Mapping carbon emission networks in China: insights from province-level spatial differentiation
Abstract
In the current global economic downturn and energy transition period, how to better coordinate the differences in carbon emission footprints among sub-regions has become an emerging issue. With the Gini decomposition method, social network analysis, and difference-in-differences estimation, this study explores the spatial differentiation of China’s province-level carbon emission footprint from 2000 to 2021. The findings of this study indicate that: (1) The Gini-based carbon emission footprint index shows an overall upward trend, revealing the constantly expanding differences among provinces. By comparison, the crude oil difference between the low-carbon pilot and non-pilot provinces is evident, reaching more than 0.15; (2) The carbon emission footprint spatial correlation network structure shows strong spillover characteristics. Provinces with higher network centrality have better structural holes, maintaining closer relationships with surrounding provinces. Those pilot provinces have a comparative advantage regarding social network position, as they have more effective mutual node connections; and (3) China’s low-carbon pilot policy can effectively reduce carbon emissions, with a certain reduction effect of
Keywords
INTRODUCTION
Since the 21st century, the increasing growth of carbon emissions has aroused severe global climate changes, affecting all kinds of social production and human life[1-3]. To effectively stem this devastating trend, countries worldwide have taken action together by making carbon neutrality commitments[4-6]. As of 2023, at least 137 countries have committed to the target vision through all-round net-zero emissions by the middle of this century, of which 124 countries are scheduled by 2050[7,8]. China, the largest developing country and the major carbon-emitting country, also made its own commitment at the United Nations General Assembly in 2020, with a carbon peak by 2030 and carbon neutrality by 2060[9]. In this sense, figuring out the practical pathway to achieving carbon neutrality during the critical period of combating climate change will be important.
Carbon emission footprint, an effective approach to dealing with climate change in a socio-economic manner, can deeply explore the sources, processes, and mechanisms of carbon emissions to some extent[10,11]. Evolved from the ecological footprint representing the necessary area required to sustain human existence, the carbon emission footprint focuses more on the benefits or consequences per unit of carbon emissions[12,13]. Therefore, no unified definition remains, as the “per unit” can be adjusted according to the research field[14]. Considering its unique measurement and meaning, a considerable number of documents have analyzed the carbon emission footprint in detail[15-17]. As for its calculation method, studies normally use the Intergovernmental Panel on Climate Change method, the input-output method, and the life cycle assessment[18,19]. As for its dynamic changes, research has assessed the carbon emission flow at the national, province, city, and product levels[1,20]. As for its influencing factor, numerous works have explored the economic, sectoral, industrial, and social causes of carbon emissions[21,22].
Nevertheless, some important issues still remain to be discussed in depth, especially in the current global economic downturn and energy transition[23-25]. One essential puzzle is how to balance the regional coordinated development. Although the overall development trend of carbon emissions can be controlled at the national aggregate level, the sub-regional differences are also growing continuously[26,27]. In this urgent issue, studies mainly focus on how carbon emissions are associated with sub-regional income, normally regarded as carbon inequality, carbon emission inequality, or carbon footprint inequality[28-30]. Ogede et al. pointed out the inequality-carbon emission nexus among most sub-Saharan African countries[31]. Owen and Barrett[32] discussed the low-carbon policy costs for normal household expenditure in the UK. Both
In China, the apparent carbon emissions grew from 3,152 mt in 2000 to 13,255 mt in 2021, with the Gini-based gap increasing from 0.14 to 0.25. This Gini difference will be more evident if averaging it into 30 provinces[38,39]. Therefore, while ensuring low-carbon development, how to better coordinate the differences among different sub-regions, especially the carbon emission footprint, becomes an emerging bottleneck problem that China needs to focus on to achieve carbon neutrality in the near future. In this tough background, China implemented the corresponding low-carbon pilot policy in three different batches from 2010 to 2017, mainly aiming to reduce carbon emissions through regional coordinated development. In 2010, the National Development and Reform Commission (NDRC) directly designated the first pilot province-level batch, covering Chongqing, Guangdong, Hubei, Liaoning, Shanxi, Tianjin, and Yunnan. If those pilot provincial administrative regions fail to reach their emission reduction target, the NDRC will punish them strictly from both the political and economic aspects. With this pioneering experience, the next two batches were implemented in 2012 and 2017, adding three provincial administrative regions of Beijing, Shanghai, and Hainan[40,41]. Until now, with a series of successful emission reduction experiences, this low-carbon pilot policy has been extended across the country gradually, including ten provincial administrative regions, 65 prefecture-level cities, and hundreds of county-level cities (towns)[42,43].
Hence, this study explores the spatial differentiation of China’s province-level carbon emission footprint, mainly using the Gini decomposition method, the social network analysis, and the difference-in-differences estimation. The results show that the Gini gap in carbon emissions between provinces increased gradually from 2000 to 2021, accomplished by the stronger mutual connection between those central node provinces. With the help of the low-carbon pilot policy, those central node provinces will gradually use their siphoning effect to improve carbon emission status. Compared to the non-pilot provinces, those pilot provinces have a certain reduction effect on all kinds of carbon emissions. The average treatment effect for the total carbon emissions is -17.433 at a 1% significance level. Moreover, structural transformation and improvement are the ultimate goals for future sustainable growth, among which industrial rational transformation and green innovation performance play essential mechanism roles. Compared to previous studies, this study may have two contributions. (1) This study can supplement a growing research stream on the carbon emission footprint by providing insights from regional balanced development. Although most studies have analyzed the overall development trend of carbon emissions, the sub-regional differences still need in-depth discussion. With the help of social network analysis, this study can discuss spatial differentiation deep across different provinces; and (2) This study can supplement how a national pilot policy can affect and promote regional energy transition. In addition to the Gini-based carbon emission footprint gap, this study also evaluates the emission reduction effect of China’s low-carbon pilot policy, which will help accelerate the speed and efficiency of carbon neutrality.
This study is as follows. Section "THEORETICAL ANALYSIS" provides the theoretical analysis. Section "RESEARCH DESIGN" describes the research design. Section "RESULTS" provides three kinds of analysis results. Section "CONCLUSION AND POLICY IMPLICATION" contains the concluding remarks and policy implications. Additional figures omitted from the main text are contained in the Supplementary Material.
THEORETICAL ANALYSIS
Policy background
China has achieved remarkable economic success in the past forty years, with coal as the main energy source. However, due to this extensive growth model, China has contributed nearly 25% of global carbon emissions since 2007, making environmentally friendly development an urgent priority[44-46]. To develop a low-carbon economy and mitigate climate change problems, China formally adopted the Resolution on Actively Responding to Climate Change at the Standing Committee of the National People’s Congress in 2009. Subsequently, China implemented the corresponding low-carbon pilot policy in three different batches from 2010 to 2017, mainly aiming to reduce carbon emissions from the economic structure[47,48].
As for the first batch in 2010, the NDRC directly designated the pilot provinces without advance information, requiring them to finish the emission reduction target in multiple ways. Those pilot provincial administrative regions, including Tianjin, Chongqing, Guangdong, Hubei, Liaoning, Shanxi, and Yunnan, have to achieve a certain degree of carbon emission reduction without any anticipation preparation. In this first batch, the NDRC mainly focuses on establishing and promoting the low-carbon economic framework at the provincial administrative level[49,50]. With this pioneering experience, the NDRC then allowed other regions to apply for low-carbon pilots in the latter two batches implemented in 2012 and 2017, including three provincial administrative regions (Beijing, Hainan, and Shanghai), several prefecture-level cities, and some county-level cities (towns). In these two batches, the NDRC wants to expand the low-carbon economic framework designed in the first batch to the whole national level by gradually refining it from the province-level administrative region to the prefecture-level and county-level[43,51,52]. In this sense, those pilot provincial administrative regions still play an essential role in implementing this low-carbon pilot policy. Without the prefecture-level framework, those subsequent pilot prefecture-level and county-level regions cannot advance this low-carbon pilot policy in an orderly manner. This is also the main reason why we conduct the analyses from the perspective of the provincial administrative regions in the study[53-55].
In addition, this low-carbon pilot policy has two advantages compared to other environmental regulations[40,56,57]. First, it is a comprehensive environmental regulation. Since the NDRC does not provide specific measures to reduce emissions, each pilot region can make emission reduction plans according to its own development situation. Therefore, this pilot policy can incorporate different types of environmental regulations. Second, it is a weak constraint and strong governance regulation. Besides submitting an overall plan to the NDRC, the pilot regions have the right to decide how to reduce emissions and develop native energy. However, the NDRC can also manage and regulate pilot regions through its effective administrative powers if they do not accomplish the established target.
Literature review
As an important reflection of sustainable development, carbon emission footprint has been highly documented and recognized by countries worldwide in all environment-related fields, among which most studies focus on its concept scopes, measurement methods, and influencing factors[10,13,15].
Since the introduction of the carbon emission footprint, studies on its definition and scope have continued till now. Although there is still no common sense about its unified definition, the general understanding is usually expressed as a measure of weight in a certain “unit” of carbon emissions[58,59]. Without loss of generality, the carbon emission footprint can be reflected by the carbon emissions equivalent per year in some research[60,61]. Following this framework, numerous studies have assessed various types of carbon emission footprints at the national, provincial, city, and product levels, providing many constructive suggestions for global carbon reduction and eco-friendly development[62-64]. Wiedmann and Minx[65] pointed out that, except for its concept and term used in research, the carbon emission footprint is also a widely acknowledged pathway in the public debate on addressing the threat of global climate change. Academically, since the carbon emission footprint exists in every economic activity, the Organization for Economic Co-Operation and Development[66,67] proposed “carbon decoupling” to support sustainable economic transition effectively. Regarded as the state in which economic growth is separated from energy consumption, carbon decoupling can further alleviate the convergence problem of high carbon intensity in industrial economic growth[68,69]. In this sense, how to measure carbon emission footprint becomes relatively necessary and important.
Currently, three classical methods are widely used to assess carbon emission footprint: the Intergovernmental Panel on Climate Change method, the input-output method, and the life cycle assessment[18]. The Intergovernmental Panel on Climate Change method, as an application of the carbon emission factor method, has become one of the most accepted guidelines for calculating macro-national carbon emission footprint top-down[70,71]. To some extent, this method can accurately measure different types of carbon emission footprints in various activities, combining information on human activity and quantified emissions per unit[72]. In different countries, sectors, and energy types, the carbon emission factor used for standard coal transformation is not the same, with a larger self-decision-making right within a certain range allowed according to the real emission situation[73]. Many scholars can use it as a classical method in various fields. Azarkamand et al. developed a new standard tool to calculate carbon footprint in ports, which is a creative practice and endeavor[74]. Foo and Tan even used it in carbon-constrained energy planning and carbon emission pinch analysis[75].
