Machine learning accelerated discovery of high transmittance in (K0.5Na0.5)NbO3-based ceramics
Abstract
High optical transmittance (T%) has always been an important indicator of transparent-ferroelectric ceramics for optoelectronic coupling. However, the pathway of pursuing high transparency has been at the experimental trial-and-error stage over the past decades, manifesting major drawbacks of being time-consuming and resource-wasting. The present work introduces a machine learning (ML) accelerated development of highly transparent-ferroelectrics by taking potassium-sodium niobate (KNN)-based ceramics as the model material. It is highlighted that by using a small data set of 118 sample data and four key features, we predict the T% of un-synthesized KNN-based ceramics and evaluate the importance of key features. Meanwhile, the screened (K0.5Na0.5)0.956Tb0.004Ba0.04NbO3 ceramics were successfully realized by the conventional solid-state synthesis, and the experimental measured T% is in full agreement with the predicted results, exhibiting a satisfactory high T% of ~78% at 800 nm. In addition, ML is also used to explore the best experimental parameters, and the prediction results of T% are particularly sensitive to changes in sintering temperature (ST). Eventually, the predicted optimal ST is highly consistent with the experimental one. This study constructs a new avenue for exploring high T% ferroelectric KNN ceramics based on ML, ascertaining optimal process parameters, and guiding the development of other transparent-ferroelectrics in optoelectronic fields.
Keywords
INTRODUCTION
Transparent-ferroelectric ceramics, capable of coupling multiple properties such as light, electricity, and mechanical deformation, are imminently demanded in industries such as energy storage[1,2], optical-electrical devices[3,4], and construction materials[5]. Traditionally, ferroelectric ceramics with high optical transparency are mainly lead-based materials in virtue of their synchronous excellent optical and electrical properties[6]. However, new material technology of environmental protection and low carbon, coupled with the industrial requirement of sustainable development, impels the urgent research of lead-free transparent-ferroelectric ceramics. Recently, a series of lead-free potassium-sodium niobate (KNN)-based ceramics with perovskite structure and good transparency have been successfully fabricated to achieve coupling of high optical transmittance (T%) and other optical-electrical performance through reasonable component design, accumulating a large amount of valuable literature[7-11] and experimental data. For instance, the combination of solid-state reaction and pressureless sintering techniques has been employed to introduce ions (e.g., rare-earth ion RE3+) into KNN[12] or incorporate solid solution [e.g., Ca(Sc0.5Nb0.5)O3] in the KNN host[13], leading to KNN-based ceramics with high T% and favorable di-/ferro-/piezoelectric properties. Nonetheless, substantial tentative experiments are required to validate if high performance can be obtained, which can exhibit inefficient and time-consuming drawbacks. Hence, the potential data value in these literature sources needs to be discovered via data mining. Moreover, the space of unknown components in KNN-based ceramics remains huge, highlighting the importance of integrating novel methods to accelerate the experimental design[14-16].
The research paradigm of AI For Science (AI4S) is gradually standardized[17]. Many new materials with outstanding characteristics so far have been found through machine learning (ML)[18-22], such as high Curie temperature (Tc) ferroelectric material[23-25], perovskite solar cells[26], double perovskites[27], microwave dielectric ceramics[28], photovoltaic peroxides[29], and energy-storage ceramics[30]. By efficiently searching for a high piezoelectric coefficient (d33) in a huge component space of BaTiO3-based ceramics using an ML strategy, He et al successfully found and synthesized the best component (Ba0.95Ca0.05)(Ti0.9Sn0.1)O3, in which the experimental value of d33 was consistent with the ML prediction[31]. Importantly, the structure-property relationship was analyzed by predicting phase diagrams, demonstrating that the highest d33 tended to appear in the multi-phase coexistence region, especially on the side of the rhombohedral phase. Additionally, Zhai et al. used ML models, such as Support Vector Machine (SVR) Regression and Random Forest (RF) Regression, to predict the Tc of perovskites and successfully searched for potential materials with high Tc using the SVR model and genetic algorithms[24].
It is well known that the T% of a ferroelectric ceramic is influenced by factors such as phase structure, density, porosity, birefringence and crystal anisotropy, grain size, size of grain boundary and corresponding phase, domain walls, and surface roughness of ceramic specimen. Moreover, over the past decades, the pursuit of high T% ferroelectric ceramics has predominantly relied on traditional empirical trial-and-error methods or the development and optimization of new sintering processes. These approaches aim to effectively and accurately control the above-mentioned factors in materials. However, the problem is that the process requires a long cycle time as T% is only obtained after successfully preparing the materials, which is inefficient for the development of transparent-ferroelectric ceramics. Therefore, it is of great interest and importance to analyze the relationship between material-related structures, process parameters, and the optimal T% values in ferroelectric ceramics of performance using ML models.
In this work, we used ML for elemental composition space search to guide the experimental design of KNN-based ferroelectric ceramics with high T%. We focused on specific components of KNN-based ceramics modified by both rare-earth ions and alkaline-earth ions. T% is used as a target function to build a mapping model between quantitative structure and property, and the process parameters are also considered in it. Among them, the high T% is what we are pursuing, and the best component searched by this model is finally successfully synthesized and characterized. The features affecting the transparency of ferroelectric ceramics were discovered for the first time through ML, allowing us to establish a clearer set of structure-performance correlative mechanisms and providing guidance for the accelerated design of ferroelectric-transparent ceramics.
