Variation in metal concentration across tree organs and stands: implications for reference specimen development
Abstract
Air pollution is a major environmental and public health issue in urban areas. Biomonitoring using trees has been widely used to determine atmospheric pollution due to its advantages of low cost, high spatial distribution, and accumulation of a wide range of air contaminants. However, challenges exist in applying the data obtained from biomonitoring trees, including differences in pollutant concentrations in samples of the same species from different locations, and the influence of biological and environmental parameters on metal accumulation. This study aims to inform the design of biomonitoring sampling for urban air quality studies and increase their applications to exposure assessment. Specifically, the study evaluates the variation in metal concentration in tree organs of two regionally relevant tree species (Picea pungens and Populus Alba), explores the implications of different aspects of field sampling, such as variation within tree stands, tree age, and develops a reference specimen to establish threshold values for differentiating between background levels and increased exposure. The results provide insight into the complexity of using biomonitoring trees for exposure assessment and the importance of considering multiple factors in study design.
Keywords
INTRODUCTION
Exposure studies investigating the health hazard associated with urban air pollutants have found that long-term exposure and proximity to traffic emissions pose health risks, e.g., increased emergency visits reporting certain health conditions and mortality rates[1-4]. Thousands of pollutants have been identified in urban air, with hundreds emitted from traffic sources[5]. Concerns over urban air pollution have led many countries to establish policies and regulatory instruments to manage emissions and their effects.
In practice, managing and controlling urban air quality is challenging due to the complexity of point and non-point emission sources and the fate and behavior of contaminants[6,7]. The complex nature of urban air pollution requires information such as emission inventories, air modeling, exposure and risk assessment, and immission monitoring. Immission monitoring is essential; it is used to validate models and identify unknown sources[6]. Measurement of polluting substances such as NO2, SOx, PM, VOC, and trace metals is traditionally achieved through permanent monitoring stations, which are typically sparsely distributed throughout a city, mainly due to cost. Due to the heterogeneous nature of urban areas, e.g., microclimates, diversity of topography, and pollution sources, it is very challenging to obtain reliable high-resolution data to determine exposure and develop mitigation strategies[8].
Biomonitoring using trees has been widely used to determine atmospheric pollution[8-13]. The uptake of metals in trees can occur through several pathways, including absorption from soil/water by the root system, wet and/or dry deposition onto foliage and translocation via the phloem, and direct deposition through the bark to inner wood. Generally, studies that apply biomonitoring as a tool aim to either survey pollution emitters[12] or examine spatial and temporal trends[8,14-17]. The advantages of using trees include accumulating a wide range of air contaminants, high spatial distribution and availability (urban and remote location), and low cost of sampling.
However, several challenges exist when applying the data obtained from biomonitoring trees. For instance, differences in pollutant concentration in samples of the same species from different locations might not necessarily indicate variation in exposure. They may be associated with variations in biological factors (such as tree age, duration of exposure, physiological conditions, etc.) or environmental parameters (including soil composition, weather conditions, climate variations, etc.)[18,19]. To ensure the design of an appropriate sample plan to meet the need of the study objective, it is essential to explore the pattern of accumulation of metals in different organs of the same tree species and the variations in concentration among trees in tree stands.
In addition to pollution sources contributing to variation in plant metal concentrations, geographical location will also have a significant influence. The rate of metal uptake will be impacted by factors such as soil properties (pH, organic matter content, geology) and regional climate conditions (e.g., temperature, rainfall). Markert (1991)[20] introduced the concept of a reference plant, which is a plant species that is used as a standard for comparison in biomonitoring studies to determine whether the elemental concentrations in a particular plant species are elevated compared to the natural background levels or whether they are within the normal range. Markert's concept of a reference plant has been applied in various biomonitoring studies, including the European Union's Heavy Metals in European Mosses project, Canadian Reference Plant Project, and the National Bioaccumulation and Biomonitoring Program. Although the concept of a reference plant has proven helpful in biomonitoring studies, there are still some knowledge gaps and challenges, including identifying suitable reference plants in different regions and ecosystems.
