Application of artificial intelligence to hepatobiliary cancer clinical outcomes research
Abstract
The rapid evolution of modern technology has made artificial intelligence (AI) an important emerging tool in healthcare. AI, which is a broad field of computer science, can be used to develop systems or machines equipped with the ability to tackle tasks that traditionally necessitate human intelligence. AI can be used to perform multifaceted tasks that involve the synthesis of large amounts of data with the generation of solutions, algorithms, and decision support tools. Various AI approaches, including machine learning (ML) and natural language processing (NLP), are increasingly being used to analyze vast healthcare datasets. In addition, visual AI has the potential to revolutionize surgery and the intraoperative experience for surgeons through augmented reality enhancing surgical navigation in real-time. Specific applications of AI in hepatobiliary tumors such as hepatocellular carcinoma and biliary tract cancer can improve patient diagnosis, prognostic risk stratification, as well as treatment allocation based on ML-based models. The integration of radiomics data and AI models can also improve clinical decision making. We herein review how AI may be of particular interest in the care of patients with complex cancers, such as hepatobiliary tumors, as these patients often require a multimodal treatment approach.
Keywords
INTRODUCTION
With the rapid evolution of modern technology, artificial intelligence (AI) has increasingly become integrated into various aspects of human life, particularly the healthcare domain[1,2]. AI, which is a broad field of computer science, can be used to develop systems or machines equipped with the ability to tackle tasks that traditionally necessitate human intelligence[1]. Specifically, AI can be used to perform multifaceted tasks that involve the synthesis of large amounts of data with the generation of solutions, algorithms, and decision support tools[2]. Synthesizing and analyzing large volumes of data, AI can incorporate “unstructured” information including images or text and, in turn, identify a complex interplay among various data points[3,4]. In turn, the integration of AI into healthcare has the potential to yield numerous benefits, including tools to refine patient outcomes, streamline healthcare delivery processes, and inform medical research[5].
AI systems can analyze medical data, such as images, diagnostic tests, and patient records, yielding more accurate tools for diagnosis, as well as prognostically stratifying patients[6,7]. In particular, AI algorithms, which are adept at analyzing large datasets, can identify intricate patterns and trends that are challenging for humans to discern, enabling more accurate identification of disease patterns, as well as prediction of potential health risks. Furthermore, AI holds the promise to prevent or manage diseases more proactively[8,9]. AI can also analyze genetic, clinical, and lifestyle data to facilitate the customization of treatment plans tailored for individual patients[10]. This personalized medicine approach, which more adeptly considers the unique characteristics of each patient, may optimize treatment and increase therapeutic efficacy while minimizing adverse effects. For instance, AI algorithms such as Virtual Twin analysis or Optimal Policy Tree (OPT)[11,12] can differentiate patients into subsets, identifying who may benefit from specific treatment plans, such as upfront surgery versus neoadjuvant or adjuvant chemotherapy[13,14]. Moreover, AI can facilitate drug discovery and selection of chemotherapeutic agents by analyzing massive datasets to predict the most promising drug candidates, as well as potentially optimizing clinical trial designs, thereby reducing the time and cost of developing new drugs to market[15]. Natural Language Processing (NLP) algorithms can also sift through unstructured healthcare data, distilling valuable insights in the electronic health records and medical literature, thus enhancing the accessibility of critical information for healthcare providers and researchers[16]. AI may also help to address healthcare disparities by improving access to medical expertise in underserved or remote areas through AI-powered telemedicine, thereby enabling remote consultations and earlier disease diagnoses[17].
Overall, AI plays a crucial role in healthcare transformation, enhancing diagnostic accuracy, personalizing treatment, expediting research, and enhancing patient care. The ongoing integration of AI technologies holds the promise of revolutionizing healthcare practices and outcomes. AI may be of particular interest in the care of patients with complex cancers such as hepatobiliary (HB) tumors as these patients often require a multimodal treatment approach with surgical resection, as well as therapy with a wide array of different chemotherapy agents[18].