By comparison, the input-output method and the life cycle assessment calculate the carbon emission footprint from the overall economic process and the individual product cycle[76,77]. Based on the historical input and output data, the input-output method can systematically embed carbon emission footprint into each economic activity, following the “one ton of carbon emission equals one ton” theory (carbon eternal theory)[78]. In recent emerging studies, the carbon emission footprint inventory has aroused a lot of attraction, especially in the tourism and building fields. Abbood et al. quantified the carbon activities in the US manufacturing sector, with international trade considered in a multi-region input-output life cycle assessment framework[79]. Both Demeter et al.[80] and Sun et al.[81] extended the input-output model to compile tourism sectoral emissions. In addition to carbon emission tracking and assessment, Sheng et al. also used the input-output method for pure statistical carbon accounting from a multi-perspective of data[82], topics, and applications. Similar to the input-output method, the life cycle assessment analyzes the carbon emission footprint from a specific economic activity or product using the same carbon eternal theory[83]. Based on this method, Kanemoto et al. mapped the US carbon emission footprints across several states[84]; Li et al. evaluated the emission footprint of electric vehicle batteries[85]; Farzaneh and Jung pointed out that electrification can lead to about a 22.6% decrease in carbon emission footprint in Florida[86].
With relatively accurate measurement, analyzing and predicting the future carbon emission footprint is important in achieving balanced development[87]. Regarding influencing factors, economic growth, population activities, energy structure, imported trade, and foreign direct investment are the main driving items for the domestic carbon emission footprint[88,89]. The “Impact = Population * Affluence * Technology” model, proposed by Ehrlich and Holdren, was the first formulaic method in exploring the influencing factors of environmental impacts[90,91]. After that, the “ln (Impact) = ln (Population) + ln (Affluence) + ln (Technology) + ln (Errors)” model further used the natural logarithm form to promote this framework[92,93]. With this systematic measurement, studies can link various socio-economic activities to carbon emission footprint at the statistical level, thus helping to calculate the current and future trends more intuitively[94-96]. In addition, on more accurate carbon emission footprint predictions, the logarithmic mean Divisia index decomposition approach[97-99], the three-stage least squares structural model[100,101], the computable general equilibrium method[102-104], and other systems engineering methods have been gradually applied in different fields[105,106].
Nevertheless, some emerging issues also need to be discussed and addressed, except for the above concept scopes, measurement methods, and influencing factors. With the increasing attention on the socio-economic network, studies have explored how carbon emission is generated and transferred, accompanied by its adverse effects[10]. To some extent, the carbon-related socio-economic network combines measurement methods and influencing factors, considering both the static emission measurement and the dynamic agent correlation. At the macro level, Gao et al. analyzed carbon emission footprint changes in Chinese large-scale population migration[107]. At the medium level, Zhang et al. investigated whether the digital economy can reduce carbon emissions[108]. At the micro level, Yin and Shi assessed Chinese residents’ low-carbon consumption behaviors[109]. Therefore, since the socio-economic network provides many social, economic, and structural relationships at different levels, figuring out the role and effect of carbon emission becomes relatively essential, especially during the global economic downturn and energy transition period after the COVID-19 shocks.
RESEARCH DESIGN
Sample data
This study compiles a panel dataset of China’s apparent province-level carbon emission data from 2000 to 2021, accompanied by some necessary socio-economic data such as gross domestic product, total population, foreign direct investment, etc. There are several reasons for using this sample data. (1) Based on the Carbon Emission Accounts and Datasets (CEADs), this study obtains the latest province-level carbon emission data from 1997 to 2021[110-114]. Considering the advantages of long-time series data, especially in uncovering and revealing the phenomenon law, this study chooses the beginning year of 2000; and (2) As for the missing values in the sample data, the predictive mean matching and the linear interpolation methods are applied at the same time[115]. Table 1 shows the descriptive statistics.
Descriptive statistics
Var | Definition | Obs | Mean | SD | Min | Max | Source |
CEi,t | Carbon emissions (apparent) | 660 | 5.27 | 0.98 | 0.81 | 7.64 | CEADs |
COALi,t | Raw coal emissions (apparent) | 660 | 4.93 | 1.03 | 0.00 | 7.62 | CEADs |
OILi,t | Crude oil emissions (apparent) | 660 | 3.02 | 2.16 | 0.00 | 6.12 | CEADs |
GASi,t | Natural gas emissions (apparent) | 660 | 1.42 | 2.17 | 0.00 | 4.14 | CEADs |
CEMi,t | Cement emissions (apparent) | 660 | 2.34 | 1.13 | 0.00 | 4.03 | CEADs |
GDPi,t | Gross domestic product | 660 | 9.14 | 1.17 | 5.57 | 11.73 | CSMAR |
AGDPi,t | Gross domestic product per capita | 660 | 10.18 | 0.87 | 7.92 | 12.14 | CSMAR |
STRi,t | Secondary industry product | 660 | 6.75 | 1.16 | 3.69 | 8.70 | CSMAR |
ENEi,t | Coal consumption proportion | 660 | 0.73 | 0.14 | 0.00 | 0.94 | CSMAR |
FDIi,t | Foreign direct investment | 660 | 10.64 | 1.63 | 6.36 | 15.33 | CSMAR |
INTi,t | Import trade | 660 | 16.11 | 1.91 | 10.73 | 20.03 | CSMAR |
EXTi,t | Export trade | 660 | 16.34 | 1.81 | 11.63 | 20.47 | CSMAR |
POPi,t | Total population | 660 | 8.17 | 0.76 | 6.25 | 9.45 | CSMAR |
STi,t | Industrial rational index | 660 | 1.07 | 0.36 | 0.23 | 2.55 | CSMAR |
GPi,t | Green patent | 660 | 4.29 | 1.73 | 0.00 | 9.62 | CSMAR |
OFDIi,t | Outward foreign direct investment | 660 | 15.31 | 6.39 | 0.00 | 30.81 | CSMAR |
Evaluation method
Gini decomposition method
As a common measure of economic inequality, the Gini index reflects the income gap between the real income distribution curve and the absolute average curve[116,117]. As shown in Eq. (1), under the definite integral method, the Gini index equals the weighted average sum of the differences between the cumulative total population proportion
Following this decomposition method, this study calculates the Gini-based carbon emission footprint index at the province level in Eq. (2). As for a certain year t of the sample period, this Gini-based index (GICi,t) is equal to the weighted average sum of the differences between the cumulative total population proportion
Compared to other economic inequality indices, such as the Theil index, the Wolfson polarization index, and the World Bank inequality index, this Gini index has several distinct advantages in the carbon emission decomposition, especially in regional comparison. First, the Gini index is more convenient for measuring carbon emission differences, considering its straightforward calculation. Using the Lorenz curve framework, He et al. calculate the inequality in carbon emission allowances, revealing the seriously aggravated misallocation in the Chinese provincial administrative regions[120]. Teng et al. constructed the carbon Gini index to measure inequality in climate change areas[121]. They both pointed out that the Gini-based carbon index effectively measures inequality in the distribution of carbon space among different regions. Second, the Gini index is more intuitional for the overall and partial comparison, with effective and classified illustration in the inequality degree. Studies around this issue have documented that carbon inequality differs in character within various national and sub-national scales[122,123]. Clarke-Sather et al. found that the inequality of Chinese interprovincial carbon emissions is slightly lower than that of income inequality[124]. Third, the Gini index is much more universal for common understanding, especially in a new application field. From a comparative perspective, Hou et al. figured out how to decouple economic growth from carbon emissions in terms of income inequality[38]. Jorgenson et al. explored the causal relationship between consumption-driven carbon emission differences and the Gini-based domestic income gap among
Social network analysis
As a form of social structure, the social network is a relatively stable relationship system connected by individual (organization) interaction[126]. In the social network analysis, the individual (organization) is the social node, and the mutual relationship is the social network. With various mutual relationships making up social networks at different levels, social network analysis focuses more on the mutual relationships between nodes and the socio-economic effects among nodes[127]. Following this framework, this study constructs a province-level carbon emission footprint spatial correlation network.
In Eqs. (3)-(5), i, j, and t represent the node province, the other node province, and the year, respectively. As for a certain year t of the sample period: SPi,j,t represents the mutual network relationship between the node province i and the node province j; Ki,j,t represents the carbon emission proportion of the node province i among the overall mutual carbon emissions; Di,j,t represents the weighted average spatial correlation between the node province i and the node province j; DISTi,j,t represents the spherical distance between the node province i and the node province j; CEi,t represents the carbon emission of the node province i; GDPi,t represents the gross domestic product of the node province i; AGDPi,t represents the gross domestic product per capita of the node province i; and POPi,t represents the total population of the node province i.
In Eq. (6), the mutual spatial network relationship (SPi,j,t) is converted into an absolute value matrix (SPt). According to the general definition, for a certain node province i and node province j in a certain year t, if their mutual network value (SPvalue) is larger than the average value of the whole matrix row, then the average mutual network relationship will be set to 1. As shown in Eq. (7), the average dummy value matrix
First, for the global network characteristics, network density, network connectedness, network hierarchy, network efficiency, network graph clustering coefficient, network hybrid reciprocity, network non-vacuous transitive ordered triples, network average distance, network compactness distance, and network breadth distance will be calculated, respectively. These indices, to a certain extent, can describe the overall characteristics of the global network from scale, rank, and structure[128,129]. Take network density, network hierarchy, and network efficiency as examples.
In Eq. (8), network density (DENt) represents the carbon emission footprint intensity. Mi,j,t is the number of network connections between two provinces, and N is the number of connected nodes. In Eq. (9), network hierarchy (HIEt) represents the network status of the node provinces. Vi,j,t is the number of unreachable node relations, and MAX(Vi,j,t) is the maximum number of unreachable node relations. In Eq. (10), network efficiency (EFFt) represents the redundant connections of node provinces. Wi,j,t is the number of redundant node relations, and MAX(Wi,j,t) is the maximum number of redundant node relations.
Second, regarding node network characteristics, this study focuses on degree centrality, closeness centrality, and betweenness centrality in particular. As the most direct reflection of node status, the three indices describe the connection numbers, the shortest connected lengths, and the shortest connected frequencies of a certain node province i, respectively[130,131].
In Eq. (11), degree centrality (DCi,t) represents the outward direct connection numbers of a certain node province i. Mi,j,tis the direct connection numbers, and N is the number of connected nodes. In Eq. (12), closeness centrality (CCi,t) represents the average distance of the outward shortest connected lengths of a certain node province i. L(i, j) is the shortest length between a certain node province i and another node province j. In Eq. (13), betweenness centrality (BCi,t) represents the average percentage of all the outward shortest connected lengths that pass through the certain node province i. LM(k, j) is the number of the shortest length between a third-party node province k and another node province j, and LM(i, k, j) is the number of the shortest length between the third-party node province k and another node province j that must pass through the certain node province i.
Third, in terms of the dynamic network characteristics (mutual non-redundant relations between nodes), the structural hole is the essential index in assessing node dynamic competitive advantage[132]. As for the node provinces, those possessing structural holes will have better advantages in handling redundant relationships with other nodes, thus stimulating their own network competitiveness[133]. In this framework, this study estimates structural hole scale, structural hole efficiency, structural hole constraints, and structural hole hierarchy. As for node province i, all connected node provinces will be set to j, and all third-party node provinces will be set to k.
