian A, Sun L, et al. E(3)-equivariant graph neural networks for data-efficient and accurate interatomic potentials. Nat Commun 2022;13:2453.
13. Brandstetter J, Hesselink R, van der Pol E, Bekkers EJ, Welling M. Geometric and physical quantities improve E(3) equivariant message passing. arXiv.[Preprint] 26 Mar 2022 [accessed on 2024 Mar 28]. Available from: https://arxiv.org/abs/2110.02905.
14. Batatia I, Kovács DP, Simm GNC, Ortner C, Csányi G. MACE: higher order equivariant message passing neural networks for fast and accurate force fields. arXiv.[Preprint] 26 Jan 2023 [accessed on 2024 Mar 28]. Available from: https://arxiv.org/abs/2206.07697.
15. Musaelian A, Batzner S, Johansson A, et al. Learning local equivariant representations for large-scale atomistic dynamics. Nat Commun 2023;14:579.
16. Liao YL, Smidt T. Equiformer: equivariant graph attention transformer for 3D atomistic graphs. arXiv.[Preprint] 28 Feb 2023 [accessed on 2024 Mar 28]. Available from: https://arxiv.org/abs/2206.11990.
17. Zitnick CL, Das A, Kolluru A, et al. Spherical channels for modeling atomic interactions. arXiv.[Preprint] 13 Oct 2022 [accessed on 2024 Mar 28]. Available from: https://arxiv.org/abs/2206.14331.
18. Chanussot L, Das A, Goyal S, et al. Open catalyst 2020 (OC20) dataset and community challenges. ACS Catal 2021;11:6059-72.
19. Passaro S, Zitnick CL. Reducing SO(3) convolutions to SO(2) for efficient equivariant GNNs. arXiv.[Preprint] 14 Jun 2023 [accessed on 2024 Mar 28]. Available from: https://arxiv.org/abs/2302.03655.
20. Lan J, Palizhati A, Shuaibi M, et al. AdsorbML: a leap in efficiency for adsorption energy calculations using generalizable machine learning potentials. npj Comput Mater 2023;9:172.
21. Wang Z, Wang C, Zhao S, et al. Heterogeneous relational message passing networks for molecular dynamics simulations. npj Comput Mater 2022;8:53.
22. Zhong Y, Yu H, Su M, Gong X, Xiang H. Transferable equivariant graph neural networks for the Hamiltonians of molecules and solids. npj Comput Mater 2023;9:182.
23. Al Zoubi W, Assfour B, Allaf AW, Leoni S, Kang JH, Ko YG. Experimental and theoretical investigation of high-entropy-alloy/support as a catalyst for reduction reactions. J Energy Chem 2023;81:132-42.
24. Liu W, Tkatchenko A, Scheffler M. Modeling adsorption and reactions of organic molecules at metal surfaces. Acc Chem Res 2014;47:3369-77.
25. Shee J, Rudshteyn B, Arthur EJ, Zhang S, Reichman DR, Friesner RA. On achieving high accuracy in quantum chemical calculations of 3d transition metal-containing systems: a comparison of auxiliary-field quantum monte carlo with coupled cluster, density functional theory, and experiment for diatomic molecules. J Chem Theory Comput 2019;15:2346-58.
26. Ghanekar PG, Deshpande S, Greeley J. Adsorbate chemical environment-based machine learning framework for heterogeneous catalysis. Nat Commun 2022;13:5788.
27. Yang Z, Gao W. Applications of machine learning in alloy catalysts: rational selection and future development of descriptors. Adv Sci 2022;9:2106043.
28. George J, Hautier G. Chemist versus machine: traditional knowledge versus machine learning techniques. Trends Chem 2021;3:86-95.
29. Zebari RR, Abdulazeez AM, Zeebaree DQ, Zebari DA, Saeed JN. A comprehensive review of dimensionality reduction techniques for feature selection and feature extraction. J Appl Sci Technol Trends 2020;1:56-70.
30. Otchere DA, Ganat TOA, Gholami R, Ridha S. Application of supervised machine learning paradigms in the prediction of petroleum reservoir properties: comparative analysis of ANN and SVM models. J Petrol Sci Eng 2021;200:108182.
31. Huang X, Ma S, Zhao CY, Wang H, Ju S. Exploring high thermal conductivity polymers via interpretable machine learning with physical descriptors. npj Comput Mater 2023;9:191.
32. Li CN, Liang HP, Zhang X, Lin Z, Wei SH. Graph deep learning accelerated efficient crystal structure search and feature extraction. npj Comput Mater 2023;9:176.
33. Isayev O, Fourches D, Muratov EN, et al. Materials cartography: representing and mining materials space using structural and electronic fingerprints. Chem Mater 2015;27:735-43.
34. Ward L, Agrawal A, Choudhary A, Wolverton C. A general-purpose machine learning framework for predicting properties of inorganic materials. npj Comput Mater 2016;2:16028.
35. Schütt KT, Glawe H, Brockherde F, Sanna A, Müller KR, Gross EKU. How to represent crystal structures for machine learning: towards fast prediction of electronic properties. Phys Rev B 2014;89:205118.
36. Luo Y, Du X, Wu L, Wang Y, Li J, Ricardez-Sandoval L. Machine-learning-accelerated screening of double-atom/cluster electrocatalysts for the oxygen reduction reaction. J Phys Chem C 2023;127:20372-84.
