Artificial intelligence in abdominal wall reconstruction: where are we now and where are we going?
Abstract
From novel reconstructive operations to the creation of negative pressure wound therapy, plastic surgery is defined by a rich history of surgical and technological innovation. One of the latest technologies changing the face of medicine is artificial intelligence (AI), with its increasing popularity embodied by the meteoric rise in AI-related publications over the last decade. Abdominal wall reconstruction (AWR) is a discipline within plastic surgery that has taken an interest in AI technology, incorporating it into research to better understand hernia outcomes, interpret preoperative data, and improve patient-specific care and education. This review aims to explore the current breadth of AI use within AWR to give readers a better understanding of where the field currently stands and inspire ideas for where it may go in the future.
Keywords
INTRODUCTION
Artificial intelligence (AI) has taken the world by storm, integrating itself into our everyday lives with better technology seeming to emerge by the day. Medicine, specifically surgery, has been quick to adopt this disruptive technology, finding various ways to incorporate it into research and practice. The volume of AI publications within surgical fields has increased drastically in the last decade without signs of slowing down[1]. Numerous early studies have shown the benefits of AI within medicine, surgery, and, specifically, plastic surgery, including database analysis, image analysis, predictive algorithms, computer vision, and intraoperative guidance[2-8].
To understand the various applications of AI algorithms, it is important to first develop a basic understanding of the types of AI algorithms available and the terminology currently in use. AI, machine learning (ML), and deep learning (DL) are just a few of the oft-confused terms currently in use. While AI is a general term that captures any technology mimicking human intelligence, ML and DL are subtypes of AI, with unique characteristics distinguishing their algorithms from one another[9,10]. ML is a form of AI where models learn to perform tasks without prior instruction, learning only from the dataset and the output of interest. However, ML requires time-intensive feature extraction and the identification of key features for the algorithm to focus on to help guide the algorithm. DL models, on the other hand, do not require feature extraction; instead, they identify important features independently. The tradeoff is that DL models generally require larger data sets and higher computing power, with the added difficulty of determining what features the model actually finds important[9,11,12]. Both ML and DL models can be either supervised or unsupervised. Supervised models are given labeled outputs, meaning the model knows the outcome and has the goal of creating a map from the input to the output. Supervised models are more frequently employed in medicine, where a specific question is trying to be answered. Unsupervised learning entails giving a model unlabeled data, serving a more exploratory purpose where the model tries to identify unknown structures and relationships within the data[11]. With this basic understanding of AI, we can better interpret the types of models being created and used within plastic surgery.
Abdominal wall reconstruction (AWR) is an expansive field that crosses general and plastic surgery, and as a specialty, it has produced significant research to provide patients with reproducible, reliable, and lasting outcomes. Ventral and incisional hernias are commonplace, with incisional hernias estimated to occur in more than 1 in 8 patients after laparotomy. Thus, hernia repair and AWR are among the most common surgical procedures performed across the globe[13-15]. With the increasing complexity of hernia repair, there is a concomitant increase in complications, with a higher incidence of wound complications, infections, and recurrences[16-18]. Described as a “vicious cycle of complications”, it has become well understood that complications after AWR and hernia repair, specifically wound complications and infection, lead to recurrence, and reoperation for hernia recurrence incurs added risk of complications and recurrence with each operation[16].
With the emergence of AI technology, AWR, a subspecialty of medicine with the need for such quality outcomes, and surgeons motivated to study and pursue these outcomes, is a field that is well positioned to take advantage of the technological disruption of AI in medicine. This review aims to summarize the current body of evidence for the use of AI in AWR and evaluate the future directions and new applications of AI technology in the field.
AI DATABASE ANALYSIS
A clear early indication of the use of AI within any field is database analysis, due to AI algorithms’ immense power and speed in the analysis of large datasets. This trend has held true in AWR, where AI has been used to detect long-term infections following inguinal, umbilical, and ventral hernia repairs[19]. Using a ML algorithm, O’Brien et al. were able to analyze 96,435 inguinal, umbilical, and ventral hernia repairs with synthetic mesh for the occurrence of a skin and soft tissue infection related to their hernia repair over a five-year period. The model performed very well in discriminating between patients with and without infection. The authors believe their model could aid in future outcomes research by decreasing administrative burden in assessing infectious outcomes over extended periods of time, as well as possibly automating surveillance programs for identifying patients with an infection[19].