In Eq. (14), the structural hole scale (HSi,t) equals the network scale minus network redundancy. pik is the proportion that the node province i used for connecting the third-party node provinces k. mjk is the marginal intensity that the node province j used for connecting the third-party node provinces k. In
Difference-in-differences model
Since China’s low-carbon pilot policy has three different batches, this study employs the staggered DID model to observe how it works for carbon emission reduction[134]. The specific empirical model is set as follows in Eq. (18), where i and t represent the province and the year, respectively.
CEi,t is the dependent variable, referring to the apparent carbon emissions of province i in the year t. LCCi,t is the independent variable (as dummy variable form), referring to the implementation of the low-carbon pilot policy. The value will be set to 1 if the province i is on the low-carbon pilot list in the year t. θ is the estimated coefficient, referring to the overall average treatment effect of the low-carbon pilot policy on carbon emission reduction. Xi,t is a set of control variables, with β as their corresponding coefficients. fi,t is the potential province-fixed and year-fixed effects. α is the constant term. εi,t is the remaining residuals.
As illustrated in the policy background section, the first batch of the low-carbon pilot policy mainly targets seven provincial administrative regions. With this pioneering experience, the latter two batches then expand the low-carbon pilot policy from the province-level administrative region to the prefecture-level and county-level, with the low-carbon economic framework designed in the first batch. Therefore, the pilot provincial administrative regions still play an essential role in implementing this low-carbon pilot policy. Those subsequent pilot prefecture-level and county-level regions cannot advance this low-carbon pilot policy in an orderly manner if the prefecture-level framework is lacking. Considering this crucial reason, we set the independent variable at the provincial administrative region level in Eq. (18)[43,51,52].
RESULTS
Gini decomposition results
Overall analyses
Figure 1 shows the Gini decomposition results of China’s apparent province-level carbon emissions from 2000 to 2021. The horizontal axis represents the year, the left vertical axis represents the stack area plot value, and the right vertical axis represents the line plot value. Regarding carbon emission composition, the total Gini-based carbon emission footprint index can be divided into four aspects: raw coal, crude oil, natural gas, and cement. The line plot with marked points expresses the total Gini-based carbon emission footprint index. The stack area plots express the Gini-based carbon emission gap in those four sub-indices from bottom to top in an orderly fashion.
Figure 1. The total Gini-based carbon emission footprint index. It shows the Gini decomposition results of China’s apparent province-level carbon emissions from 2000 to 2021. The horizontal axis represents the year, the left vertical axis represents the stack area plot value, and the right vertical axis represents the line plot value. The total Gini-based carbon emission footprint index can be divided into four aspects: raw coal, crude oil, natural gas, and cement. The line plot with marked points expresses the total Gini-based carbon emission footprint index. The stack area plots express the Gini-based carbon emission gap in those four sub-indices from bottom to top in an orderly fashion.
In terms of the total Gini-based carbon emission footprint index, as the line plot goes, it shows an overall growth trend from 2000 to 2021, indicating the increasing Gini gap in carbon emissions between provinces. From 2000 to 2007, the total Gini-based carbon emission footprint index has a clear downward trend, falling from 0.14 to 0.11, with a maximum decline larger than 16%. After a positive promotion to 0.14 in 2008, it kept a relatively stable growth development from 2009 to 2012. Since then, the index has been on a roll to 0.25 around 2020, with a considerable annual growing trend at 5%.
In terms of the four sub-indices, as the stack area plots show, to some extent, they exhibit two distinct types of developmental characteristics from 2000 to 2021, suggesting two contradictory Gini gaps in carbon emissions. On the one hand, raw coal and crude oil sub-indices maintain a continuous growth trend, increasing from 0.04 to 0.08 and from 0.14 to 0.16, respectively. On the other hand, natural gas and cement sub-indices have been falling constantly, with the decline from 0.26 to 0.06 and from 0.04 to 0.02, respectively. By comparison, although the Gini gaps in natural gas and cement become narrow from 2000 to 2021, they can not make up for the increasingly considerable Gini differences in raw coal and crude oil, thus leading the growth trend for the total Gini-based carbon emission footprint index. In sum, the Gini gap in carbon emissions becomes larger across provinces from 2000 to 2021, with raw coal and crude oil being the main causes.
Comparative analyses
Figure 2 shows the total Gini-based carbon emission footprint index among the pilot and non-pilot provinces. The horizontal axis represents the year, the left vertical axis represents the stack area plot value, and the right vertical axis represents the line plot value. The line plot with marked points expresses the total Gini-based carbon emission footprint index. The stack area plots express the Gini-based carbon emission gap in the pilot and non-pilot provinces from bottom to top. In a statistical sense, the Gini-based carbon emission footprint index in non-pilot provinces is almost twice as large as that of pilot provinces, revealing the relatively balanced growth trend in carbon emissions across pilot provinces. As the main policy implementers, those pilot provinces must finish the targeted emission reduction goals after 2010. If failed, they will be punished strictly by the NDRC.
Figure 2. Gini-based carbon emission footprint index among different provinces. It shows the total Gini-based carbon emission footprint index among the pilot and non-pilot provinces. The horizontal axis represents the year, the left vertical axis represents the stack area plot value, and the right vertical axis represents the line plot value. The line plot with marked points expresses the total Gini-based carbon emission footprint index. The stack area plots express the Gini-based carbon emission gap in the pilot and non-pilot provinces from bottom to top.
In Figure 3, the stack waterfall plots further show the four sub-indices among the pilot and non-pilot provinces. The horizontal axis represents the Gini decomposition value, and the middle vertical axis represents the year. As for the pilot provinces on the left side, except for the raw coal, the other three sub-indices have shown a shrinking development trend. These phenomena indicate that the pilot provinces have better governance in crude oil, natural gas, and cement emissions. As for the non-pilot provinces on the right side, the development trends of the four sub-indices are the same as the total situation in Figure 1. While the raw coal and crude oil sub-indices keep a growth trend, the natural gas and cement sub-indices present the decline characteristics. To sum up, it is evident that China’s low-carbon pilot policy has a positive effect on narrowing the province-level gap in crude oil emissions, to some extent in the statistical sense of the Gini decomposition.
Social network analysis results
Figure 4 shows China’s province-level carbon emission footprint spatial network in 2000, 2010, and 2021. Regarding the overall social network structure, its directed network level has undergone obvious changes. On the one hand, the mutual connection between node provinces increases as the overall social network structure becomes tighter. On the other hand, the central node province is also constantly changing. In 2000, the central node provinces were the Anhui, Fujian, Hunan, and Tianjin. In 2010, Anhui, Chongqing, Guangxi, Liaoning, and Tianjin became the central ones. In 2021, the central node provinces changed to Guangxi, Liaoning, Shandong, and Tianjin.
Global network characteristics
Table 2 shows the global network characteristics of China’s province-level carbon emission footprint from 2000 to 2021, containing four aspects totaling 11 indices. First, the network scale shows a relatively stable trend, with the network density (DENt) around 0.16-0.17 and standard deviation (SD) around 0.3. It shows that there are no obvious mutual relationship changes within the current carbon emission footprint spatial network. Second, the network level increases slightly, especially in the network hierarchy. While network connectedness (CONt) and network efficiency (EFFt) remain relatively stable, the network hierarchy (HIEt) changes from 0.067 in 2000 to 0.151 in 2021 (the maximum value is 0.189 in 2020). These dynamic changes in network hierarchy indicate progressively increasing inner network layers. Regarding the relatively stable network scale and the increased network level, those node provinces in the inner network center will have more outward mutual connections.
Global network characteristics
Year | Scale | Level | Interaction | Distance | |||||||
DENt | SD | CONt | HIEt | EFFt | GRAt | RECt | TRIt | NADt | NCDt | NBDt | |
2000 | 0.175 | 0.380 | 1.000 | 0.067 | 0.727 | 0.213 | 0.086 | 170 | 2.256 | 0.506 | 0.494 |
2001 | 0.167 | 0.373 | 1.000 | 0.067 | 0.754 | 0.235 | 0.124 | 177 | 2.386 | 0.488 | 0.512 |
2002 | 0.164 | 0.371 | 1.000 | 0.067 | 0.741 | 0.210 | 0.067 | 162 | 2.415 | 0.482 | 0.518 |
2003 | 0.164 | 0.371 | 1.000 | 0.067 | 0.746 | 0.196 | 0.083 | 153 | 2.371 | 0.487 | 0.513 |
2004 | 0.167 | 0.373 | 1.000 | 0.067 | 0.761 | 0.215 | 0.151 | 160 | 2.257 | 0.503 | 0.497 |
2005 | 0.166 | 0.372 | 1.000 | 0.067 | 0.761 | 0.237 | 0.143 | 166 | 2.229 | 0.504 | 0.496 |
2006 | 0.176 | 0.381 | 1.000 | 0.067 | 0.722 | 0.223 | 0.078 | 199 | 2.259 | 0.506 | 0.494 |
2007 | 0.175 | 0.380 | 1.000 | 0.129 | 0.734 | 0.221 | 0.110 | 189 | 2.336 | 0.484 | 0.516 |
2008 | 0.172 | 0.378 | 1.000 | 0.067 | 0.746 | 0.264 | 0.136 | 177 | 2.276 | 0.503 | 0.497 |
2009 | 0.176 | 0.381 | 1.000 | 0.067 | 0.724 | 0.181 | 0.085 | 164 | 2.420 | 0.491 | 0.509 |
2010 | 0.184 | 0.387 | 1.000 | 0.129 | 0.714 | 0.243 | 0.103 | 233 | 2.243 | 0.497 | 0.503 |
2011 | 0.168 | 0.374 | 1.000 | 0.129 | 0.759 | 0.178 | 0.150 | 158 | 2.366 | 0.477 | 0.523 |
2012 | 0.166 | 0.372 | 1.000 | 0.129 | 0.759 | 0.208 | 0.134 | 144 | 2.232 | 0.489 | 0.511 |
2013 | 0.164 | 0.371 | 1.000 | 0.129 | 0.749 | 0.246 | 0.092 | 179 | 2.287 | 0.482 | 0.518 |
2014 | 0.167 | 0.373 | 1.000 | 0.188 | 0.739 | 0.222 | 0.074 | 166 | 2.215 | 0.476 | 0.524 |
2015 | 0.163 | 0.370 | 1.000 | 0.129 | 0.754 | 0.201 | 0.101 | 146 | 2.321 | 0.478 | 0.522 |
2016 | 0.168 | 0.374 | 1.000 | 0.188 | 0.744 | 0.225 | 0.098 | 179 | 2.396 | 0.461 | 0.539 |
2017 | 0.166 | 0.372 | 1.000 | 0.129 | 0.749 | 0.252 | 0.099 | 175 | 2.319 | 0.480 | 0.520 |
2018 | 0.166 | 0.372 | 1.000 | 0.129 | 0.744 | 0.234 | 0.083 | 152 | 2.246 | 0.488 | 0.512 |
2019 | 0.166 | 0.372 | 1.000 | 0.188 | 0.749 | 0.197 | 0.099 | 138 | 2.280 | 0.469 | 0.531 |
2020 | 0.169 | 0.375 | 1.000 | 0.189 | 0.732 | 0.196 | 0.065 | 167 | 2.339 | 0.465 | 0.535 |
2021 | 0.169 | 0.363 | 1.000 | 0.181 | 0.803 | 0.153 | 0.067 | 166 | 3.022 | 0.479 | 0.621 |
Third, network interaction is a dynamic development trend. Whether it is network graph clustering coefficient (GRAt), network hybrid reciprocity (RECt), or network non-vacuous transitive ordered triples
Node network characteristics
Table 3 shows the node network characteristics of China’s province-level carbon emission footprint in 2000, 2010, and 2021, containing three indices of degree centrality (DCi,t), closeness centrality (CCi,t), and betweenness centrality (BCi,t).