37. Tran K, Ulissi ZW. Active learning across intermetallics to guide discovery of electrocatalysts for CO2 reduction and H2 evolution. Nat Catal 2018;1:696-703.
38. Calle-Vallejo F, Martínez JI, García-Lastra JM, Sautet P, Loffreda D. Fast prediction of adsorption properties for platinum nanocatalysts with generalized coordination numbers. Angew Chem Int Edit 2014;53:8316-9.
39. Cao S, Luo Y, Li T, Li J, Wu L, Liu G. Machine learning assisted screening of doped metals phosphides electrocatalyst towards efficient hydrogen evolution reaction. Mol Catal 2023;551:113625.
40. Calle-Vallejo F, Tymoczko J, Colic V, et al. Finding optimal surface sites on heterogeneous catalysts by counting nearest neighbors. Science 2015;350:185-9.
41. Zhou C, Chen C, Hu P, Wang H. Topology-determined structural genes enable data-driven discovery and intelligent design of potential metal oxides for inert C–H bond activation. J Am Chem Soc 2023;145:21897-903.
42. Li X, Chiong R, Hu Z, Page AJ. A graph neural network model with local environment pooling for predicting adsorption energies. Comput Theor Chem 2023;1226:114161.
43. Li Y, Zhu R, Wang Y, Feng L, Liu Y. Center-environment deep transfer machine learning across crystal structures: from spinel oxides to perovskite oxides. npj Comput Mater 2023;9:109.
44. Chen R, Liu F, Tang Y, et al. Combined first-principles and machine learning study of the initial growth of carbon nanomaterials on metal surfaces. Appl Surf Sci 2022;586:152762.
45. Yang T, Zhou J, Song TT, Shen L, Feng YP, Yang M. High-throughput identification of exfoliable two-dimensional materials with active basal planes for hydrogen evolution. ACS Energy Lett 2020;5:2313-21.
46. Zhou J, Shen L, Costa MD, et al. 2DMatPedia, an open computational database of two-dimensional materials from top-down and bottom-up approaches. Sci Data 2019;6:86.
47. Tran K, Palizhati A, Back S, Ulissi ZW. Dynamic workflows for routine materials discovery in surface science. J Chem Inf Model 2018;58:2392-400.
48. Tanemura M, Ogawa T, Ogita N. A new algorithm for three-dimensional voronoi tessellation. J Comput Phys 1983;51:191-207.
49. Saal JE, Kirklin S, Aykol M, Meredig B, Wolverton C. Materials design and discovery with high-throughput density functional theory: the open quantum materials database (OQMD). JOM 2013;65:1501-9.
50. Li Z, Wang S, Chin WS, Achenie LE, Xin H. High-throughput screening of bimetallic catalysts enabled by machine learning. J Mate Chem A 2017;5:24131-8.
51. Calle-Vallejo F, Loffreda D, Koper MTM, Sautet P. Introducing structural sensitivity into adsorption-energy scaling relations by means of coordination numbers. Nat Chem 2015;7:403-10.
52. Cao Z, Dan Y, Xiong Z, et al. Convolutional neural networks for crystal material property prediction using hybrid orbital-field matrix and magpie descriptors. Crystals 2019;9:191.
54. Cover T, Hart P. Nearest neighbor pattern classification. IEEE Trans Inform Theory 1967;13:21-7.
56. He K, Zhang X, Ren S, Sun J. Deep residual learning for image recognition. In: 2016 IEEE Conference on Computer Vision and Pattern Recognition (CVPR); 2016 Jun 27-30; Las Vegas, USA. IEEE; 2016. pp. 770–8.
Cite This Article
Export citation file: BibTeX | RIS
OAE Style
Li Y, Wu Y, Han Y, Lyu Q, Wu H, Zhang X, Shen L. Local environment interaction-based machine learning framework for predicting molecular adsorption energy. J Mater Inf 2024;4:4. http://dx.doi.org/10.20517/jmi.2023.41
AMA Style
Li Y, Wu Y, Han Y, Lyu Q, Wu H, Zhang X, Shen L. Local environment interaction-based machine learning framework for predicting molecular adsorption energy. Journal of Materials Informatics. 2024; 4(1): 4. http://dx.doi.org/10.20517/jmi.2023.41
Chicago/Turabian Style
Li, Yifan, Yihan Wu, Yuhang Han, Qiujie Lyu, Hao Wu, Xiuying Zhang, Lei Shen. 2024. "Local environment interaction-based machine learning framework for predicting molecular adsorption energy" Journal of Materials Informatics. 4, no.1: 4. http://dx.doi.org/10.20517/jmi.2023.41
ACS Style
Li, Y.; Wu Y.; Han Y.; Lyu Q.; Wu H.; Zhang X.; Shen L. Local environment interaction-based machine learning framework for predicting molecular adsorption energy. J. Mater. Inf. 2024, 4, 4. http://dx.doi.org/10.20517/jmi.2023.41
About This Article
Special Issue
Copyright
Data & Comments
Data
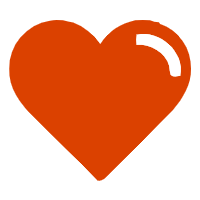

Comments
Comments must be written in English. Spam, offensive content, impersonation, and private information will not be permitted. If any comment is reported and identified as inappropriate content by OAE staff, the comment will be removed without notice. If you have any queries or need any help, please contact us at support@oaepublish.com.