This study aims to inform the design of biomonitoring sampling for urban air quality studies and increase their applications to exposure assessment. Specifically, the study will address three key objectives. Firstly, we will evaluate the variation in metal concentration in tree organs of two regionally relevant tree species (Picea pungens and Populus Alba). Secondly, we will explore the implications of different aspects of field sampling, such as variation within tree stands, tree age, and exposure. Finally, we will develop a reference specimen to establish threshold values for differentiating background levels and increased exposure.
EXPERIMENTAL
The study sites are located in the city of Calgary (N 51°00'29.45"; W 114°08'0.32"), which is the fourth largest municipality in Canada with a population of approximately 1.2 million people. The city is situated at an elevation of 1050 m (above sea level), 80km east of the Canadian Rocky Mountains at the confluence of the Bow and Elbow river. Calgary experiences strong seasonal changes in weather, with average monthly temperature ranging from -15.1 °C (January) to 22.9 °C (July), and total annual precipitation is 420 mm (http://www.statcan.gc.ca). The city has a well-defined downtown commercial area, industrial areas to the northeast and southeast and surrounding residential [Figure 1].
Figure 1. Location of sampled trees, airport, cement/asphalt plant, power plant, major traffic junction, and wastewater treatment plant in the study area (Calgary, Alberta, Canada). The inlay in the top right corner shows the position of Alberta in Canada. The inlay in the bottom left shows the additional study site on the Mount Royal University campus. ArcMap version 10.6.1 with a Datum of NAD 1983 3TM 114.
Study area and foliage sample collection
Tree stand study
To explore the influence of biological and environmental variations, we investigated the concentration and variation of trace metal within and between different tree organs in three tree stands of two species, Blue Spruce (Picea pungens) and Populars (Populus Alba). The research was conducted within the grounds of Mount Royal University (MRU) over a single day in the summer of 2018. Samples were collected from 30 trees within 6 mono species tree stands [Figure 2, Table 1]. At each tree, a section of bark (1.5’ × 3’) was collected from four cardinal directions and combined into one sample. Leaves were collected from accessible branches around the trees at each popular stand and a composite for each stand was created. At the pine tree stands, current (C) year needles and the previous year needles (C+1) were collected from all accessible whorls and combined into a composite sample. Soil samples were collected from a depth of 20-60 cm from the interior and exterior of each tree stand. The distance between trees and to the nearest roadways, stand area, and tree diameter at breast height were measured for each tree stand [Table 1].
Figure 2. Map of Mount Royal University campus showing the location of the 6 mono species tree stands. ArcMap version 10.6.1 with a Datum of WGS 1984.
Characteristic of tree stand and distance from the nearest roadways
Stand Number | Tree Species | Area of Stand (m2) | Distance between trees in stand (m) | Distance to roadway (m) (cardinal direction) | Average Diameter Breast Height (mm) | Bark Bulk Density (g/cm3) |
1 | Pine | 60 | 2.8 (± 0.4) | 54 (N), 27.5 (S), 20.4 (E) | 131 (± 10) | 0.73 (± 0.24) |
2 | Pine | 29 | 4.1 (± 1.2) | 31.1 (N) | 125 (± 19) | 0.47 (± 0.07) |
3 | Pine | 45 | 4.2 (± 1.1) | 38.5 (N), 12.6 (S), 9.9 (W) | 134 (± 29) | 0.50 (± 0.05) |
4 | Popular | 111 | 7.0 (± 1.6) | 25.4 (N), 7.6 (E) | 69 (± 9) | 0.70 (± 0.16) |
5 | Popular | 126 | 8.0 (± 1.9) | 30.8 (N), 8.6 (S) | 88 (± 10) | 0.65 (± 0.06) |
6 | Popular | 52 | 5.2 (± 1.6) | 18.3 (E) | 104 (± 18) | 0.54 (± 0.07) |
Reference tree study
For the development of a ‘reference specimen’, samples were collected from 150 trees at 70 discrete sampling sites [Figure 1] during the summer of 2017 and 2019. As coniferous trees are the primary focus of the majority of higher plant biomonitoring studies and are commonly found in Calgary, these were chosen for the development of a reference specimen. A 1.5’× 3’ section of bark was collected from the western side (prevailing wind direction) of each tree. A composite sample of C and C + 1 needles was collected from each tree. The sampling sites within the city were primarily elementary schools, as the majority of communities in the city have at least one elementary school with green spaces planted with pine trees.
