Machine learning applications in adult spinal deformity corrective surgery: a narrative review
Abstract
Adult spinal deformity (ASD) poses significant challenges in spinal surgery, requiring precise planning and execution for successful correction. Additionally, optimization of outcomes and reducing the high complication rates of ASD surgeries are additional challenges facing spinal deformity surgeons. The advent of machine learning (ML) has revolutionized various aspects of healthcare, including spinal surgery. This review provides a comprehensive overview of the current state of ML applications in spinal deformity corrective surgery, highlighting its potential benefits and challenges.
Keywords
INTRODUCTION
With the aging population, the incidence and prevalence of adult spinal deformity (ASD) are on the rise[1], affecting millions worldwide and significantly impacting their quality of life. This often leads to the necessity of complex surgical interventions. Planning ASD surgery involves evaluating not only the entire spinal column but also the entirety of the skeleton to ensure appropriate radiographic alignment. ASD patients present with a variety of heterogeneous clinical manifestations, and there is a vast array of surgical methods available for their treatment, making the treatment algorithm quite complex. Additionally, ASD surgery is associated with high complication rates in both the short and long term. These observations make ASD an ideal candidate for leveraging the significant potential offered by artificial intelligence and machine learning (ML).
Computational techniques have been used in the past several years to process large datasets and create complex mathematical models to determine the relationship between different variables affecting the outcomes of surgery. The idea behind ML, a subset of artificial intelligence, is to develop a system similar to the human brain to learn from clinical and radiographic data and apply the knowledge to new situations. In other words, ML employs computer algorithms to learn from data and past experiences, enabling the creation of intelligent models. These algorithms enable computers to identify patterns in datasets without relying on predefined rules, allowing them to learn relationships from the data and make predictions or decisions based on that knowledge. It has been shown that validated ML risk calculators can provide more accurate and objective prognoses to adjust patient expectations during patient care than expert surgeons’ perception of risks in ASD surgery[2]. The development of predictive models via ML algorithms for prognosticating patient outcomes following ASD surgery represents a significant advancement over traditional statistical models, which are more adept at identifying statistical associations between variables rather than providing predictive value[3].
ML has shown promise in enhancing the accuracy and efficiency of various medical procedures, including spinal surgery. By taking advantage of large datasets and advanced algorithms, ML can assist surgeons in preoperative planning, intraoperative decision-making, and postoperative care, leading to improved patient outcomes.
The aim of this narrative review is to provide an overview of the current status of ML in enhancing spinal deformity correction surgery and its applicability in preplanning, intraoperative guidance, predictive modeling, and postoperative risk assessment.
METHODOLOGY
The authors conducted a non-systematic review of recent literature to support their perspectives on the applicability of ML in corrective spine surgery for adult ASD. This narrative review addresses three key stages in surgical practice [Figure 1] where ML can be impactful, and concludes by discussing the major challenges and future directions in the field.
Figure 1. An overview of the most common applications of ML in ASD. ML: Machine learning; ASD: adult spinal deformity.
Preoperative planning
Appropriate preoperative patient selection significantly impacts patient satisfaction, individualized decision-making by surgeons, and hospital resource utilization. Identifying patients with favorable outcomes preoperatively is a challenging task. Traditional statistical methods, such as multiple regression analyses, are better suited for hypothesis testing rather than predicting individual patient outcomes. In contrast, ML algorithms can readily identify patterns within large datasets without the need to test a specific hypothesis[4]. However, this advantage of ML algorithms comes at the cost of interpretability. Predictive models generated by ML are more difficult to interpret than risk factors identified by traditional statistical tests[3].
ASD patients exhibit significant heterogeneity in demographics, comorbidities, spinal pathologies, and genetic factors. Traditional outcome predictive models often overlook these individual variabilities, leading to suboptimal predictions. However, ML models excel in accounting for these differences. By analyzing comprehensive datasets that include detailed individual patient profiles, these models can generate personalized predictions, enhancing clinical decision-making and patient outcomes.
