Supporting decarbonization through vehicle rightsizing, automation, and ride-splitting
Abstract
Vehicle automation and smartphone app-based ride-splitting are commonly discussed topics in the transportation literature. While these technologies have been examined for their role in transportation decarbonization through simulation study, the motivation for such work is rarely made explicit. In this commentary, we provide a motivation for research in this area based on our own simulation research, as well as land use and vehicle operational factors. Specifically, land use factors such as density and the speed of its adjustment make traditional transit operations using large vehicles cost-prohibitive in most U.S. communities (and many other communities around the world). Automation and ride-splitting technologies may offer digitized transportation solutions that can match vehicle size to local land development density and passenger demand. In addition, we highlight a difference in the supply-demand relationship for freight transportation that causes additional challenges for decarbonizing that sector. Finally, we emphasize that fleet ownership is key to ensuring timely vehicle fleet turnover as safer and more efficient technologies enter the market.
Keywords
Transportation surpassed electricity in the United States as the largest economic sector emitter of greenhouse gases (GHGs) in 2018 (assuming electricity for heating is allocated to the electricity sector)[1]. The potential solutions to mitigate these emissions are diverse. The United Nations sees compact urban growth - and associated policies - as central to global climate change mitigation, including for the transportation sector[2]. However, new buildings, neighborhood designs, and land use patterns can take decades to implement at scale[3] and face political and social barriers[4]. At the same time, the U.S. transit share of passenger miles traveled is under 1%[5], and conventional transit is not cost-effective at densities less than about 35 dwelling units per acre[6]. Taken together, these factors raise the question of how to ensure climate goals are met for the transportation sector in a timely manner. In contrast to land use change, digital technology can be adopted at a rapid pace. The transportation sector is currently undergoing a technology transition driven by vehicle electrification, app-based ride-hailing, and vehicle automation. Unfortunately, the potential roles played jointly by automation and ride-hailing in climate change policy are often lost within technical reports and articles. For example, the U.S. Department of Energy SMART (Systems and Modeling for Accelerated Research in Transportation) consortium is a multi-year, multi-laboratory collaboration dedicated to understanding the energy implications and opportunities of the evolving transportation technology ecosystem through simulation and behavioral analyses[7]. We provide a climate mitigation case for digital technologies in transportation based on our own contributions to the SMART consortium and other related research. This commentary contributes to the Carbon Footprint Special Issue entitled “Digitizing Carbon Footprint Management” by providing a synthesis of how digital technology (i.e., automation and app-based ride-hailing) might contribute to achieving net-zero climate targets within the transportation sector.
There is a consensus that vehicle electrification is key to transportation GHG mitigation in the U.S., but private-vehicle electrification will be insufficient without lowered vehicle use[8]. Development densification, coupled with transit investments, will be needed to shorten travel distances and encourage more active and shared travel modes. However, as we outline below, traditional transit solutions suffer from a supply-demand mismatch. We argue that vehicle rightsizing, made cost-viable by automation and ride-splitting (We distinguish in this paper between ride-sharing as sharing a vehicle (but not a trip) and ride-splitting as sharing a trip (or a portion thereof). Ride-splitting describes the sharing of travel miles by unaffiliated travelers in a single vehicle along a common route[9]) technologies, is a feasible solution to this mismatch and provides a pathway to transportation decarbonization, given land use and transportation considerations in the U.S.
GHG emissions intensity analyses by travel mode illustrate the relationships between land use, mode choice, and travel distance. Several studies have compared the life-cycle emissions of transit to those of private vehicles[10,11], highlighting the importance of vehicle occupancy (i.e., load factor). Most recently, Soukhov and Mohamed estimate that battery-electric vehicles, relying on Canada’s power mix, need to average 2 to 2.4 passengers to be comparable to battery-electric buses carrying 15 passengers[12]. Research by Wang et al. in Toronto estimates that bus transit produces higher emissions (per passenger-mile traveled) than private vehicles during the evening off-peak period, owing to low vehicle occupancy[13]. This occupancy dilemma represents a supply-demand mismatch. That is, vehicles cannot be sized to adapt to variable passenger demand. In the U.S., pre-COVID, average bus occupancy varied by state from 3.9 in Wyoming to 15.6 in New Jersey - with an average of 9.2[14].
