Figure2
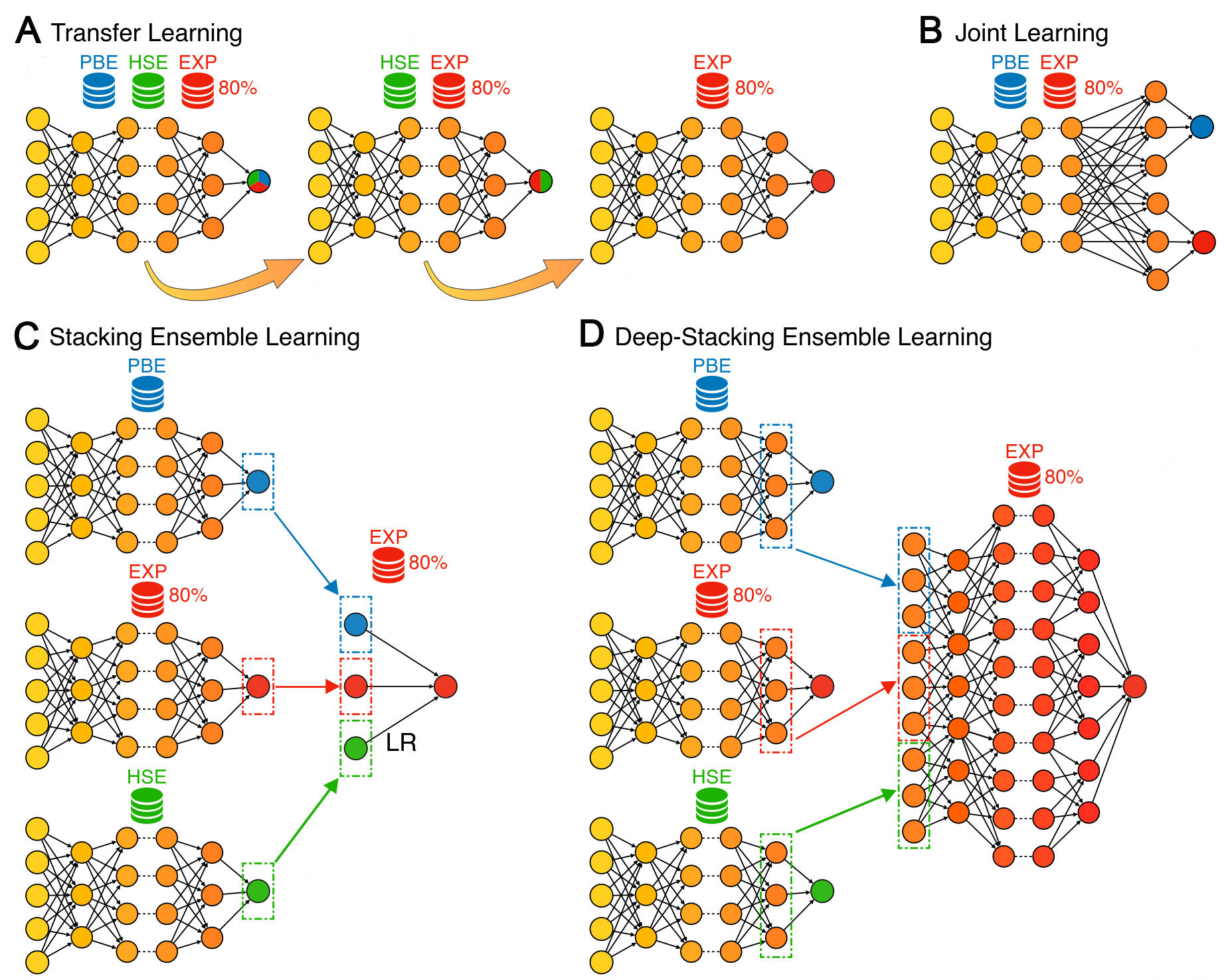
Figure 2. Schematic of the different multi-fidelity methods. (A) Transfer Learning, a model is sequentially trained on
Figure 2. Schematic of the different multi-fidelity methods. (A) Transfer Learning, a model is sequentially trained on
All published articles are preserved here permanently:
https://www.portico.org/publishers/oae/