APPLICATION OF AI IN THE MEDICAL FIELD
Different AI approaches to healthcare data
AI can involve various statistical approaches based on different techniques and methodologies. Machine learning (ML) and natural language processing (NLP) are generally among the most used methodologies[19,20]. ML utilizes algorithms and statistical models to refine the performance of designated tasks through experiential learning, while not utilizing explicit factor-based programming. In this way, ML can facilitate the identification of new patterns of knowledge and enhance inferences that enable the forecasting of outcomes or decision tools that are grounded in data. Through the analysis of large amounts of data, ML algorithms facilitate the identification of data patterns and enhance data-based predictions with incrementally improved performance optimization over time. There are various types of ML approaches, such as supervised learning and unsupervised learning[21]. In addition, ML can utilize several different types of algorithms that can recognize patterns within a set of data including artificial neural networks, decision tree algorithms [i.e., random forest (RF), gradient-boosting][22,23], and instance-based algorithms (i.e., support vector machine, k-nearest neighbor)[24,25]. Deep learning (DL) is a subset of ML that involves neural networks with multiple layers (i.e., deep neural networks) and has been demonstrated to be particularly effective in handling complex tasks. DL has gained prominence within the broader field of AI due to its ability to process data in a hierarchical fashion, thereby allowing for more sophisticated and nuanced decision making[26]. DL techniques, particularly convolutional neural networks (CNN), have been applied to radiomics, which is a field of medical imaging that involves extracting a large number of quantitative features from medical images[27]. NLP encompasses the interaction between computers and human language, including tasks such as speech recognition, language translation, sentiment analysis, and language understanding. NLP has given rise to applications such as chatbots, language translation services, and voice-activated assistants[28-30]. NLP enables the handling of vast “unstructured” text-based data, and can enhance the ability to make clinical diagnoses and risk stratification of patients[20,31].
Visual AI
Visual AI involves the utilization of artificial intelligence techniques to analyze and comprehend visual information. Visual AI involves empowering machines to interpret, understand, and make decisions based on visual data (i.e., intraoperative images and videos). Visual AI applications leverage technologies such as computer vision, DL, and various image processing techniques to extract meaningful insights from visual content. The significance of visual AI has gained considerable attention, especially in its potential applications in the field of surgery[32]. For instance, the automation of surgical phase recognition, utilizing AI and computer vision algorithms for image interpretation through DL, has been a subject of recent interest. This application has demonstrated its effectiveness in enhancing surgeon performance in both basic procedures like inguinal hernia repair, as well as complex surgeries such as robot-assisted minimally invasive esophagectomy and hepatectomy[33,34]. In the field of surgical procedures, particularly in liver surgery, in which the understanding of complex anatomy is crucial to improve perioperative outcomes, the implementation of AI as an operative aid is likely to become a hot topic in the future.
Various applications of AI to HB cancer patients
Table 1 summarizes several representative studies related to the application of AI to HB cancer patients.
Summary of representative studies using machine learning related to hepatobiliary cancers
Ref. | Year | Patient | Clinical application | Machine learning approach |
Famularo et al.[37] | 2022 | Hepatocellular carcinoma | Prediction of survival after recurrence | Least Absolute Shrinkage and Selection Operator |
Moazzam et al.[38] | 2024 | Hepatocellular carcinoma | Prediction of survival after recurrence | Optimal Survival Tree |
Iseke et al.[42] | 2023 | Hepatocellular carcinoma | Prediction of recurrence incorporating MRI data | Conventional neural network |
Saillard et al.[43] | 2020 | Hepatocellular carcinoma | Prediction of survival incorporating digitazed histological whole-slide data | Conventional neural network |
Jiang et al.[49] | 2021 | Hepatocellular carcinoma | Prediction of microvascular invasion | Radiomics and conventional neural network |
Alaimo et al.[51] | 2023 | Intrahepatic cholangiocarcinoma | Prediction of early recurrence | Random forest |
Cotter et al.[53] | 2022 | Gallbladder cancer | Prediction of overall survival | Classification and Regression Tree |
Tsilimigras et al.[54] | 2020 | Intrahepatic cholangiocarcinoma | Classification based on machine learning | Hierarchical machine-learning |
