A machine learning-driven model predicts patient-reported dysphagia after instrumented cervical fusion
Abstract
Aim: We aim to develop a machine learning (ML)-driven predictive algorithm for patient-reported dysphagia after instrumented cervical fusion. Additionally, we aim to identify features for the prediction of dysphagia and to develop a web-based risk calculator for outcome prediction.
Methods: We identified consecutive adults who underwent instrumented cervical fusion at a single institution between 2013-2020. We developed regression-based and ML-based prognostic models and assessed model performance using discrimination and calibration. Additionally, we identified patient features driving the performance of the most effective model.
Results: Nine hundred and forty-seven patients were included in this study. There were 62 cases of dysphagia. The gradient boosting model was well-calibrated and demonstrated the highest discrimination of all tested models. The most important features for model performance included: anterior approach, deformity, revision procedure, bipolar disorder, diabetes mellitus, depression/anxiety, male sex, and myelopathy.
Conclusion: We report a ML-driven model that accurately predicts patient-reported dysphagia after instrumented cervical fusion. Prediction of dysphagia risk may inform preoperative counseling and appropriate risk stratification. Furthermore, this model may identify modifiable risk factors that may be addressed preoperatively to reduce the risk of dysphagia after cervical fusion.
Keywords
INTRODUCTION
The number of cervical fusion procedures has been increasing in recent decades due to an aging population as well as an increasing prevalence of degenerative cervical spine pathology[1,2]. One of the most common perioperative complications associated with cervical fusion is dysphagia, defined as difficulty or discomfort with swallowing. Dysphagia is common after anterior cervical spine surgery; it is also observed after posterior approaches, albeit less often[3-6]. Reported rates of dysphagia display wide variation, ranging from 2% to 83%[3-5,7-11]. Dysphagia tends to be a transient complication, typically resolving within 3 months[3,8,9]. In addition to being a source of discomfort to patients, the development of postoperative dysphagia is associated with poor outcomes. Patients who develop dysphagia have significantly decreased physical health status up to 24 months after surgery, even after symptoms resolve[4]. Furthermore, patients who develop dysphagia are more likely to suffer from related complications such as dehydration, malnutrition, and pneumonia[10]. Accurately predicting whether a patient is at high risk for dysphagia is thus of great utility and can allow for preoperative counseling and potential preventative measures[6].
There is a paucity of models predicting the risk of dysphagia after instrumented cervical fusion. The utilization of machine learning (ML) methods has grown for the prediction of clinical outcomes in spinal surgery[12-14]. We aim to develop an ML-driven algorithm for the prediction of patient-reported dysphagia in an institutional cohort undergoing instrumented cervical fusion. Although they are increasingly utilized, ML models carry limitations; the complex algorithms underlying ML models often sacrifice interpretability for predictive power. Therefore, we also aim to identify the features important for risk prediction. Finally, our goal is to develop a web-based risk calculator that provides individualized dysphagia risk estimates.
METHODS
Study design and subjects
Our institutional review board approved a waiver of consent for this retrospective study. All patients ≥ 18 years who underwent instrumented cervical spinal fusion at our academic health system, which includes two tertiary care hospitals, between 2013-2020 were included. Revision procedures were excluded if new instrumentation was not placed. Patients with < 2 weeks of follow-up were excluded to ensure that cases of delayed-onset dysphagia were not missed. The cohort was identified by querying case schedules and reviewing operative notes from the electronic medical record.
Outcome and other variables
The primary outcome measure was postoperative dysphagia, defined as patient-reported pain or difficulty with swallowing within the index admission or in follow-up encounters within one month postoperatively. We extracted the following explanatory features: procedure performed, number of fused levels, surgical approach, surgical indication, preoperative motor deficit, preoperative neurologic symptoms, medical and psychiatric comorbidities, body mass index, and smoking history. We also extracted preoperative laboratory values if obtained within 30 days prior to index cervical fusion. Preoperative use of antidepressants, benzodiazepines, and opioids was additionally documented.