In 2022, Hassan et al. published their work on using AI to predict complications following AWR. Utilizing a dataset of 725 patients and nine supervised ML algorithms, they aimed to preoperatively predict hernia recurrence, surgical site occurrences, and 30-day readmission following AWR. Their algorithms demonstrated good discriminatory performance for predicting hernia recurrence and 30-day readmission measured by area under the receiver operating curve (AUC), with AUCs of 0.71 and 0.75, respectively. Additionally, their algorithms were able to identify many unique significant predictors for hernia recurrence, surgical site occurrences, and 30-day readmission. This study was an early example of an AI algorithm using preoperative clinical data to predict postoperative outcomes in AWR - a tool that the authors conjecture could help in providing patient-specific risk assessment and preoperative counseling[20].
In 2023, two studies focused on using AI to identify risk factors for hernia development rather than outcome prediction[21,22]. Ortega-Deballon et al. investigated the predictors of hernia formation. Utilizing a large national database, they evaluated 710,074 patients who underwent abdominal surgery, looking for predictors of hernia recurrence by analyzing the patients who subsequently underwent at least one incisional hernia repair within five years. They utilized both a Cox multivariable analysis and a ML analysis. The study identified several risk factors for the development of incisional hernia, including age, length of hospital stay, laparoscopy vs. laparotomy, and intraabdominal surgical site. When focusing on the ML evaluation, pancreatic operations and colorectal operations were the procedures with the highest risk of incisional hernia repair within five years. Age, laparotomy, and obesity also proved to be important risk factors, even in surgical sites with lower risks of eventual need for hernia repair[21].
Choi et al. similarly used ML database analysis to evaluate the risk of hernia occurrence after laparoscopic cholecystectomy. Their main aim was to evaluate if there was an increased risk of post-laparoscopy incisional hernia for index operations performed at a teaching hospital. Utilizing multiple different ML algorithms, they evaluated 117,570 laparoscopic cholecystectomy cases and the risk factors that led to subsequent hernia development. They found that surgery performed at a teaching hospital did not increase the risk of post-laparoscopy hernia formation. However, ML did identify other patient- and hospital-specific factors that impacted the risk of incisional hernia development both positively and negatively[22].
AI IMAGING ANALYSIS
While database analysis using AI, such as in the studies detailed above, can afford more efficient and timely analysis of small to large datasets, imaging analysis can potentially open even more doors in AWR research. Medical imaging is comprised of a wealth of data, nuances of which, such as slight differences in shades of grey, can be imperceptible to the human eye. AI can analyze images not just at record speeds compared to humans, but can also assess changes and differences in images that humans cannot appreciate[23,24].
The first study utilizing AI imaging analysis in the hernia and AWR population was by Elhage et al. in 2021. This also happens to be the first use of DL algorithms in the field of AWR research. The authors utilized DL algorithms to analyze preoperative computed tomography (CT) scans of 369 patients, with the goals of predicting the need for component separation (a proxy for surgical complexity) and predicting postoperative surgical site infections and pulmonary failure. Using preoperative images alone, the surgical complexity and surgical site infection models performed very well, with AUCs of 0.744 and 0.898, respectively. Additionally, the surgical complexity model outperformed a panel of expert surgeons on a separate validation set, with an accuracy of 81.3% compared to the expert surgeon accuracy of 65.0%. However, the model for predicting pulmonary failure was unsuccessful, with an AUC of 0.545, and this was thought to be due to the low sample size of patients who developed postoperative pulmonary failure[25].
A follow-up study by Ayuso et al. in 2023 aimed to utilize specialized techniques to improve the accuracy of DL models for predicting rare but often devastating outcomes in AWR. Querying a database of 510 patients, they utilized framework-augmented DL models that incorporated image augmentation and anomaly detection, as a way to give an algorithm more data for learning when original unique data may be limited. Despite the variables of interest occurring at low rates, pulmonary failure at 5.6% and mesh infection at 3.7%, they were able to successfully predict these outcomes with the augmented DL models. In comparison, these models significantly outperformed conventional DL models assessing the same outcomes[26].
In 2022, McAuliffe et al. utilized ML to successfully predict the development of incisional hernia after colorectal operations. Rather than AI analyzing images on its own, the authors identified features on preoperative CT images, 21 of which were capable of discriminating between incisional hernia and non-incisional hernia patients. With these features, they utilized ML to generate predictive algorithms based on three unique pathophysiologic domains: structural widening of the rectus complex, increased visceral volume, and atrophy of the abdominopelvic skeletal muscle. Based on the importance of these domains, the authors hypothesized a mechanism for hernia formation based on the premise of early fascial microgap and failed healing, in the setting of decreased fascial integrity and higher forces on the fascia via increased intra-abdominal pressure. Work such as this can not only help with the prediction of hernia development, but can also improve our understanding of the underlying pathophysiology[27]. Furthermore, this study highlights important traits of supervised ML algorithms, where the significance of specific features can be uncovered, as opposed to DL models where feature significance is often hidden in a black box[11].