Analytical methods
The foliage was oven dried at 100 °C for 24 h and moisture content was determined by loss of weight. The bulk density of bark samples was determined by calculating the ratio of the oven-dry mass divided by the mass of water displaced by its green volume.
To determine trace metal composition in tree foliage sample, 2 g of each oven-dried sample was ashed in a muffle furnace (6 °C per minute to 500 °C, held for 16 h). Ashed samples were digested using 1 mL of Optima Ultra-pure nitric acid, and 3 drops of 30 % hydrogen peroxide were added as a flocculent. Samples were filtered using a 0.45 µm disk filter and diluted using 18 Ω Milli-Q water. Soil samples were prepared in accordance with EPA method 3,050b.
The concentration of beryllium (Be), sodium (Na), magnesium (mg), aluminum (Al), potassium (K), calcium (Ca), vanadium (V), chromium (Cr), manganese (Mn), iron (Fe), cobalt (Co), nickle (Ni), copper (Cu), Zinc (Zn), arsenic (As), selenium (Se), molybdenum (Mo), silver (Ag), cadmium (Cd), Antimony (Sb), barium (Ba), thallium (TI), lead (Pb), thorium (U), and uranium (U) were measured by inductively coupled mass spectrometry (ICP-MS) (Thermo Scientific X Series 2). Concentrations were determined using a ten-point calibration curve of aqueous standard solutions (Agilent Technologies Environmental Calibration Standard) in nitric acid using Rh as an internal standard. The method detection limit (MDL) was determined by extrapolating the calibration curve to the point where the signal reaches three times the standard deviation of the extraction blanks [Supplementary Table 1]. All concentrations were reported on a dry weight (d.w.) basis. For each ten[10] samples, a nitric acid blank, and a 1.5 ppb and 25 ppb checks were analyzed to control any possible contamination or drift. Each sample was analyzed six[6] times, with a 30-second pre-uptake and 45-second wash between samples. Method validation and accuracy were checked by replicate analysis of a certified reference material (CRM) for pine needles (High Purity Standards CRM-PN-A). A comparison of the target and analyzed values may be found in [Supplementary Table 1] where it can be seen that the average recovery is 82 % (± 25 %).
Statistical analysis
ICP-MS data output was mass and dilution corrected. Metals that were not found to be present above MDL for the majority of sampled sites were removed from further analysis. Additionally, during the MRU field study, major plant nutrients and metals that were not linked to pollution sources were removed. Outlier testing was conducted using a Grubbs’ test; this data was excluded in the creation of the reference tree but carried forwards in all other respects. Log normalized results were preliminarily analyzed (mean, std, dev, variance, max/min), and single factor ANOVA coupled with a Tukey HSD test (Excel/MegaStat/astatsa.com) was employed to determine the significance of differences between the metal loadings of tree components, within a stand and between stands. Cluster analysis and Principal Component Analysis were completed (JMP) and used to further identify metal distribution patterns.
In the establishment of the reference tree, needle and bark data from all trees and their respective metal loadings, less those found to be below MDL or determined to be outliers via the Grubbs’ test, were averaged. Conditional formatting (Excel) was employed to initially identify trends in the data. The data sets were divided into Tree Nutrients and Trace Metals subsections and compared to Markert’s (1992)[20] Reference Plant metal loadings. A Student’s t-test was then employed to compare individual trees against the reference tree components that were established.
RESULTS AND DISCUSSION
Tree stand study - variation amongst tree organs & within tree stand
Table 2 provides a summary of the average concentration and standard deviation of metals in the soil and various tree organs across six stands. The soil has the highest concentration of almost all metals, followed by bark, two-year needles, leaves, and one-year needles. However, the poplar leaves have the highest Mg and K concentrations. Major tree nutrients such as Mg, K, and Ca are the most abundant metals present and are likely actively drawn into the tree from crustal sources via the root system. The transport of metals from the soil into the tree largely depends on soil chemistry, with more acidic soil conditions typically making more metals biologically available to the plant.
