Machine learning-assisted advances and perspectives for electrolytes of protonic solid oxide fuel cells
Abstract
Protonic solid oxide fuel cells (P-SOFCs), as a promising power generation technology, have garnered increasing attention due to their advantages of cleanliness, high efficiency, and high reliability. As a critical component of
Keywords
INTRODUCTION
Global emission of carbon caused by the extensive utilization of traditional fossil fuel has posed an unprecedented challenge to the ecological environment, prompting countries around the world to accelerate the search for clean, efficient, sustainable, and renewable energy alternatives. Various new energy technologies, such as solar photovoltaic cells, wind power generation, and fuel cells, have emerged accordingly[1-3]. Among them, solar photovoltaic cells have the advantages of clean, renewable, and low maintenance costs. However, they are limited by weather conditions and their energy conversion efficiencies need to be improved[4,5]. Although the wind power generation technology is mature, it has requirements for geographical locations and may have impacts on the ecological environment[6,7]. Hydroelectric power generation is stable and schedulable, but it has requirements for water resources and may affect river ecosystems[8]. Geothermal energy technology is stable and schedulable, but it is restricted by the high initial investment cost and the potential impacts on ecosystems and natural resources[9]. Fuel cells can directly convert chemical energy into electrical energy through electrochemical reactions. Since the process does not involve combustion, it has the advantages of high efficiency, low emissions, and high flexibility[10].
At present, the typical fuel cells include proton exchange membrane fuel cells (PEMFCs), alkaline fuel cells (AFCs), phosphoric acid fuel cells (PAFCs), solid oxide fuel cells (SOFCs), etc., as summarized in
Figure 1. Schematic diagram of (A) PEMFC, reproduced with permission from Tellez-Cruz et al.[11] Copyright 2021 MDPI; (B) AFC, reproduced with permission from Das et al.[12] Copyright 2022 MDPI; (C) PAFC, reproduced with permission from Petrovic et al.[13] Copyright 2020 IEEE; (D) SOFC and (E) P-SOFC, reproduced with permission from Jang et al.[14] Copyright 2024 American Chemical Society. PEMFC: Proton exchange membrane fuel cell; AFC: alkaline fuel cell; PAFC: phosphoric acid fuel cell; P-SOFC: Protonic solid oxide fuel cell.
Protonic SOFCs (P-SOFCs), as a new generation of SOFCs, have become a focus of attention in recent years and are considered as one of the most promising hydrogen utilization technologies. Among the components of P-SOFCs, the electrolyte directly determines the overall efficiency of the cell and the widely used electrolyte materials are limited to the BaZr1-x-yCexMyO3 (BZCM, M = Y, Yb, etc.) systems[3,19], whose proton conductivity at low temperature (≤ 500 °C) is far from meeting the requirements of operation performance. Therefore, rapidly developing excellent electrolyte materials has become the core issue in achieving the iterative innovation of P-SOFCs. Traditional material development methods mainly rely on trial-and-error experiments, which are not only time-consuming and costly, but also make it difficult to systematically understand the structure-property relationships of materials. With the advancement of artificial intelligence (AI) technology and the continuous improvement of materials databases, machine learning (ML) has demonstrated unique advantages and enormous potential in material development. The advantages of
ML-assisted P-SOFC electrolyte material development has made significant progress, including
OVERVIEW OF ELECTROLYTES
As the most important component of P-SOFCs, the primary function of the electrolyte is to facilitate proton transport while blocking electron transfer and gas molecule permeation. In order to realize the possible applications of such electrochemical devices in energy conversion and storage, the electrolyte must meet some stringent requirements, especially in terms of proton conductivity and stability. Therefore, in this chapter, an overview of the efforts that have been devoted to protonic electrolyte materials is provided.
Operating principles and requirements of electrolytes
In P-SOFCs, hydrogen gas is first oxidized at the fuel electrode to produce protons, and then, the protons are driven by concentration gradients to diffuse through the dense electrolyte to the air electrode. Some protons can also be produced by hydration reaction [Figure 2A-C] in wet hydrogen fuel. At the air electrode, these protons react with oxygen ions generated by oxygen reduction reaction (ORR) to produce water[25-29]. Simultaneously, the electrons flow through the external circuit to form a loop, thus completing the entire cell operation. The operating principle of proton-conducting electrolytes is based on the conduction processes of protons in solid oxides [Figure 2D and E], which are mainly divided into two categories: One is the Vehicle mechanism considering the proton defect (OH-) formed through the combination of proton with lattice oxygen and then transport by the diffusion of oxygen vacancies
Figure 2. Schematic illustration of proton defect formation and diffusion in perovskite-type structure, reproduced with permission from Seong et al.[35] Copyright 2021 John Wiley & Sons.
Electrolyte materials
ABO3 perovskite-type electrolytes
Iwahara discovered the proton conductivity in ABO3 perovskite-type oxides and elucidated the possibility of this characteristic in energy applications in the early 1980s and 1990s[36-41], which has a profound impact on the development of P-SOFC electrolytes. The most typically protonic ABO3 perovskite electrolytes are obtained by various dopants at different sites as shown in Figure 3A[42]. Cerate- and zirconate-based oxides (BaCeO3 and BaZrO3) have been intensively studied by such doping approaches due to their excellent proton conductivity[43]. As summarized in Figure 3B, BaCeO3 oxides with B-site dopants of Y, Er, or Gd exhibit high proton conductivity. However, the increase of the doping ratio may lead to the formation of impurity phases due to the solid solution boundary, which degrade the proton conductivity. BaCeO3-based oxides generally possess favorable sintering properties, allowing for the achievement of larger grain sizes at relatively low sintering temperatures, thereby reducing grain boundary resistance. Nevertheless, due to the intrinsic characteristics of these materials, they are highly susceptible and can react with CO2 or H2O to form BaCO3 or Ba (OH)2[44]. Therefore, more efforts are still needed to develop stable electrolyte materials. Doped BaZrO3 oxides exhibit exceptional chemical stability in the aforementioned environments, and the Y-doped BaZrO3 electrolyte thin films demonstrate superior proton conductivity [Figure 3C]. However, their high sintering temperatures complicate the subsequent preparation of P-SOFC and result in higher grain boundary resistance. Furthermore, BaZrO3-based oxides are prone to oxidation reaction that introduces holes, leading to an electronic leakage phenomenon, which deteriorates the efficiencies of the devices. Therefore, the BaZrO3 and BaCeO3 solid solution has become the most extensively studied oxide. By adjusting the Zr-Ce ratio and the dopant elements, a series of excellent electrolyte materials have been developed, exhibiting exceptionally superior electrochemical performance for P-SOFC. As shown in Figure 3D, Y and Yb co-doped BaZr0.1Ce0.7Y0.1Yb0.1O3-δ (BZCYYb1711) possesses extremely high proton conductivity of ~1.35 × 10-2 S cm-1 at 500 °C[45]. However, according to the report of Duan et al., BZCYYb1711 still decomposed under 30 vol% CO2 + 30 vol% H2O + 40 vol% Ar for 100 h at 500 °C[46]. Subsequently, Choi et al. proposed the BaZr0.4Ce0.4Y0.1Yb0.1O3-δ (BZCYYb4411) electrolyte by increasing the Zr content. The X-ray diffraction (XRD) patterns before and after exposure to 100% CO2 for 100 h at 500 °C confirmed the outstanding stability of BZCYYb4411 [Figure 3E], and the material maintained high proton conductivity of ~2.02 × 10-2 S cm-1 at 500 °C under wet N2[47]. Recently, Saito et al. reported BaSc0.8Mo0.2O2.8 oxide with high proton conductivity of 1 × 10-2 S cm-1 at 320 °C and excellent chemical stability under reducing, oxidizing and CO2 atmospheres. This also implies that the design of proton conductors should not be limited to traditional materials, necessitating the exploration of new dopant ions and structures[48].