Continuous variables were included if < 30% of values were missing. Multiple imputation was performed with MissForest. The rates of missing data for white blood cell (WBC) count and hemoglobin were 4.3%
Model development and evaluation
We built logistic regression and four ML models: XGBoost, AdaBoost, gradient boosting, and random forest. These models were chosen as they represent established ML modeling approaches; they span regression, boosting, tree-based, and ensemble modeling. Five-fold stratified cross-validation was used to avoid model overfitting. Logistic regression, random forest, and AdaBoost models were built using the scikit-learn Python library. XGBoost was built using the xgboost Python library. For random forest, AdaBoost, and gradient boosting models, the number and trees were chosen from the set {50, 100, 200, 300}. For XGBoost, the number of trees and maximum depth of each tree were selected from sets {50, 100, 200, 300} and {2, 3, 4, 5}, respectively.
Discrimination measures how accurately a model distinguishes between patients who did and did not develop dysphagia. We assess discrimination by the area under the receiver operating characteristic curve (AUROC). AUROC represents the probability that a randomly selected patient who developed dysphagia was assigned a higher risk probability by the model than a patient who did not experience this complication. Calibration signifies the agreement between the model’s predictions and observed outcomes in the study population. The calibration slope measures the spread of model prediction; a slope of 1 indicates perfect spread. Calibration intercept is a measure of whether a model overestimates or underestimates the probability of an outcome. A perfect model has a calibration intercept of 0[15,16].
Anaconda Distribution (Anaconda, Inc. Austin, TX), R version 3.5.1 (The R Foundation, Vienna, Austria), and Python version 3.6 (Python Software Foundation, Wilmington, DE) were used for analysis.
Feature importance
To aid the interpretation of the predictions issued by the models, we utilize a partial dependence function that measures the importance of a feature to model performance[17].
Risk calculator
The best-performing survival and classification models on the testing cohort were deployed as a web-based application with Streamlit (Bozeman, MT), an open-access platform.
RESULTS
Cohort characteristics and demographics
Nine hundred and forty-seven patients were included in this study, with a median age of 60 years; over half of the patients (56.8%) were male. Four hundred and fifty-four patients (47.9%) underwent an anterior approach and 458 (48.4%) underwent a posterior approach. Thirty-five patients (3.7%) underwent a combined anterior/posterior approach. A total of 489 patients (51.6%) thus underwent an anterior approach - either isolated or combined anterior/posterior. A median number of two levels were fused. Junctional fusion crossing the cervicothoracic junction was performed in 97 patients (9.5%).
The most common surgical indication was myelopathy (39.2%), followed by trauma (10.5%) and degenerative disc disease (3.6%). Deformity and infection were indications for 2.4% and 1.2% of patients, respectively. The most common medical comorbidities were diabetes mellitus (19.5%), hypothyroidism (14.1%), and malignancy (6.7%). One hundred and three patients (10.9%) were in an immunosuppressed state (e.g., immunodeficiency, immunosuppressive drugs, steroids). The median Charlson comorbidity index was 2. Depression and/or anxiety were present in 249 patients (26.3%) and 12 patients (1.3%) suffered from bipolar disorder.
With respect to preoperative symptoms, 550 patients (58.1%) had paresthesias and 535 (56.5%) had radicular pain. Four hundred and sixty-two patients (48.8%) had preoperative motor weakness. Sixty-two patients (6.1%) reported postoperative dysphagia. The median hospitalization length was 3 days. Cohort characteristics and outcomes are detailed in Table 1.