METHODS
An ML procedure with T% as the target property is illustrated in Figure 1, including data collection and preprocessing, model training, model prediction, and experimental validation. Considering the generalization and feasibility of the data, we chose basic elemental properties to construct the characteristic pools, such as melting point, electronegativity, atomic number, and radius. After proving the reliability of the generalization ability of the established regression model, we combined predicted values of the model with correlations of the features to guide the quest for components with large T% in a wide component space.
Figure 1. Schematic workflow of the ML process, including data collection and preprocessing, model training, model prediction, and experimental validation. ETR: extreme Tree Regression; GBR: gradient boosting regression; KNN: potassium-sodium niobate; ML: machine learning; RF: random forest; ST: sintering temperature; SVR: support vector machine; T%: transmittance.
Dataset and feature pool
The introduction of rare-earth and alkaline-earth elements into KNN ceramics has been proven to be a feasible means to boost the optical T%. The corresponding component systems have so far accumulated a large amount of experimental data, and the hidden values contained are beneficial for data mining. The dataset includes 118 experimental samples from the published literature [Supplementary Information 1], with known sintering temperature (ST) and unified T% values (at 800 nm). All the samples were prepared via solid-state reaction, and the purpose is to minimize the influence of experimental parameters (holding temperature, holding time, pressure, etc.) on the ML model.
The features used in our study include electronegativity, ionic radius, valence electron distance, and many others that have been extensively reported in previous studies on ferroelectric materials[20,32,33]. In addition, we introduced ST as a new feature by considering the effect of process parameters on T%. And the features for each component were obtained by relative molar fraction weighting calculations (the calculation methods refer to Supplementary Information 2).
Machine Learning sections
All the algorithms used in the ML session are from the scikit-learn package. The pandas package and the SHapley Additive exPlanations (SHAP) package are used for data analysis and processing. The entire work is conducted in Python language. Optimal Extreme Tree Regression (ETR) model hyperparameters are as follows (n_estimators = 76, max_depth = 12, min_samples_split = 4), and the rest of the parameters are default. The 10-Fold Cross Validation (10-CV) is to divide the training data into ten parts, each as a test set and the rest as a training set, and average the results ten times to evaluate the generalization ability of the ML model. Leave-One-Out Cross-Validation (LOOCV) is similar to 10-CV in principle, leaving only one sample as the test set, which is suitable for small data sets, and LOOCV is more accurate but requires a lot of computational resources. The code used for feature screening, model training, and forecasting has been uploaded to GitHub (https://github.com/BWMa688/BWMa688/tree/jermyn_dev).
Experimental synthesis and measurements
(K0.5Na0.5)0.996-zTb0.004BazNbO3 (z = 0.01, 0.02, 0.03, 0.04, 0.05) (abbreviated as zBa-0.004Tb-KNN) ceramic samples were produced via traditional solid-state reaction method. According to the composition predicted from ML, Na2CO3 (99.99%), K2CO3 (99.99%), Nb2O5 (99.99%), Tb2O3 (99.99%), and BaCO3 (99.99%) were used as the raw materials (the supplier of chemicals is Shanghai Aladdin Biochemical Technology Co., Ltd., China), and ZrO2 balls (using planetary ball mill, QM-3SP2, Nanjing Nanda Instrument Co., Ltd., China) and anhydrous ethanol were ball-milled together for 12 h. The obtained mixture was thoroughly dried at 80 ºC and then calcined at 850 ºC for 4 h. After that, the calcined powders were again ball-milled for 12 h to promote mixing. After being dried and sieved, the acquired powders and 6 wt% polyvinyl alcohol (PVA) binder were mixed thoroughly and pressed with 300 MPa pressure to shape into discs (12 mm diameter and 1 mm thickness), followed by kept at 800 ºC for 2 h to dislodge the PVA. Eventually, all the wafers were sintered at 1,165 ºC, 1,180 ºC, or 1,195 ºC for 4 h. To maintain comparability, all the as-prepared ceramics were thinned and polished to a desired thickness to measure the optical (~0.4 mm) and ferroelectric (~0.2 mm) properties.
The T% spectra of the ceramic samples were measured using an ultraviolet-visible-infrared (UV-VIS-IR) spectrophotometer (PerkinElmer Lambda 950, USA). Their phase structures were characterized by X-ray diffraction (XRD, Rigaku Ultima III, Japan). Microstructures on the surface and in cross-section were observed and recorded with scanning electron microscopy (SEM, Zeiss Supra 55, Germany). According to the SEM images, grain size statistics were calculated using the “Nano Measure” software. The polarization-electric field (P-E) hysteresis loops were performed at room temperature under an applied electric field of 100 kV/cm by a ferroelectric test system (TF Analyzer 2000E, aixACCT Systems GmbH, Aachen, Germany). Prior to the test, Ag paste of 2 mm in diameter was coated on both surfaces of ceramics. The samples were then heated to 650 ºC in a box furnace and kept at that temperature for 15 minutes.
ML-BASED MODEL DESIGN FOR KNN-BASED TRANSPARENT CERAMICS
Regression models of optical transmittance
Among the constructed feature pools, we use two steps to identify the best combination of features: Pearson correlation analysis and model selection. The features were grouped based on the Pearson correlation coefficients greater than 0.8 and less than -0.8 so as to determine that the features in each group are highly correlated, as shown in Figure 2A. In each assigned subgroup, we picked one common feature related to the target attribute and then removed the remaining redundant features in each group. Supplementary Table 2 shows the specific feature groupings. Four features, i.e., ST, the ratio of valence electron number with nuclear charge (Ven/NC), electron affinity (EA), and cell parameters in the c-direction (C-c), are not correlated with the remaining features. The final seven features chosen are ST, Ven/NC, EA, C-c, Schubert valence electron distance (Dve), Schubert core electron distance (Dce), and Matyonov-Batsanov electronegativity (E-MB).