Figure 3. ABO3 perovskite-type electrolytes. (A) Possible element doping strategies of ABO3 perovskite electrolytes, reproduced with permission from Tarasova et al.[42] Copyright 2024 Elsevier. Conductivity of doped; (B) BaCeO3, (C) BaZrO3, and (D) Ba(Ce, Zr)O3 electrolytes as a functional of temperature, reproduced with permission from Matkin et al.[43] Copyright 2024 Royal Society of Chemistry; (E) XRD patterns of BZCYYb4411 before and after exposure to 100% CO2 at 500 °C, reproduced with permission from Choi et al.[47] Copyright 2018 Springer Nature. XRD: X-ray diffraction.
Electrolytes with other structures
In addition to the ABO3 perovskite-type electrolytes, the proton conductivities of many other materials with different structures have also been studied, such as oxides with An+1BnO3n+1 Ruddlesden-Popper structure, hexagonal perovskites with mixed hexagonal and cubic-packing layers, A2B2O5 Brownmillerite structure, ReMO4 structure, A2B2O7 structure and A10-xB6O26±δ apatite-type structure, as shown in Figure 4[49]. The total conductivity of some typical proton conductors at 500 °C was summarized in Figure 5[45,47,50-57]. However, the proton conductivities of these materials are still lower than 10-3 S cm-1 and the proton transfer number of some materials is also relatively low. For example, Tarasova et al. prepared a BaLaInO4 Ruddlesden-Popper structure oxide and characterized its conductivity in dry and humid to be ~2 × 10-7 and ~7 × 10-7 S cm-1 near 500 °C, respectively. The calculated proton transfer number is only 0.7[50], which is lower than that of BZCM-based electrolytes. Ba5Er2Al2ZrO13 oxide was reported as a new class of proton conductor, which consists of triple-layer cubic perovskite blocks that are separated by an oxygen-deficient hexagonal BaO layer[51]. The proton conductivity of Ba5Er2Al2ZrO13 can reach ~3 × 10-3 S cm-1 at 500 °C and the proton transfer number is about 1. La0.99Ca0.01NbO4 and La1.95Ca0.05Zr2O7-δ with ReMO4 and A2B2O7 structures also have ideal proton transfer number, but the proton conductivity is limited to 10-4 S cm-1 at 500 °C[52]. The proton conductivities of these materials have not reached the ideal level; their performance needs to be further improved by a large number of experimental explorations in the future. Overall, the development of proton-conducting electrolytes still faces many challenges.
Figure 4. Electrolytes with other structure types. (A) Oxides with An+1BnO3n+1 Ruddlesden-Popper structure; (B) Hexagonal perovskites with mixed hexagonal and cubic-packing layers; (C) Oxides with A2B2O5 Brownmillerite structure; (D) ReMO4 phase; (E) A2B2O7 oxides (F) Oxides with A10-xB6O26±δ apatite-type structure. Reproduced with permission from Fop et al.[49] Copyright 2021 Royal Society of Chemistry.
Figure 5. Total conductivity of proton conductors at 500 °C. Abbreviations: (1) BaZr0.1Ce0.7Y0.2O3-δ, BZCY172[45]; (2) BZCYYb1711[45];
Current challenges of electrolytes
As discussed in the section of electrolyte materials, the BZCM oxides still exhibit higher proton conductivity compared to other structural oxides. However, there are also several challenges in BZCM electrolyte design and preparation, primarily including the following aspects: (1) the balance between the stability and the proton conductivity should be achieved. This can be optimized by changing the preparation method of the electrolytes, such as using wet chemical methods or choosing some superior sintering additives to reduce the fabrication temperature of electrolytes with a high Zr content. Simultaneously, the influence of electronic leakage can be mitigated through the optimization of doped ions or functional layers. For electrolytes with a high Ce content, depositing some barrier layer on the electrolyte surface can prevent direct contact of electrolytes with the reactive atmosphere, thereby enhancing their stability; (2) The mechanical strength of the electrolytes and the compatibility with electrodes should be improved. Optimizing the size and shape of the cells can reduce the stress distribution on the materials, thereby improving mechanical strength. Additionally, the compatibility between the electrolyte and electrodes can be enhanced by closely matching their thermal expansion coefficients. Adjustments to the coating method of the air electrode and annealing process can also be made to reduce cracking and peeling during startup, shutdown, or thermal cycling of the devices; (3) Optimization of the electrolyte-air electrode interface. The high-temperature sintering process during the preparation of half-cells often leads to deviations in the composition of the electrolyte surface from its actual composition. This, combined with the subsequent assembly process with the air electrode, generates significant interface resistance, hindering proton transport at the interface and thus limiting the overall performance of the cells. Overcoming the aforementioned challenges and verifying the performance of designed electrolytes usually require considerable time and cost. Recently, the data-driven ML technology has garnered increasing attention. The combination between experiments and ML prediction models should be promising to streamline the electrolyte discovery process from design to validation.
ADVANCES AND PERSPECTIVES OF ML IN ELECTROLYTES
In the development of electrolytes for P-SOFCs, ML has shown significant advantages in material optimization, performance prediction, and mechanism research[58-61]. In addition to the above benefits, ML can deeply investigate proton conduction mechanisms and doping effects, achieving customized oxide development. This data-driven research paradigm has not only greatly improved the efficiency of electrolyte development but also provided new insights for innovative electrolyte design. Therefore, this chapter reviewed the latest advances in ML for P-SOFC electrolyte development, analyzed its advantages and limitations, and explored future applications.