Chen et al.[55] | 2023 | Intrahepatic cholangiocarcinoma | Prediction of very early recurrence | Radiomics and K-means clustering |
Alaimo et al.[60] | 2023 | Intrahepatic cholangiocarcinoma | Prediction of survival and recurrence relative to margin width | Optimal policy tree |
Hepatocellular carcinoma
Treatment strategies for hepatocellular carcinoma (HCC) are diverse and usually based on factors such as tumor morphology, biology, patient performance status, and background liver condition[35]. The decision-making process becomes even more complex in the retreatment of patients with recurrent lesions post initial liver resection, as many different multidisciplinary approaches need to be considered[36]. To this end, some researchers have sought to establish optimal treatment ML algorithms to inform the care of these patients[37,38]. For example, Famularo et al. examined an Italian registry of patients with HCC and an external Japanese cohort to develop and validate a prediction model related to survival after recurrence (SAR)[37]. This model, based on a standard Cox model that incorporated all second-order interactions of treatment with features selected by the Least Absolute Shrinkage and Selection Operator (LASSO)[39], included treatment-related variables and time to recurrence. The resulting ML-based model demonstrated high predictive ability, as shown by the area under the curve (AUC) values (3-year SAR: AUC 0.805; 5-year SAR: AUC 0.785). The authors concluded that an ML-based model could assist in allocating treatment for patients with recurrent HCC[37]. Another study by our own research group utilized an international, multi-institutional database to formulate a prediction model for SAR. In this study, the SARScore was proposed as a prediction model based on clinicopathological determinants such as cirrhosis, number of primary tumors, macrovascular invasion, R1 resection margin, alpha-fetoprotein (AFP) > 400 ng/mL on diagnosis of recurrent disease, extrahepatic recurrence, radiologic size and number of recurrent lesions, radiologic recurrent bilobar disease, and recurrence within 24 months after hepatectomy. The clinical applicability of SARScore was assessed using Optimal Survival Tree (OST) analysis[12]. This ML-based tool demonstrated that patients with high SARScores experienced the worst survival outcomes (5-year AUC; training: 0.79 vs. testing: 0.71). In turn, the combination of SARScore and OST analysis can provide risk stratification and therapeutic guidance in the treatment of individuals with recurrent HCC.
AI has also been applied to develop prognostic risk scores following hepatectomy[40,41]. Traditionally, prognostic risk stratification has relied on “structured” or pre-specified data including patient characteristics, and tumor size/number. In recent years, AI has evolved to integrate structured information with more detailed yet previously untapped data. For example, Iseke et al. employed ML to predict recurrence using pretreatment laboratory, clinical, and magnetic resonance imaging (MRI) data among patients with early-stage HCC initially eligible for liver transplantation[42]. This study demonstrated that combining MRI radiomics with clinical parameters yielded the most accurate prediction of post-treatment recurrence. These data suggested that ML-based models can forecast recurrence before therapy allocation among patients eligible for liver transplantation with early-stage HCC. The incorporation of MRI data into the model significantly enhanced predictive performance compared with reliance on clinical parameters alone. Saillard et al. adopted a deep learning technique, utilizing whole-slide digitized histological slides (i.e., whole-slide imaging; WSI) to construct models to predict the survival of HCC patients undergoing surgical resection[43]. Notably, the derived scores demonstrated high C-indexes of 0.78 and 0.75, respectively, outperforming other models using structured clinicopathologic features. AI enabled the exploration of previously untapped data and the identification of distinctive features that may have been overlooked. One significant challenge, however, lies in the external application of imaging-based AI models to other cohorts, as the lack of a user-friendly interface hinders the broad adoption of this technique[44]. Addressing this challenge with the creation of easy-to-use, online applications will be crucial in the future.
Radiomics has emerged as a tool to diagnose, risk stratify, and predict prognosis among patients with HCC[45,46]. For instance, several studies have focused on developing preoperative models to predict microvascular invasion, an important prognostic factor that is traditionally identified only after surgery on pathological examination[47,48]. Jiang et al. demonstrated the effectiveness of a ML-based model combining radiomics with other clinicopathologic factors that resulted in high predictive accuracy[49]. In the future, radiomics will likely play a significant role in facilitating decision making regarding the treatment of HCC.