Mean elemental composition of tree organs (bark, needle, leaf) and soil for stand study in mg kg-1 dry matter
Element (mg kg-1) | Pine bark | Popular bark | Popular leaf | Pine needle Year 1 | Pine needle Year 2 | Pine soil | Popular soil |
Mg | 434 ± 77 | 663 ± 325 | 2,854 ± 1,067 | 370 ± 55 | 442 ± 92 | 1,217 ± 825 | 1,940 ± 1,022 |
K | 1,130 ± 266 | 2,062 ± 1,523 | 6,263 ± 1,685 | 4,024 ± 491 | 1,504 ± 407 | 1,203 ± 91 | 1,447 ± 171 |
Ca | 6,259 ± 1,198 | 10,171 ± 2,812 | 10,911 ± 4,037 | 2,207 ± 771 | 10,134 ± 2,383 | 19,677 ± 6931 | 22,542 ± 7,455 |
Cr | 0.5 ± 0.2 | 0.3± 0.1 | 0.03 ± 0.01 | 0.1 ± 0.02 | 0.1 ± 0.03 | 4.8 ± 1.4 | 6.1 ± 1.2 |
Co | 0.10 ± 0.01 | 0.10 ± 0.04 | 0.3 ± 0.2 | 0.009 ± 0.002 | 0.020 ± 0.005 | 0.6 ± 0.3 | 1.3 ± 0.5 |
Ni | 0.4 ±0.1 | 0.5 ± 0.2 | 0.7 ± 0.3 | 0.10 ± 0.03 | 0.3 ± 0.1 | 2.4 ± 1.1 | 4.1 ± 1.6 |
Cu | 2.8 ± 0.4 | 2.9 ± 0.9 | 2.0 ± 0.6 | 0.9 ± 0.1 | 0.4 ± 0.2 | 1.5 ± 0.2 | 1.5 ± 0.4 |
Pb | 1.4 ± 0.9 | 0.5 ± 0.3 | 0.020 ± 0.008 | 0.010 ± 0.007 | 0.10 ± 0.03 | 6.6 ± 1.2 | 8.0 ± 1.8 |
Copper was found to be elevated above soil levels in the bark of both tree species. Uptake from the soil is expected, plant uptake factors for Cu in pines range from 0.2 to 0.6[21], and while the values are higher than soil, they are well within the normal range (5-30 mg kg-1) for plants[22]. The accumulation of Cu may be due to contaminants being directly deposited on the bark. Unlike leaves and needles, the bark is not shed in the same manner and is typically better sheltered from weather events by the tree's canopy, preventing contaminants from being washed away. As a result, the bark can indicate accumulation over a more extended period of time.
Using a one-way ANOVA and Tukey HSD, it was determined that there was no significant difference between an individual tree organ and the same organ of any tree with the same stand. For example, each of the tree's one-year needles has approximately the same metal composition and concentration as each of the five trees in the stand. This is applied for bark, needles, and leaves. Between stands of the same species, there was also an insignificant difference for the same tree organ.
To explore the variation amongst tree organs, principal component analysis was completed using the five traffic-related metals – Co, Cr, Cu, Ni, and Pb [Figure 3]. The first two principal components (PC) explain just over 85% of the variance in the data set. Samples were grouped based on the type of tree organ. Separation of groups on PC1 occurs due to Cr, Pb, Ni, and Co, with concentrations increasing from right to left. By contrast, separation on PC2 is driven by the concentration of Cu, which increases as you move from the bottom to the top of the plot. Using one-way ANOVA and Tukey HSD test, significant differences between tree organs and soils were observed [Table 3]. Exceptions include bark, where there was no significant difference between the two-tree species across all the stands for Co, Cu, and Ni. In addition, there was no significant difference between Ni concentrations in bark, C + 1 needles, and popular leaves.
Figure 3. Principal component analysis using concentrations of Co, Cr, Cu, Ni, and Pb in tree organs – soils ( ,
), bark (
,
), popular leaves (
), and pine needles (
,
).