Screened electrolytes by ML
In 2021, Hyodo et al.[23] constructed gradient boosting regressor (GBR) model to predict the hydrated proton concentrations (CH), equilibrium constants for hydration reactions (Khyd), hydration entropies
Figure 6. (A) Schematic workflow for discovering proton-conducting oxides by constructing gradient boosting regressor (GBR) model;
Figure 7. High-throughput calculation results of hydration energy of (A) BaCeO3-derived; (B) BaZrO3-derived; (C) BaHfO3-derived; and (D) BaSnO3-derived oxides; (E) Cross-sectional SEM image of BSCYb172/BSCYb172/BPHYC single cell; (F) Typical I-V curves measured in electrolysis mode at 500-650 °C air (30% H2O) in the air electrode; (G) and (H) I-V-P curves measured in the fuel mode at 400-650 °C with H2 (3% H2O) in the fuel electrode and air in the air electrode; (I) Comparison of peak power densities of
Figure 8. (A) The activation process of proton conduction in oxides; (B) Schematic workflow to screen the unconventional
In addition to the performance data from literature, the results from first-principles calculations can also serve as valuable input data for ML models. Several of the cited references are based on this type of calculation. For instance, in 2022, Islam et al.[64] reported systematic first-principles calculations on a wide range of terpolymer oxide materials to identify new proton conductors and to understand the role of cations and compositions on material stability and proton conductivity [Figure 9A]. By analyzing the calculated results of more than 5000 kinds of oxide materials, the effects of cation type and mole fraction on water stability and hydrogen insertion ability were revealed. By studying proton diffusion in many different materials, the analysis showed that oxide materials with connected BO6 octahedrons were most suitable for fast proton diffusion, and a dozen oxides with good water stability, good proton binding ability, and fast proton diffusion were identified using high-throughput calculations. This study provides basic understanding and design principles for developing oxide materials with fast proton diffusion and good stability [Figure 9]. In 2024, Szaro et al.[65] performed a high-throughput scan of 4,793 materials to determine how different cations regulate stability, electronic conductivity, and defect formation. As a result, 116 materials were identified as electrically inactive and stable under reduction and oxidation conditions, and 43 materials were also identified as stable under pure carbon dioxide. Moreover, the stability, formation of oxygen vacancies and proton defects of all 116 materials were analyzed to determine trends in ionic conductivity. This study provided an additional understanding of the role of elemental identity and doping ratios in material stability and activity, aiding in the design of perovskite oxides for proton conduction applications. To conclude, this subsection provided examples and summarized recent ML applications in screening proton-conducting electrolytes.
Figure 9. (A) High-throughput computational workflow for studying proton-conducting materials. Low-energy proton migration pathways and the energy profiles in representative proton-conducting materials; (B) and (C) perovskite YbCoO3; (D) and (E) SrMnO3, (F) and (G) Tb2Mo2O7, (H) and (I) CrMoO4, (J) and (K) MoPO5, (L) and (M) Eu3MoO7. Reproduced with permission from Islam et al.[64] Copyright 2022 American Chemical Society.
Perspectives of ML in electrolyte
Both ML and first-principles calculation speed up the development of electrolytes, thus accelerating the development of P-SOFCs. First-principles calculations are renowned for their universality and high precision, capable of providing accurate predictions of properties such as electronic structures for systems ranging from simple molecules to complex materials. These prediction results are directly related to physical laws, possess good theoretical interpretability, and, in most cases, do not rely on empirical parameters, thus reducing human errors. However, first-principles calculations require a large number of computational resources[66,67]. Especially for large systems or complex quantum chemistry problems, it may take hours or even days to complete a single calculation, which limits their application in large-scale material simulations and the construction of material big data. Meanwhile, ML stands out for its advantages such as fast computing speed, cost-effectiveness, and strong scalability. Once a model is trained, predictions can be completed rapidly and can be easily extended to new datasets. However, its prediction results are highly dependent on data quality, and ML models are usually regarded as “black boxes”, whose prediction results may be difficult to interpret from a physical perspective. Moreover, when trained on limited datasets, models may overfit, resulting in poor generalization ability[68,69]. Therefore, combining first-principles calculations with ML can fully utilize the advantages of both. First-principles calculations can generate accurate and relatively large databases for ML, while ML can significantly improve the efficiency of prediction and accelerate the iterative update of materials. Meanwhile, we can introduce physical knowledge into ML models to improve their accuracy and interpretability. For example, by combining first-principles calculations and ML, a theoretical-based proton conduction transport path can be constructed for more precise prediction of proton migration energy barriers. In conclusion, ML technology has already played an important role in the development of proton-SOFC electrolytes, but there are not many relevant reports at present. In the future, ML technique can play an even greater role, which is specifically manifested in the following aspects as shown in Figure 10.
Figure 10. Future applications of ML in electrolyte in terms of properties, stability, and microstructure prediction. ML: Machine learning.
Screen of high-performance electrolytes
By applying ML techniques, build models for predicting formation energy of oxides as the preliminary screening criteria for potential electrolytes. For the oxides with lower formation energy, ML models can further predict key properties such as proton conductivity, concentration of proton defects in hydration reaction, and thermal expansion coefficient[70-72]. Combined with first-principles calculations, to predict and screen thermodynamic parameters such as proton migration energy barriers, reaction equilibrium constants, entropy changes, and enthalpy changes in the hydration reaction of substances, clarify the ion transport mechanism in the electrolyte and the thermodynamic essence of the reactions in the electrolyte. Through integrating the results of ML and theoretical calculations, a composition-structure-property relationship model can be established to provide guidance for the design and optimization of electrolyte materials.
Prediction of electrolyte stability
The stability of electrolytes is one of the key indicators for measuring their performance. During the actual working process, electrolytes need to be in contact with oxidizing and reducing gases at high temperatures, such as oxygen and hydrogen. Therefore, electrolytes must possess sufficient chemical stability to avoid adverse reactions with these gases. For this case, the first-principles calculations can be used to calculate changes in some energies. By analyzing the energy changes of reactions, the reactivity and stability of electrolytes under specific conditions can be predicted. Specifically, indicators such as reaction energy, adsorption energy, and migration energy barriers can be calculated, and in-depth understanding of the electronic interactions among them can be achieved through electronic structure analysis, so as to evaluate the stability of electrolytes as follows:
Reaction energy: By calculating the energy difference before and after the reaction between the electrolyte and gas molecules, the thermodynamic feasibility of the reaction can be evaluated. If the reaction energy is high, it indicates that the reaction is difficult to proceed and the electrolyte has good stability.
Adsorption energy: Analyzing the adsorption energy of gas molecules on the surface of the electrolyte can help understand the strength of the interaction between gas molecules and the electrolyte. A lower adsorption energy usually means a weaker interaction, which is beneficial to the stability of the electrolyte.
Migration energy barriers: Calculating the migration energy barriers of gas molecules in the electrolyte can predict the diffusion behavior of gas molecules in the electrolyte. Higher migration energy barriers help to limit the diffusion of gas molecules, thereby improving the stability of the electrolyte.