Cohort characteristics
Feature | All patients (n = 947) |
Median (IQR) | |
Age (years) | 60 (50, 69) |
Body mass index (kg/m2) | 26.9 (24.6, 29.1) |
Number of fused levels | 2 (1, 3) |
Preoperative WBC count (1,000/mL) | 6.9 (5.6, 8.4) |
Preoperative hemoglobin (g/dL) | 13.7 (12.7, 14.8) |
Charlson comorbidity index | 2 (1, 3) |
Number (%) | |
Male | 538 (56.8) |
Approach | |
Isolated posterior | 458 (48.4) |
Isolated anterior approach | 454 (47.9) |
Combined anterior/posterior | 35 (3.7) |
Any anterior approach | 489 (51.6) |
Surgical indications | |
Myelopathy | 371 (39.2) |
Trauma | 99 (10.5) |
Degenerative disc disease | 34 (3.6) |
Deformity | 23 (2.4) |
Infection | 11 (1.2) |
Revision | 44 (4.6) |
Medical/psychiatric comorbidities | |
Diabetes mellitus | 185 (19.5) |
Hypothyroidism | 134 (14.1) |
Immunosuppressed state | 103 (10.9) |
Malignancy | 63 (6.7) |
Dialysis dependence | 17 (1.8) |
Depression and/or anxiety | 249 (26.3) |
Bipolar disorder | 12 (1.3) |
Preoperative symptoms | |
Paresthesias | 550 (58.1) |
Radicular pain | 535 (56.5) |
Motor weakness | 462 (48.8) |
Dysphagia | 62 (6.5) |
Median (IQR) | |
Hospitalization length (days) | 3 (1, 5) |
Model performances
Algorithms for predicting dysphagia after instrumented cervical fusion were built with logistic regression, XGBoost, AdaBoost, gradient boosting, and random forest. The gradient boosting model demonstrates the highest discrimination (AUROC 0.766 ± 0.048) and is well-calibrated with a calibration slope of 1.082 and a calibration intercept of -0.012. The logistic regression model is poorly calibrated, with a calibration slope of 196.278 and a calibration intercept of -11.926. We additionally calculated negative and positive predictive values with a sensitivity-based threshold where the gradient boosting model’s cut-off is adjusted to achieve a sensitivity of 0.8; the negative predictive value was 0.983 ± 0.003 and the positive predictive value was 0.136 ± 0.025. Discrimination and calibration statistics are detailed in Table 2. The receiver-operating characteristic curves for the gradient boosting and logistic regression models are depicted in Figure 1.
Figure 1. Receiver operating characteristic curves for gradient boosting and logistic regression models.
Discrimination statistics of tested models
Model | AUROC | Calibration slope | Calibration intercept |
Logistic regression | 0.691 ± 0.035 | 196.278 | -11.926 |
XGBoost | 0.717 ± 0.039 | 0.3285 | 0.0447 |
Gradient boosting | 0.766 ± 0.048 | 1.082 | -0.012 |
AdaBoost | 0.596 ± 0.105 | 0.1717 | 0.0277 |
Random forest | 0.735 ± 0.039 | 0.5214 | 0.0210 |
Feature importance
To determine relative feature importance, we applied a partial dependence function on the cross-validation fold in which the greatest performance gain of the gradient boosting model was noted. The importance of each feature to gradient boosting model performance is shown in Table 3. The most important features to gradient boosting model performance include degenerative disc disease, revision procedure, surgical approach, spinal trauma, comorbid psychiatric conditions, diabetes mellitus, and the presence of preoperative paresthesias.
Relative feature importance for gradient boosting
Feature | Rank | Change to risk prediction |
Binary features | ||
Anterior approach | 1 | 0.080 |
Deformity | 2 | 0.057 |
Revision procedure | 3 | 0.043 |
Bipolar disorder | 4 | 0.041 |
Diabetes mellitus | 5 | 0.012 |
Depression and/or anxiety | 6 | 0.006 |
Male sex | 7 | 0.005 |
Cervical myelopathy | 8 | 0.003 |
Continuous features | ||
Preoperative hemoglobin | 1 | 0.058 |
Age | 2 | 0.052 |
Preoperative WBC count | 3 | 0.026 |
Risk calculator
The gradient boosting model was incorporated into a risk calculator: https://chl88856-risk-calculator-dysphagia.streamlit.app/. Users may input feature values and receive a probability of dysphagia after instrumented cervical fusion.