Node network characteristics
Degree centrality | Closeness centrality | Betweenness centrality | |||||||
2000 | 2010 | 2021 | 2000 | 2010 | 2021 | 2000 | 2010 | 2021 | |
Beijing | 15.57 | 13.35 | 17.79 | 30.47 | 25.11 | 14.33 | 6.76 | 2.31 | 2.94 |
Chongqing | 15.57 | 33.36 | 17.79 | 37.46 | 37.83 | 34.24 | 3.71 | 31.81 | 9.24 |
Guangdong | 8.90 | 17.79 | 15.57 | 30.79 | 27.52 | 23.23 | 0.00 | 2.89 | 4.35 |
Hainan | 17.79 | 26.69 | 26.69 | 38.42 | 37.19 | 35.30 | 8.34 | 34.49 | 35.18 |
Hubei | 13.35 | 15.57 | 13.35 | 37.01 | 31.19 | 26.99 | 14.92 | 5.58 | 22.28 |
Liaoning | 24.47 | 33.36 | 33.36 | 41.90 | 42.83 | 40.96 | 45.61 | 49.99 | 29.83 |
Shaanxi | 22.24 | 20.02 | 24.47 | 37.40 | 31.73 | 29.19 | 33.29 | 12.10 | 23.26 |
Shanghai | 17.79 | 20.02 | 20.02 | 41.30 | 38.69 | 36.03 | 27.01 | 7.72 | 35.20 |
Tianjin | 44.48 | 37.81 | 35.59 | 48.37 | 45.87 | 42.20 | 35.32 | 11.25 | 19.83 |
Yunnan | 17.79 | 8.90 | 13.35 | 35.27 | 29.41 | 27.13 | 13.10 | 0.49 | 4.99 |
Average | 19.79 | 22.69 | 21.80 | 37.84 | 34.74 | 30.96 | 18.81 | 15.86 | 18.71 |
Anhui | 33.36 | 40.03 | 13.35 | 47.81 | 45.91 | 29.14 | 70.79 | 45.76 | 15.84 |
Fujian | 31.14 | 24.47 | 26.69 | 46.07 | 32.71 | 33.01 | 27.54 | 24.22 | 32.98 |
Gansu | 20.02 | 22.24 | 13.35 | 41.00 | 36.78 | 32.07 | 8.89 | 46.80 | 4.79 |
Guangxi | 31.14 | 40.03 | 40.03 | 43.54 | 45.61 | 42.02 | 25.73 | 57.77 | 73.69 |
Guizhou | 22.24 | 13.35 | 13.35 | 42.44 | 28.85 | 29.13 | 20.76 | 20.30 | 12.02 |
Hebei | 20.02 | 15.57 | 17.79 | 35.44 | 31.16 | 29.20 | 28.62 | 5.29 | 11.69 |
Heilongjiang | 22.24 | 15.57 | 20.02 | 36.90 | 32.02 | 30.95 | 13.25 | 12.93 | 26.38 |
Henan | 20.02 | 13.35 | 17.79 | 35.22 | 32.13 | 31.19 | 15.14 | 7.05 | 22.00 |
Hunan | 33.36 | 15.57 | 13.35 | 46.79 | 18.73 | 17.78 | 33.41 | 0.00 | 0.00 |
Inner Mongolia | 17.79 | 22.24 | 26.69 | 38.72 | 35.85 | 35.69 | 14.16 | 14.10 | 30.56 |
Jiangsu | 13.35 | 20.02 | 20.02 | 33.63 | 34.47 | 27.82 | 2.05 | 20.74 | 14.84 |
Jiangxi | 22.24 | 20.02 | 20.02 | 41.73 | 39.48 | 34.73 | 35.66 | 16.53 | 9.50 |
Jilin | 17.79 | 15.57 | 24.47 | 35.66 | 36.96 | 34.31 | 7.15 | 19.42 | 53.11 |
Ningxia | 4.45 | 8.90 | 8.90 | 19.35 | 17.09 | 12.65 | 0.00 | 0.00 | 0.00 |
Qinghai | 15.57 | 22.24 | 20.02 | 38.12 | 37.78 | 32.25 | 12.68 | 18.04 | 14.96 |
Shandong | 13.35 | 31.14 | 28.91 | 37.90 | 38.43 | 32.15 | 14.59 | 35.10 | 32.46 |
Shanxi | 17.79 | 13.35 | 8.90 | 38.12 | 31.52 | 24.49 | 6.81 | 1.56 | 8.76 |
Sichuan | 24.47 | 24.47 | 22.24 | 37.43 | 36.06 | 33.09 | 22.47 | 20.02 | 19.77 |
Xinjiang | 15.57 | 17.79 | 17.79 | 31.02 | 35.57 | 27.79 | 3.68 | 5.73 | 9.01 |
Zhejiang | 28.91 | 22.24 | 22.24 | 40.25 | 38.55 | 30.62 | 41.57 | 36.65 | 10.19 |
Average | 21.24 | 20.91 | 19.79 | 38.36 | 34.28 | 30.00 | 20.25 | 20.40 | 20.13 |
All average | 20.76 | 21.50 | 20.46 | 38.18 | 34.43 | 30.32 | 19.77 | 18.89 | 19.66 |
In terms of degree centrality, the average value among 30 provinces does not change significantly from 2000 to 2021. As for pilot provinces, their average value shows a slight upward trend. However, the opposite goes for the non-pilot provinces. In this sense, those pilot provinces gradually become the center of China’s carbon emission footprint spatial network. In addition to the average value changes, some development trends are also noteworthy. First, provinces like Beijing, Hebei, and Jilin keep stable network positions throughout the sample period. Second, provinces like Hainan, Inner Mongolia, and Ningxia are making concerted efforts to improve their network position. Third, provinces like Chongqing, Jiangsu, Qinghai, and Shandong are engaged in fierce competition with each other. In the last, provinces like Tianjin and Hunan are experiencing a downward growth trend.
In terms of closeness centrality, the average value among 30 provinces goes down throughout the whole sample period, both in pilot and non-pilot provinces. In terms of betweenness centrality, the average value among 30 provinces remains relatively stable, with a slight dynamic change only in pilot provinces. Combined with the average variation of closeness centrality and betweenness centrality, it will be evident that those non-pilot provinces have more internal motivations for the network central position. With the competition in non-pilot provinces being more intense than that in pilot provinces, node provinces will correspondingly reduce the connection with other competitive provinces, thus consolidating the existing network position.
Dynamic network characteristics
Table 4 shows the dynamic network characteristics of China’s province-level carbon emission footprint in 2000, 2010, and 2021, containing three indices of structural hole efficiency (HFi,t), structural hole constraints (HCi,t), and structural hole hierarchy (HHi,t).
Dynamic network characteristics
Efficiency (HFi,t) | Constraint (HCi,t) | Hierarchy (HHi,t) | |||||||
2000 | 2010 | 2021 | 2000 | 2010 | 2021 | 2000 | 2010 | 2021 | |
Beijing | 0.857 | 0.778 | 0.758 | 0.339 | 0.400 | 0.376 | 0.054 | 0.095 | 0.070 |
Chongqing | 0.786 | 0.740 | 0.688 | 0.377 | 0.237 | 0.421 | 0.071 | 0.061 | 0.113 |
Guangdong | 0.688 | 0.555 | 0.786 | 0.747 | 0.419 | 0.325 | 0.195 | 0.023 | 0.039 |
Hainan | 0.738 | 0.735 | 0.857 | 0.447 | 0.421 | 0.325 | 0.107 | 0.052 | 0.123 |
Hubei | 0.657 | 0.743 | 0.767 | 0.308 | 0.226 | 0.221 | 0.064 | 0.042 | 0.058 |
Liaoning | 0.760 | 0.648 | 0.711 | 0.308 | 0.378 | 0.309 | 0.081 | 0.046 | 0.041 |
Shaanxi | 0.757 | 0.661 | 0.692 | 0.340 | 0.354 | 0.347 | 0.100 | 0.050 | 0.092 |
Shanghai | 0.765 | 0.749 | 0.724 | 0.180 | 0.213 | 0.216 | 0.037 | 0.061 | 0.057 |
Tianjin | 0.695 | 0.594 | 0.736 | 0.392 | 0.749 | 0.505 | 0.073 | 0.087 | 0.142 |
Yunnan | 0.857 | 0.778 | 0.758 | 0.339 | 0.400 | 0.376 | 0.054 | 0.095 | 0.070 |
Average | 0.745 | 0.689 | 0.747 | 0.382 | 0.377 | 0.338 | 0.087 | 0.057 | 0.082 |
Anhui | 0.760 | 0.737 | 0.667 | 0.216 | 0.199 | 0.463 | 0.046 | 0.048 | 0.037 |
Fujian | 0.694 | 0.702 | 0.681 | 0.252 | 0.317 | 0.294 | 0.055 | 0.089 | 0.054 |
Gansu | 0.572 | 0.745 | 0.611 | 0.375 | 0.326 | 0.487 | 0.074 | 0.055 | 0.063 |
Guangxi | 0.778 | 0.794 | 0.772 | 0.248 | 0.197 | 0.197 | 0.073 | 0.052 | 0.053 |
Guizhou | 0.690 | 0.903 | 0.667 | 0.339 | 0.320 | 0.454 | 0.064 | 0.111 | 0.016 |
Hebei | 0.674 | 0.781 | 0.676 | 0.395 | 0.260 | 0.283 | 0.139 | 0.054 | 0.027 |
Heilongjiang | 0.827 | 0.607 | 0.719 | 0.290 | 0.416 | 0.365 | 0.037 | 0.080 | 0.073 |
Henan | 0.714 | 0.634 | 0.794 | 0.317 | 0.449 | 0.311 | 0.069 | 0.066 | 0.055 |
Hunan | 0.839 | 0.905 | 0.719 | 0.280 | 0.286 | 0.358 | 0.053 | 0.039 | 0.041 |
Inner Mongolia | 0.733 | 0.592 | 0.667 | 0.245 | 0.479 | 0.434 | 0.082 | 0.061 | 0.038 |
Jiangsu | 0.694 | 0.623 | 0.788 | 0.373 | 0.360 | 0.263 | 0.034 | 0.075 | 0.084 |
Jiangxi | 0.625 | 0.662 | 0.753 | 0.516 | 0.356 | 0.310 | 0.054 | 0.058 | 0.064 |
Jilin | 0.695 | 0.672 | 0.656 | 0.334 | 0.356 | 0.366 | 0.075 | 0.042 | 0.057 |
Ningxia | 0.750 | 0.778 | 0.629 | 0.345 | 0.368 | 0.305 | 0.041 | 0.073 | 0.057 |
Qinghai | 0.500 | 0.625 | 0.688 | 1.125 | 0.684 | 0.695 | 0.000 | 0.168 | 0.072 |
Shandong | 0.776 | 0.646 | 0.611 | 0.399 | 0.321 | 0.377 | 0.043 | 0.092 | 0.033 |
Shanxi | 0.667 | 0.695 | 0.769 | 0.443 | 0.258 | 0.254 | 0.077 | 0.044 | 0.068 |
Sichuan | 0.590 | 0.536 | 0.875 | 0.417 | 0.545 | 0.406 | 0.065 | 0.049 | 0.055 |
Xinjiang | 0.678 | 0.740 | 0.654 | 0.306 | 0.291 | 0.344 | 0.076 | 0.038 | 0.073 |
Zhejiang | 0.633 | 0.556 | 0.758 | 0.408 | 0.444 | 0.369 | 0.077 | 0.096 | 0.040 |
Average | 0.697 | 0.694 | 0.708 | 0.375 | 0.361 | 0.364 | 0.060 | 0.069 | 0.052 |
All average | 0.711 | 0.692 | 0.720 | 0.377 | 0.366 | 0.357 | 0.068 | 0.066 | 0.061 |
Regarding structural hole efficiency (HFi,t), the average value among 30 provinces fluctuates down and up (decrease-increase) from 2000 to 2021, where those pilot provinces perform better than the non-pilot provinces. Since structural hole efficiency equals the ratio of effective network scale to actual network scale, this comparative result shows that pilot provinces have better abilities in handling invalid redundant relationships, thus improving the main effective mutual node connections. Regarding structural hole constraints (HCi,t), the average value among 30 provinces goes down throughout the whole sample period, both in pilot and non-pilot provinces. With the gradual reduction in structural hole constraints, node provinces will normally have more socio-economic resource connections with the neighboring node provinces. Regarding structural hole hierarchy (HHi,t), the average value among 30 provinces also decreases throughout the sample period. However, those pilot provinces present a decrease-increase trend, while non-pilot provinces show an increase-decrease one. For example, with increasing structural hole efficiency and decreasing structural hole constraints, Guangdong, Hubei, and Jiangsu have become progressively important in the carbon emission footprint spatial network. This kind of “resource-bridge” role can give them a more structural hole hierarchy in the overall network position, thus obtaining better development opportunities.