Electronic structure analysis: By analyzing the electron density distribution and energy band structure between the electrolyte and gas molecules, an in-depth understanding of the electronic interactions between them can be achieved, so as to evaluate the stability of the electrolyte.
In summary, through these calculations, the stability of electrolyte materials in high-temperature and gas environments can be comprehensively evaluated, providing a theoretical basis for the selection and optimization of materials. This method helps to screen out electrolyte materials with high stability in practical applications.
Analyzation of microstructure of electrolytes
Combining image recognition technology and materials science, use high-resolution microscopes [such as scanning electron microscopy (SEM) and transmission electron microscopy (TEM) images] to obtain microstructure information of electrolyte materials[73-75]. Apply image recognition techniques, such as edge detection, texture analysis, and pattern recognition, to process and analyze these images, and extract key microstructure features of electrolytes, such as grain size, shape, distribution, grain boundary characteristics, pores, and other microscopic defects. Utilize ML algorithms to correlate the extracted microstructure features with performance data such as the conductivity, mechanical strength, and thermal stability of electrolyte materials, and construct a microstructure-property (conductivity, etc.) mapping model. By analyzing the changes in microstructure under different conditions (such as temperature, humidity, and electrochemical environment), predict the evolution law of the microstructure of electrolyte materials and analyze the performance degradation mechanism. Meanwhile, according to the prediction results of the microstructure-property mapping model, adjust the composition and processing technology of the electrolyte to optimize its microstructure and improve the performance and stability of the materials.
In conclusion, the combination of ML technology and first-principles calculations provides a powerful tool for the research on electrolyte materials, making the screening and optimization process of materials more efficient and accurate. With the continuous progress of ML technology and the expansion of its application scope, it is expected that more breakthroughs will be achieved in the field of P-SOFCs in the future. Meanwhile, this interdisciplinary approach is becoming an active research field in materials science. It can provide strong support for the development of future clean energy technologies and pave the way for the progress of sustainable energy conversion technologies.
CHALLENGES AND SOLUTIONS OF ML IN ELECTROLYTES
Although ML has shown great potential in the research on P-SOFC electrolytes and provided new possibilities for accelerating the discovery of new materials and the iterative innovation of P-SOFCs, it still faces several challenges. In this chapter, we summarize the existing challenges of ML in the design of electrolyte materials and their future solutions, as shown in Figure 11.
Figure 11. The challenges and possible solutions for ML in proton-conducting electrolyte predictions. ML: Machine learning.
Data scarcity and quality
Data plays a crucial role in promoting AI’s understanding and innovation of material properties, and its quality directly determines the accuracy of prediction results[76,77]. In materials science, the scarcity of high-quality experimental data is a significant problem, especially for data that systematically characterizes material properties, such as proton conductivity and transport number. These data form the basis for understanding and predicting the behavior of materials. However, due to the complexity of experimental conditions and the limitation of resources, obtaining such data is both costly and time-consuming. In addition, the lack of uniformity in data standards is also a challenge. Different laboratories and research institutions may adopt different experimental conditions and testing methods, making it difficult to directly compare and integrate data from different sources. This heterogeneity of data limits the effectiveness of model training and may affect the accuracy and reliability of the model. The widespread absence of failed experiment data is another issue, which leads to biases in datasets. In scientific research, unreported failure cases may distort the results of model learning and affect the model’s ability to comprehensively understand material properties. Furthermore, some key parameters, such as interface properties and microstructural features, are difficult to accurately quantify. The uncertainty of these parameters increases the complexity of model prediction and may affect the generalization ability of the model. In conclusion, ML models in materials design face multiple challenges regarding data quality and availability. These challenges need to be overcome by enhancing datasets, unifying data standards, collecting and utilizing failed experiment data, and developing new measurement techniques to improve the accuracy and reliability of the models.
The materials science has already adopted a series of strategies to improve the data quality and availability. Firstly, establishing a standardized materials database is the foundation, including unifying characterization methods and data formats to ensure that data from different sources and types can be effectively compared and integrated. Meanwhile, a data quality assessment system is crucial for monitoring and improving the accuracy and reliability of data. By this way, the multi-source experimental data can be integrated, thus providing a more comprehensive view of material properties and enhancing the generalization ability of the model. Secondly, developing a high-throughput experimental platform with automated material preparation and standardized testing procedures is important for improving experimental efficiency and ensuring consistency of experimental conditions, which is essential for comparing the properties of different materials and building a reliable database. Real-time data acquisition and analysis can quickly provide feedback on experimental results and deliver immediate data support for model training. Finally, constructing a data-sharing mechanism is a key strategy to enhancing data quality. An open data platform encourages collaboration among researchers and accelerates new material discovery, while standardized sharing protocols ensure data reliability, interoperability, and reproducibility across studies. Through these strategies, the data quality in the field of materials science can be significantly improved, providing more accurate and reliable training data for ML models, and thus promoting the process of materials design and discovery.
Descriptor selection and feature engineering
In materials design, descriptor selection and feature engineering are crucial steps that directly affect the performance (accuracy and interpretability) and prediction ability of ML models[78-80]. However, this process faces a series of challenges. Firstly, it is extremely difficult to establish a comprehensive and effective material descriptor system. The properties of materials are influenced by multiple factors, including their chemical composition, crystal structure, and electronic properties, and there are complex interactions among these factors. Therefore, to comprehensively capture the impact of these factors on material properties, it is necessary to construct a descriptor system that considers various physical and chemical attributes. Secondly, the structure-property relationships in materials are complex and difficult to fully express with simple features. This is because the properties of materials are often jointly influenced by their microscopic structure and macroscopic properties, and there are nonlinear and multiscale interactions among these properties. For example, the ion conduction mechanism involves multiscale factors and requires multi-level descriptors to comprehensively capture its influence. Thirdly, it is challenging to characterize the features of dynamic processes and interface effects. The dynamic behavior of material interfaces, such as the dynamic enrichment, movement, and trajectories of molecules, is crucial for understanding the interface properties of materials. However, due to the inherent fluidity and deformability of liquid interfaces, these interfaces exhibit complex dynamic interface properties that are different from the basic chemical and physical properties of the two phases, and the dynamically changing structures are inhomogeneous. In addition, the state of interface molecular behavior is affected by the states of the two phases (e.g., the concentration distributions of impurities and specific molecules) and the environmental conditions of the interface (e.g., temperature and pressure), making it challenging to distinguish locations and interfaces in a short time. In conclusion, the importance of descriptor selection and feature engineering in materials design is self-evident; however, at the same time, it also faces difficulties in establishing a comprehensive descriptor system, challenges in expressing complex structure-property relationships, and problems in characterizing dynamic processes and interface effects. Solving these problems requires interdisciplinary cooperation, innovative experimental techniques, and advanced computational methods.