ML algorithms can analyze preoperative imaging studies, such as X-rays, CT scans, and MRI scans, to provide detailed insights into the patients’ spinal alignments[5-7]. This includes assessing the degree of deformity, identifying critical structures, and predicting the optimal surgical approach[8]. ML models can also assist in selecting the appropriate implants (like pre-bent patient-specific rods) and predicting the postoperative spinal alignment, helping surgeons customize their surgical plan for each patient[9,10]. Using ML algorithms, a group of investigators could accurately predict spinopelvic parameters and thoracic kyphosis after deformity correction surgery in 20 adult patients with spinal deformity[11]. ML models can preoperatively be used to estimate the likelihood of extended length of stay following ASD surgery[12,13]. Thus, the surgeon can optimize modifiable risk factors, enhance preoperative planning, and manage patients’ expectations. Other investigators have developed predictive models to estimate the risk of rehabilitation discharge for adult patients undergoing elective surgeries, including ASD patients[14].
Lafage et al. used artificial neural network based on preoperative data and alignment goals to accurately (81%) predict the upper instrumented vertebra (UIV) in a series of 143 ASD patients. This study showed how “to employ a neural network to mimic surgeon decision-making for UIV selection”[15]. A neural network is a type of ML model inspired by the human brain’s structure and functioning. It consists of interconnected nodes or neurons organized into layers: an input layer, one or more hidden layers, and an output layer. Each connection between neurons has a weight that adjusts during training to minimize error.
Additionally, prognosis can be predicted by using ML algorithms to identify different patient phenotypes preoperatively. In a recent prospective multi-center study on 570 ASD patients conducted by European and US-based Spine Study Group, investigators could identify three different qualitative preoperative phenotypes in ASD patients based on demographics, surgical history, frailty, radiographic measures, and patient-reported outcome measures. These phenotypes had been identified through unsupervised machine-based clustering. Based on these phenotypes, one can augment preoperative decision-making, predict the clinical outcome of deformity surgery (prognostic values), and tailor treatment approaches[16].
An international team of researchers used a predictive ML model preoperatively to predict the individual answers to the Scoliosis Research Society-22R (SRS-22R) questionnaire at 1 and 2 years after ASD surgery in 561 patients. This prediction provides the patients with reasonable preoperative counseling based on their expectations and perceptions of the corrective surgery clinical outcomes[17]. Mekhael et al. used a random forest ML model to accurately predict health-related quality of life outcomes after ASD surgery in 173 patients. They found that three-dimensional movement assessment of ASD patients can better predict clinical outcomes than stand-alone radiographic parameters, not only for physical but also for mental scores[18]. Random forest is an ensemble learning method that constructs multiple decision trees during training and merges their results to improve accuracy and control overfitting.
In another study, the researchers used a “ML model based on random forest regression and a systematic decision tree-like approach” to predict health-related quality of life scores, gait kinematics, and spatial-temporal parameters based on radiographic global alignment parameters[19]. They found that Global Sagittal Angle[20] and T9 tilt[21] were the best predictors of joint kinematics and health-related quality of life scores based on the results from 127 primary ASD patients and 47 controls.
Conditional inference tree run ML analysis was used to identify the baseline threshold for different radiographic parameters to achieve a good outcome following ASD surgery in 431 patients. These parameters were: sagittal vertical axis, pelvic incidence-lumbar lordosis mismatch, pelvic tilt, T1 pelvic angle, L1 pelvic angle, L4-S1 lordosis, C2-C7 sagittal vertical axis, C2-T3, C2 slope[22]. Conditional inference tree is a type of decision tree used in ML that addresses some of the biases found in traditional decision trees. It helps to make decisions by asking questions about data and splitting it accordingly, aiming to improve predictions and analyses.