Comparison of individual elements across tree organs using Tukey HSD test.
Treatment Pair | Co | Ni | Cu | Pb | Cr |
Pop Bark vs. Pine Bark | 0.900 | 0.900 | 0.900 | 0.001 | 0.001 |
Pop Bark vs. Soil | 0.001 | 0.001 | 0.002 | 0.001 | 0.001 |
Pop. Bark vs. 2YNeedle | 0.001 | 0.200 | 0.001 | 0.001 | 0.001 |
Pop. Bark vs. 1YNeedle | 0.001 | 0.001 | 0.001 | 0.001 | 0.001 |
Pop Bark vs. Leaves | 0.001 | 0.190 | 0.072 | 0.001 | 0.001 |
Pine Bark vs. Soil | 0.001 | 0.001 | 0.001 | 0.001 | 0.001 |
Pine Bark vs. 2YNeedle | 0.001 | 0.476 | 0.001 | 0.001 | 0.001 |
Pine Bark vs. 1YNeedle | 0.001 | 0.001 | 0.001 | 0.001 | 0.001 |
Pine Bark vs. Pop Leaves | 0.001 | 0.079 | 0.042 | 0.001 | 0.001 |
Soil vs. 2YNeedle | 0.001 | 0.001 | 0.001 | 0.001 | 0.001 |
Soil vs. 1YNeedle | 0.001 | 0.001 | 0.012 | 0.001 | 0.001 |
Soil vs. Pop Leaves | 0.001 | 0.001 | 0.690 | 0.001 | 0.001 |
2YNeedle vs. 1YNeedle | 0.001 | 0.001 | 0.001 | 0.001 | 0.126 |
2YNeedle vs. Pop Leaves | 0.001 | 0.001 | 0.001 | 0.001 | 0.001 |
1YNeedle vs. Pop Leaves | 0.001 | 0.001 | 0.001 | 0.090 | 0.001 |
The variation in concentrations and profiles of metals between organs depends on multiple factors, including physiological functions, chemical composition variations, and environmental conditions. Factors such as the age, size, and surface area of the organs, as well as the solubility and reactivity of the metals in the air, can influence the uptake rate. Bark and needles have the potential to take up metals either through direct contact with the atmosphere or via uptake from the xylem, which transports water and minerals throughout the tree. However, the efficiency of uptake may vary depending on the thickness and composition of the bark or needles, as well as the chemical properties of the metals[21]. Additionally, the age of a tree organ can also play a role in metal uptake, as older organs might have different absorption capacities compared to younger ones, further contributing to the overall variation observed.
These research findings informed our collection of samples for developing a reference specimen. The lack of significant difference between trees in a well-defined stand means we could sample a wider area without the need to sample each tree. Importantly, the results indicate the need to develop reference information for each organ type, e.g., bark and needles, to ensure the appropriate use of the reference data as a comparison.
Reference tree study
One hundred fifty samples were collected from 70 discreet sampling points across Calgary [Figure 1]. Data from the analysis of bark and needles were screened, and individual metal outliers were removed using a Grubbs test. The remaining values were averaged for each organ type (Picea-Bark and Picea- needles) and are presented in Table 4, along with values from Markert's (1992) reference plant. Setting the reference plant to zero, values for macro & minor and trace elements within bark and needles were plotted as deviations from the Markert reference plant [ Supplementary Figures 1-4].