While larger vehicles’ emissions rates per vehicle mile can benefit from economies of scale, this benefit is only true for a loaded vehicle, with most seats occupied - as illustrated by Schipper et al. ASIF equation[15]. While larger vehicles’ emissions rates per vehicle mile benefit from economies of scale, this benefit is only realized at relatively high vehicle occupancy, with most seats occupied
GHG emissions = travel activity [A] × modal structure [S] × energy intensity [I] × fuel carbon content [F]
Realizing GHG benefits for a full-size bus requires high passenger loadings to mitigate its high energy intensity per vehicle mile. Between the two extremes of large buses and small private vehicles, one can imagine a transit fleet with variously sized vehicles exploiting scale benefits when made feasible by land development density. 4- and 6-seater shared autonomous electric vehicle (SAEV) fleets are a new form of transit, both privately and publicly incentivized for shared rides (with unaffiliated riders, called dynamic ride-sharing (DRS), pooling, or splitting). These smaller vehicles would operate in lower-density suburban areas. Larger SAEVs (i.e., autonomous mini-buses) operating in higher-density areas may have attendants on board to serve riders and maintain vehicles. Competition among various SAEV fleet operators - e.g., Waymo, Cruise, and Argo - should also ensure lower service costs than the current U.S. average of $1.70 per passenger mile transit operating costs for bus transit[16]. In summary, a main climate mitigation benefit of SAEVs with DRS arises from the digitization of the driving and passenger matching tasks, facilitating efficient transit operations across a diversity of development densities.
Simulation analyses suggest that DRS services can reduce vehicle miles traveled (VMT) and GHG emissions by as much as 20% relative to private vehicle use[17-21]. There are also potential synergies with the electrical grid using vehicles as mobile storage devices (through vehicle-to-grid discharging) or as mobile energy consumers to absorb excess power generation[19,21]. As a complement to land use reform, SAEVs with DRS could reduce parking demand by 90%, freeing up land for other uses, including less expensive housing[22,23]. However, without price signals set by carbon taxes, congestion fees, and other policies, privately owned autonomous vehicles may increase total VMT by as much as 30% relative to their current levels[24,25]. Fleets of SAEVs can facilitate ride-splitting, even in places such as sprawling Orlando, Florida, where nearly 60% of person trips could be feasibly shared with a “stranger” at less than
A final set of benefits associated with SAEVs with DRS stems from its fleet ownership model. By one estimate, the introduction of managed charging for battery electric buses (BEBs) would save operators
Thus far, we have focused on short-distance passenger travel. Long-distance passenger travel and freight transport are more difficult to decarbonize the components of the transportation system[31]. We leave this discussion for other venues but will highlight one difference between technology-based decarbonization pathways in passenger and freight transportation. Vehicle rightsizing in the freight context exhibits a non-monotonic relationship that is not present in the passenger case [Figure 1]. Delivery cost efficiency tends to be highest at middling densities, with increasing density leading to inefficiencies because smaller vehicles must be used for delivery. The solutions for freight will differ from those outlined in this commentary.
Vehicle electrification and automation rely on individual and industry actions through technology development and deployment. Government and community policies such as credit-based congestion pricing[31] can help ensure those actions deliver climate mitigation, by promoting SAEV rides in right-sized vehicles, land use mixing at higher densities, and active modes of travel, while prohibiting empty travel by privately owned autonomous vehicles (AVs) and limiting empty travel by SAEV fleets. Private technological advances are often at odds with public policy, but private innovation in vehicle technology (i.e., electrification and automation) can complement and support land use and public transport policies. On the path to net zero, fleet automation facilitates vehicle rightsizing to match passenger loads and travel demands, while denser and more varied land use patterns remain fundamental to long-term urban sustainability. Vehicle fleets benefit from scale economies in both vehicle sizing and contracting, and higher rates of fleet-vehicle replacement can ensure faster adoption of more efficient transportation technologies.