Biliary tract cancer
Bile duct cancer is a rare disease that often poses a diagnostic challenge[50]. To assist in tumor classification and postsurgical prognosis, several researchers have used ML-based approaches to the analysis of international multi-institutional datasets to improve the performance of prognostic tools. For instance, Alaimo et al. developed and validated three ML models aimed at predicting early recurrence (within
Recently, other research teams have examined the integration of radiomics data into prediction models to enhance discrimination ability. For instance, Chen et al. incorporated the 3D tumor region of interest (ROI) derived from contrast-enhanced CT to predict very early recurrence of intrahepatic cholangiocarcinoma. In this study, the K-means clustering algorithm was employed to identify novel radiomics-based subtypes of intrahepatic cholangiocarcinoma[55]. Notably, two distinct subtypes based on radiomics features were identified, with subtype 2 tumors demonstrating a higher proportion of very early recurrence (VER) (47.6%) versus subtype 1 lesions (25.5%).
Biliary tract cancer, particularly perihilar cholangiocarcinoma or central-type ICC, often necessitates extensive surgery[56,57]. While major liver resection with vascular resection may lead to a high incidence of morbidity and mortality, patients who require such radical resection might have limited survival benefits, raising questions about the effectiveness of the procedure[58]. To that end, the optimal allocation of treatment in these patients has been a topic of debate. AI has emerged as a possible tool to address this challenge. For example, Ratti et al. used an ML algorithm to identify patients most at risk for s “futile” outcomes after surgery for hilar perihilar cholangiocarcinoma defined as severe complications with early recurrence[59]. Of note, independent predictors of futility included an American Society of Anesthesiology (ASA) score ≥ 3, bilirubin at diagnosis ≥ 50 mmol/L, CA 19-9 ≥ 100 U/mL, preoperative cholangitis, portal vein involvement, tumor diameter ≥ 3 cm, and left-sided liver resection. The ML-based scoring system demonstrated good accuracy (AUC 0.755) in the validation cohort. The authors suggested that identifying patients at high risk of “futility” using this AI approach may help guide the consideration of alternative treatment options. In another study, Alaimo et al. utilized OPT analysis to define the optimal surgical margin width based on individual clinicopathologic factors[60]. The OPT categorized surgical patients into five groups based on age, tumor size, extent of hepatectomy, and CA19-9 levels. This personalized approach to determining margin width may assist in identifying patients who would benefit most from a wider negative resection margin. Moreover, using such a tool may help avoid unnecessarily wider margins, preventing radical surgery and subsequent surgical morbidity.
Operative aids for HB surgery
AI holds substantial promise in HB surgery, especially related to potential intraoperative support. Previous studies have highlighted the potential usefulness of AI in laparoscopic cholecystectomy[61,62]. For instance, Madani et al. demonstrated the efficacy of AI technology employing deep learning algorithms to identify safe and hazardous zones of dissection and other anatomical structures during laparoscopic cholecystectomy, improving the performance of operating surgeons[62]. Intraoperative AI can assist surgeons, particularly trainees, in decision making during surgery and help maintain quality control, as well as facilitate training efficiency.
Accurate assessment of the future liver remnant (FLR) volume is widely acknowledged to reduce the risk of post-hepatectomy liver failure[63]. To mitigate this complication, it is crucial to calculate the precise volume of FLR preoperatively and plan a surgical approach accordingly. Therefore, there is a need to improve conventional methods for this calculation (i.e., contrast-enhanced CT scan). Winkel et al. developed a CNN-based algorithm that demonstrated good accuracy, speed, and agreement with manual segmentation[64]. This approach could potentially improve the quality of 3D reconstruction of the liver, which may help more accurately estimate the FLR[65,66]. Incorporating techniques such as augmented reality (AR) and mixed reality allows for the synchronization of 3D-reconstructed images with real-time surgery, representing a safer and more reliable surgical navigation method. Notably, Ntourakis et al. reported in a pilot study that AR aided in detecting missing lesions post-chemotherapy for colorectal liver metastases, resulting in a higher likelihood of a margin-negative resection without any local recurrence[67]. The application of AR in robotic hepatectomy also has the potential to enhance a surgeon’s ability to achieve a safe tumor resection with an adequate margin[68].