DISCUSSION
Dysphagia is a common and disabling complication after cervical spine surgery. Although often a transient complication, dysphagia is associated with poor clinical outcomes[4,10]. Most studies that have identified predictors for dysphagia after cervical spine surgery have employed multivariable logistic regression[3-5,8-10,18-22]. ML methods recognize underlying nonlinear and factor-factor interactions in datasets through iterative learning processes, often detecting relationships between variables that regression techniques may not be able to[23,24]. ML has been sparingly applied for the prediction of specific complications after cervical fusion; to our knowledge, ML modeling techniques have not been used for the prediction of dysphagia[13,25].
There have been multiple studies performed identifying risk factors for postoperative dysphagia. These include preoperative and intraoperative risk factors[26]. Preoperative factors include sex, age, smoking status, recurrent laryngeal nerve injury, and pre-existing dysphagia[26-29]. Intraoperative factors include surgical approach, operative time, and number of levels operated on[9,30]. This study expands on the literature in two primary ways. The aforementioned studies utilize multivariable logistic regression to identify risk factors. We perform the first ML-driven analysis for the prediction of dysphagia after cervical fusion. Furthermore, while the existing literature provides important data regarding factors that may influence the risk of postoperative dysphagia, they do not provide the probability of dysphagia. While other studies have identified risk factors for dysphagia, we provide a risk calculator that clinicians can consult to predict the preoperative risk of dysphagia. To our knowledge, this is the first such risk calculator in the literature that quantifies the risk of dysphagia.
With a cohort of 947 consecutive patients, we report an ML-driven model that accurately predicts patient-reported dysphagia after instrumented cervical fusion. This gradient boosting model is well-calibrated and displays high discrimination with an AUROC of 0.766. To facilitate preoperative risk stratification, we include only preoperative patient characteristics in the model. Additionally, we identify the patient features that are most important to model performance.
Anterior approach to the cervical spine is the most important feature for model performance. The anterior approach is widely recognized as a risk factor for dysphagia[3,4,21,31]. Anterior cervical fusion requires retraction of the esophagus, a known risk factor for developing dysphagia[21,32]. Additionally, a combined approach to the cervical spine has been shown to have overall higher complication rates, including dysphagia, compared to isolated anterior or posterior approaches[10,11].
We find that surgical indication is important for model performance. Surgery for deformity was the 2nd most important feature for the model. Cervical deformity procedures may result in more trauma to anterior and posterior soft tissues due to increased retractor time and changes in tension after deformity correction. These are also longer surgeries with larger exposures and higher intraoperative blood loss - all factors associated with the development of dysphagia[3,20,21]. Revision procedure was the 3rd most important feature. Patients undergoing revision surgery are more likely to undergo complex surgery with scar tissue complicating the approach, leading to increased operative time, soft tissue injury, and multilevel fusion. These factors are associated with an increased risk of swallowing difficulty after cervical fusion[6,8,9,20,21,31]. Patients with cervical spondylotic myelopathy have been hypothesized to have an increased risk of dysphagia, likely due to the chronicity of degenerative disease with osteophytic changes required to develop progressive myelopathy from spondylosis[18]. Additionally, local inflammation from the degenerative uncovertebral disease may increase preoperative prevertebral swelling, a known risk factor for postoperative dysphagia[21].