Element content of reference Picea- Needles and Picea- Bark in relation to Markert’s reference plant specimen
Element (Mass Number) | Concentration (mg kg-1) | ||
Picea- Needles | Picea- Bark | Markert 1992 [20] | |
Beryllium (9) | 0.001 | 0.01 | 0.001 |
Sodium (23) | 435 | 463 | 150 |
Magnesium (24) | 605 | 692 | 200 |
Aluminum (27) | 33 | 272 | 80 |
Potassium (39) | 1,900 | 1,480 | 1900 |
Calcium (44) | 6,526 | 9,547 | 1000 |
Vanadium (51) | 0.08 | 0.7 | 0.5 |
Chromium (52) | 0.10 | 0.5 | 1.5 |
Manganese (55) | 20 | 42 | 200 |
Iron (56) | 50 | 295 | 150 |
Cobalt (59) | 0.0 | 0.2 | 0.2 |
Nickle (60) | 0.3 | 0.7 | 1.5 |
Copper (65) | 0.7 | 5.3 | 10 |
Zinc (66) | 24 | 48 | 50 |
Arsenic (75) | 0.045 | 0.14 | 0.1 |
Selenium (82) | 0.009 | 0.01 | 0.02 |
Molybdenum (95) | 0.087 | 0.14 | 0.5 |
Silver (107) | 0.005 | 0.01 | 0.2 |
Cadmium (111) | 0.003 | 0.04 | 0.05 |
Antimony (121) | 0.018 | 0.05 | 0.1 |
Barium (137) | 31 | 48 | 40 |
Thallium (205) | 0.0002 | 0.003 | 0.05 |
Lead (208) | 0.073 | 1.64 | 1 |
Thorium (232) | 0.010 | 0.08 | 0.005 |
Uranium (238) | 0.008 | 0.03 | 0.01 |
From these elemental signatures, it is evident that many of the element values are substantially different. For example, within the major and minor nutrient elements, such as Ca, Mg, and Na, Picea-Bark had three times higher values than Markert's reference plant, while Cu, Ni, and Zn were about half that of Markert's reference plant [Supplementary Figure 1]. When comparing trace elements, Picea-Bark had values ten times higher for Be, 1.5 times higher for Pb, and three times higher for U, while Markert's reference plant had three times higher values for Cr, Mo, and Ag [Supplementary Figure 2]. The Picea-Needles profile was slightly different, still higher in Ca, Mg, and Na but depleted in Fe and similar to Markert's for K. The difference between Picea-Bark and Needle is expected, as different tree components are involved in varying processes to bring in both micro and macro nutrients[23,24]. Differences would be expected between Picea and Markert reference plants, as they are from entirely different geological areas. In addition, Markert describes an entire plant (root and shoot system) as opposed to an organ of a plant. Interestingly, when the bark and needle values are averaged, almost all the metals move closer to Markert's values.
The application of the Picea reference data can be seen when exploring the broader sample set with statistical analysis. Principal component analysis (PCA) was conducted using the broader data, and the reference data for each tree organ fell in proximity to the majority of the samples of that organ
This highlights the importance of using reference data as a baseline to identify and address the sources and types of pollutants affecting a particular area. It provides a benchmark to determine the extent to which an area is more impacted relative to an urban background.
The data can also serve as indicators of the overall health of local ecosystems. By comparing the health and abundance of the trees in a polluted area to the reference data, we can gain insights into the extent of damage to local ecosystems. This could act as an early warning of environmental problems and help implement measures to prevent or mitigate the impacts of pollution before it becomes too severe. Reference values are dynamic, meaning that additional data can be successively added to refine them over time. Changes to the urban landscape, fluctuations in industrial activity, shifts to electric vehicles, and climate change all have implications on urban air quality. Reference data can be used to track these changes over time, evaluate the risks, and assess the exposure for our urban population.
CONCLUSIONS
Air pollution is a pressing concern for both the environment and public health in urban areas. Trees have proven to be effective biomonitoring tools due to their wide distribution, cost-effectiveness, and ability to accumulate various air contaminants. However, challenges arise when using data obtained from biomonitoring trees, including variations in pollutant concentrations among samples of the same species from different locations, as well as the influence of biological and environmental factors on metal accumulation. This study aims to inform the design of biomonitoring sampling for urban air quality studies and expand their applications in exposure assessment.
The study evaluates the variation in metal concentration within tree organs of two relevant tree species, Picea pungens and Populus Alba, while exploring different aspects of field sampling, such as variations within tree stands and tree age. Furthermore, the research establishes a reference specimen to differentiate between background levels and increased exposure, setting threshold values. The findings highlight the complexity of using biomonitoring trees for exposure assessment and emphasize the importance of considering multiple factors in study design.