Figure 2. Building a T% prediction model. (A) Plot of Pearson correlation coefficient for 28 initial features; (B) Model selection using seven features ST, Dve, Dce, Ven/NC, E-MB, EA, and C-c; (C) 10-CVerror from ETR model containing a subset of the retained descriptors; (D) Performance of ETR models trained with 4-tuple features (ST, Ven/NC, Dce, and Dve) on the training and testing sets. C-c: cell parameters in the c-direction; Dve: schubert valence electron distance; Dce: schubert core electron distance; EA: electron affinity; E-MB: matyonov-batsanov electronegativity; ETR: extreme tree regression; GBR: gradient boosting regression; GPR: gaussian process regression; PLS: partial least squares; RF: random forest; ST: sintering temperature; SVR: support vector machine; T%: transmittance; Ven/NC: the ratio of valence electron number with nuclear charge.
We use the seven features that have been retained from the feature pool to evaluate the performance of several common ML models, including Ridge, RF, SVR, Gradient Boosting Regression (GBR), ETR, Gaussian Process Regression (GPR), and Partial Least Squares (PLS), as shown in Figure 2B. Then the prediction accuracy was evaluated by using Eq. (1) and (2):
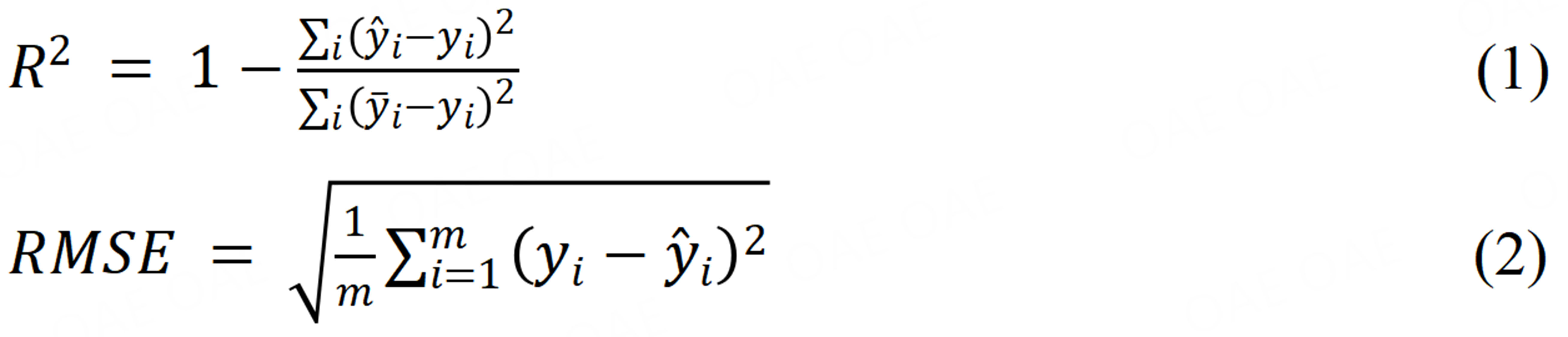
where m is the number of samples in the training dataset, yi is the measured value, ŷi is the predicted value, and
Design of highly transparent ceramics
Based on the best ETR model for predicting T%, we attempted to find out the highest T% in the given composition, (K0.5Na0.5)xRyMzNbO3, where x and z vary in steps of 0.01 with constraints 0.94 ≤ x ≤ 0.98, 0.01 ≤ z ≤ 0.05, y = 0.004, 0.01, and x + y + z = 1 (R = Tb, Sm, and Pr; M = Ba, Sr, Ca, and Mg). The component boundaries refer to the solubility of dopants, the region of dopant elements in the dataset, and the feasibility of the experimental part. There are 60 possible compounds in the search space, among which the Pr-Ba and Sm-Ba[34] components in the R-M system appearing in the training set exhibit high T%, and the remaining components are not present in the training data. The ability to generalize the model in the prediction process is important to guide the design of KNN-based material.
The component search of the ETR model for the T% of KNN-based ceramics is shown in Figure 3, and the top 5 components sorted by T% are listed in Table 1. The rare-earth and alkaline-earth co-doped KNN-based ceramics exhibit high T% in the predicted results, mainly existing in the Tb-doped components. Tb[35,36] or Ba[34,37] alone doped KNN-based ceramics are easy to obtain high transparency, which is attributed to their large band gap energy (Eg). The grain size was reduced with the increase of Tb3+ doping, resulting in lattice distortion and then a pinning effect to inhibit the grain growth. Ba2+-modified KNN-based ceramics possess high relative density, high crystal structure symmetry, nanosized grains, and large Eg, benefitting in high optical transparency. It can be predicted that Tb and Ba co-doping may further improve the optical transparency of KNN ceramics. To validate the prediction, we finally chose the zBa-0.004Tb-KNN system with the predicted highest T% for the following experiments
Figure 3. The predicting T% of (K0.5Na0.5)xRyMzNbO3 components based on the best ETR model. (A) R = Sm, ST = 1,175 ºC ; (B) R = Pr, ST = 1,175 °C; (C) R = Tb, ST = 1,175 °C; (D) R = Sm, ST = 1,180 °C; (E) R = Pr, ST = 1,180 °C; (F) R = Tb, ST = 1,180 °C. ETR: extreme tree regression; ST: sintering temperature; T%: transmittance.