To overcome these challenges, optimization strategies for feature engineering are particularly important. Firstly, constructing a multiscale descriptor system is crucial for comprehensively capturing the complex properties of materials. This includes combining quantum mechanical calculations to understand the behavior of materials at the atomic and electronic levels, integrating experimental characterization data to obtain the macroscopic properties of materials, and introducing physicochemical features to describe the thermodynamic and kinetic properties of materials. This multiscale approach can provide more comprehensive inputs for the model, thereby improving the prediction accuracy. Secondly, dynamic feature characterization is the key to capturing the behavior of materials in practical applications. Developing in situ characterization techniques can monitor the performance changes of materials under different environments and conditions in real time; capturing the interface evolution process provides an in-depth understanding of the stability and reaction mechanism of materials, and quantifying dynamic parameters can reveal the response characteristics of materials under actual working conditions. Finally, feature selection and dimensionality reduction are important steps to improve the efficiency and interpretability of the model. Applying dimensionality reduction techniques such as principal component analysis can help identify the key influencing factors in the data, remove redundancies and noise, and thus simplify the feature space. This not only reduces the complexity of the model but also improves the computational efficiency and helps researchers understand which features have the most significant impact on material properties. In conclusion, the execution of these strategies can significantly improve the applications of ML models in materials design, enabling them to predict and optimize the properties of materials more accurately.
Model limitations
In the fields of ML and materials design, the limitations of models are a problem that cannot be ignored. Firstly, prediction models are highly dependent on the training dataset, which means that the performance and accuracy of the models are largely limited by the quality and diversity of the training dataset[81,82]. If the training data is biased or incomplete, the prediction results of the model will also be affected, resulting in limited extrapolation ability of the model when facing materials with completely new structures and making it difficult to make accurate performance predictions. In addition, many models, especially deep learning models, are regarded as “black boxes” whose internal decision-making processes are opaque and difficult to interpret, which limits our understanding of the deep physical mechanisms behind the model prediction results. Finally, in materials design, it is often necessary to optimize multiple performance indicators simultaneously, such as strength, toughness, and electrical conductivity. There may be conflicts among these objectives, and quantifying the trade-off relationships among these objectives is a challenge because they may be affected by multiple factors, including the microscopic structure of materials and processing conditions. These limitations jointly restrict the application effect of ML models in materials design and need to be overcome through methods such as enhancing datasets, transfer learning, interpretable ML, and multi-objective optimization algorithms.
Model optimization strategies are crucial for improving the accuracy and reliability of predictions. Firstly, the adoption of hybrid modeling methods can effectively combine the advantages of physical models and data-driven models. This method can more comprehensively capture the complexity of material behavior by integrating physics-based first-principles calculations and data-based ML techniques. Multi-model integrated prediction can combine the prediction results of different models to improve the overall prediction performance, and introducing expert knowledge to guide model training can further enhance the generalization ability and prediction accuracy of the model. Secondly, the application of transfer learning is another important direction for model optimization. By utilizing the knowledge of existing models, the demand for a large amount of labeled data can be reduced, especially in the prediction of new materials or unknown materials. Transfer learning enables models to quickly adapt to new tasks, improves the accuracy of predictions, and reduces training costs and time. Finally, uncertainty quantification is the key to optimizing model reliability. Establishing an error assessment system can help us understand the sources of uncertainty in model predictions, and providing confidence intervals can provide important information for decision-makers about the credibility of prediction results. In conclusion, through these methods, the reliability of the model can be optimized, which makes it more robust in practical applications and provides strong support for the discovery and development of new materials.
Experimental verification bottlenecks
Experimental verification is a crucial link for the prediction results of ML models, but this process faces a series of challenges and bottlenecks[81]. Firstly, the prediction results of ML models require a large number of experiments for verification, which involves high costs. Secondly, the preparation and characterization processes of materials themselves are time-consuming, which makes the cycle from prediction to verification quite long and affects the efficiency of new material research and development. In addition, it is difficult to standardize the performance testing conditions. Different laboratories or research teams may adopt varying testing methods and conditions, making it difficult to directly compare and integrate data and increasing the complexity of experimental verification. Finally, it is challenging to integrate the experimental feedback data. Due to the diverse data sources and inconsistent formats, effectively integrating these data and using them for further training and optimization of the model is a technical challenge. These bottlenecks not only limit the speed of material research and development but also affect the reliability and universality of the research results. Therefore, to accelerate the research and development process of new materials, it is necessary to develop new experimental techniques and data processing methods to improve the efficiency and accuracy of experimental verification.
In materials science research, the establishment of an experimental verification system is crucial for ensuring the accuracy and practicability of the prediction results of ML models. Firstly, intelligent experimental design, by adopting active learning strategies, can effectively optimize the experimental scheme and select the most valuable experiments to be conducted, thereby reducing the verification cost and improving the experimental efficiency. This strategy allows researchers to decide the next best experiment according to the current knowledge state of the model, making the experimental process more goal-oriented. Secondly, the development of rapid characterization methods is crucial for accelerating the experimental verification process. High-throughput testing techniques make it possible to test multiple materials simultaneously, and automated data analysis can quickly process a large amount of experimental data, providing a real-time feedback mechanism that enables researchers to quickly adjust the research direction or optimize the experimental conditions to obtain more accurate results. Finally, a standardized evaluation process is the key to ensuring the reliability and comparability of the experimental verification results. Establishing a performance index system can provide a unified standard for the performance evaluation of different materials, and unifying the testing conditions ensures the consistency of the results of different experiments. Standardizing the evaluation method can reduce the errors caused by differences in experimental operations and improve the accuracy and reliability of the experimental results. In conclusion, the above methods can significantly improve the efficiency and effect of materials science research, providing a solid experimental basis for the predictions of ML models and accelerating the discovery and development process of new materials.
CONCLUSION AND OUTLOOK
This review comprehensively summarized the different sustainable energy utilization technologies, including PEMFC, AFC, PAFC, and SOFC, and discussed their advantages and challenges. Specially, the promising prospects of P-SOFC in the field of hydrogen energy utilization were addressed. The working mechanism of P-SOFC relies on proton-conducting electrolyte materials, which require high proton conductivity and extremely low electronic conductivity to ensure efficient energy conversion processes. More importantly, the P-SOFC electrolytes should be endured to the operating temperature, high-water vapor pressure and CO2 environments. Accordingly, an overview of the proton conductivities and the chemical stabilities of the most popular Ba(Zr, Ce)O3 perovskite electrolytes were addressed and only a handful of electrolytes can meet these requirements despite decades of efforts. Recently, ML has shown excellent advantages and enormous potential in electrolyte material development. Following these, the latest ML-assisted advances of electrolyte properties, including hydration ability, conductivity, and oxygen vacancy formation energy were summarized and discussed. The integration of ML with first-principles calculations can achieve efficient and precise screening of high-performance and high-stability electrolytes in the future. Additionally, by combining image recognition technology, it becomes feasible to investigate the microstructure-property relationships of electrolytes under various operating conditions. However, the design of electrolytes by ML still faces multiple challenges in high-quality and availability data, effective material feature engineering, model limitations and experimental verification. Finally, corresponding solutions including establishing standardized materials databases, constructing multiscale descriptor systems, applying hybrid modeling methods, and developing intelligent experimental processes were proposed to solve these challenges. Thus, accelerating the design of P-SOFC electrolytes and the discovery of other energy conversion materials by effective and accurate ML models.