In conclusion, this study uncovers variations in metal uptake across different tree organs, influenced by physiological functions and chemical composition. It reveals that sampling wider areas within well-defined tree stands can be sufficient without individual tree sampling. Additionally, the development of elemental reference signatures provides a benchmark for biomonitoring using Picea species, applicable not only in Calgary but also in other urban environments. These insights contribute to the understanding and improvement of biomonitoring sampling methods for urban air quality studies, ultimately aiding in the assessment of exposure levels and the formulation of effective mitigation strategies.
DECLARATIONS
Authors’ contributionsMade substantial contributions to the conception and design of the study, performed data analysis and interpretation, and constructed the manuscript: O’Sullivan G, Hayes K
Performed data acquisition, as well as provided administrative, technical, and material support: Hart E, Ursino A
Availability of data and materialsNot applicable.
Financial support and sponsorshipThis work was supported by MRU’s Faculty of Science & Technology Innovation grant.
Conflicts of interestAll authors declared that there are no conflicts of interest.
Ethical approval and consent to participateNot applicable.
Consent for publicationNot applicable.
Copyright© The Author(s) 2023.
Supplementary MaterialsREFERENCES
1. Buckeridge DL, Glazier R, Harvey BJ, Escobar M, Amrhein C, Frank J. Effect of motor vehicle emissions on respiratory health in an urban area. Environ Health Perspect 2002;110:293-300.
2. Crouse DL, Peters PA, van Donkelaar A, et al. Risk of nonaccidental and cardiovascular mortality in relation to long-term exposure to low concentrations of fine particulate matter: a Canadian national-level cohort study. Environ Health Perspect 2012;120:708-14.
3. Gan WQ, Tamburic L, Davies HW, Demers PA, Koehoorn M, Brauer M. Changes in residential proximity to road traffic and the risk of death from coronary heart disease. Epidemiology 2010;21:642-9.
4. Smargiassi A, Kosatsky T, Hicks J, et al. Risk of asthmatic episodes in children exposed to sulfur dioxide stack emissions from a refinery point source in Montreal, Canada. Environ Health Perspect 2009;117:653-9.
5. Cohen AJ, Ross Anderson H, Ostro B, et al. The global burden of disease due to outdoor air pollution. J Toxicol Environ Health A 2005;68:1301-7.
6. Wolterbeek B. Biomonitoring of trace element air pollution: principles, possibilities and perspectives. Environ Pollut 2002;120:11-21.
7. Gulia S, Shiva Nagendra S, Khare M, Khanna I. Urban air quality management-a review. Atmospheric Pollution Research 2015;6:286-304.
8. Brignole D, Drava G, Minganti V, et al. Chemical and magnetic analyses on tree bark as an effective tool for biomonitoring: a case study in Lisbon (Portugal). Chemosphere 2018;195:508-14.
9. Hatami-Manesh M, Mortazavi S, Solgi E, Mohtadi A. Assessing the uptake and accumulation of heavy metals and particulate matter from ambient air by some tree species in Isfahan Metropolis, Iran. Environ Sci Pollut Res Int 2021;28:41451-63.
10. Salmani-ghabeshi S, Fadic-ruiz X, Miró-rodríguez C, Pinilla-gil E, Cereceda-balic F. Trace element levels in native plant species around the industrial site of puchuncaví-ventanas (central chile): evaluation of the phytoremediation potential. Appl Sci 2021;11:713.
11. Subpiramaniyam S. Portulaca oleracea L. for phytoremediation and biomonitoring in metal-contaminated environments. Chemosphere 2021;280:130784.
12. Pająk M, Halecki W, Gąsiorek M. Accumulative response of scots pine (Pinus sylvestris L.) and silver birch (Betula pendula Roth) to heavy metals enhanced by Pb-Zn ore mining and processing plants: Explicitly spatial considerations of ordinary kriging based on a GIS approach. Chemosphere 2017;168:851-9.
13. Rea-Downing G, Quirk BJ, Wagner CL, Lippert PC. Evergreen needle magnetization as a proxy for particulate matter pollution in urban environments. Geohealth 2020;4:e2020GH000286.