Future perspectives and potential challenges
Looking ahead, the future of AI in healthcare holds promise, yet significant challenges remain. Addressing knowledge gaps surrounding data quality, data governance, interoperability, and algorithm transparency will be paramount. Researchers will need to focus on developing robust frameworks for data integration, standardization, and ethical AI deployment[69]. Additionally, efforts to enhance the interpretability and accountability of AI algorithms will be essential to foster trust among healthcare professionals and patients. The success of AI integration into the clinical setting will be related to external validation in multiple cohorts. In addition, the applicability of AI can be hindered by the dearth of an easy-to-use interface for AI-based models. Over the next five years, we anticipate continued progress in AI-driven diagnostics, personalized medicine, and surgical innovations. However, realizing the full potential of AI in healthcare will require collaborative efforts across academia, industry, and regulatory bodies to ensure responsible and equitable implementation while maximizing patient outcomes.
CONCLUSIONS
Recent advancements in AI offer the chance to enhance the care of patients, as demonstrated in the current study that highlighted the integration of AI into the care of patients with HB tumors. Specifically, AI models have the potential to impact patient stratification and decision making and are poised to become integral components of future surgical research and care. As these technologies continue to evolve, their application could revolutionize medical practices, introducing an era of more precise diagnostics, personalized treatment plans, and innovative approaches to surgical advancements. The fusion of AI and healthcare holds immense promise to optimize patient outcomes, as well as drive transformative breakthroughs in the field.
DECLARATIONS
Authors’ contributions
Conceived the idea: Endo Y, Alaimo L, Pawlik TM
Wrote the manuscript: Endo Y, Alaimo L
Reviewed the manuscript: Catalano G, Chatzipanagiotou OP, Pawlik TM
Availability of data and materials
The data that support the findings of this study are available from the corresponding author upon reasonable request.
Financial support and sponsorship
None.
Conflicts of interest
All authors declared that there are no conflicts of interest.
Ethical approval and consent to participate
Not applicable.
Consent for publication
Not applicable.
Copyright
© The Author(s) 2024.
REFERENCES
1. McCarthy J. What is artificial intelligence? 2004. Available from: https://cse.unl.edu/~choueiry/S09-476-876/Documents/whatisai.pdf. [Last accessed on 24 May 2024].
3. Jha S, Topol EJ. Adapting to artificial intelligence: radiologists and pathologists as information specialists. JAMA 2016;316:2353-4.
4. Placona AM, Martinez C, McGehee H, Carrico B, Klassen DK, Stewart D. Can donor narratives yield insights? A natural language processing proof of concept to facilitate kidney allocation. Am J Transplant 2020;20:1095-104.
5. Kelly CJ, Karthikesalingam A, Suleyman M, Corrado G, King D. Key challenges for delivering clinical impact with artificial intelligence. BMC Med 2019;17:195.
6. Hua KL, Hsu CH, Hidayati SC, Cheng WH, Chen YJ. Computer-aided classification of lung nodules on computed tomography images via deep learning technique. Onco Targets Ther 2015;8:2015-22.
7. Jiang Z, Gandomkar Z, Trieu PDY, et al. Evaluating recalibrating AI models for breast cancer diagnosis in a new context: insights from transfer learning, image enhancement and high-quality training data integration. Cancers 2024;16:322.
8. Escobar GJ, Turk BJ, Ragins A, et al. Piloting electronic medical record-based early detection of inpatient deterioration in community hospitals. J Hosp Med 2016;11:S18-24.
9. Rajkomar A, Oren E, Chen K, et al. Scalable and accurate deep learning with electronic health records. NPJ Digit Med 2018;1:18.
10. Xu J, Yang P, Xue S, et al. Translating cancer genomics into precision medicine with artificial intelligence: applications, challenges and future perspectives. Hum Genet 2019;138:109-24.
11. Foster JC, Taylor JM, Ruberg SJ. Subgroup identification from randomized clinical trial data. Stat Med 2011;30:2867-80.
12. Interpretable AI. Optimal decision trees. Available from: https://www.interpretable.ai/products/optimal-trees/. [Last accessed on 24 May 2024].
13. Endo Y, Alaimo L, Moazzam Z, et al. Postoperative morbidity after simultaneous versus staged resection of synchronous colorectal liver metastases: impact of hepatic tumor burden. Surgery 2024;175:432-40.
14. Endo Y, Alaimo L, Moazzam Z, et al. Optimal policy tree to assist in adjuvant therapy decision-making after resection of colorectal liver metastases. Surgery 2024;175:645-53.
15. Paul D, Sanap G, Shenoy S, Kalyane D, Kalia K, Tekade RK. Artificial intelligence in drug discovery and development. Drug Discov Today 2021;26:80-93.