Aiming to preoperatively predict proximal junctional kyphosis (PJK) after ASD corrective surgery in 191 patients, a team of researchers included preoperative thoracic T1 MRIs in a deep learning ML model (convolutional neural network) to increase the accuracy of the prediction[23]. Using a large prospective multi-center database, a group of investigators constructed a supervised ensemble of decision trees to preoperatively predict the risk of pseudarthrosis at 2 years after ASD surgery in 336 patients with 91% accuracy[24].
Intraoperative guidance
During surgery, ML algorithms can provide surgeons with real-time guidance, enhancing the accuracy of instrument placement and overall surgical technique. By integrating with navigation systems, ML can track the position of surgical instruments relative to the patient’s anatomy, ensuring precise correction of spinal deformity. Using ML methodology, Burström et al. were able to accurately place pedicle screws during CT-based navigation[25]. Preplanning the pedicle screw trajectory using the ML system has yielded highly accurate results[26]. ML can also be used to analyze intraoperative data, such as neuromonitoring signals, to alert surgeons of potential complications, such as nerve injury, enabling prompt intervention. Real-time automated decision-making systems regularly integrate inputs from intraoperative neuromonitoring and the operating room environment, utilizing predictive models to generate instructions or warnings for the surgical team. These systems continuously update their predictive models and decision-making processes based on new data and feedback from the surgeon and neurophysiologist, ensuring adaptive and accurate responses during surgery[27].
Using perioperative data, ML-based risk calculators can predict the 30-day complication and mortality risk following ASD corrective surgery in 9,143 patients from The American College of Surgeons National Surgical Quality Improvement Program (ACS-NSQIP) database[28]. Kim et al. could use ML algorithms to predict mortality and medical complications following ASD surgery. Using the data of 4,073 patients queried from the ACS-NSQIP database, they found that ML algorithms outperformed American Society of Anesthesiologists score in predicting individual risk prognosis[29].
Using conditional inference tree analysis, a team of investigators could predict blood loss and perioperative blood transfusion in 909 ASD patients undergoing surgery[30]. The artificial neural network was used to predict perioperative blood transfusion after ASD corrective surgery in 1,173 cases identified from the NSQIP database between 2017 and 2019, with 81% accuracy[31]. Furthermore, another group of researchers found no difference between random forest and tree-based ML models to predict blood transfusion following ASD corrective surgery in 1,029 patients[32].
A team of researchers used ML-based predictive models to estimate the likelihood of overall improvement and surpassing the minimal clinically important difference (MCID) following adult spinal deformity (ASD) surgery, testing their models with eight patient-reported outcome measure instruments. The models could predict accurately and consistently whether a procedure would achieve MCID for a given patient using a given outcome instrument across a given time interval[33,34].
Postoperative care
After ASD surgery, ML can aid in monitoring patients’ recovery and predicting potential complications. By analyzing postoperative imaging[35] and clinical data, ML models can identify early signs of implant failure, infection, or other complications, allowing for timely intervention[36]. Since ASD surgery is fraught with complications postoperatively, many different characterizations have been developed to predict the complications after the surgery or determine risk profiles for the development of complications following deformity correction. The success of computer vision, large language models, and genome-wide association (incorporating advanced ML technologies) in predicting various complications in a cohort of ASD patients has been shown recently by a group of investigators[37]. Major medical complications, discharge to a facility, and 90-day readmission were predicted using ML methods with decent accuracy[38,39].
ML can also assist in predicting long-term complications, such as the risk of adjacent segment degeneration and PJK, and help surgeons and patients make informed decisions about follow-up care. Korean investigators recently developed and verified an online calculator for predicting PJK risk following ASD surgery using a ML model. They based their study on the radiographic outcomes obtained from 16 surgical centers[40]. Moreover, to predict mechanical complications following ASD surgery, some investigators tried different ML models and found that random forest had the best prediction accuracy of 73.2%[41]. Additionally, in a postoperatively well-aligned group of 244 patients following ASD surgery, some researchers could predict the mechanical complications with moderate accuracy (74%) using extreme gradient boosting ML algorithms. The mechanical complications investigated were: proximal junctional kyphosis and failure, distal junctional kyphosis and failure, rod breakage, and implant-related complications[42]. Extreme gradient boosting is a technique that builds a strong predictive model by combining several weaker models, learning from mistakes, and doing so in a very efficient way.