14. Moreira TCL, Amato-lourenço LF, da Silva GT, et al. The Use of tree barks to monitor traffic related air pollution: a case study in São Paulo-Brazil. Front Environ Sci 2018;6:72.
15. Drava G, Brignole D, Giordani P, Minganti V. The bark of the branches of holm oak (Quercus ilex L.) for a retrospective study of trace elements in the atmosphere. Environ Res 2017;154:291-5.
16. Kosiorek M, Modrzewska B, Wyszkowski M. Levels of selected trace elements in Scots pine (Pinus sylvestris L.), silver birch (Betula pendula L.), and Norway maple (Acer platanoides L.) in an urbanized environment. Environ Monit Assess 2016;188:598.
17. Kara M, Dumanoglu Y, Altiok H, Elbir T, Odabasi M, Bayram A. Seasonal and spatial variations of atmospheric trace elemental deposition in the Aliaga industrial region, Turkey. Atmospheric Research 2014;149:204-16.
18. Tarricone K, Wagner G, Klein R. Toward standardization of sample collection and preservation for the quality of results in biomonitoring with trees - a critical review. Ecological Indicators 2015;57:341-59.
19. Alaqouri HAA, Genc CO, Aricak B, Kuzmina N, Menshikov S, Cetin M. The possibility of using Scots pine needles as biomonitor in determination of heavy metal accumulation. Environ Sci Pollut Res Int 2020;27:20273-80.
20. Markert B. Establishing of ‘Reference Plant’ for inorganic characterization of different plant species by chemical fingerprinting. Water Air Soil Pollut 1992;64:533-8.
21. Jonczak J, Sut-lohmann M, Polláková N, Parzych A, Šimanský V, Donovan S. Bioaccumulation of potentially toxic elements by the needles of eleven pine species in low polluted area. Water Air Soil Pollut 2021;232:28.
22. Ariyakanon N, Winaipanich B. Phytoremediation of Copper Contaminated Soil by Brassica juncea (L.) Czern and Bidens alba (L.) DC. var. radiata. Available from: https://www.thaiscience.info/journals/Article/CJSR/10324263.pdf [Last accessed on 29 Jun 2023].
23. Odabasi M, Dumanoglu Y, Ozgunerge Falay E, et al. Investigation of spatial distributions and sources of persistent organic pollutants (POPs) in a heavily polluted industrial region using tree components. Chemosphere 2016;160:114-25.
Cite This Article
Export citation file: BibTeX | RIS
OAE Style
O’Sullivan G, Hayes K, Ursino A, Hart E. Variation in metal concentration across tree organs and stands: implications for reference specimen development. J Environ Expo Assess 2023;2:14. http://dx.doi.org/10.20517/jeea.2023.09
AMA Style
O’Sullivan G, Hayes K, Ursino A, Hart E. Variation in metal concentration across tree organs and stands: implications for reference specimen development. Journal of Environmental Exposure Assessment. 2023; 2(3): 14. http://dx.doi.org/10.20517/jeea.2023.09
Chicago/Turabian Style
O’Sullivan, Gwen, Kevin Hayes, Alex Ursino, Emily Hart. 2023. "Variation in metal concentration across tree organs and stands: implications for reference specimen development" Journal of Environmental Exposure Assessment. 2, no.3: 14. http://dx.doi.org/10.20517/jeea.2023.09
ACS Style
O’Sullivan, G.; Hayes K.; Ursino A.; Hart E. Variation in metal concentration across tree organs and stands: implications for reference specimen development. J. Environ. Expo. Assess. 2023, 2, 14. http://dx.doi.org/10.20517/jeea.2023.09
About This Article
Special Issue
Copyright
Data & Comments
Data
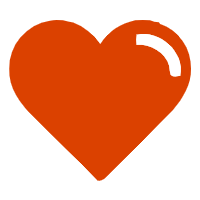

Comments
Comments must be written in English. Spam, offensive content, impersonation, and private information will not be permitted. If any comment is reported and identified as inappropriate content by OAE staff, the comment will be removed without notice. If you have any queries or need any help, please contact us at support@oaepublish.com.