16. Sageshima J, Than P, Goussous N, Mineyev N, Perez R. Prediction of high-risk donors for kidney discard and nonrecovery using structured donor characteristics and unstructured donor narratives. JAMA Surg 2024;159:60-8.
17. Wahl B, Cossy-Gantner A, Germann S, Schwalbe NR. Artificial intelligence (AI) and global health: how can AI contribute to health in resource-poor settings? BMJ Glob Health 2018;3:e000798.
18. Mollica V, Rizzo A, Marchetti A, et al. The impact of ECOG performance status on efficacy of immunotherapy and immune-based combinations in cancer patients: the MOUSEION-06 study. Clin Exp Med 2023;23:5039-49.
19. Gong X, Hu M, Zhao L. Big data toolsets to pharmacometrics: application of machine learning for time-to-event analysis. Clin Transl Sci 2018;11:305-11.
20. Barber EL, Garg R, Persenaire C, Simon M. Natural language processing with machine learning to predict outcomes after ovarian cancer surgery. Gynecol Oncol 2021;160:182-6.
21. Resende V, Tsilimigras DI, Endo Y, et al. Machine-based learning hierarchical cluster analysis: sex-based differences in prognosis following resection of hepatocellular carcinoma. World J Surg 2023;47:3319-27.
22. Boulesteix AL, Janitza S, Kruppa J, König IR. Overview of random forest methodology and practical guidance with emphasis on computational biology and bioinformatics. WIREs Data Mining Knowl Discov 2012;2:493-507.
23. Friedman JH. Greedy function approximation: a gradient boosting machine. Ann Statist 2001;29:1189-232.
24. Lai Q, Spoletini G, Mennini G, et al. Prognostic role of artificial intelligence among patients with hepatocellular cancer: a systematic review. World J Gastroenterol 2020;26:6679-88.
25. Cover T, Hart P. Nearest neighbor pattern classification. IEEE Trans Inform Theory 1967;13:21-7.
26. Patel H, Zanos T, Hewitt DB. Deep learning applications in pancreatic cancer. Cancers 2024;16:436.
27. Wakabayashi T, Ouhmich F, Gonzalez-Cabrera C, et al. Radiomics in hepatocellular carcinoma: a quantitative review. Hepatol Int 2019;13:546-59.
28. Endo Y, Sasaki K, Moazzam Z, et al. Quality of ChatGPT responses to questions related to liver transplantation. J Gastrointest Surg 2023;27:1716-9.
29. Rajkomar A, Kannan A, Chen K, et al. Automatically charting symptoms from patient-physician conversations using machine learning. JAMA Intern Med 2019;179:836-8.
30. Moazzam Z, Lima HA, Endo Y, Noria S, Needleman B, Pawlik TM. A Paradigm shift: online artificial intelligence platforms as an informational resource in bariatric surgery. Obes Surg 2023;33:2611-4.
31. Ali SR, Dobbs TD, Tarafdar A, et al. Natural language processing to automate a web-based model of care and modernize skin cancer multidisciplinary team meetings. Br J Surg 2024;111:znad347.
32. Bcharah G, Gupta N, Panico N, et al. Innovations in spine surgery: a narrative review of current integrative technologies. World Neurosurg 2024;184:127-36.
33. Choksi S, Szot S, Zang C, et al. Bringing Artificial Intelligence to the operating room: edge computing for real-time surgical phase recognition. Surg Endosc 2023;37:8778-84.
34. Takeuchi M, Kawakubo H, Saito K, et al. Automated surgical-phase recognition for robot-assisted minimally invasive esophagectomy using artificial intelligence. Ann Surg Oncol 2022;29:6847-55.
35. Reig M, Forner A, Rimola J, et al. BCLC strategy for prognosis prediction and treatment recommendation: the 2022 update. J Hepatol 2022;76:681-93.
36. Matsumoto M, Yanaga K, Shiba H, et al. Treatment of intrahepatic recurrence after hepatectomy for hepatocellular carcinoma. Ann Gastroenterol Surg 2021;5:538-52.
37. Famularo S, Donadon M, Cipriani F, et al; HE.RC.O.LE.S. Group. Machine learning predictive model to guide treatment allocation for recurrent hepatocellular carcinoma after surgery. JAMA Surg 2023;158:192-202.