Inter-provincial dynamic interaction
After considering global network, node network, and dynamic network characteristics, we further analyze how those central node provinces interact with other non-central ones. According to Figure 4, we set three different baseline conditions: the 2000, 2010, and 2021 cases. Figure 5 shows the average prediction networks for the next five years. Table 5 shows the average prediction value of degree centrality (DCi,t), betweenness centrality (BCi,t), and structural hole efficiency (HFi,t) for the next five years.
Inter-provincial dynamic interaction
Centrality (DCi,t) | Betweenness (BCi,t) | Efficiency (HFi,t) | |||||||
Baseline year | 2000 | 2010 | 2021 | 2000 | 2010 | 2021 | 2000 | 2010 | 2021 |
Beijing | 9.483 | 18.966 | 6.897 | 0.767 | 0.595 | 0.200 | 0.461 | 0.337 | 0.405 |
Chongqing | 8.621 | 70.690 | 9.483 | 0.543 | 19.320 | 0.003 | 0.496 | 0.688 | 0.467 |
Guangdong | 6.897 | 22.414 | 6.897 | 0.003 | 0.476 | 0.333 | 0.438 | 0.255 | 0.365 |
Hainan | 10.345 | 27.586 | 10.345 | 0.619 | 3.190 | 1.500 | 0.481 | 0.408 | 0.494 |
Hubei | 8.621 | 18.966 | 8.621 | 0.500 | 0.810 | 0.733 | 0.478 | 0.321 | 0.465 |
Liaoning | 12.931 | 77.586 | 38.793 | 2.110 | 19.320 | 24.893 | 0.452 | 0.701 | 0.759 |
Shaanxi | 12.069 | 24.138 | 9.483 | 2.900 | 1.310 | 1.200 | 0.516 | 0.306 | 0.420 |
Shanghai | 9.483 | 24.138 | 8.621 | 1.910 | 0.762 | 0.333 | 0.550 | 0.371 | 0.436 |
Tianjin | 41.379 | 81.034 | 41.379 | 15.960 | 19.320 | 24.893 | 0.758 | 0.706 | 0.767 |
Yunnan | 10.345 | 15.517 | 6.034 | 1.133 | 0.005 | 0.200 | 0.447 | 0.250 | 0.382 |
Average | 13.017 | 38.104 | 14.655 | 2.644 | 6.510 | 5.429 | 0.508 | 0.434 | 0.496 |
Anhui | 37.069 | 79.310 | 6.034 | 15.960 | 19.32 | 0.367 | 0.771 | 0.706 | 0.425 |
Fujian | 38.793 | 27.586 | 6.897 | 15.960 | 2.435 | 0.767 | 0.717 | 0.383 | 0.476 |
Gansu | 11.207 | 24.138 | 9.483 | 0.876 | 2.077 | 1.000 | 0.450 | 0.373 | 0.449 |
Guangxi | 13.793 | 79.310 | 37.069 | 4.038 | 19.320 | 24.893 | 0.544 | 0.706 | 0.782 |
Guizhou | 11.207 | 18.966 | 7.759 | 1.000 | 0.667 | 0.002 | 0.412 | 0.423 | 0.502 |
Hebei | 11.207 | 20.690 | 7.759 | 3.410 | 1.071 | 0.200 | 0.598 | 0.292 | 0.343 |
Heilongjiang | 12.069 | 18.966 | 8.621 | 2.762 | 0.810 | 1.200 | 0.476 | 0.265 | 0.435 |
Henan | 11.207 | 18.966 | 5.172 | 2.333 | 1.190 | 0.002 | 0.526 | 0.342 | 0.392 |
Hunan | 38.793 | 20.690 | 6.897 | 15.960 | 0.000 | 0.003 | 0.75 | 0.286 | 0.346 |
Inner Mongolia | 9.483 | 25.862 | 9.483 | 1.333 | 1.143 | 1.167 | 0.457 | 0.314 | 0.441 |
Jiangsu | 8.621 | 24.138 | 7.759 | 0.143 | 1.310 | 0.200 | 0.434 | 0.334 | 0.357 |
Jiangxi | 11.207 | 20.690 | 9.483 | 2.300 | 0.929 | 1.710 | 0.516 | 0.313 | 0.462 |
Jilin | 10.345 | 18.966 | 12.069 | 1.252 | 1.952 | 2.533 | 0.442 | 0.429 | 0.455 |
Ningxia | 5.172 | 15.517 | 10.345 | 0.003 | 0.003 | 0.002 | 0.364 | 0.272 | 0.405 |
Qinghai | 8.621 | 24.138 | 10.345 | 1.595 | 1.625 | 1.567 | 0.466 | 0.348 | 0.451 |
Shandong | 7.759 | 32.759 | 31.034 | 0.743 | 3.595 | 24.893 | 0.403 | 0.407 | 0.763 |
Shanxi | 10.345 | 18.966 | 7.759 | 0.200 | 0.003 | 0.600 | 0.386 | 0.187 | 0.391 |
Sichuan | 12.931 | 27.586 | 12.931 | 4.010 | 1.786 | 3.376 | 0.505 | 0.377 | 0.509 |
Xinjiang | 9.483 | 22.414 | 12.931 | 0.910 | 0.500 | 2.867 | 0.467 | 0.232 | 0.494 |
Zhejiang | 14.655 | 24.138 | 9.483 | 7.243 | 1.167 | 1.376 | 0.524 | 0.343 | 0.447 |
Average | 14.698 | 28.190 | 11.466 | 4.101 | 3.045 | 3.436 | 0.510 | 0.367 | 0.466 |
All average | 14.138 | 31.494 | 12.529 | 3.616 | 4.200 | 4.100 | 0.510 | 0.389 | 0.476 |
As can be seen in Figure 5, the average prediction network structure becomes stable and dense for the next five years, regardless of different baseline conditions. For the whole network density (DENt), it has increased nearly fourfold than the original baseline years: from 0.175 to 0.479 in 2000, from 0.184 to 0.556 in 2010, and from 0.169 to 0.436 in 2021. These considerable changes indicate that the whole carbon emission footprint spatial network will gradually mature with the increasing inter-provincial dynamic interactions. In addition, although those central node provinces play an essential role in maintaining and improving network structure, they actually also produce a certain central siphon effect. Within the prediction network, the spatial network relationship of the central circle layer intensifies more than the outer circle layer.
In Table 5, the most noticeable performance is that the siphoning effect of the central node provinces is too strong, which causes other node provinces to lose their positions. To some extent, although the siphoning effect plays a role among those pilot provinces, they still have an essential position in the whole spatial network. For example, in the 2000 baseline, the central node provinces were the Anhui, Fujian, Hunan, and Tianjin. After five years of average prediction, the pilot province Guandong suffers a great shock in terms of betweenness centrality (BCi,t). The same goes for the 2010 baseline but not for the 2021 baseline. By contrast, those non-pilot provinces suffer more from the siphon effect. Without the low-carbon pilot policy, they will gradually lose their network competitiveness if they are not closely linked to the central node, especially as the baseline condition changes from 2000 to 2021. In sum, under different scenarios, the central node provinces will gradually use their siphoning effect to improve carbon emission status. With the help of the low-carbon pilot policy, the siphoning effect can be further enhanced.
Difference-in-differences results
Benchmark analyses
Table 6 shows the corrected relationship between China’s low-carbon pilot policy (LCCi,t) and province-level carbon emissions (CEi,t) using the staggered DID estimation regression. In column (1), the foundational effect of China’s low-carbon pilot policy is explored without any control variables. In column (2), all control variables and fixed effects are used together. In columns (3)-(6), province-level carbon emissions (CEi,t) are replaced by its four compositions: raw coal emissions
Effect of China’s low-carbon pilot policy, benchmark
(1) | (2) | (3) | (4) | (5) | (6) | |
CEi,t | CEi,t | COALi,t | OILi,t | GASi,t | CEMi,t | |
LCCi,t | -12.250** | -17.433*** | -12.243*** | -2.119** | -0.778** | -2.290** |
(-1.97) | (-2.67) | (-2.71) | (-2.18) | (-2.33) | (-2.13) | |
AGDPi,t | 47.978** | 83.941** | 43.820** | 14.210** | 22.066*** | |
(2.23) | (2.48) | (2.37) | (2.48) | (2.64) | ||
STRi,t | 9.898** | 10.244* | 5.060** | 3.320** | 2.036** | |
(2.57) | (1.72) | (2.17) | (2.55) | (2.35) | ||
ENEi,t | -31.148* | -35.311* | -33.581* | -14.99* | -15.582** | |
(-1.90) | (-1.85) | (-1.85) | (-1.86) | (-1.98) | ||
FDIi,t | 6.412 | 7.121* | -9.798* | 0.568 | 0.520 | |
(1.55) | (1.72) | (-2.04) | (1.49) | (1.49) | ||
INTi,t | 3.997 | 13.022 | 6.413* | 2.804 | 0.193 | |
(0.13) | (0.43) | (1.82) | (1.44) | (0.14) | ||
EXTi,t | -18.932 | -29.321 | -4.574 | -2.286** | -3.527*** | |
(-0.56) | (-0.94) | (-1.18) | (-1.96) | (-2.74) | ||
CONS | 21.640** | 24.835** | 16.664*** | 28.871** | 29.060*** | |
(2.02) | (2.51) | (2.78) | (2.34) | (4.22) | ||
Covariant | No | Yes | Yes | Yes | Yes | Yes |
Year-fixed | Yes | Yes | Yes | Yes | Yes | Yes |
Province-fixed | Yes | Yes | Yes | Yes | Yes | Yes |
Cluster | Yes | Yes | Yes | Yes | Yes | Yes |
N | 660 | 660 | 660 | 660 | 660 | 660 |
Adjusted R2 | 0.45 | 0.49 | 0.40 | 0.31 | 0.64 | 0.76 |
The estimated results show that China’s low-carbon pilot policy can effectively reduce carbon emissions. Compared to the non-pilot provinces, this pilot policy has a certain reduction effect on all kinds of carbon emissions. The average treatment effect is -17.433 at a 1% significance level for the total carbon emissions. However, this overall reduction effect varies if total carbon emissions are decomposed into four compositions. In terms of the raw coal emissions, the average treatment effect is -12.243 at a 1% significance level. In terms of crude oil emissions, natural gas emissions, and cement emissions, the average treatment effect becomes -2.119, -0.778, and -2.290, both at a 5% significance level. In this sense, China’s low-carbon pilot policy is more evident in raw coal emission reduction, thus helping to reduce the total emissions.