DECLARATIONS
Authors’ contributions
Conceptualization and design, writing-original draft: Tang, C.; Yuan, B.
Conceptualization and design: Wang, N.
Writing-review and editing: Wang, N.; Ye, S.
Provided useful suggestions: Aoki, Y.; Xie, X.
Contributed equally to this work: Tang, C.; Yuan, B.
Availability of data and materials
Not applicable.
Financial support and sponsorship
This work was supported by the National Natural Science Foundation of China (Nos. 12301626, 22409033, and 22409035), China Post-doctoral Science Foundation (No. 2024M750615), Guangdong Basic and Applied Basic Research Foundation (Nos. 2022A1515110612, 2022A1515110470 and 2024A1515011849), Funding by Science and Technology Projects in Guangzhou (Nos. 2025A03J3089 and 2024A04J4111), and Guangdong Engineering Technology Research Center for Hydrogen Energy and Fuel Cells.
Conflicts of interest
All authors declared that there are no conflicts of interest.
Ethical approval and consent to participate
Not applicable.
Consent for publication
Not applicable.
Copyright
© The Author(s) 2025.
REFERENCES
1. Chen, P.; Xiao, Y.; Li, S.; et al. The promise and challenges of inverted perovskite solar cells. Chem. Rev. 2024, 124, 10623-700.
2. Pryor, S. C.; Barthelmie, R. J.; Bukovsky, M. S.; Leung, L. R.; Sakaguchi, K. Climate change impacts on wind power generation. Nat. Rev. Earth. Environ. 2020, 1, 627-43.
3. Wang, N.; Tang, C.; Du, L.; et al. Advanced cathode materials for protonic ceramic fuel cells: recent progress and future perspectives. Adv. Energy. Mater. 2022, 12, 2201882.
4. Hu, A.; Yang, C.; Li, Y.; et al. High-entropy driven self-assembled dual-phase composite air electrodes with enhanced performance and stability for reversible protonic ceramic cells. Adv. Energy. Mater. 2025, 2405466.
5. Oni, A. M.; Mohsin, A. S.; Rahman, M. M.; Hossain, Bhuian., M. B. A comprehensive evaluation of solar cell technologies, associated loss mechanisms, and efficiency enhancement strategies for photovoltaic cells. Energy. Rep. 2024, 11, 3345-66.
6. Dai, K.; Bergot, A.; Liang, C.; Xiang, W.; Huang, Z. Environmental issues associated with wind energy - a review. Renew. Energy. 2015, 75, 911-21.
7. Sayed, E. T.; Wilberforce, T.; Elsaid, K.; et al. A critical review on environmental impacts of renewable energy systems and mitigation strategies: wind, hydro, biomass and geothermal. Sci. Total. Environ. 2021, 766, 144505.
8. Kuriqi, A.; Pinheiro, A. N.; Sordo-ward, A.; Garrote, L. Water-energy-ecosystem nexus: Balancing competing interests at a run-of-river hydropower plant coupling a hydrologic–ecohydraulic approach. Energy. Convers. Manag. 2020, 223, 113267.
9. Soltani, M.; Moradi, Kashkooli., F.; Souri, M.; et al. Environmental, economic, and social impacts of geothermal energy systems. Renew. Sustain. Energy. Rev. 2021, 140, 110750.
10. Mekhilef, S.; Saidur, R.; Safari, A. Comparative study of different fuel cell technologies. Renew. Sustain. Energy. Rev. 2012, 16, 981-9.
11. Tellez-Cruz, M. M.; Escorihuela, J.; Solorza-Feria, O.; Compañ, V. Proton exchange membrane fuel cells (PEMFCs): advances and challenges. Polymers 2021, 13, 3064.
12. Das, G.; Choi, J. H.; Nguyen, P. K. T.; Kim, D. J.; Yoon, Y. S. Anion exchange membranes for fuel cell application: a review. Polymers 2022, 14, 1197.
13. Petrovic, S.; Hossain, E. Development of a novel technological readiness assessment tool for fuel cell technology. IEEE. Access. 2020, 8, 132237-52.
14. Yu, G.; Dai, C.; Liu, N.; Xu, R.; Wang, N.; Chen, B. Hydrocarbon extraction with ionic liquids. Chem. Rev. 2024, 124, 3331-91.
15. Alaswad, A.; Omran, A.; Sodre, J. R.; et al. Technical and commercial challenges of proton-exchange membrane (PEM) fuel cells. Energies 2021, 14, 144.
16. Xiao, F.; Wang, Y. C.; Wu, Z. P.; et al. Recent advances in electrocatalysts for proton exchange membrane fuel cells and alkaline membrane fuel cells. Adv. Mater. 2021, 33, 2006292.
17. Mclean, G. An assessment of alkaline fuel cell technology. Int. J. Hydrogen. Energy. 2002, 27, 507-26.
18. Kirubakaran, A.; Jain, S.; Nema, R. A review on fuel cell technologies and power electronic interface. Renew. Sustain. Energy. Rev. 2009, 13, 2430-40.
19. Tang, C.; Yao, Y.; Wang, N.; et al. Green hydrogen production by intermediate-temperature protonic solid oxide electrolysis cells: advances, challenges, and perspectives. InfoMat 2024, 6, e12515.
20. Weng, B.; Song, Z.; Zhu, R.; et al. Simple descriptor derived from symbolic regression accelerating the discovery of new perovskite catalysts. Nat. Commun. 2020, 11, 3513.
21. Li, Z.; Mao, X.; Feng, D.; et al. Prediction of perovskite oxygen vacancies for oxygen electrocatalysis at different temperatures. Nat. Commun. 2024, 15, 9318.
22. Divilov, S.; Eckert, H.; Hicks, D.; et al. Disordered enthalpy-entropy descriptor for high-entropy ceramics discovery. Nature 2024, 625, 66-73.
23. Hyodo, J.; Tsujikawa, K.; Shiga, M.; Okuyama, Y.; Yamazaki, Y. Accelerated discovery of proton-conducting perovskite oxide by capturing physicochemical fundamentals of hydration. ACS. Energy. Lett. 2021, 6, 2985-92.