38. Moazzam Z, Alaimo L, Endo Y, et al. A prognostic model to predict survival after recurrence among patients with recurrent hepatocellular carcinoma. Ann Surg 2024;279:471-8.
39. Tibshirani R. Regression shrinkage and selection via the lasso. J R Stat Soc Ser B Stat Method 1996;58:267-88.
40. Wang K, Xiang Y, Yan J, et al. A deep learning model with incorporation of microvascular invasion area as a factor in predicting prognosis of hepatocellular carcinoma after R0 hepatectomy. Hepatol Int 2022;16:1188-98.
41. Ji GW, Fan Y, Sun DW, et al. Machine learning to improve prognosis prediction of early hepatocellular carcinoma after surgical resection. J Hepatocell Carcinoma 2021;8:913-23.
42. Iseke S, Zeevi T, Kucukkaya AS, et al. Machine learning models for prediction of posttreatment recurrence in early-stage hepatocellular carcinoma using pretreatment clinical and MRI features: a proof-of-concept study. AJR Am J Roentgenol 2023;220:245-55.
43. Saillard C, Schmauch B, Laifa O, et al. Predicting survival after hepatocellular carcinoma resection using deep learning on histological slides. Hepatology 2020;72:2000-13.
44. Endo Y, Alaimo L, Lima HA, et al. A novel online calculator to predict risk of microvascular invasion in the preoperative setting for hepatocellular carcinoma patients undergoing curative-intent surgery. Ann Surg Oncol 2023;30:725-33.
45. Yao S, Ye Z, Wei Y, Jiang HY, Song B. Radiomics in hepatocellular carcinoma: a state-of-the-art review. World J Gastrointest Oncol 2021;13:1599-615.
46. Lewis S, Hectors S, Taouli B. Radiomics of hepatocellular carcinoma. Abdom Radiol 2021;46:111-23.
47. Ma X, Wei J, Gu D, et al. Preoperative radiomics nomogram for microvascular invasion prediction in hepatocellular carcinoma using contrast-enhanced CT. Eur Radiol 2019;29:3595-605.
48. Zhu YJ, Feng B, Wang S, et al. Model-based three-dimensional texture analysis of contrast-enhanced magnetic resonance imaging as a potential tool for preoperative prediction of microvascular invasion in hepatocellular carcinoma. Oncol Lett 2019;18:720-32.
49. Jiang YQ, Cao SE, Cao S, et al. Preoperative identification of microvascular invasion in hepatocellular carcinoma by XGBoost and deep learning. J Cancer Res Clin Oncol 2021;147:821-33.
50. American Cancer Society. Key statistics for bile duct cancer. Available from: https://www.cancer.org/cancer/types//bile-duct-cancer/about/key-statistics.html. [Last accessed on 24 May 2024].
51. Alaimo L, Lima HA, Moazzam Z, et al. Development and validation of a machine-learning model to predict early recurrence of intrahepatic cholangiocarcinoma. Ann Surg Oncol 2023;30:5406-15.
52. Sasaki K, Morioka D, Conci S, et al. The tumor burden score: a new “metro-ticket” prognostic tool for colorectal liver metastases based on tumor size and number of tumors. Ann Surg 2018;267:132-41.
53. Cotter G, Beal EW, Poultsides GA, et al. Using machine learning to preoperatively stratify prognosis among patients with gallbladder cancer: a multi-institutional analysis. HPB 2022;24:1980-8.
54. Tsilimigras DI, Hyer JM, Paredes AZ, et al. A novel classification of intrahepatic cholangiocarcinoma phenotypes using machine learning techniques: an international multi-institutional analysis. Ann Surg Oncol 2020;27:5224-32.
55. Chen B, Mao Y, Li J, et al. Predicting very early recurrence in intrahepatic cholangiocarcinoma after curative hepatectomy using machine learning radiomics based on CECT: a multi-institutional study. Comput Biol Med 2023;167:107612.
56. Endo Y, Moazzam Z, Lima HA, et al. The impact of tumor location on the value of lymphadenectomy for intrahepatic cholangiocarcinoma. HPB 2023;25:650-8.
57. Mueller M, Breuer E, Mizuno T, et al. Perihilar cholangiocarcinoma - novel benchmark values for surgical and oncological outcomes from 24 expert centers. Ann Surg 2021;274:780-8.