Inter-provincial interaction
In addition, we change the dependent variable from carbon emissions (CEi,t) to structural hole efficiency
Effect of China’s low-carbon pilot policy, discussions
(1) | (2) | (3) | (4) | (5) | (6) | |
HFi,t | HCi,t | HHi,t | CEi,t+1 | CEi,t+1 | CEi,t+1 | |
LCCi,t | 0.0311*** | -0.0219** | 0.0330*** | |||
(3.05) | (-2.11) | (2.93) | ||||
LCCi,t * STi,t+1 | -0.1271** | |||||
(-2.44) | ||||||
LCCi,t * GPi,t+1 | -0.3009** | |||||
(-2.04) | ||||||
LCCi,t * OFDIi,t+1 | -0.0061 | |||||
(-1.37) | ||||||
Covariant | No | Yes | Yes | Yes | Yes | Yes |
Year-fixed | Yes | Yes | Yes | Yes | Yes | Yes |
Province-fixed | Yes | Yes | Yes | Yes | Yes | Yes |
Cluster | Yes | Yes | Yes | Yes | Yes | Yes |
N | 660 | 660 | 660 | 630 | 630 | 630 |
Adjusted R2 | 0.37 | 0.33 | 0.35 | 0.66 | 0.83 | 0.62 |
Underlying reduction mechanism
In columns (4)-(6) of Table 7, we then conduct the potential mechanism analyses using the interaction term regression processes. Following the method of Liu et al.., we use the industrial rational index (STi,t), green patent output (GPi,t), and outward foreign direct investment (OFDIi,t) as the respective mechanism variables[41]. The industrial rational index reflects the degree of resource utilization among sectors, calculated by the average ratio of the output percentage to the labor force percentage in each sector. The green patent output reflects the innovation output in green, measured by the number of green patents granted[135]. Outward foreign direct investment reflects the direct outflows of investment funds. The estimated results show that local governments prefer to conduct internal improvements instead of outward emission transfers to address their local carbon emissions. For industrial rational index and green patent output, their mechanism effects between the low-carbon pilot policy and province-level carbon emissions are 12.71% and 30.09% at the 5% significance level. For outward foreign direct investment, no obvious mechanism effect shows even at the 10% significance level. By promoting industrial rational transformation and green innovation performance, provinces (especially pilot provinces) will optimize resource allocation and guide funds flows reasonably and effectively, thus helping carbon emission reduction at the source stage. These results also hold the view that domestic improvements have more sustainable development potential than outward transfers.
CONCLUSION AND POLICY IMPLICATION
Conclusions
Global climate change and the accompanying energy consumption have aroused widespread societal concerns and brought new challenges to countries. As a responsible developing country, China is trying hard to reduce carbon emissions to achieve carbon neutrality by 2060. This study explores the spatial differentiation of China’s province-level carbon emission footprint from 2000 to 2021, using the Gini decomposition method, the social network analysis, and the difference-in-differences estimation. With some theoretical analysis and empirical evidence provided, this study may be useful for achieving carbon neutrality through regional carbon emission footprint coordinated development. The main conclusions are as follows:
First, the total Gini-based carbon emission footprint index shows an overall growth trend from 2000 to 2021, indicating the increasing Gini gap in carbon emissions between provinces. However, the four sub-indices exhibit two distinct types of developmental characteristics. While raw coal and crude oil sub-indices keep a growth trend, natural gas and cement sub-indices have been falling constantly. In comparison, the Gini-based carbon emission footprint index in non-pilot provinces is almost twice as large as that of pilot provinces, revealing the relatively balanced growth trend in carbon emissions across pilot provinces. Among these considerable differences, it is evident that China’s low-carbon pilot policy has a positive effect on narrowing the province-level gap in crude oil emissions.
Second, the overall social network structure has undergone obvious changes from loose to tight. Regarding global network characteristics, those node provinces in the inner network center will have more outward mutual connections, as the siphoning effect can bring extra socio-economic benefits. Regarding node network characteristics, those pilot provinces have a comparative advantage regarding social network position. In response, the competition in non-pilot provinces is more intense than those in the pilot provinces, thus consolidating their existing network position. Regarding dynamic network characteristics, pilot provinces have better abilities in handling invalid redundant relationships, thereby stimulating the “resource-bridge” role to obtain better development opportunities. Considering the interaction between those central node provinces and other non-central ones, it is found that the average prediction network structure becomes stable and dense for the next five years, regardless of different baseline conditions. Nevertheless, although those central node provinces play an essential role in maintaining and improving network structure, they also produce a certain central siphon effect, thus causing other node provinces to lose their positions gradually.
Third, compared to the non-pilot provinces, China’s low-carbon pilot policy can effectively reduce all kinds of carbon emissions in pilot provinces. The average treatment effect is -17.433 for the total carbon emissions, -12.243 for the raw coal emissions, -2.119 for the crude oil emissions, -0.778 for the natural gas emissions, and -2.290 for the cement emissions. In a statical sense, this low-carbon pilot policy is more evident in raw coal emission reduction. By broadening the understanding of national policy effectiveness in regional energy transition, this result can help us achieve the pressing objectives of reducing carbon emissions from burning raw coal, thus increasing climate resilience. Furthermore, structural transformation and improvement are the ultimate goals for future sustainable growth if compared to simple emission reduction. After the emission reduction process, the estimated results show that the pilot node provinces will use more resources to strengthen their structural ability, status, and rank. Regarding the internal mechanisms, provinces (especially pilot provinces) will optimize resource allocation and guide funds flows reasonably through industrial rational transformation and green innovation performance. No direct evidence supports the outward transfer mechanism.
Policy implications
At the crossroads of sustainable development, how to speed up the green transformation through regional coordinated development will be incredibly important. This study will put forward the following policy implications based on our conclusion.
First, considering the increasing Gini gap in carbon emissions between provinces, the Chinese central government should further promote the low-carbon pilot policy at the nationwide level. In terms of laws, the central government should formulate enough auxiliary environmental regulations to coordinate the relationship between low-carbon pilot policy and the current national carbon market, thus helping narrow the inter-provincial Gini gaps to some extent. In practice, the corresponding environmental regulations have already performed well among some Chinese urban agglomerations, such as the Yangtze River Delta and Pearl River Delta urban agglomerations. One effective measurement will be the regional environmental court, which has addressed many cross-regional pollution and emission events.
Second, considering global networks, node networks, dynamic networks, and especially the inter-provincial interaction prediction of the carbon emission footprint, the Chinese local governments should formulate some sub-regional cooperation development plans. In terms of dynamic scenario analysis, the future network structure will be more centralized and stable in the prediction. With the increasing central siphon effects, those central node provinces will become the core of the carbon emission footprint network. For other node provinces, while maintaining their own advantages, choosing the right central node to communicate and cooperate will be an effective way of sustainable development. For those areas in the middle level of the central node provinces, figuring out how to make strong relationships with both central nodes should be the major task.
Last, considering the effective effects of the low-carbon pilot policy on emission reduction, the Chinese local governments should enhance the mechanism processes at both the industrial and corporate levels. In terms of the current mechanism implementations, more funds should be directed toward the environmental-related sectors and projects, especially those that should be used domestically. At the industrial level, local governments should formulate appropriate and specialized industrial funding plans based on their own industrial structure, thus cooperating with the low-carbon pilot policy for industrial upgrading. At the corporate level, management should set corporate strategies from the long-term sustainable development perspective. By guiding funds flows, more low-carbon technologies can be cultivated.
To a certain extent, this study also has some limitations. Although this study has analyzed the spatial differentiation of China’s province-level carbon emission footprint, it has not assessed it at the city level. In the next plan, we will refine this research topic further at a more detailed city level.
DECLARATIONS
Authors’ contributions
Data curation, formal analysis, methodology, writing - original draft preparation, writing - review & editing, funding acquisition: Liu Y
Data curation, formal analysis, conceptualization, writing - review & editing: Deng L
Availability of data and materials
Data will be made available from the corresponding author upon reasonable request. All the data sets and materials used have been retrieved, cited, and explained in an appropriate manner.
Financial support and sponsorship
This study is supported by the Fundamental Research Funds for the Central Universities of Wuhan University [Grant No. 1203-413000172].
Conflicts of interest
Deng L is affiliated with CISDI Group Co., Ltd. While the other author declared that there are no conflicts of interest.
Ethical approval and consent to participate
Not applicable.
Consent for publication
Not applicable.
Copyright
© The Author(s) 2024.
Supplementary Materials
REFERENCES
1. Bruckner B, Hubacek K, Shan Y, Zhong H, Feng K. Impacts of poverty alleviation on national and global carbon emissions. Nat Sustain 2022;5:311-20.
2. Dong H, Xue M, Xiao Y, Liu Y. Do carbon emissions impact the health of residents? Considering China's industrialization and urbanization. Sci Total Environ 2021;758:143688.
3. Steinberger JK, Roberts JT, Peters GP, Baiocchi G. Pathways of human development and carbon emissions embodied in trade. Nat Clim Chang 2012;2:81-5.
4. Salvia M, Reckien D, Pietrapertosa F, et al. Will climate mitigation ambitions lead to carbon neutrality? An analysis of the local-level plans of 327 cities in the EU. Renew Sustain Energy Rev 2021;135:110253.
5. Wu X, Tian Z, Guo J. A review of the theoretical research and practical progress of carbon neutrality. Sustain Oper Comput 2022;3:54-66.
6. Wu Z, Huang X, Chen R, Mao X, Qi X. The United States and China on the paths and policies to carbon neutrality. J Environ Manag 2022;320:115785.