Lovecchio et al. used decision tree analysis to predict the risk of proximal junctional failure and PJK by studying pre-discharge standing radiographs of 117 ASD patients[43]. A group of Korean investigators could identify risk factors for unplanned readmission after ASD in 210 patients and predict it using a ML model[44].
Some researchers used a conditionally unbiased regression tree and random forest algorithm to predict cost outliers in ASD correction up to 2 years after the index surgery in 210 patients[45]. Conditionally unbiased regression tree is a type of decision tree used for making predictions, particularly when numbers or continuous data are studied. It avoids bias by splitting data into different groups based on certain conditions.
Challenges and future directions
Despite its potential benefits, the integration of ML into spinal deformity correction surgery faces several challenges. These include the need for large, high-quality datasets, the interpretability of ML models, and the ethical and regulatory implications of ML algorithmic decision-making[46,47]. Some investigators have suggested using biological samples (muscle and bone sampling, assessment of circulating biomarkers,…) to improve the accuracy of ML predictions in the future[48,49]. Furthermore, most current studies employ a random split approach, in which the majority (70%-90%) of the available data are used for training the model, while the remaining 10%-30% for testing its performance. This approach is not generally deemed sufficient for the aim of “external” validation[50]. Moreover, the extent to which ML-based predictions meaningfully affect clinical decisions and practices in real life has yet to be investigated. Future research should focus on addressing these challenges, as well as exploring new applications of ML, such as personalized surgical planning and robotic-assisted surgery, to further improve patient outcomes.
For spine surgeons embarking on ML collaborations, key considerations include:
1. Data quality and privacy: ensure high-quality, well-annotated data while adhering to patient privacy regulations like HIPAA.
2. Interdisciplinary communication: foster clear communication between clinicians and data scientists to bridge the gap between medical expertise and technical execution.
3. Clinical relevance: focus on models that address specific clinical challenges, ensuring they provide actionable insights in surgical planning, outcome prediction, or patient monitoring.
4. Validation and bias: rigorously validate ML models in diverse clinical settings to avoid biases and ensure generalizability.
5. Regulatory compliance: stay informed about the evolving regulatory landscape for AI in healthcare to ensure compliance with FDA or other relevant bodies.
These considerations are crucial for developing impactful and ethical ML solutions in spine surgery.
CONCLUSION
ML has the potential to revolutionize spinal deformity correction surgery by enhancing preoperative planning, intraoperative guidance, and postoperative care. By leveraging the power of large datasets and advanced algorithms, ML can assist surgeons in achieving more precise and personalized surgical outcomes, ultimately benefiting patients with spinal deformities.
DECLARATIONS
Authors’ contributions
Made substantial contributions to the conception and design of the study and drafted the manuscript: Toossi N
Performed literature search, as well as providing administrative, technical, and material support: Jerry O
Availability of data and materials
Not applicable.
Financial support and sponsorship
None.
Conflicts of interest
Both authors declared that there are no conflicts of interest.
Ethical approval and consent to participate
Not applicable.
Consent for publication
Not applicable.
Copyright
© The Author(s) 2024.
REFERENCES
1. Schwab F, Dubey A, Gamez L, et al. Adult scoliosis: prevalence, SF-36, and nutritional parameters in an elderly volunteer population. Spine 2005;30:1082-5.
2. Pellisé F, Vila-Casademunt A, Núñez-Pereira S, et al; European Spine Study Group, International Spine Study Group. Surgeons’ risk perception in ASD surgery: the value of objective risk assessment on decision making and patient counselling. Eur Spine J 2022;31:1174-83.