DECLARATIONS
Acknowledgments
The authors also thank Aditi Bhaskar for her excellent editing and submission support.
Authors’ contributions
Made substantial contributions to the conception and design of the study: Hawkins J, Kockelman K
Performed data analysis and interpretation: Hawkins J
Made substantial contributions to the drafting and editing of the manuscript: Hawkins J, Kockelman K
Availability of data and materials
Not applicable.
Financial support and sponsorship
None.
Conflicts of interest
Both authors declared that there are no conflicts of interest.
Ethical approval and consent to participate
Not applicable.
Consent for publication
Not applicable.
Copyright
© The Author(s) 2024.
REFERENCES
1. EPA. Inventory of U.S. greenhouse gas emissions and sinks. 2021. Available from: https://www.epa.gov/ghgemissions/inventory-us-greenhouse-gas-emissions-and-sinks [Last accessed on 22 Dec 2023].
2. United Nations. The weight of cities: resource requirements of future urbanization. 2018. Available from: https://www.resourcepanel.org/reports/weight-cities [Last accessed on 22 Dec 2023].
3. Limb M, Murray C. We zoned for density and got higher house prices: supply and price effects of upzoning over 20 years. 2021. Available from: https://eprints.qut.edu.au/209104/ [Last accessed on 22 Dec 2023].
4. Mawhorter S, Reid C. Local housing policies across California: presenting the results of a new statewide survey. 2018. Available from: https://ternercenter.berkeley.edu/wp-content/uploads/2023/04/Terner_California_Residential_Land_Use_Survey_Report.pdf [Last accessed on 22 Dec 2023].
5. Bureau of Transportation Statistics. U.S. passenger-miles. 2022. Available from: https://www.bts.gov/content/us-passenger-miles [Last accessed on 22 Dec 2023].
6. Federal Transit Administration. Planning for transit-supportive development: a practitioner’s guide. 2014. Available from: https://www.transit.dot.gov/funding/funding-finance-resources/transit-oriented-development/planning-transit-supportive [Last accessed on 22 Dec 2023].
7. United States Department of Energy Vehicle Technologies Office. Energy efficient mobility systems. 2023. Available from: https://www.energy.gov/eere/vehicles/energy-efficient-mobility-systems [Last accessed on 22 Dec 2023].
8. Milovanoff A, Posen ID, Maclean HL. Electrification of light-duty vehicle fleet alone will not meet mitigation targets. Nat Clim Chang 2020;10:1102-7.
9. Chester MV, Horvath A. Environmental assessment of passenger transportation should include infrastructure and supply chains. Environ Res Lett 2009;4:024008.
10. Shaheen S, Cohen A. Shared ride services in North America: definitions, impacts, and the future of pooling. Transp Rev 2019;39:427-42.
11. Sinha R, Olsson LE, Frostell B. Sustainable personal transport modes in a life cycle perspective - public or private? Sustainability 2019;11:7092.
12. Soukhov A, Mohamed M. Occupancy and GHG emissions: thresholds for disruptive transportation modes and emerging technologies. Transp Res D Transp Environ 2022;102:103127.
13. Wang A, Stogios C, Gai Y, et al. Automated, electric, or both? Investigating the effects of transportation and technology scenarios on metropolitan greenhouse gas emissions. Sustain Cities Soc 2018;40:524-33.
14. Zhu M, Zhu W, Lutin JM, Cui Z, Wang Y. Developing a practical method to compute state-level bus occupancy rate. J Transp Eng Part A Syst 2021;147:05021001.
15. Schipper L, Marie-Lilliu C, Gorham R. Flexing the link between transport and greenhouse gas emissions: a path for the world bank. 2000. 55p. Available from: https://trid.trb.org/view/851906 [Last accessed on 22 Dec 2023].
16. DOT. 2018 national transit summaries and trends. 2019. Available from: https://www.transit.dot.gov/sites/fta.dot.gov/files/docs/ntd/data-product/134401/2018-ntst_1.pdf [Last accessed on 22 Dec 2023].