The top 5 components are sorted by T% value
Rank | Component | ST (ºC) | T% |
1 | (K0.5Na0.5)0.956Tb0.004Ba0.04NbO3 | 1,180 | 80.1% |
2 | (K0.5Na0.5)0.946Tb0.004Ba0.05NbO3 | 1,180 | 76.5% |
3 | (K0.5Na0.5)0.966Tb0.004Ba0.03NbO3 | 1,180 | 71.3% |
4 | (K0.5Na0.5)0.956Tb0.004Sr0.04NbO3 | 1,180 | 65.8% |
5 | (K0.5Na0.5)0.95Sm0.01Sr0.04NbO3 | 1,180 | 65.0% |
Composition-property relationship for transmittance analysis
In order to in-depth understand the relationship between the optimal feature combination and the high T% of KNN-based ceramics, we used a SHAP interpreter that originated from cooperative game theory to explain the global and local aspects of the sample and prediction models. In 2017, Lundberg and Lee[38] first used SHAP values to explain various ML models, making them explicable. Figure 4 shows the global explanation of SHAP theory for the factors influencing the T% of KNN-based ceramics. We analyzed four features that influence the T% of KNN-based ceramics and ranked the influences. The most influential four features are ST, Ven/NC, Dce, and Dve. It can be observed that the contribution of the process parameter ST to T% is much greater than other features. This indicates that accurate ST is the key to achieving optimal T%, in which low ST can deteriorate T%, but high ST has a very low gain in T%. And only moderate ST can obtain high T% in KNN-based ceramics.
Figure 4. Plot of feature importance ranking based on SHAP value. (A) each point represents a sample; each row corresponds to a feature. Crowded places indicate the accumulation of a large number of samples. The color indicates the size of the feature value (red indicates high feature value, and blue indicates low feature value), and the horizontal axis indicates the positive or negative SHAP value; (B) histogram of SHAP average absolute value sorting. Dve: schubert valence electron distance; Dce: schubert core electron distance; SHAP: shapley additive explanations; ST: sintering temperature; Ven/NC: the ratio of valence electron number with nuclear charge.
To further explain the relationship between each feature and the T% of KNN-based ceramics, the feature combination was partially explained. As shown in Figure 5, the solid green box represents the region with positive SHAP values (gain contribution region). In Figure 5A, the SHAP value of ST is maximum when ST is near 1,180 ºC, indicating that the gain to T% increases significantly at this time. As the ST increases, the SHAP value gradually decreases and then stabilizes. As shown in Figure 5B, the SHAP value shows a clear upward trend and increases rapidly from near 0 to ~15 as Ven/NC increases to a certain range, indicating that higher Ven/NC has a positive effect on the T% of KNN-based ceramics. This is also consistent with the trend of the joint distribution of Ven/NC and T%, as shown in Supplementary Figure 2. Figure 5C shows that the SHAP value of Dce fluctuates widely, then the SHAP value mapping relationship between Dce and ST was analyzed in Supplementary Figure 3. Obviously, a lower Dce is more favorable for KNN-based ceramics to obtain higher T% when ST is 1,180 ºC. In Figure 5D, the SHAP value of Dve varies dramatically within a certain range, but as the fourth important feature, Dve combined with other features (i.e., ST, Ven/NC, and Dce) enhances the performance of the ETR model.
Figure 5. Dependency plots of individual features versus SHAP values in the ETR mode. Each feature contributes a SHAP value to the predicted value, and the positive and negative SHAP values represent gains and deductions. (A) SHAP value of ST; (B) SHAP value of Ven/NC; (C) SHAP value of Dce; (D) SHAP value of Dve. Dve: schubert valence electron distance; Dce: schubert core electron distance; SHAP: shapley additive explanations; ST: sintering temperature; Ven/NC: the ratio of valence electron number with nuclear charge.
We mapped the feature values corresponding to the five components in Table 1 into the SHAP interpreter (dashed part), also meeting sufficient conditions to obtain a high T%, indicating that the training effect of the ETR model follows the prediction effect. In addition, we fine-tuned the ST when predicting the optimal components. The ETR model was identified to be highly sensitive to the key process parameter ST, and the highest T% was eventually considered to be at ST = 1,180 ºC. The predicted results are consistent with the SHAP explanation of the training set regarding ST, further demonstrating that the appropriate ST can promote high transparency of KNN-based ceramics. Lin et al. investigated the effect of different STs on the transparency of KNN-based ceramics, and a slightly varying ST (10-15 ºC) resulted in a change in transparency[3]. This conclusion will be verified in the subsequent experimental section.