3. Joshi RS, Lau D, Scheer JK, et al. State-of-the-art reviews predictive modeling in adult spinal deformity: applications of advanced analytics. Spine Deform 2021;9:1223-39.
4. Akosman I, Lovecchio F, Lyons K, et al. The emerging role of artificial intelligence in adult spinal deformity. Semin Spine Surg 2022;34:100986.
5. Haselhuhn JJ, Soriano PBO, Grover P, et al. Spine surgeon versus AI algorithm full-length radiographic measurements: a validation study of complex adult spinal deformity patients. Spine Deform 2024;12:755-61.
6. Harake ES, Linzey JR, Jiang C, et al. Development and validation of an artificial intelligence model to accurately predict spinopelvic parameters. J Neurosurg Spine 2024;41:88-96.
7. Suri A, Tang S, Kargilis D, et al. Conquering the cobb angle: a deep learning algorithm for automated, hardware-invariant measurement of cobb angle on radiographs in patients with scoliosis. Radiol Artif Intell 2023;5:e220158.
8. Galbusera F, Niemeyer F, Wilke HJ, et al. Fully automated radiological analysis of spinal disorders and deformities: a deep learning approach. Eur Spine J 2019;28:951-60.
9. Faulks CR, Biddau DT, Munday NR, McKenzie DP, Malham GM. Patient-specific spinal rods in adult spinal deformity surgery reduce proximal junctional failure: a review of patient outcomes and surgical technique in a prospective observational cohort. J Spine Surg 2023;9:409-21.
10. Picton B, Stone LE, Liang J, et al. Patient-specific rods in adult spinal deformity: a systematic review. Spine Deform 2024;12:577-85.
11. Lee NJ, Sardar ZM, Boddapati V, et al. Can machine learning accurately predict postoperative compensation for the uninstrumented thoracic spine and pelvis after fusion from the lower thoracic spine to the sacrum? Global Spine J 2022;12:559-66.
12. Arora A, Demb J, Cummins DD, Callahan M, Clark AJ, Theologis AA. Predictive models to assess risk of extended length of stay in adults with spinal deformity and lumbar degenerative pathology: development and internal validation. Spine J 2023;23:457-66.
13. Li W, Zhang Y, Zhou X, et al. Ensemble learning-assisted prediction of prolonged hospital length of stay after spine correction surgery: a multi-center cohort study. J Orthop Surg Res 2024;19:112.
14. Arora A, Lituiev D, Jain D, et al. Predictive models for length of stay and discharge disposition in elective spine surgery: development, validation, and comparison to the ACS NSQIP risk calculator. Spine 2023;48:E1-13.
15. Lafage R, Ang B, Alshabab BS, et al. Predictive model for selection of upper treated vertebra using a machine learning approach. World Neurosurg 2021;146:e225-32.
16. Mohanty S, Hassan FM, Lenke LG, et al; International Spine Study Group. Machine learning clustering of adult spinal deformity patients identifies four prognostic phenotypes: a multicenter prospective cohort analysis with single surgeon external validation. Spine J 2024;24:1095-108.
17. Ames CP, Smith JS, Pellisé F, et al; European Spine Study Group; International Spine Study Group. Development of predictive models for all individual questions of SRS-22R after adult spinal deformity surgery: a step toward individualized medicine. Eur Spine J 2019;28:1998-2011.
18. Mekhael E, El Rachkidi R, Saliby RM, et al. Functional assessment using 3D movement analysis can better predict health-related quality of life outcomes in patients with adult spinal deformity: a machine learning approach. Front Surg 2023;10:1166734.
19. Nassim N, Mekhael E, Rachkidi RE, et al. Global Sagittal Angle and T9-tilt seem to be the most clinically and functionally relevant global alignment parameters in patients with Adult Spinal Deformity. Brain Spine 2024;4:102805.