Talwar et al. similarly aimed to predict the risk of hernia development after abdominal operations, utilizing optimal biomarkers identified on preoperative CT imaging to train their predictive ML model. Optimal biomarker methodology can utilize unstructured data to “identify a small subset from a large set of features that are both discriminative for the outcome of interest and independent from other features”. Using this technique, their most successful ML model was able to predict incisional hernia formation well, with an AUC of 0.85, accuracy of 0.83, sensitivity of 0.86, and specificity of 0.81[28].
An interesting study by Wilson et al. published in 2024 was the first in the hernia literature to compare three DL predictive models using images alone, clinical data alone (age, sex, body mass index, diabetes status, and history of tobacco use), and a combined model using images and clinical data. All three models were found to be poorly predictive of hernia recurrence; however, a noteworthy conclusion from this study was that the DL model based only on clinical data outperformed the image-based model and the combined model. This observation suggests that certain outcomes may benefit more from image analysis or clinical data, and that the combination of the two does not necessarily purport an additive effect in terms of accuracy[29].
GENERATIVE AI
Generative AI is a fascinating field within AI that focuses on AI’s ability to create new content. Much of the AI technology that has infiltrated our day-to-day lives, such as Chatbots and music-, image-, and video-generating software, are examples of generative AI. There has been much speculation as to the future implications of generative AI on medicine and within AWR specifically, though the field remains ripe for study[30]. Though there has been very little published on the use of generative AI within AWR, momentum appears to be building. A recent study published in 2024 by Lima et al. aimed to evaluate the appropriateness of recommendations from a publicly available Chatbot to common questions about ventral hernia repair. Based on an expert review of the Chatbot’s answers to 23 questions, 74% of answers were deemed to be appropriate by a majority of the reviewers. However, the authors highlighted known difficulties with generative AI in the medical field, one importantly being the lack of, or the generation of, false citations. A specific area where the Chatbot struggled in this scenario was in discussing the use of mesh and surgical approaches. While these findings are exciting and encouraging, the technology remains far from being applied in clinical practice[31].
FUTURE DIRECTIONS
The future of AI in AWR is exciting and we are merely at the tip of the iceberg. Much of future research will be focused not only on improving upon current algorithms and developing new ones, but also on how we can best validate these algorithms to ensure their safety[32]. One can imagine a future where AI assistance occurs at every level of surgical patient care: as an adjunct in preoperative decision making, for assistance and guidance in the operating room, and even in the postoperative setting for early detection of complications. Beyond simply creating new algorithms to answer questions or make predictions, the surgical community must focus efforts on studying the best ways to deploy this technology into our daily workflows, ensuring that we are using AI not only to improve patient outcomes, but also to improve surgeon efficiency.
Another key direction for future research in the realm of AI and AWR will certainly be robotics. While the implementation of computer vision into robotic platforms and autonomous robotics is beyond the scope of this review, research has already started in the realm of AWR[33]. Studies have begun to assess AI’s ability for phase recognition within robotic inguinal hernia repair; however, this is proving difficult, and will only be more difficult for AWR outside of inguinal hernia repair due to significant heterogeneity in case steps, order of operations, and unique surgical anatomy[34]. Beyond computer vision and autonomous robotic platforms, AI research can influence the future of robotics by assisting with defining indications and predicting outcomes for robotic versus open AWR. Current research, including randomized controlled trials, has not shown significant differences in outcomes between the two modalities, leaving room for AI analytical technology to potentially answer this question[35].
The future of AI lies not just in database and imaging analysis, but also in generative AI, which is poised to play a larger and larger role in our clinical practice. While the aforementioned AWR-specific study evaluates a chatbot’s response to questions, other fields in plastic surgery are pushing the envelope with generative AI technologies. Within plastic surgery, generative AI has been used to generate consent material and answer surgeon questions for intraoperative assistance[36,37]. While this technology is far from being ready to be used clinically, it is exciting to picture a world where it will be seamlessly integrated into the framework of our daily practice. Future studies focused on generative AI in AWR can utilize this technology to improve the consent process and create new and improved mechanisms to educate patients and disseminate medical information.
CONCLUSION
AI will continue to grow its presence in medicine as technology improves and our research into the field deepens. AWR as a specialty has embraced this technology and is using it to better study and predict hernia occurrence and AWR outcomes. Our review highlights key studies in the field, encompassing database analysis, image analysis, and generative AI, and we encourage AWR surgeons to pursue future AI research.