17. Chen TD, Kockelman KM, Hanna JP. Operations of a shared, autonomous, electric vehicle fleet: implications of vehicle & charging infrastructure decisions. Transp Res Part A Policy Pract 2016;94:243-54.
18. Gawron JH, Keoleian GA, De Kleine RD, Wallington TJ, Kim HC. Deep decarbonization from electrified autonomous taxi fleets: life cycle assessment and case study in Austin, TX. Transp Res D Transp Environ 2019;73:130-41.
19. Naumov S, Keith DR, Fine CH. Unintended consequences of automated vehicles and pooling for urban transportation systems. Prod Oper Manag 2020;29:1354-71.
20. Loeb B, Kockelman KM. Fleet performance and cost evaluation of a shared autonomous electric vehicle (SAEV) fleet: a case study for Austin, Texas. Transp Res Part A Policy Pract 2019;121:374-85.
21. Soteropoulos A, Berger M, Ciari F. Impacts of automated vehicles on travel behaviour and land use: an international review of modelling studies. Transp Rev 2019;39:29-49.
23. Wadud Z, Mackenzie D, Leiby P. Help or hindrance? The travel, energy and carbon impacts of highly automated vehicles. Transp Res Part A Policy Pract 2016;86:1-18.
24. Huang Y, Kockelman KM, Quarles N. How will self-driving vehicles affect U.S. megaregion traffic? The case of the Texas triangle. 2020. Available from: https://www.caee.utexas.edu/prof/kockelman/public_html/TRB19TXMegaregionAVs.pdf [Last accessed on 22 Dec 2023].
25. Gurumurthy KM, Kockelman KM. Analyzing the dynamic ride-sharing potential for shared autonomous vehicle fleets using cellphone data from Orlando, Florida. Comput Environ Urban Syst 2018;71:177-85.
26. Wellik T, Griffin J, Kockelman K, Mohamed M. Utility-transit nexus: leveraging intelligently charged electrified transit to support a renewable energy grid. Renew Sustain Energy Rev 2021;139:110657.
27. Gurumurthy KM, Dean MD, Kockelman KM. Strategic charging of shared fully-automated electric vehicle (SAEV) fleets in a large-scale model. 2021. Available from: https://www.researchgate.net/publication/347945624_Strategic_Charging_of_Shared_Fully-Automated_Electric_Vehicle_SAEV_Fleets_in_a_Large-Scale_Model [Last accessed on 22 Dec 2023].
28. How many miles does a car last? 2021. Available from: https://www.caranddriver.com/research/a32758625/how-many-miles-does-a-car-last/ [Last accessed on 22 Dec 2023].
29. FOTW #1046, September 10, 2018: the average household vehicle was driven 10,200 miles in 2017. Available from: https://www.energy.gov/eere/vehicles/articles/fotw-1046-september-10-2018-average-household-vehicle-was-driven-10200-miles [Last accessed on 22 Dec 2023].
30. Rodrigue JP, Dablanc L, Giuliano G. The freight landscape: convergence and divergence in urban freight distribution. J Transp Land Use 2017;10:557-72.
Cite This Article
How to Cite
Download Citation
Export Citation File:
Type of Import
Tips on Downloading Citation
Citation Manager File Format
Type of Import
Direct Import: When the Direct Import option is selected (the default state), a dialogue box will give you the option to Save or Open the downloaded citation data. Choosing Open will either launch your citation manager or give you a choice of applications with which to use the metadata. The Save option saves the file locally for later use.
Indirect Import: When the Indirect Import option is selected, the metadata is displayed and may be copied and pasted as needed.
About This Article
Copyright
Data & Comments
Data
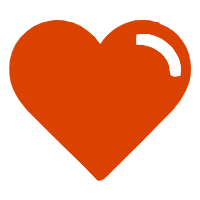
Comments
Comments must be written in English. Spam, offensive content, impersonation, and private information will not be permitted. If any comment is reported and identified as inappropriate content by OAE staff, the comment will be removed without notice. If you have any queries or need any help, please contact us at [email protected].