Experimental results
In order to validate the predicted T% and prove the reliability of the ETR model, we synthesized zBa-0.004Tb-KNN (z = 0.03, 0.04, 0.05) ceramics using the traditional solid-state reaction. T% of the ceramic samples and T% of optimal samples sintered at different temperatures were measured. Figure 6 shows the comparison between the T% spectrum and ML predictions of the zBa-0.004Tb-KNN ceramics. It can be clearly observed from Figure 6A that T% first increases and then decreases with increasing Ba2+ concentration (i.e., z). Among them, 0.04Ba-0.004Tb-KNN exhibits the highest T% of 78% at 800 nm, and the outstanding optical transparency can be substantiated by the photographs [Figure 6A]. Importantly, the trend of T% and the highest experimental value (78% at 800 nm) are in line with the ML predictions (80.1% at 800 nm) [Figure 6B]. In addition, the best sample 0.04Ba-0.004Tb-KNN with the optimal T% was used to investigate the spectra of ceramics at different STs, as displayed in Figure 6C. The T% of 0.04Ba-0.004Tb-KNN first increases and then slightly decreases with rising ST, showing the highest T% (monitored > 600 nm) at ST ~ 1,180 ºC. The change tendency is also compatible with that predicted by ML [Figure 6D], demonstrating that this ML method is extremely sensitive to the key process parameter, i.e., ST.
Figure 6. (A) Optical transmittance spectra of the zBa-0.004Tb-KNN ceramics at ST = 1180 °C and photograph of the highest T% sample (i.e., z = 0.04) with a thickness of ~0.4 mm (the insets); (B) Comparison between the experimentally measured T% and ML predictions for different Ba2+ concentrations (i.e., z); (C) Optical transmittance spectra of the 0.04Ba-0.004Tb-KNN ceramics at different ST; (D) Comparison between the experimental results and ML predictions for T% of 0.04Ba-0.004Tb-KNN at different STs. KNN: potassium-sodium niobate; ST: sintering temperature.
Figure 7 shows the XRD patterns of the zBa-0.004Tb-KNN ceramics. As all samples possess typical perovskite phases of KNN, indicating that Tb3+ and Ba2+ have well diffused into the lattice. Ion substitutions in other reported ferroelectrics are usually based on similar ionic radii. Depending on the ionic radii of K+ (1.64 Å), Na+ (1.39 Å), Ba2+ (1.61 Å), Tb3+ (1.095 Å) having a coordination number of 12, and Nb5+ (0.69 Å) having a coordination number of 6, most Ba2+ preferentially replace K+ at the A site and the rest of Ba2+ may displace Na+ at the A site. Similarly, most Tb3+ tend to displace Na+ first and later K+ site. The content of Tb3+ in this experiment was only 0.4%, so combined with doping concentration and ionic radius, Tb mainly replaces the A-site and does not enter the B-site. Previous studies have reported that Ba2+ and Tb3+ preferentially replace the A-site in KNN[5,36]. When the KNN-based ceramics are sintered at high temperatures ( > 1,100 ºC), the inevitable volatilization of alkali metal elements leads to the formation of impurity phases. Obviously, zBa-0.004Tb-KNN does not exhibit secondary phases at z = 0.03 and 0.04, indicating very low volatilization of alkali metal elements. With further increasing the Ba2+ content to 0.05, Ba2+ cannot fully enter the KNN matrix, resulting in the production of secondary phase BaNb3.6O10 (PDF#46-0942) at z = 0.05, which can partly reduce the T%. Similar effects of BaNb3.6O10 on T% were also found in other KNN-based ferroelectric ceramics[5,34]. Additionally, the phase structure of KNN-based ferroelectrics can be roughly judged from the diffraction peaks in the 2θ range of 45-47º. For the samples with z = 0.03 and 0.04, only one (200) diffraction peak can be observed at ~45.8º, verifying that they own pseudo-cubic phases with high symmetry. And the optical anisotropy of the pseudo-cubic structure is slight, which effectively reduces the light scattering and ultimately improves the light transmission of samples. While z = 0.05, the diffraction peak at ~45.5º shows a splitting tendency, and the phase structure deviates from pseudo-cubic, thus deteriorating the transparency. Therefore, we can conclude that the 0.04Ba-0.004Tb-KNN ceramic has the highest T%.
Figure 7. XRD patterns of the zBa-0.004Tb-KNN ceramics. (A) z = 0.03, 0.04, and 0.05; (B) XRD amplification of 2θ at 45-47°. KNN: potassium-sodium niobate.