DECLARATIONS
Authors’ contributions
Involved in study designs, critically drafting, and editing the manuscript: Elhage SA, Terry PH, Villavisanis D, Fischer JP, Percec I
Availability of data and materials
Publicly available.
Financial support and sponsorship
None.
Conflicts of interest
Fischer JP reports receiving funding from 3M, Becton, Dickinson, Integra, Gore, and Allergan for speaking and teaching, honoraria, and consulting fees, as well as National Center for Advancing Translational Sciences of the National Institutes of Health, R01 grant. Percec I is a consultant and trainer for Allergan and Galderma and a consultant for AlumierMD and Pierre Fabre Dermocosmetique. The other authors declared that there are no conflicts of interest.
Ethical approval and consent to participate
Not applicable.
Consent for publication
Not applicable.
Copyright
© The Author(s) 2025.
REFERENCES
1. Lima DL, Kasakewitch J, Nguyen DQ, et al. Machine learning, deep learning and hernia surgery. Are we pushing the limits of abdominal core health? A qualitative systematic review. Hernia. 2024;28:1405-12.
2. Chilamkurthy S, Ghosh R, Tanamala S, et al. Deep learning algorithms for detection of critical findings in head CT scans: a retrospective study. Lancet. 2018;392:2388-96.
3. Gong D, Wu L, Zhang J, et al. Detection of colorectal adenomas with a real-time computer-aided system (ENDOANGEL): a randomised controlled study. Lancet Gastroenterol Hepatol. 2020;5:352-61.
4. Gulshan V, Peng L, Coram M, et al. Development and validation of a deep learning algorithm for detection of diabetic retinopathy in retinal fundus photographs. JAMA. 2016;316:2402-10.
5. Esteva A, Kuprel B, Novoa RA, et al. Dermatologist-level classification of skin cancer with deep neural networks. Nature. 2017;542:115-8.
6. Hassan AM, Biaggi-Ondina A, Asaad M, et al. Artificial intelligence modeling to predict periprosthetic infection and explantation following implant-based reconstruction. Plast Reconstr Surg. 2023;152:929-38.
7. Mavioso C, Araújo RJ, Oliveira HP, et al. Automatic detection of perforators for microsurgical reconstruction. Breast. 2020;50:19-24.
8. Kenig N, Monton Echeverria J, De la Ossa L. Identification of key breast features using a neural network: applications of machine learning in the clinical setting of plastic surgery. Plast Reconstr Surg. 2024;153:273e-80e.
9. Loftus TJ, Tighe PJ, Filiberto AC, et al. Artificial intelligence and surgical decision-making. JAMA Surg. 2020;155:148-58.
10. Gumbs AA, Parretta S, d’Allemagne B, Chouillard E. What is artificial intelligence surgery? Artif Intell Surg. 2021;1:1-10.
11. Choi RY, Coyner AS, Kalpathy-Cramer J, Chiang MF, Campbell JP. Introduction to machine learning, neural networks, and deep learning. Transl Vis Sci Technol. 2020;9:14. Available from: https://www.researchgate.net/publication/344419928_Introduction_to_Machine_Learning_Neural_Networks_and_Deep_Learning. [Last accessed on 22 May 2025]
12. TerKonda SP, TerKonda AA, Sacks JM, et al. Artificial intelligence: singularity approaches. Plast Reconstr Surg. 2024;153:204e-17e.
14. Deerenberg EB, Harlaar JJ, Steyerberg EW, et al. Small bites versus large bites for closure of abdominal midline incisions (STITCH): a double-blind, multicentre, randomised controlled trial. Lancet. 2015;386:1254-60.
15. Poulose BK, Shelton J, Phillips S, et al. Epidemiology and cost of ventral hernia repair: making the case for hernia research. Hernia. 2012;16:179-83.
16. Holihan JL, Alawadi Z, Martindale RG, et al. Adverse events after ventral hernia repair: the vicious cycle of complications. J Am Coll Surg. 2015;221:478-85.
17. Maloney SR, Schlosser KA, Prasad T, et al. Twelve years of component separation technique in abdominal wall reconstruction. Surgery. 2019;166:435-44.
18. Heniford BT, Ross SW, Wormer BA, et al. Preperitoneal ventral hernia repair: a decade long prospective observational study with analysis of 1023 patient outcomes. Ann Surg. 2020;271:364-74.
19. O’Brien WJ, Ramos RD, Gupta K, Itani KMF. Neural network model to detect long-term skin and soft tissue infection after hernia repair. Surg Infect. 2021;22:668-74.