24. Priya, P.; Aluru, N. R. Accelerated design and discovery of perovskites with high conductivity for energy applications through machine learning. npj. Comput. Mater. 2021, 7, 90.
25. Cao, J.; Ji, Y.; Shao, Z. Perovskites for protonic ceramic fuel cells: a review. Energy. Environ. Sci. 2022, 15, 2200-32.
26. Duan, C.; Huang, J.; Sullivan, N.; O’hayre, R. Proton-conducting oxides for energy conversion and storage. Appl. Phys. Rev. 2020, 7, 011314.
27. Liu, F.; Deng, H.; Diercks, D.; et al. Lowering the operating temperature of protonic ceramic electrochemical cells to < 450 °C. Nat. Energy. 2023, 8, 1145-57.
28. Bello, I. T.; Zhai, S.; Zhao, S.; Li, Z.; Yu, N.; Ni, M. Scientometric review of proton-conducting solid oxide fuel cells. Int. J. Hydrogen. Energy. 2021, 46, 37406-28.
29. Sajid, M.; Hassan, I.; Rahman, A. An overview of cooling of thermoelectric devices. Renew. Sustain. Energy. Rev. 2017, 78, 15-22.
30. Kreuer, K.; Rabenau, A.; Weppner, W. Vehicle Mechanism, A new model for the interpretation of the conductivity of fast proton conductors. Angew. Chem. Int. Ed. 1982, 21, 208-9.
31. Su, H.; Hu, Y. H. Degradation issues and stabilization strategies of protonic ceramic electrolysis cells for steam electrolysis. Energy. Sci. Eng. 2022, 10, 1706-25.
32. Kim, J.; Sengodan, S.; Kim, S.; Kwon, O.; Bu, Y.; Kim, G. Proton conducting oxides: a review of materials and applications for renewable energy conversion and storage. Renew. Sustain. Energy. Rev. 2019, 109, 606-18.
33. Jing, J.; Pang, J.; Chen, L.; Zhang, H.; Lei, Z.; Yang, Z. Structure, synthesis, properties and solid oxide electrolysis cells application of Ba(Ce, Zr)O3 based proton conducting materials. Chem. Eng. J. 2022, 429, 132314.
34. Geneste, G. Proton transfer in barium zirconate: lattice reorganization, landau-zener curve-crossing approach. Solid. State. Ion. 2018, 323, 172-202.
35. Seong, A.; Kim, J.; Jeong, D.; et al. Electrokinetic proton transport in triple (H+/O2-/e-) conducting oxides as a key descriptor for highly efficient protonic ceramic fuel cells. Adv. Sci. 2021, 8, 2004099.
36. Iwahara, H.; Esaka, T.; Uchida, H.; Maeda, N. Proton conduction in sintered oxides and its application to steam electrolysis for hydrogen production. Solid. State. Ion. 1981, 3-4, 359-63.
37. Iwahara, H.; Uchida, H.; Maeda, N. High temperature fuel and steam electrolysis cells using proton conductive solid electrolytes. J. Power. Sources. 1982, 7, 293-301.
38. Iwahara, H.; Uchida, H.; Tanaka, S. High temperature type proton conductor based on SrCeO3 and its application to solid electrolyte fuel cells. Solid. State. Ion. 1983, 9-10, 1021-5.
39. Iwahara, H.; Yajima, T.; Ushida, H. Effect of ionic radii of dopants on mixed ionic conduction (H++O2-) in BaCeO3-based electrolytes. Solid. State. Ion. 1994, 70-71, 267-71.
40. Iwahara, H.; Yajima, T.; Hibino, T.; Ozaki, K.; Suzuki, H. Protonic conduction in calcium, strontium and barium zirconates. Solid. State. Ion. 1993, 61, 65-9.
41. Iwahara, H. Proton conducting ceramics and their applications. Solid. State. Ion. 1996, 86-88, 9-15.
42. Tarasova, N.; Hanif, M. B.; Janjua, N. K.; Anwar, S.; Motola, M.; Medvedev, D. Fluorine-insertion in solid oxide materials for improving their ionic transport and stability. A brief review. Int. J. Hydrogen. Energy. 2024, 50, 104-23.
43. Matkin, D. E.; Starostina, I. A.; Hanif, M. B.; Medvedev, D. A. Revisiting the ionic conductivity of solid oxide electrolytes: a technical review. J. Mater. Chem. A. 2024, 12, 25696-714.
44. Tian, Y.; Abhishek, N.; Yang, C.; et al. Progress and potential for symmetrical solid oxide electrolysis cells. Matter 2022, 5, 482-514.
45. Yang, L.; Wang, S.; Blinn, K.; et al. Enhanced sulfur and coking tolerance of a mixed ion conductor for SOFCs: BaZr0.1Ce0.7Y0.2-xYbxO3-δ. Science 2009, 326, 126-9.
46. Duan, C.; Tong, J.; Shang, M.; et al. Readily processed protonic ceramic fuel cells with high performance at low temperatures. Science 2015, 349, 1321-6.
47. Choi, S.; Kucharczyk, C. J.; Liang, Y.; et al. Exceptional power density and stability at intermediate temperatures in protonic ceramic fuel cells. Nat. Energy. 2018, 3, 202-10.
48. Saito, K.; Yashima, M. High proton conductivity within the ‘Norby gap’ by stabilizing a perovskite with disordered intrinsic oxygen vacancies. Nat. Commun. 2023, 14, 7466.
50. Tarasova, N.; Animitsa, I.; Galisheva, A.; Korona, D. Incorporation and conduction of protons in Ca, Sr, Ba-doped BaLaInO4 with ruddlesden-popper structure. Materials 2019, 12, 1668.
51. Murakami, T.; Hester, J. R.; Yashima, M. High proton conductivity in Ba5Er2Al2ZrO13, a hexagonal perovskite-related oxide with intrinsically oxygen-deficient layers. J. Am. Chem. Soc. 2020, 142, 11653-7.
52. Haugsrud, R.; Norby, T. High-temperature proton conductivity in acceptor-substituted rare-earth ortho-tantalates, LnTaO4. J. Am. Ceram. Soc. 2007, 90, 1116-21.
53. Kim, D.; Bae, K. T.; Kim, K. J.; et al. High-performance protonic ceramic electrochemical cells. ACS. Energy. Lett. 2022, 7, 2393-400.
54. Fop, S.; McCombie, K. S.; Wildman, E. J.; et al. High oxide ion and proton conductivity in a disordered hexagonal perovskite. Nat. Mater. 2020, 19, 752-7.
55. Ling, C. D.; Avdeev, M.; Kharton, V. V.; Yaremchenko, A. A.; Macquart, R. B.; Hoelzel, M. Structures, phase transitions, hydration, and ionic conductivity of Ba4Ta2O9. Chem. Mater. 2010, 22, 532-40.