Figure 8 displays the SEM images of the external surfaces and cross-sections and the grain size of the zBa-0.004Tb-KNN ceramics. Typical cubic grains are present in all ceramics. For the sample with z = 0.04, homogeneous grains (with a size in the range of 80 - 100 nm) and smaller pores can be observed [Figure 8B), while the grain distribution is not uniform at z = 0.03 [Figure 8A] and z = 0.05 [Figure 8C], especially for z = 0.05 that some grains appear to be fused to cause abnormal growth. Besides, the donor-doping feature of Tb3+ and Ba2+ at A-sites leads to the appearance of cation vacancies for maintaining the charge balance. A certain number of defects caused by these vacancies are often accumulated near the grain borders at low energy, which leads to restricted grain motion and suppresses grain growth. Defects in the zBa-0.004Tb-KNN ceramics are mainly divided into intrinsic and non-intrinsic defects. Due to the volatilization of potassium and sodium during high-temperature sintering, intrinsic defects include cationic and anionic vacancies. On the other hand, aliovalent doping of Ba2+ and Tb3+ at the A-sites of KNN produces non-intrinsic defects. The defect equations can be obtained as follows:
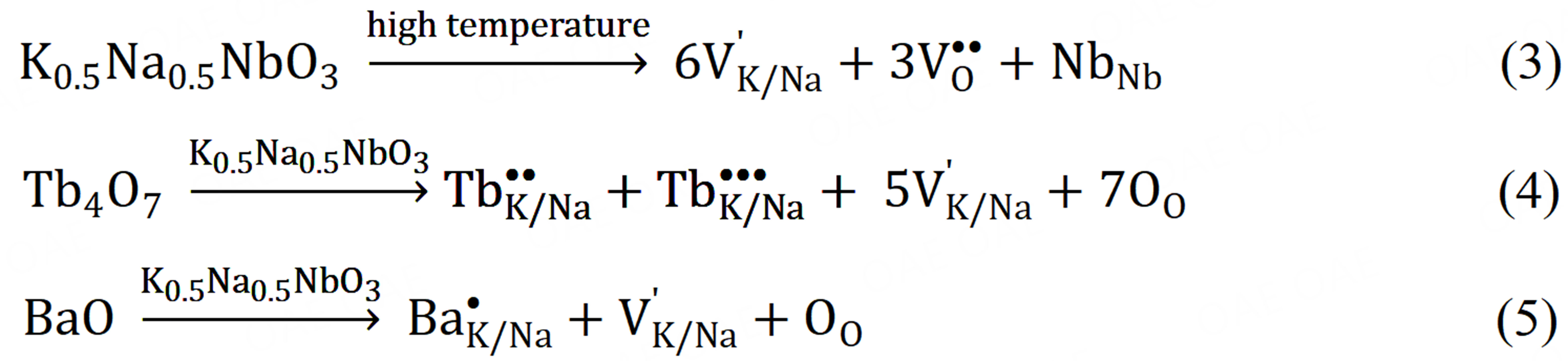
Figure 8. SEM images of the external surfaces and cross-sections (insets of A, B, C) of the zBa-0.004Tb-KNN ceramics. (A) z = 0.03; (B) z = 0.04; (C) z = 0.05, Grain size distribution and mean size of the ceramics; (D) z = 0.03; (E) z = 0.04; (F) z = 0.05. KNN: potassium-sodium niobate; ST: sintering temperature.
These point defects tend to aggregate at the grain boundaries with low energy, resulting in weakened mass transfer and effective suppression of grain growth. This indicates that the dopants possess a grain refinement effect in the KNN-based ceramics, consistent with the previous reports[1,7]. Other donor ion-doped ceramics also exhibit similar grain refinement phenomena[39,40]. Fine grains with high homogeneity are essential to achieve high optical transparency in ceramics[41]. In addition, the SEM images of 0.04Ba-0.004Tb-KNN ceramics sintered at different temperatures are shown in Supplementary Figure 4. All three ceramics possess small grain size (in the range of 90-100 nm) without an obvious difference, and the sample at ST = 1,180 ºC exhibits the best uniformity of grain size, which proves that the modulation of ST is also crucial to obtain high T%.
The P-E loops of zBa-0.004Tb-KNN ceramics are shown in Figure 9. Each ceramic sample presents a typical P-E loop and has obvious ferroelectric properties. In Figure 9A, remnant polarization (Pr) and coercive field (Ec) gradually increase with the increase of Ba content. Since at lower Ba content, smaller grain size of ceramics with grain boundaries are observed [Figure 8A and B], and the ferroelectric domains are difficult to switch near grain boundaries, resulting in lower Pr. For the 0.05Ba-0.004Tb-KNN ceramic with larger grain size [Figure 8C], high Pr (18.9 μC/cm2) and Ec (30 kV/cm) values are obtained. Besides, high-symmetry phase structures of the zBa-0.004Tb-KNN (z = 0.03, 0.04) ceramics (based on the XRD analysis from Figure 7) also induce weak ferroelectricity. Additionally, the ferroelectric properties of 0.04Ba-0.004Tb-KNN ceramics at lower STs (i.e., 1,165 and 1,180 °C) are similar, but too high STs (i.e., 1,195 °C) can deteriorate the ferroelectricity with low Pr. The piezoelectric performances of ceramics are very low (d33 ~ 3 pC/N), which should be attributed to the high-symmetry pseudo-cubic phase and small grains. Large grain size of a ceramic commonly shows good ferroelectric and piezoelectric properties, but it is not conducive to obtaining high optical transparency. ST has little effect on the ferroelectric properties of the 0.04Ba-0.004Tb-KNN ceramic but has a great impact on transparency. Hence, there is a trade-off relationship between piezoelectric/ferroelectric properties and transparency. It is expected to obtain both excellent electrical properties and high transparency by adjusting the composition and ST of KNN-based ceramics in the future.
ASSUMPTIONS AND LIMITATIONS OF CURRENT WORK
In this work, the ceramic samples involved in the experimental part were all prepared by traditional solid-state reactions, and the training data were all based on them. As a mature ceramic sintering process, this method involves several steps, including ball milling, pre-burning, granulation, pressing, PVA discharge, and sintering. These steps require multiple heat treatments at various temperatures that are closely associated with the optical transparency of KNN-based ceramics. Due to the limited availability of data, our work only considered the ST as the process parameter during data collection. With the advancement of big data technologies in material science, considering multiple process parameters (i.e., sintering atmosphere, holding time, pre-burning temperature and time, etc.) of ceramic manufacture simultaneously has the potential to further enhance the predictive accuracy of the model. According to the survey, research in this area is still lacking and ongoing.
In addition, AI4S technologies are continuously evolving. Relying solely on single-model prediction has certain limitations in this work. It remains to be further studied whether different model coupling strategies and experimental strategies based on optimized methods[30] can improve the prediction of optical T% of KNN-based ceramics.