Finally, medical and psychiatric comorbidities were identified as important features for dysphagia risk prediction. Diabetes mellitus was the 5th most important feature and is a known risk factor for adverse outcomes after spinal surgery[33,34]. Bipolar disorder and depression/anxiety were the 4th and 6th most important features, respectively. Patients with mood disorders and other psychiatric comorbidities have been shown to have lower rates of satisfaction and higher rates of perioperative complications across spinal surgery[35]. Kang et al. showed that the presence of psychiatric factors is associated with persistent dysphagia after single-level anterior cervical fusion; however, bipolar disorder has not been specifically shown to be associated with dysphagia[36]. Patients with psychiatric disorders are more likely to report higher rates of acute pain with longer duration of symptoms[35]. Increased duration of pain is a risk factor for the development of dysphagia[4]. Providers should thus screen patients for psychiatric conditions such as bipolar disorder and depression/anxiety and attempt to optimize management prior to elective surgery by ensuring appropriate psychiatric follow-up; these features may influence the risk of postoperative dysphagia. We identify male sex as the 7th most feature for model performance. The role of patient sex in postoperative dysphagia is debated and reported inconsistently. While studies by Lee et al. and Cho et al. have identified female sex as a risk factor for dysphagia, sex does not have an association with dysphagia in a study by Riley et al.[4,8,27].
The current study has limitations, the first of which is its retrospective single-center design. Selection bias exists in this cohort as it is comprised of patients for whom it was perceived that the benefit of surgery would outweigh the risks. An additional source of selection bias is that surgeons may have avoided anterior cervical fusion - or cervical fusion altogether - in patients for whom a high risk of dysphagia was suspected. While our observed dysphagia rate of 6.5% is consistent with the existing retrospective literature, it has been shown that when dysphagia is assessed prospectively, the reported rates are notably higher[3,7,37]. Additionally, validated measures of dysphagia such as videofluoroscopic swallow evaluation or standardized questionnaires were not employed in this study. Radiographic alignment parameters, operative time, or specific levels (i.e., upper versus lower cervical spine) were not included as explanatory features. While recurrent laryngeal nerve injury is a known risk factor for dysphagia, the injury was not typically noted intraoperatively, nor was it specifically investigated for postoperatively. Relying on subjective patient-reported symptoms may represent a source of bias. Finally, overfitting is a concern when building any prognostic model; this is especially the case with ML modeling. We attempt to protect against overfitting with our model development and validation strategy; however, external validation studies are necessary to establish generalizability.
We report an ML algorithm with high discrimination and calibration that accurately predicts patient-reported dysphagia after instrumented cervical fusion at a single institution. This represents the first ML model predicting dysphagia after cervical fusion. By providing an accurate estimate of the risk for developing dysphagia, the reported model can improve preoperative shared decision making and facilitate more informed patient counseling. We identify potentially modifiable patient features such as diabetes and psychiatric comorbidities that may be preoperatively optimized for elective procedures to potentially reduce the risk of developing dysphagia. We additionally provide a user-friendly risk calculator that providers can consult to determine patient-specific risk of dysphagia. This tool can be employed during the preoperative evaluation to provide a probability of this complication and better counsel patients regarding the risks and benefits of the planned surgery.
For patients with a high predicted risk of dysphagia, preventative or mitigating measures can be implemented. If appropriate decompression and fusion can be performed through either an anterior or posterior approach, the surgeon may consider proceeding with a posterior approach in patients with a high risk of dysphagia. Preoperative tracheal/esophageal traction exercises may improve tracheal and esophageal compliance, reducing the risk of dysphagia[38]. Multiple intraoperative measures can be taken as well. Reduction in endotracheal tube cuff pressure after placement of retractors during anterior cervical exposure may decrease the risk of postoperative dysphagia[39]. Minimizing esophageal retraction time and using dynamic retractors instead of self-retaining retractors may decrease the risk of postoperative dysphagia by reducing ischemic changes to the tracheal mucosa[6,40]. The use of a low-profile smooth anterior cervical plate or a zero-profile implant may reduce the risk of postoperative prevertebral swelling with associated dysphagia[41,42]. Additionally, avoiding routine use of recombinant bone morphogenetic proteins (BMPs) may reduce dysphagia risk; intraoperative use of BMPs may lead to dysphagia by increasing the incidence of postoperative swelling[6,43]. Systemic administration or local injection of corticosteroids to the retropharyngeal region after closure may reduce the risk of dysphagia by decreasing esophageal inflammation and soft-tissue swelling[44]. In addition to preoperative and intraoperative measures, specific postoperative measures may also be taken in those with a high risk of developing dysphagia. For example, these patients may benefit from a soft diet immediately postoperatively with a slower advancement of diet and low threshold to obtain swallow studies.