7. Dong H, Liu Y, Zhao Z, Tan X, Managi S. Carbon neutrality commitment for China: from vision to action. Sustain Sci 2022;17:1741-55.
8. Sun LL, Cui HJ, Ge QS. Will China achieve its 2060 carbon neutral commitment from the provincial perspective? Adv Clim Chang Res 2022;13:169-78.
9. Liu Z, Deng Z, He G, et al. Challenges and opportunities for carbon neutrality in China. Nat Rev Earth Environ 2022;3:141-55.
10. Dong Q, Zhong C, Geng Y, Dong F, Chen W, Zhang Y. A bibliometric review of carbon footprint research. Carbon Footprints 2024;3:3.
11. Weber CL, Matthews HS. Quantifying the global and distributional aspects of American household carbon footprint. Ecol Econ 2008;66:379-91.
12. Mancini MS, Galli A, Niccolucci V, et al. Ecological footprint: refining the carbon footprint calculation. Ecol Indic 2016;61:390-403.
14. Shi S, Yin J. Global research on carbon footprint: a scientometric review. Environ Impact Assess Rev 2021;89:106571.
15. Bailis R, Drigo R, Ghilardi A, Masera O. The carbon footprint of traditional woodfuels. Nat Clim Chang 2015;5:266-72.
16. Fenner AE, Kibert CJ, Woo J, et al. The carbon footprint of buildings: a review of methodologies and applications. Renew Sustain Energy Rev 2018;94:1142-52.
17. Lenzen M, Sun Y, Faturay F, Ting Y, Geschke A, Malik A. The carbon footprint of global tourism. Nat Clim Chang 2018;8:522-8.
18. Jóhannesson SE, Heinonen J, Davíðsdóttir B. Data accuracy in Ecological Footprint’s carbon footprint. Ecol Indic 2020;111:105983.
19. Nassar YF, El-Khozondar HJ, El-Osta W, et al. Carbon footprint and energy life cycle assessment of wind energy industry in Libya. Energy Convers Manag 2024;300:117846.
20. Matthews HS, Hendrickson CT, Weber CL. The importance of carbon footprint estimation boundaries. Environ Sci Technol 2008;42:5839-42.
21. Chen J, Fan W, Li D, Liu X, Song M. Driving factors of global carbon footprint pressure: based on vegetation carbon sequestration. Appl Energy 2020;267:114914.
22. Chen X, Ma C, Zhou H, et al. Identifying the main crops and key factors determining the carbon footprint of crop production in China, 2001-2018. Resour Conserv Recy 2021;172:105661.
23. La Peña L, Guo R, Cao X, Ni X, Zhang W. Accelerating the energy transition to achieve carbon neutrality. Resour Conserv Recy 2022;177:105957.
24. Dong F, Li Y, Gao Y, Zhu J, Qin C, Zhang X. Energy transition and carbon neutrality: exploring the non-linear impact of renewable energy development on carbon emission efficiency in developed countries. Resour Conserv Recy 2022;177:106002.
25. Tian J, Yu L, Xue R, Zhuang S, Shan Y. Global low-carbon energy transition in the post-COVID-19 era. Appl Energy 2022;307:118205.
26. Xia C, Zheng H, Meng J, Li S, Du P, Shan Y. The evolution of carbon footprint in the yangtze river delta city cluster during economic transition 2012-2015. Resour Conserv Recy 2022;181:106266.
27. Xing Z, Jiao Z, Wang H. Carbon footprint and embodied carbon transfer at city level: a nested MRIO analysis of central plain urban agglomeration in China. Sustain Cities Soc 2022;83:103977.
28. Nielsen KS, Bauer JM, Debnath R, et al. Underestimation of personal carbon footprint inequality in four diverse countries. Nat Clim Chang 2024;14:1136-43.
29. Theine H, Humer S, Moser M, Schnetzer M. Emissions inequality: disparities in income, expenditure, and the carbon footprint in Austria. Ecol Econ 2022;197:107435.
30. Wang M, Feng C. The inequality of China’s regional residential CO2 emissions. Sustain Prod Consump 2021;27:2047-57.
31. Ogede JS, Oduola MO, Tiamiyu HO. Income inequality and carbon dioxide (CO2) in sub-Saharan Africa countries: the moderating role of financial inclusion and institutional quality. Environ Dev Sustain 2024;26:18385-409.
32. Owen A, Barrett J. Reducing inequality resulting from UK low-carbon policy. Clim Policy 2020;20:1193-208.
33. Cheng S, Fan W, Zhang J, Wang N, Meng F, Liu G. Multi-sectoral determinants of carbon emission inequality in Chinese clustering cities. Energy 2021;214:118944.
34. Wang S, Wang J, Fang C, Feng K. Inequalities in carbon intensity in China: a multi-scalar and multi-mechanism analysis. Appl Energy 2019;254:113720.
35. Zhang S, Kharrazi A, Yu Y, Ren H, Hong L, Ma T. What causes spatial carbon inequality? Evidence from China’s Yangtze River economic Belt. Ecol Indic 2021;121:107129.
36. Bolea L, Duarte R, Sánchez-Chóliz J. Exploring carbon emissions and international inequality in a globalized world: a multiregional-multisectoral perspective. Resour Conserv Recy 2020;152:104516.
37. Liu Y, Wang M, Feng C. Inequalities of China’s regional low-carbon development. J Environ Manag 2020;274:111042.
38. Hou A, Liu A, Chai L. Does reducing income inequality promote the decoupling of economic growth from carbon footprint? World Dev 2024;173:106423.
39. Liu X, Wang K. The inequality of household carbon footprint in China: a city-level analysis. Energy Policy 2024;188:114098.
40. Guo S. Exploring low-carbon pilot city policy implementation: evidence from China. Clim Policy 2023;23:1045-57.
41. Liu Y, Huang J, Xu J, Xiong S. Natural resource dependence and sustainable development policy: insights from city-level analysis. Resour Policy 2024;91:104928.
42. Zeng S, Jin G, Tan K, Liu X. Can low-carbon city construction reduce carbon intensity? Empirical evidence from low-carbon city pilot policy in China. J Environ Manag 2023;332:117363.
43. Zhang H, Feng C, Zhou X. Going carbon-neutral in China: does the low-carbon city pilot policy improve carbon emission efficiency? Sustain Prod Consump 2022;33:312-29.
44. He G, Lu Y, Mol AP, Beckers T. Changes and challenges: China’s environmental management in transition. Environ Dev 2012;3:25-38.
47. Cao J, Li W, Bilokha A. Low-carbon city initiatives and analyst behaviour: a quasi-natural experiment. J Financ Stabil 2022;62:101042.
48. Khanna N, Fridley D, Hong L. China’s pilot low-carbon city initiative: a comparative assessment of national goals and local plans. Sustain Cities Soc 2014;12:110-21.
49. Du X, Shen L, Ren Y, Meng C. A dimensional perspective-based analysis on the practice of low carbon city in China. Environ Impact Assess Rev 2022;95:106768.
50. Liu X, Xu H. Does low-carbon pilot city policy induce low-carbon choices in residents’ living: Holistic and single dual perspective. J Environ Manage 2022;324:116353.
51. Huwei W, Shuai C, Chien-Chiang L. Impact of low-carbon city construction on financing, investment, and total factor productivity of energy-intensive enterprises. Energy J 2023;44:79-102.
52. Yu Y, Zhang N. Low-carbon city pilot and carbon emission efficiency: quasi-experimental evidence from China. Energy Econ 2021;96:105125.
53. Li H, Wang J, Yang X, Wang Y, Wu T. A holistic overview of the progress of China’s low-carbon city pilots. Sustain Cities Soc 2018;42:289-300.
54. Li S, Xu Q, Liu J, Shen L, Chen J. Experience learning from low-carbon pilot provinces in China: pathways towards carbon neutrality. Energy Strategy Rev 2022;42:100888.
55. Ren YS, Liu PZ, Klein T, Sheenan L. Does the low-carbon pilot cities policy make a difference to the carbon intensity reduction? J Econ Behav Organ 2024;217:227-39.
56. He G, Morse R. Addressing carbon Offsetters’ paradox: lessons from Chinese wind CDM. Energy Policy 2013;63:1051-5.
57. Pan A, Zhang W, Shi X, Dai L. Climate policy and low-carbon innovation: evidence from low-carbon city pilots in China. Energy Econ 2022;112:106129.
58. Lannelongue L, Grealey J, Inouye M. Green algorithms: quantifying the carbon footprint of computation. Adv Sci 2021;8:2100707.
59. Valls-Val K, Bovea MD. Carbon footprint in higher education institutions: a literature review and prospects for future research. Clean Technol Environ Policy 2021;23:2523-42.
60. Siau K, Hayee BH, Gayam S. Endoscopy’s current carbon footprint. Tech Innov Gastrointest Endosc 2021;23:344-52.
61. Chen L, Xu L, Cai Y, Yang Z. Spatiotemporal patterns of industrial carbon emissions at the city level. Resour Conserv Recy 2021;169:105499.
62. Lu J, Chen H, Cai X. From global to national scenarios: exploring carbon emissions to 2050. Energy Strategy Rev 2022;41:100860.
63. Zhou X, Zhou D, Wang Q, Su B. How information and communication technology drives carbon emissions: a sector-level analysis for China. Energy Econ 2019;81:380-92.
64. Lombardi M, Laiola E, Tricase C, Rana R. Assessing the urban carbon footprint: an overview. Environ Impact Assess Rev 2017;66:43-52.
65. Wiedmann T, Minx J. A definition of ‘carbon footprint’. In: Ecological Economics Research Trends. Available from: https://books.google.com/books?id=GCkU1p_6HNwC&dq=carbon+emission+footprint&lr=&source=gbs_navlinks_s [Last accessed on 16 Nov 2024].
66. Huang Y, Yu Q, Wang R. Driving factors and decoupling effect of carbon footprint pressure in China: based on net primary production. Technol Forecast Soc Chang 2021;167:120722.
67. Liang D, Lu H, Guan Y, Feng L. Drivers for decoupling carbon footprint pressure from economic growth in China’s provinces. Geogr Sustain 2022;3:258-67.
68. Pan G, Li X, Pan D, Liu W. Decoupling effect and driving factors of carbon footprint in megacity Wuhan, Central China. Ecol Process 2023;12:23.
69. Yang Y, Meng G. The decoupling effect and driving factors of carbon footprint in megacities: the case study of Xi’an in western China. Sustain Cities Soc 2019;44:783-92.
70. Dirix J, Peeters W, Eyckmans J, Jones PT, Sterckx S. Strengthening bottom-up and top-down climate governance. Clim Policy 2013;13:363-83.
71. Pardo G, Casas R, del Prado A, Manzano P. Carbon footprint of transhumant sheep farms: accounting for natural baseline emissions in Mediterranean systems. Int J Life Cycle Assess 2023.
72. Pandey D, Agrawal M, Pandey JS. Carbon footprint: current methods of estimation. Environ Monit Assess 2011;178:135-60.
73. Achten WM, Almeida J, Muys B. Carbon footprint of science: more than flying. Ecol Indic 2013;34:352-5.
74. Azarkamand S, Ferré G, Darbra RM. Calculating the carbon footprint in ports by using a standardized tool. Sci Total Environ 2020;734:139407.