CONCLUSIONS
In summary, we used ML to accelerate the discovery of KNN-based highly transparent ceramics. According to the data from literature and experiments, we developed a regression model with the optical T% as the target performance and constructed the mapping relationship with T% only using a minor number of elemental properties and ST as features. Ultimately, the model was applied to search a widespread space of unknown components and to identify experimental subjects. Meanwhile, we synthesized (K0.5Na0.5)0.956Tb0.004Ba0.04NbO3 with the highest T% and fine-tuned the ST to analyze the process parameter-property relationship. The highest T% occurs in the moderate ST range, and the experimental optimum ST (1,175 ~ 1,180 ºC) is highly consistent with the ML predicted results (ST = 1,180 ºC). The (K0.5Na0.5)0.956Tb0.004Ba0.04NbO3 ceramic exhibits a high T% of 78%, matching well with the ML prediction (80.1%); the transparent ceramic also has certain ferroelectric properties. Our work shows that accurate component tuning and appropriate ST act together to control the transparency of KNN-based ceramics. While traditional experiments often require multiple exploratory experiments to determine the optimal ST, the present work can determine the range of optimal ST using only computer and big data techniques, and the constructed ML model can be extended to accelerate the search process for new transparent-ferroelectric materials.
DECLARATIONS
Authors’ contributionsConceptualization and Experiments: Ma B, Yu F, Zhou P
Writing-Reviewing and Editing, Supervision: Wu X
Conceptualization, Methodology: Zhao C
Validation: Lin C
Visualization: Gao M, Lin T
Discussion, Writing, and Revision: Sa B
Availability of data and materialsThe data that support the findings of this study are available in the Supporting Information of this article and from the corresponding author upon reasonable request Supplementary Information Description
This work was supported by the National Key Research and Development Program of China (No.2022YFB3807200),the National Natural Science Foundation of China (No. 52072075,52102126, and 12104093), the Natural Science Foundation of Fujian Province (No. 2021J05122, 2021J05123,2022J01087, and 2022J01552), the Qishan Scholar Financial Support from Fuzhou University (GXRC-20099).
Conflicts of interestAll authors declared that there are no conflicts of interest.
Ethical approval and consent to participateNot applicable.
Consent for publicationNot applicable.
Copyright© The Author(s) 2023.
Supplementary MaterialsREFERENCES
1. Lin J, Ge G, Zhu K, et al. Simultaneously achieving high performance of energy storage and transparency via A-site non-stoichiometric defect engineering in KNN-based ceramics. Chem Eng J 2022;444:136538.
2. Ren X, Jin L, Peng Z, et al. Regulation of energy density and efficiency in transparent ceramics by grain refinement. Chem Eng J 2020;390:124566.
3. Lin J, Zhai J, Wu X, Ye H, Wang H. Expedient red emitting and transparency dual modulation in KNN-based transparent ceramics via sensitive photothermochromic behavior. ACS Appl Electron Mater 2021;3:1394-402.
4. Liu X, Tan P, Ma X, et al. Ferroelectric crystals with giant electro-optic property enabling ultracompact Q-switches. SCI 2022;376:371-7.
5. Wu X, Lin J, Xu Z, et al. Defect management and multi-mode optoelectronic manipulations via photo-thermochromism in smart windows. Laser Photonics Rev 2021;15:2100211.
6. Qiu C, Wang B, Zhang N, et al. Transparent ferroelectric crystals with ultrahigh piezoelectricity. Nature 2020;577:350-4.
7. Lin J, Wang P, Wang H, et al. Significantly Photo-Thermochromic KNN-Based “smart window” for sustainable optical data storage and anti-counterfeiting. Adv Opt Mater 2021;9:2100580.
8. Wang H, Lin J, Deng B, et al. Reversible multi-mode modulations of optical behavior in photochromic-translucent Nd-doped K0.5Na0.5NbO3 ceramics. J Mater Chem C 2020;8:2343-52.
9. Zhao X, Chao X, Wu D, Liang P, Yang Z. Evaluation of birefringence contribution to transparency in (1-x)KNN-xSr(Al0.5Ta0.5)O3 ceramics: a phase structure tailoring. J.Alloys Compd 2019;798:669-77.
10. Li K, Li FL, Wang Y, Kwok KW, Chan HLW. Hot-pressed K0.48Na0.52Nb1-xBixO3 (x = 0.05-0.15) lead-free ceramics for electro-optic applications. Mater Chem Phys 2011;131:320-4.
11. Xing J, Huang Y, Wu B, et al. Energy storage behavior in ErBiO3 -Doped (K,Na)NbO3 lead-free piezoelectric ceramics. ACS Appl Electron Mater 2020;2:3717-27.
12. Lin J, Jing W, Wang H, et al. Emission color-tunable and optical temperature sensing properties of Er3+/La3+ co-doped (K0.5Na0.5)NbO3 optoelectronic transparent ceramic. J.Lumin 2019;213:158-63.
13. Yang D, Ma C, Yang Z, et al. Optical and electrical properties of pressureless sintered transparent (K0.37Na0.63)NbO3-based ceramics. Ceram Int 2016;42:4648-57.
14. Zhang L, He M, Shao S. Machine learning for halide perovskite materials. Nano Energy 2020;78:105380.
15. Tao Q, Xu P, Li M, Lu W. Machine learning for perovskite materials design and discovery. npj Comput Mater 2021;7:23.
16. Mao X, Li Z, Li M, et al. Computational design and experimental validation of the optimal bimetal-doped SrCoO3-δ perovskite as solid oxide fuel cell cathode. J Am Chem Soc 2021;143:9507-14.