20. Diebo BG, Oren JH, Challier V, et al. Global sagittal axis: a step toward full-body assessment of sagittal plane deformity in the human body. J Neurosurg Spine 2016;25:494-9.
21. Legaye J, Duval-Beaupere G. Sagittal plane alignment of the spine and gravity: a radiological and clinical evaluation. Acta Orthop Belg 2005;71:213-20.
22. Williamson TK, Krol O, Tretiakov P, et al. Crossing the bridge from degeneration to deformity: when does sagittal correction impact outcomes in adult spinal deformity surgery? Spine 2023;48:E25-32.
23. Johnson GW, Chanbour H, Ali MA, et al. Artificial intelligence to preoperatively predict proximal junction kyphosis following adult spinal deformity surgery: soft tissue imaging may be necessary for accurate models. Spine 2023;48:1688-95.
24. Scheer JK, Oh T, Smith JS, et al; International Spine Study Group. Development of a validated computer-based preoperative predictive model for pseudarthrosis with 91% accuracy in 336 adult spinal deformity patients. Neurosurg Focus 2018;45:E11.
25. Burström G, Buerger C, Hoppenbrouwers J, et al. Machine learning for automated 3-dimensional segmentation of the spine and suggested placement of pedicle screws based on intraoperative cone-beam computer tomography. J Neurosurg Spine 2019;31:147-54.
26. Siemionow KB, Forsthoefel CW, Foy MP, Gawel D, Luciano CJ. Autonomous lumbar spine pedicle screw planning using machine learning: A validation study. J Craniovertebr Junction Spine 2021;12:223-7.
27. Wilson JP Jr, Kumbhare D, Kandregula S, Oderhowho A, Guthikonda B, Hoang S. Proposed applications of machine learning to intraoperative neuromonitoring during spine surgeries. Neurosci Inf 2023;3:100143.
28. Pierce KE, Kapadia BH, Naessig S, et al. Validation of the ACS-NSQIP risk calculator: a machine-learning risk tool for predicting complications and mortality following adult spinal deformity corrective surgery. Int J Spine Surg 2021;15:1210-6.
29. Kim JS, Arvind V, Oermann EK, et al. Predicting surgical complications in patients undergoing elective adult spinal deformity procedures using machine learning. Spine Deform 2018;6:762-70.
30. Raman T, Vasquez-Montes D, Varlotta C, Passias PG, Errico TJ. Decision tree-based modelling for identification of predictors of blood loss and transfusion requirement after adult spinal deformity surgery. Int J Spine Surg 2020;14:87-95.
31. De la Garza Ramos R, Hamad MK, Ryvlin J, et al. An artificial neural network model for the prediction of perioperative blood transfusion in adult spinal deformity surgery. J Clin Med 2022;11:4436.
32. Durand WM, DePasse JM, Daniels AH. Predictive modeling for blood transfusion after adult spinal deformity surgery: a tree-based machine learning approach. Spine 2018;43:1058-66.
33. Ames CP, Smith JS, Pellisé F, et al; European Spine Study Group, International Spine Study Group. Development of deployable predictive models for minimal clinically important difference achievement across the commonly used health-related quality of life instruments in adult spinal deformity surgery. Spine 2019;44:1144-53.
34. Patel AV, White CA, Schwartz JT, et al. Emerging technologies in the treatment of adult spinal deformity. Neurospine 2021;18:417-27.
35. Löchel J, Putzier M, Dreischarf M, et al. Deep learning algorithm for fully automated measurement of sagittal balance in adult spinal deformity. Eur Spine J 2024;Online ahead of print.
36. Hopkins BS, Mazmudar A, Driscoll C, et al. Using artificial intelligence (AI) to predict postoperative surgical site infection: a retrospective cohort of 4046 posterior spinal fusions. Clin Neurol Neurosurg 2020;192:105718.