56. Haugsrud, R.; Norby, T. Proton conduction in rare-earth ortho-niobates and ortho-tantalates. Nature. Mater. 2006, 5, 193-6.
57. Wang, J.; Xie, Y.; Zhang, Z.; Liu, R.; Li, Z. Protonic conduction in Ca2+-doped La2M2O7 (M=Ce, Zr) with its application to ammonia synthesis electrochemically. Mater. Res. Bull. 2005, 40, 1294-302.
58. Wang, N.; Yuan, B.; Zheng, F.; et al. Machine-learning assisted screening proton conducting Co/Fe based oxide for the air electrode of protonic solid oxide cell. Adv. Funct. Mater. 2024, 34, 2309855.
59. Nomura, K.; Shimada, H.; Yamaguchi, Y.; et al. Machine learning based prediction of space group for Ba(Ce0.8-xZrx)Y0.2O3 perovskite-type protonic conductors. Ceram. Int. 2023, 49, 5058-65.
60. Bello, I. T.; Guan, D.; Yu, N.; et al. Revolutionizing material design for protonic ceramic fuel cells: bridging the limitations of conventional experimental screening and machine learning methods. Chem. Eng. J. 2023, 477, 147098.
61. Zhai, S.; Xie, H.; Cui, P.; et al. A combined ionic Lewis acid descriptor and machine-learning approach to prediction of efficient oxygen reduction electrodes for ceramic fuel cells. Nat. Energy. 2022, 7, 866-75.
62. Luo, Z.; Hu, X.; Zhou, Y.; et al. Harnessing high-throughput computational methods to accelerate the discovery of optimal proton conductors for high-performance and durable protonic ceramic electrochemical cells. Adv. Mater. 2024, 36, 2311159.
63. Fujii, S.; Shimizu, Y.; Hyodo, J.; Kuwabara, A.; Yamazaki, Y. Discovery of unconventional proton-conducting inorganic solids via defect-chemistry-trained, interpretable machine learning. Adv. Energy. Mater. 2023, 13, 2301892.
64. Islam, M. S.; Wang, S.; Hall, A. T.; Mo, Y. First-principles computational design and discovery of solid-oxide proton conductors. Chem. Mater. 2022, 34, 5938-48.
65. Szaro, N. A.; Ammal, S. C.; Chen, F.; Heyden, A. First principles material screening and trend discovery for the development of perovskite electrolytes for proton-conducting solid oxide fuel cells. J. Power. Sources. 2024, 603, 234411.
66. Freysoldt, C.; Grabowski, B.; Hickel, T.; et al. First-principles calculations for point defects in solids. Rev. Mod. Phys. 2014, 86, 253-305.
67. Peng, J.; Zhao, Y.; Wang, X.; Zeng, X.; Wang, J.; Hou, S. Metal-organic frameworks: advances in first-principles computational studies on catalysis, adsorption, and energy storage. Mater. Today. Commun. 2024, 40, 109780.
68. Tawfik, S. A.; Russo, S. P. Naturally-meaningful and efficient descriptors: machine learning of material properties based on robust one-shot ab initio descriptors. J. Cheminform. 2022, 14, 78.
69. Nazemzadeh, N.; Malanca, A. A.; Nielsen, R. F.; Gernaey, K. V.; Andersson, M. P.; Mansouri, S. S. Integration of first-principle models and machine learning in a modeling framework: an application to flocculation. Chem. Eng. Sci. 2021, 245, 116864.
70. Bello, I. T.; Taiwo, R.; Esan, O. C.; et al. AI-enabled materials discovery for advanced ceramic electrochemical cells. Energy. AI. 2024, 15, 100317.
71. Li, Z.; Wang, C.; Chen, X.; et al. A deep-learning-boosted surrogate model of a metal foam based protonic ceramic electrolysis cell stack for uncertainty quantification. Energy. Convers. Manag. 2024, 318, 118886.
72. Liu, X.; Yan, Z.; Wu, J.; et al. Prediction of impedance responses of protonic ceramic cells using artificial neural network tuned with the distribution of relaxation times. J. Energy. Chem. 2023, 78, 582-8.
73. Chowdhury, A.; Kautz, E.; Yener, B.; Lewis, D. Image driven machine learning methods for microstructure recognition. Comput. Mater. Sci. 2016, 123, 176-87.
74. Ge, M.; Su, F.; Zhao, Z.; Su, D. Deep learning analysis on microscopic imaging in materials science. Mater. Today. Nano. 2020, 11, 100087.
75. Lee, B.; Yoon, S.; Lee, J. W.; et al. Statistical characterization of the morphologies of nanoparticles through machine learning based electron microscopy image analysis. ACS. Nano. 2020, 14, 17125-33.
76. Nandy, A.; Duan, C.; Kulik, H. J. Audacity of huge: overcoming challenges of data scarcity and data quality for machine learning in computational materials discovery. Curr. Opin. Chem. Eng. 2022, 36, 100778.
77. Jablonka, K. M.; Ongari, D.; Moosavi, S. M.; Smit, B. Big-data science in porous materials: materials genomics and machine learning. Chem. Rev. 2020, 120, 8066-129.
78. Anand, D. V.; Xu, Q.; Wee, J.; Xia, K.; Sum, T. C. Topological feature engineering for machine learning based halide perovskite materials design. npj. Comput. Mater. 2022, 8, 203.
79. Himanen, L.; Jäger, M. O.; Morooka, E. V.; et al. DScribe: library of descriptors for machine learning in materials science. Comput. Phys. Commun. 2020, 247, 106949.
80. Li, Z.; Ma, X.; Xin, H. Feature engineering of machine-learning chemisorption models for catalyst design. Catal. Today. 2017, 280, 232-8.
81. Liu, Y.; Zhao, T.; Ju, W.; Shi, S. Materials discovery and design using machine learning. J. Materiomics. 2017, 3, 159-77.
Cite This Article

How to Cite
Download Citation
Export Citation File:
Type of Import
Tips on Downloading Citation
Citation Manager File Format
Type of Import
Direct Import: When the Direct Import option is selected (the default state), a dialogue box will give you the option to Save or Open the downloaded citation data. Choosing Open will either launch your citation manager or give you a choice of applications with which to use the metadata. The Save option saves the file locally for later use.
Indirect Import: When the Indirect Import option is selected, the metadata is displayed and may be copied and pasted as needed.
About This Article
Copyright
Data & Comments
Data
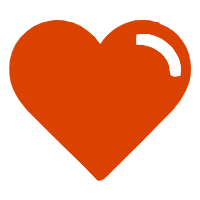
Comments
Comments must be written in English. Spam, offensive content, impersonation, and private information will not be permitted. If any comment is reported and identified as inappropriate content by OAE staff, the comment will be removed without notice. If you have any queries or need any help, please contact us at [email protected].