Potential future avenues of research include re-training this reported algorithm on multi-center data collected with standardized measures of dysphagia. Additionally, external validation studies are necessary to determine the generalizability of this algorithm.
DECLARATIONS
Authors’ contributions
Made substantial contributions to the conception and design of the study and performed data analysis and interpretation: Shah AA, Bugarin A, Lee C, Devana SK, Upfill-Brown A
Provided administrative, technical, and material support: SooHoo NF, Park DY
Availability of data and materials
The data cannot be shared in its original form in order to protect patient confidentiality. De-identified data may be shared upon reasonable request.
Financial support and sponsorship
The research reported in this publication was supported by the National Institute of Arthritis and Musculoskeletal and Skin Diseases of the National Institutes of Health under the Ruth L. Kirschstein National Research Service Award Number T32AR059033 and the H&H Lee Research Program. The content is solely the responsibility of the authors and does not necessarily represent the official views of the National Institutes of Health.
Conflicts of interest
All authors declared that there are no conflicts of interest.
Ethical approval and consent to participate
The UCLA institutional review board approved a waiver of consent for this retrospective study. The authors confirmed that consent had been waived for this retrospective study.
Consent for publication
Not applicable.
Copyright
© The Author(s) 2025.
REFERENCES
1. Wang MC, Kreuter W, Wolfla CE, Maiman DJ, Deyo RA. Trends and variations in cervical spine surgery in the United States: medicare beneficiaries, 1992 to 2005. Spine. 2009;34:955-61; discussion 962.
2. Memtsoudis SG, Hughes A, Ma Y, Chiu YL, Sama AA, Girardi FP. Increased in-hospital complications after primary posterior versus primary anterior cervical fusion. Clin Orthop Relat Res. 2011;469:649-57.
3. Smith-Hammond CA, New KC, Pietrobon R, Curtis DJ, Scharver CH, Turner DA. Prospective analysis of incidence and risk factors of dysphagia in spine surgery patients: comparison of anterior cervical, posterior cervical, and lumbar procedures. Spine. 2004;29:1441-6.
4. Riley LH 3rd, Skolasky RL, Albert TJ, Vaccaro AR, Heller JG. Dysphagia after anterior cervical decompression and fusion: prevalence and risk factors from a longitudinal cohort study. Spine. 2005;30:2564-9.
5. Tervonen H, Niemelä M, Lauri ER, et al. Dysphonia and dysphagia after anterior cervical decompression. J Neurosurg Spine. 2007;7:124-30.
6. Joaquim AF, Murar J, Savage JW, Patel AA. Dysphagia after anterior cervical spine surgery: a systematic review of potential preventative measures. Spine J. 2014;14:2246-60.
7. Bazaz R, Lee MJ, Yoo JU. Incidence of dysphagia after anterior cervical spine surgery: a prospective study. Spine. 2002;27:2453-8.
8. Lee MJ, Bazaz R, Furey CG, Yoo J. Risk factors for dysphagia after anterior cervical spine surgery: a two-year prospective cohort study. Spine J. 2007;7:141-7.
9. Rihn JA, Kane J, Albert TJ, Vaccaro AR, Hilibrand AS. What is the incidence and severity of dysphagia after anterior cervical surgery? Clin Orthop Relat Res. 2011;469:658-65.
10. Chen CJ, Saulle D, Fu KM, Smith JS, Shaffrey CI. Dysphagia following combined anterior-posterior cervical spine surgeries. J Neurosurg Spine. 2013;19:279-87.
11. Nagoshi N, Tetreault L, Nakashima H, et al. Risk factors for and clinical outcomes of dysphagia after anterior cervical surgery for degenerative cervical myelopathy: results from the AOSpine International and North America studies. J Bone Joint Surg Am. 2017;99:1069-77.