37. Schonfeld E, Pant A, Shah A, et al. Evaluating computer vision, large language, and genome-wide association models in a limited sized patient cohort for pre-operative risk stratification in adult spinal deformity surgery. J Clin Med 2024;13:656.
38. Jain D, Durand W, Burch S, Daniels A, Berven S. Machine learning for predictive modeling of 90-day readmission, major medical complication, and discharge to a facility in patients undergoing long segment posterior lumbar spine fusion. Spine 2020;45:1151-60.
39. Arora A, Demb J, Cummins DD, et al. Development and internal validation of predictive models to assess risk of post-acute care facility discharge in adults undergoing multi-level instrumented fusions for lumbar degenerative pathology and spinal deformity. Spine Deform 2023;11:163-73.
40. Lee CH, Jo DJ, Oh JK, et al. Development and validation of an online calculator to predict proximal junctional kyphosis after adult spinal deformity surgery using machine learning. Neurospine 2023;20:1272-80.
41. Noh SH, Lee HS, Park GE, et al. Predicting mechanical complications after adult spinal deformity operation using a machine learning based on modified global alignment and proportion scoring with body mass index and bone mineral density. Neurospine 2023;20:265-74.
42. Balaban B, Yilgor C, Yucekul A, et al. Building clinically actionable models for predicting mechanical complications in postoperatively well-aligned adult spinal deformity patients using XGBoost algorithm. Inform Med Unlocked 2023;37:101191.
43. Lovecchio F, Lafage R, Sheikh Alshabab B, et al. Can discharge radiographs predict junctional complications? A decision tree analysis. Global Spine J 2024;14:970-7.
44. Ryu SJ, So JY, Ha Y, et al. Risk factors for unplanned reoperation after corrective surgery for adult spinal deformity. Bone Joint Res 2023;12:245-55.
45. Ames CP, Smith JS, Gum JL, et al. Utilization of predictive modeling to determine episode of care costs and to accurately identify catastrophic cost nonwarranty outlier patients in adult spinal deformity surgery: a step toward bundled payments and risk sharing. Spine 2020;45:E252-65.
46. Wondra JP 2nd, Kelly MP, Greenberg J, et al. Validation of adult spinal deformity surgical outcome prediction tools in adult symptomatic lumbar scoliosis. Spine 2023;48:21-8.
47. Yagi M, Yamanouchi K, Fujita N, Funao H, Ebata S. Revolutionizing spinal care: current applications and future directions of artificial intelligence and machine learning. J Clin Med 2023;12:4188.
48. Haddad S, Pizones J, Raganato R, et al. Future data points to implement in adult spinal deformity assessment for artificial intelligence modeling prediction: the importance of the biological dimension. Int J Spine Surg 2023;17:S34-44.
49. Choy W, Azad TD, Scheer JK, Safaee MM, Ames CP. Biomarkers in adult spinal deformity surgery. Semin Spine Surg 2023;35:101058.
Cite This Article
How to Cite
Download Citation
Export Citation File:
Type of Import
Tips on Downloading Citation
Citation Manager File Format
Type of Import
Direct Import: When the Direct Import option is selected (the default state), a dialogue box will give you the option to Save or Open the downloaded citation data. Choosing Open will either launch your citation manager or give you a choice of applications with which to use the metadata. The Save option saves the file locally for later use.
Indirect Import: When the Indirect Import option is selected, the metadata is displayed and may be copied and pasted as needed.
About This Article
Special Issue
Copyright
Data & Comments
Data
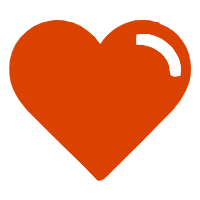
Comments
Comments must be written in English. Spam, offensive content, impersonation, and private information will not be permitted. If any comment is reported and identified as inappropriate content by OAE staff, the comment will be removed without notice. If you have any queries or need any help, please contact us at [email protected].