17. Lu T, Li M, Lu W, Zhang T. Recent progress in the data-driven discovery of novel photovoltaic materials. J Mater Inf 2022;2:7.
18. Pan Z, Zhou Y, Zhang L. Photoelectrochemical properties, machine learning, and symbolic regression for molecularly engineered halide perovskite materials in water. ACS Appl Mater Interfaces 2022;14:9933-43.
19. Weng B, Song Z, Zhu R, et al. Simple descriptor derived from symbolic regression accelerating the discovery of new perovskite catalysts. Nat Commun 2020;11:3513.
20. Yuan R, Liu Z, Balachandran PV, et al. Accelerated discovery of large electrostrains in BaTiO3-based piezoelectrics using active learning. Adv Mater 2018;30:1702884.
21. Umeda Y, Hayashi H, Moriwake H, Tanaka I. Prediction of dielectric constants using a combination of first principles calculations and machine learning. Jpn J Appl Phys 2019;58:SLLC01.
22. Liu D, Bai G, Gao C. Phase diagrams classification based on machine learning and phenomenological investigation of physical properties in K1-
23. Yang Z, Gao Z, Sun X, Cai H, Zhang F, Wu X. High critical transition temperature of lead-based perovskite ferroelectric crystals: a machine learning study. Acta Phys Sin 2019;68:210502.
24. Zhai X, Chen M, Lu W. Accelerated search for perovskite materials with higher Curie temperature based on the machine learning methods. Comput Mater Sci 2018;151:41-8.
25. Balachandran PV, Kowalski B, Sehirlioglu A, Lookman T. Experimental search for high-temperature ferroelectric perovskites guided by two-step machine learning. Nat Commun 2018;9:1668.
26. Liu Y, Yan W, Han S, et al. How machine learning predicts and explains the performance of perovskite solar cells. Solar RRL 2022;6:2101100.
27. Pilania G, Mannodi-Kanakkithodi A, Uberuaga BP, Ramprasad R, Gubernatis JE, Lookman T. Machine learning bandgaps of double perovskites. Sci Rep 2016;6:19375.
28. Qin J, Liu Z, Ma M, Li Y. Machine learning approaches for permittivity prediction and rational design of microwave dielectric ceramics. J Materiomics 2021;7:1284-93.
29. Lu S, Zhou Q, Ma L, Guo Y, Wang J. Rapid discovery of ferroelectric photovoltaic perovskites and material descriptors via machine learning. Small Methods 2019;3:1900360.
30. Yuan R, Tian Y, Xue D, et al. Accelerated search for BaTiO3-based ceramics with large energy storage at low fields using machine learning and experimental design. Adv Sci 2019;6:1901395.
31. He J, Yu C, Hou Y, et al. Accelerated discovery of high-performance piezocatalyst in BaTiO3-based ceramics via machine learning. Nano Energy 2022;97:107218.
32. Xue D, Balachandran PV, Yuan R, et al. Accelerated search for BaTiO3-based piezoelectrics with vertical morphotropic phase boundary using Bayesian learning. Proc Natl Acad Sci U S A 2016;113:13301-6.
33. Yuan R, Xue D, Xue D, et al. Knowledge-based descriptor for the compositional dependence of the phase transition in BaTiO3-based ferroelectrics. ACS Appl Mater Interfaces 2020;12:44970-80.
34. Yu F, Chi Y, Wang P, et al. Highly responsive photochromic behavior with large coloration contrast in Ba/Sm co-doped (K0.5Na0.5)NbO3 transparent ceramics. Ceram Int 2022;48:18899-908.
35. Ma Y, Yang S, Zhao C, et al. Photochromic and electric field-regulating luminescence in high-transparent (K,Na)NbO3-based ferroelectric ceramics with two-phase coexistence. ACS Appl Mater Interfaces 2022;14:35940-8.
36. Jia Q, Zhang Q, Sun H, Hao X. High transmittance and optical storage behaviors in Tb3+ doped K0.5Na0.5NbO3-based ferroelectric materials. J Eur Ceram 2021;41:1211-20.
37. Wu X, Yu F, Xiong R, et al. How to Realize ultrahigh photochromic performance for real-time optical recording in transparent ceramics. ACS Appl Mater Interfaces 2023;15:16828-41.
38. Lundberg S, Lee SI. A unified approach to interpreting model predictions. Available from: https://arxiv.org/abs/1705.07874. [Last accessed on 8 Jun 2023].
39. Gao D, Kwok KW, Lin D, Chan HLW. Microstructure and electrical properties of La-modified K0.5Na0.5NbO3 lead-free piezoelectric ceramics. J Phys D: Appl Phys 2009;42:035411.
40. Lin J, Lu Q, Wu X, et al.
Cite This Article
How to Cite
Download Citation
Export Citation File:
Type of Import
Tips on Downloading Citation
Citation Manager File Format
Type of Import
Direct Import: When the Direct Import option is selected (the default state), a dialogue box will give you the option to Save or Open the downloaded citation data. Choosing Open will either launch your citation manager or give you a choice of applications with which to use the metadata. The Save option saves the file locally for later use.
Indirect Import: When the Indirect Import option is selected, the metadata is displayed and may be copied and pasted as needed.
Comments
Comments must be written in English. Spam, offensive content, impersonation, and private information will not be permitted. If any comment is reported and identified as inappropriate content by OAE staff, the comment will be removed without notice. If you have any queries or need any help, please contact us at [email protected].