12. Hopkins BS, Yamaguchi JT, Garcia R, et al. Using machine learning to predict 30-day readmissions after posterior lumbar fusion: an NSQIP study involving 23,264 patients. J Neurosurg Spine. 2020;32:399-406.
13. Arvind V, Kim JS, Oermann EK, Kaji D, Cho SK. Predicting surgical complications in adult patients undergoing anterior cervical discectomy and fusion using machine learning. Neurospine. 2018;15:329-37.
14. Shah AA, Devana SK, Lee C, et al. Machine learning-driven identification of novel patient factors for prediction of major complications after posterior cervical spinal fusion. Eur Spine J. 2022;31:1952-9.
15. Steyerberg EW, Vergouwe Y. Towards better clinical prediction models: seven steps for development and an ABCD for validation. Eur Heart J. 2014;35:1925-31.
16. Karhade AV, Thio QCBS, Ogink PT, et al. Development of machine learning algorithms for prediction of 30-day mortality after surgery for spinal metastasis. Neurosurgery. 2019;85:E83-91.
17. Friedman JH. Greedy function approximation: a gradient boosting machine. Ann Statist. 2001;29:1189-232.
18. Wang T, Ma L, Yang DL, et al. Factors predicting dysphagia after anterior cervical surgery: a multicenter retrospective study for 2 years of follow-up. Medicine. 2017;96:e7916.
19. Perez-Roman RJ, Luther EM, McCarthy D, et al. National trends and correlates of dysphagia after anterior cervical discectomy and fusion surgery. Neurospine. 2021;18:147-54.
20. Danto J, DiCapua J, Nardi D, et al. Multiple cervical levels: increased risk of dysphagia and dysphonia during anterior cervical discectomy. J Neurosurg Anesthesiol. 2012;24:350-5.
21. Liu J, Hai Y, Kang N, Chen X, Zhang Y. Risk factors and preventative measures of early and persistent dysphagia after anterior cervical spine surgery: a systematic review. Eur Spine J. 2018;27:1209-18.
22. Siska PA, Ponnappan RK, Hohl JB, Lee JY, Kang JD, Donaldson WF 3rd. Dysphagia after anterior cervical spine surgery: a prospective study using the swallowing-quality of life questionnaire and analysis of patient comorbidities. Spine. 2011;36:1387-91.
23. Hashimoto DA, Rosman G, Rus D, Meireles OR. Artificial intelligence in surgery: promises and perils. Ann Surg. 2018;268:70-6.
24. Chen JH, Asch SM. Machine learning and prediction in medicine - beyond the peak of inflated expectations. N Engl J Med. 2017;376:2507-9.
25. Wang KY, Suresh KV, Puvanesarajah V, Raad M, Margalit A, Jain A. Using predictive modeling and machine learning to identify patients appropriate for outpatient anterior cervical fusion and discectomy. Spine. 2021;46:665-70.
26. Le HV, Javidan Y, Khan SN, Klineberg EO. Dysphagia after anterior cervical spine surgery: pathophysiology, diagnosis, and management. J Am Acad Orthop Surg. 2024;32:627-36.
27. Cho SK, Lu Y, Lee DH. Dysphagia following anterior cervical spinal surgery: a systematic review. Bone Joint J. 2013;95-B:868-73.
28. Gould FD, Lammers AR, Ohlemacher J, et al. The physiologic impact of unilateral recurrent laryngeal nerve (RLN) lesion on infant oropharyngeal and esophageal performance. Dysphagia. 2015;30:714-22.
29. Nguyen S, Sherrod BA, Paziuk TM, et al. Predictors of dysphagia after anterior cervical discectomy and fusion: a prospective multicenter study. Spine. 2022;47:859-64.
30. Vaishnav AS, Saville P, McAnany S, et al. Predictive factors of postoperative dysphagia in single-level anterior cervical discectomy and fusion. Spine. 2019;44:E400-7.