75. Foo DC, Tan RR. A review on process integration techniques for carbon emissions and environmental footprint problems. Process Saf Environ Prot 2016;103:291-307.
76. Egilmez G, Bhutta K, Erenay B, Park YS, Gedik R. Carbon footprint stock analysis of US manufacturing: a time series input-output LCA. Ind Manag Data Syst 2017;117:853-72.
77. Steubing B, de Koning A, Merciai S, Tukker A. How do carbon footprints from LCA and EEIOA databases compare? A comparison of ecoinvent and EXIOBASE. J Ind Ecol 2022;26:1406-22.
78. Lenzen M, Wood R, Wiedmann T. Uncertainty analysis for multi-region input-output models - a case study of the UK’s carbon footprint. Econ Syst Res 2010;22:43-63.
79. Abbood K, Egilmez G, Meszaros F. Multi-region input-output-based carbon and energy footprint analysis of U.S. manufacturing. Period Polytech Soc Man Sci 2023;31:91-9.
80. Demeter C, Lin P, Sun Y, Dolnicar S. Assessing the carbon footprint of tourism businesses using environmentally extended input-output analysis. J Sustain Tour 2022;30:128-44.
81. Sun Y, Cadarso MA, Driml S. Tourism carbon footprint inventories: a review of the environmentally extended input-output approach. Ann Tour Res 2020;82:102928.
82. Sheng X, Chen L, Liu M, et al. Input-output models for carbon accounting: a multi-perspective analysis. Renew Sustain Energy Rev 2025;207:114950.
83. Ji S, Chen B. LCA-based carbon footprint of a typical wind farm in China. Energy Proc 2016;88:250-6.
84. Kanemoto K, Moran D, Hertwich EG. Mapping the carbon footprint of nations. Environ Sci Technol 2016;50:10512-7.
85. Li P, Xia X, Guo J. A review of the life cycle carbon footprint of electric vehicle batteries. Sep Purif Technol 2022;296:121389.
86. Farzaneh F, Jung S. Lifecycle carbon footprint comparison between internal combustion engine versus electric transit vehicle: a case study in the U.S. J Clean Prod 2023;390:136111.
87. Liu Z, Wang F, Tang Z, Tang J. Predictions and driving factors of production-based CO2 emissions in Beijing, China. Sustain Cities Soc 2020;53:101909.
88. Nguyen Q, Diaz-Rainey I, Kuruppuarachchi D. Predicting corporate carbon footprints for climate finance risk analyses: a machine learning approach. Energy Econ 2021;95:105129.
89. Shi X, Wang K, Cheong TS, Zhang H. Prioritizing driving factors of household carbon emissions: an application of the LASSO model with survey data. Energy Econ 2020;92:104942.
90. Ehrlich PR, Holdren JP. Impact of population growth: complacency concerning this component of man’s predicament is unjustified and counterproductive. Science 1971;171:1212-7.
91. Liang D, Lu H, Guan Y, Feng L, Chen Y, He L. Further mitigating carbon footprint pressure in urban agglomeration by enhancing the spatial clustering. J Environ Manag 2023;326:116715.
92. Dietz T, Rosa EA. Effects of population and affluence on CO2 emissions. Proc Natl Acad Sci USA 1997;94:175-9.
93. Wang M, Arshed N, Munir M, Rasool SF, Lin W. Investigation of the STIRPAT model of environmental quality: a case of nonlinear quantile panel data analysis. Environ Dev Sustain 2021;23:12217-32.
94. Liu Y, Liu W, Zhang X, Dong H, Zhao Z, Zhang Z. Domestic environmental impacts of OFDI: city-level evidence from China. Int Rev Econ Financ 2024;89:391-409.
95. Solarin SA. An environmental impact assessment of fossil fuel subsidies in emerging and developing economies. Environ Impact Assess Rev 2020;85:106443.
96. Xue L, Li H, Xu C, et al. Impacts of industrial structure adjustment, upgrade and coordination on energy efficiency: empirical research based on the extended STIRPAT model. Energy Strategy Rev 2022;43:100911.
97. Ang BW, Choi K. Decomposition of aggregate energy and gas emission intensities for industry: a refined divisia index method. Energy J 1997;18:59-73.
98. Ang B, Zhang F. A survey of index decomposition analysis in energy and environmental studies. Energy 2000;25:1149-76.
99. Kaltenegger O. What drives total real unit energy costs globally? A novel LMDI decomposition approach. Appl Energy 2020;261:114340.
100. Liu H, Chen C. Prediction of outdoor PM2.5 concentrations based on a three-stage hybrid neural network model. Atmos Pollut Res 2020;11:469-81.
101. Zellner A, Theil H. Three-stage least squares: simultaneous estimation of simultaneous equations. In: Raj B, Koerts J, editors. Henri theil’s contributions to economics and econometrics. Dordrecht, Netherlands: Springer; 1992. pp. 147-78.
102. Lin Y. Carbon emission reduction policy simulation based on the CGE model. Expert Syst 2024;41:e13251.
103. Liu Y, Zhu N, Zhou M, et al. An environmental CGE model of China’s economy: modeling choices and application. Energy Clim Manag 2025;1:9400002.
104. Wang P, Huang G, Li Y, Liu Y, Li Y. An ecological input-output CGE model for unveiling CO2 emission metabolism under China’s dual carbon goals. Appl Energy 2024;365:123277.
105. Hasan MF, Zantye MS, Kazi M. Challenges and opportunities in carbon capture, utilization and storage: a process systems engineering perspective. Comput Chem Eng 2022;166:107925.
106. Li M, Wang Y, Chen W, Sun Y, Hou H, Liu Y. Assessing GHG emissions of food consumption towards low-carbon transformation in China. Environ Impact Assess Rev 2024;105:107408.
107. Gao C, Tao S, He Y, Su B, Sun M, Mensah IA. Effect of population migration on spatial carbon emission transfers in China. Energy Policy 2021;156:112450.
108. Zhang W, Liu X, Wang D, Zhou J. Digital economy and carbon emission performance: evidence at China’s city level. Energy Policy 2022;165:112927.
109. Yin J, Shi S. Social interaction and the formation of residents′ low-carbon consumption behaviors: an embeddedness perspective. Resour Conserv Recy 2021;164:105116.
110. Guan Y, Shan Y, Huang Q, Chen H, Wang D, Hubacek K. Assessment to China’s recent emission pattern shifts. Earths Future 2021;9:e2021EF002241.
111. Shan Y, Liu J, Liu Z, et al. New provincial CO2 emission inventories in China based on apparent energy consumption data and updated emission factors. Appl Energy 2016;184:742-50.
114. Xu J, Guan Y, Oldfield J, Guan D, Shan Y. China carbon emission accounts 2020-2021. Appl Energy 2024;360:122837.
115. Bartlett J, Keogh R, Bonneville EF, Ekstrøm CT. Multiple imputation of covariates by substantive model compatible fully conditional specification. Available from: https://cran.r-project.org/web/packages/smcfcs/smcfcs.pdf [Last accessed on 16 Nov 2024].
118. Sun M, Chen G, Xu X, Zhang L, Hubacek K, Wang Y. Reducing carbon footprint inequality of household consumption in rural areas: analysis from five representative provinces in China. Environ Sci Technol 2021;55:11511-20.
119. Wiedenhofer D, Guan D, Liu Z, Meng J, Zhang N, Wei Y. Unequal household carbon footprints in China. Nat Clim Chang 2017;7:75-80.
120. He W, Liu D, Wang C. Are Chinese provincial carbon emissions allowances misallocated over 2000-2017? Evidence from an extended Gini-coefficient approach. Sustain Prod Consump 2022;29:564-73.
121. Teng F, He J, Pan X, Zhang C. Metric of carbon equity: carbon Gini index based on historical cumulative emission per capita. Adv Clim Chang Res 2011;2:134-40.
122. Wang M, Feng C. Tracking the inequalities of global per capita carbon emissions from perspectives of technological and economic gaps. J Environ Manag 2022;315:115144.
123. Xing R, Luo Z, Zhang W, et al. Household fuel and direct carbon emission disparity in rural China. Environ Int 2024;185:108549.
124. Clarke-Sather A, Qu J, Wang Q, Zeng J, Li Y. Carbon inequality at the sub-national scale: a case study of provincial-level inequality in CO2 emissions in China 1997-2007. Energy Policy 2011;39:5420-8.
125. Jorgenson AK, Schor JB, Knight KW, Huang X. Domestic inequality and carbon emissions in comparative perspective. Soc Forum 2016;31:770-86.
126. Fang D, Chen B. Information-based ecological network analysis for carbon emissions. Appl Energy 2019;238:45-53.
127. Huo T, Cao R, Xia N, Hu X, Cai W, Liu B. Spatial correlation network structure of China’s building carbon emissions and its driving factors: a social network analysis method. J Environ Manag 2022;320:115808.
128. Bai C, Zhou L, Xia M, Feng C. Analysis of the spatial association network structure of China’s transportation carbon emissions and its driving factors. J Environ Manag 2020;253:109765.
129. Jiang M, An H, Gao X, Liu S, Xi X. Factors driving global carbon emissions: a complex network perspective. Resour Conserv Recy 2019;146:431-40.
130. Huang H, Jia J, Chen D, Liu S. Evolution of spatial network structure for land-use carbon emissions and carbon balance zoning in Jiangxi province: a social network analysis perspective. Ecol Indic 2024;158:111508.
131. Yu Z, Chen L, Tong H, et al. Spatial correlations of land-use carbon emissions in the Yangtze river delta region: a perspective from social network analysis. Ecol Indic 2022;142:109147.
132. Wang Y, Liu X, Han X, et al. Unique hole-accepting carbon-dots promoting selective carbon dioxide reduction nearly 100% to methanol by pure water. Nat Commun 2020;11:2531.
133. Li F, Li X. An empirical analysis on regional natural gas market of China from a spatial pattern and social network perspective. Energy 2022;244:122598.
134. Zhang H, Di Maria C, Ghezelayagh B, Shan Y. Climate policy in emerging economies: evidence from China’s low-carbon city pilot. J Environ Econ Manag 2024;124:102943.
Cite This Article
How to Cite
Download Citation
Export Citation File:
Type of Import
Tips on Downloading Citation
Citation Manager File Format
Type of Import
Direct Import: When the Direct Import option is selected (the default state), a dialogue box will give you the option to Save or Open the downloaded citation data. Choosing Open will either launch your citation manager or give you a choice of applications with which to use the metadata. The Save option saves the file locally for later use.
Indirect Import: When the Indirect Import option is selected, the metadata is displayed and may be copied and pasted as needed.
About This Article
Copyright
Data & Comments
Data
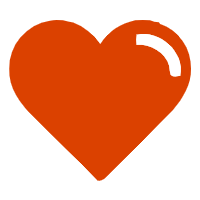
Comments
Comments must be written in English. Spam, offensive content, impersonation, and private information will not be permitted. If any comment is reported and identified as inappropriate content by OAE staff, the comment will be removed without notice. If you have any queries or need any help, please contact us at [email protected].