20. Hassan AM, Lu SC, Asaad M, et al. Novel machine learning approach for the prediction of hernia recurrence, surgical complication, and 30-day readmission after abdominal wall reconstruction. J Am Coll Surg. 2022;234:918-27.
21. Ortega-Deballon P, Renard Y, de Launay J, Lafon T, Roset Q, Passot G. Incidence, risk factors, and burden of incisional hernia repair after abdominal surgery in France: a nationwide study. Hernia. 2023;27:861-71.
22. Choi JH, Janjua H, Cios K, et al. Machine learning analysis of postlaparoscopy hernias and “I’m leaving you to close” strategy. J Surg Res. 2023;290:171-7.
23. Esteva A, Robicquet A, Ramsundar B, et al. A guide to deep learning in healthcare. Nat Med. 2019;25:24-9.
24. Rajpurkar P, Lungren MP. The current and future state of AI interpretation of medical images. N Engl J Med. 2023;388:1981-90.
25. Elhage SA, Deerenberg EB, Ayuso SA, et al. Development and validation of image-based deep learning models to predict surgical complexity and complications in abdominal wall reconstruction. JAMA Surg. 2021;156:933-40.
26. Ayuso SA, Elhage SA, Zhang Y, et al. Predicting rare outcomes in abdominal wall reconstruction using image-based deep learning models. Surgery. 2023;173:748-55.
27. McAuliffe PB, Desai AA, Talwar AA, et al. Preoperative computed tomography morphological features indicative of incisional hernia formation after abdominal surgery. Ann Surg. 2022;276:616-25.
28. Talwar AA, Desai AA, McAuliffe PB, et al. Optimal computed tomography-based biomarkers for prediction of incisional hernia formation. Hernia. 2024;28:17-24.
29. Wilson HH, Ma C, Ku D, et al. Deep learning model utilizing clinical data alone outperforms image-based model for hernia recurrence following abdominal wall reconstruction with long-term follow up. Surg Endosc. 2024;38:3984-91.
30. Mayol J. Transforming abdominal wall surgery with generative artificial intelligence. J Abdom Wall Surg. 2023;2:12419.
31. Lima DL, Nogueira R, Liu J, et al. How appropriate are recommendations of online chat-based artificial intelligence (ChatGPT) to common questions on ventral hernia repair? J Laparoendosc Adv Surg Tech A. 2024;34:365-7.
32. Haug CJ, Drazen JM. Artificial intelligence and machine learning in clinical medicine, 2023. N Engl J Med. 2023;388:1201-8.
33. Restrepo-Rodas G, Barajas-Gamboa JS, Ortiz Aparicio FM, et al. The role of AI in modern hernia surgery: a review and practical insights. Surg Innov. 2025;32:301-11. Available from: https://pubmed.ncbi.nlm.nih.gov/40104921/. [Last accessed on 22 May 2025]
34. Choksi S, Szot S, Zang C, et al. Bringing artificial intelligence to the operating room: edge computing for real-time surgical phase recognition. Surg Endosc. 2023;37:8778-84.
35. Warren JA, Blackhurst D, Ewing JA, Carbonell AM. Open versus robotic retromuscular ventral hernia repair: outcomes of the ORREO prospective randomized controlled trial. Surg Endosc. 2024;38:7466-74.
36. Atkinson CJ, Seth I, Xie Y, et al. Artificial intelligence language model performance for rapid intraoperative queries in plastic surgery: ChatGPT and the deep inferior epigastric perforator flap. J Clin Med. 2024;13:900.
Cite This Article

How to Cite
Download Citation
Export Citation File:
Type of Import
Tips on Downloading Citation
Citation Manager File Format
Type of Import
Direct Import: When the Direct Import option is selected (the default state), a dialogue box will give you the option to Save or Open the downloaded citation data. Choosing Open will either launch your citation manager or give you a choice of applications with which to use the metadata. The Save option saves the file locally for later use.
Indirect Import: When the Indirect Import option is selected, the metadata is displayed and may be copied and pasted as needed.
About This Article
Special Issue
Copyright
Data & Comments
Data
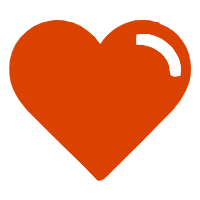
Comments
Comments must be written in English. Spam, offensive content, impersonation, and private information will not be permitted. If any comment is reported and identified as inappropriate content by OAE staff, the comment will be removed without notice. If you have any queries or need any help, please contact us at [email protected].