31. Li Z, Li G, Chen C, et al. Risk factors for dysphagia after anterior cervical spine surgery. Orthopedics. 2018;41:e110-6.
32. Papavero L, Heese O, Klotz-Regener V, Buchalla R, Schröder F, Westphal M. The impact of esophagus retraction on early dysphagia after anterior cervical surgery: does a correlation exist? Spine. 2007;32:1089-93.
33. Golinvaux NS, Varthi AG, Bohl DD, Basques BA, Grauer JN. Complication rates following elective lumbar fusion in patients with diabetes: insulin dependence makes the difference. Spine. 2014;39:1809-16.
34. Lee NJ, Kothari P, Phan K, et al. Incidence and risk factors for 30-day unplanned readmissions after elective posterior lumbar fusion. Spine. 2018;43:41-8.
35. Jackson KL, Rumley J, Griffith M, Agochukwu U, DeVine J. Correlating psychological comorbidities and outcomes after spine surgery. Global Spine J. 2020;10:929-39.
36. Kang SS, Lee JS, Shin JK, Lee JM, Youn BH. The association between psychiatric factors and the development of chronic dysphagia after anterior cervical spine surgery. Eur Spine J. 2014;23:1694-8.
37. McAfee PC, Cappuccino A, Cunningham BW, et al. Lower incidence of dysphagia with cervical arthroplasty compared with ACDF in a prospective randomized clinical trial. J Spinal Disord Tech. 2010;23:1-8.
38. Chen Z, Wei X, Li F, et al. Tracheal traction exercise reduces the occurrence of postoperative dysphagia after anterior cervical spine surgery. Spine. 2012;37:1292-6.
39. Ratnaraj J, Todorov A, McHugh T, Cheng MA, Lauryssen C. Effects of decreasing endotracheal tube cuff pressures during neck retraction for anterior cervical spine surgery. J Neurosurg. 2002;97:176-9.
40. Mendoza-Lattes S, Clifford K, Bartelt R, Stewart J, Clark CR, Boezaart AP. Dysphagia following anterior cervical arthrodesis is associated with continuous, strong retraction of the esophagus. J Bone Joint Surg Am. 2008;90:256-63.
41. Lee MJ, Bazaz R, Furey CG, Yoo J. Influence of anterior cervical plate design on Dysphagia: a 2-year prospective longitudinal follow-up study. J Spinal Disord Tech. 2005;18:406-9.
42. Qi M, Chen H, Liu Y, Zhang Y, Liang L, Yuan W. The use of a zero-profile device compared with an anterior plate and cage in the treatment of patients with symptomatic cervical spondylosis: a preliminary clinical investigation. Bone Joint J. 2013;95-B:543-7.
43. Bellamy JT, Dilbone E, Schell A, et al. Prospective comparison of dysphagia following anterior cervical discectomy and fusion (ACDF) with and without rhBMP-2. Spine J. 2022;22:256-64.
Cite This Article

How to Cite
Download Citation
Export Citation File:
Type of Import
Tips on Downloading Citation
Citation Manager File Format
Type of Import
Direct Import: When the Direct Import option is selected (the default state), a dialogue box will give you the option to Save or Open the downloaded citation data. Choosing Open will either launch your citation manager or give you a choice of applications with which to use the metadata. The Save option saves the file locally for later use.
Indirect Import: When the Indirect Import option is selected, the metadata is displayed and may be copied and pasted as needed.
About This Article
Special Issue
Copyright
Data & Comments
Data
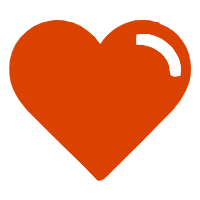
Comments
Comments must be written in English. Spam, offensive content, impersonation, and private information will not be permitted. If any comment is reported and identified as inappropriate content by OAE staff, the comment will be removed without notice. If you have any queries or need any help, please contact us at [email protected].