58. van Keulen AM, Buettner S, Erdmann JI, et al; perihilar cholangiocarcinoma collaboration group. Multivariable prediction model for both 90-day mortality and long-term survival for individual patients with perihilar cholangiocarcinoma: does the predicted survival justify the surgical risk? Br J Surg 2023;110:599-605.
59. Ratti F, Marino R, Olthof PB, et al; Perihilar Cholangiocarcinoma Collaboration Group. Predicting futility of upfront surgery in perihilar cholangiocarcinoma: Machine learning analytics model to optimize treatment allocation. Hepatology 2024;79:341-54.
60. Alaimo L, Moazzam Z, Endo Y, et al. The application of artificial intelligence to investigate long-term outcomes and assess optimal margin width in hepatectomy for intrahepatic cholangiocarcinoma. Ann Surg Oncol 2023;30:4292-301.
61. Laplante S, Namazi B, Kiani P, et al. Validation of an artificial intelligence platform for the guidance of safe laparoscopic cholecystectomy. Surg Endosc 2023;37:2260-8.
62. Madani A, Namazi B, Altieri MS, et al. Artificial intelligence for intraoperative guidance: using semantic segmentation to identify surgical anatomy during laparoscopic cholecystectomy. Ann Surg 2022;276:363-9.
63. Alaimo L, Endo Y, Lima HA, et al. A comprehensive preoperative predictive score for post-hepatectomy liver failure after hepatocellular carcinoma resection based on patient comorbidities, tumor burden, and liver function: the CTF score. J Gastrointest Surg 2022;26:2486-95.
64. Winkel DJ, Weikert TJ, Breit HC, et al. Validation of a fully automated liver segmentation algorithm using multi-scale deep reinforcement learning and comparison versus manual segmentation. Eur J Radiol 2020;126:108918.
65. Ruzzenente A, Alaimo L, D’Onofrio M, et al. Perihilar cholangiocarcinoma: three-dimensional modelling algorithm to estimate tumour extension and bile duct resection margins. Br J Surg 2024;111:znad428.
66. Tomiyama K, Ghazi A, Hernandez-Alejandro R. Looking beyond the horizon: patient-specific rehearsals for complex liver surgeries with 3D printed model. Ann Surg 2021;273:e28-30.
67. Ntourakis D, Memeo R, Soler L, Marescaux J, Mutter D, Pessaux P. Augmented reality guidance for the resection of missing colorectal liver metastases: an initial experience. World J Surg 2016;40:419-26.
68. Pessaux P, Diana M, Soler L, Piardi T, Mutter D, Marescaux J. Towards cybernetic surgery: robotic and augmented reality-assisted liver segmentectomy. Langenbecks Arch Surg 2015;400:381-5.
Cite This Article
Export citation file: BibTeX | EndNote | RIS
OAE Style
Endo Y, Alaimo L, Catalano G, Chatzipanagiotou OP, Pawlik TM. Application of artificial intelligence to hepatobiliary cancer clinical outcomes research. Art Int Surg 2024;4:59-67. http://dx.doi.org/10.20517/ais.2024.09
AMA Style
Endo Y, Alaimo L, Catalano G, Chatzipanagiotou OP, Pawlik TM. Application of artificial intelligence to hepatobiliary cancer clinical outcomes research. Artificial Intelligence Surgery. 2024; 4(2): 59-67. http://dx.doi.org/10.20517/ais.2024.09
Chicago/Turabian Style
Yutaka Endo, Laura Alaimo, Giovanni Catalano, Odysseas P. Chatzipanagiotou, Timothy M. Pawlik. 2024. "Application of artificial intelligence to hepatobiliary cancer clinical outcomes research" Artificial Intelligence Surgery. 4, no.2: 59-67. http://dx.doi.org/10.20517/ais.2024.09
ACS Style
Endo, Y.; Alaimo L.; Catalano G.; Chatzipanagiotou OP.; Pawlik TM. Application of artificial intelligence to hepatobiliary cancer clinical outcomes research. Art. Int. Surg. 2024, 4, 59-67. http://dx.doi.org/10.20517/ais.2024.09
About This Article
Special Issue
Copyright
Data & Comments
Data
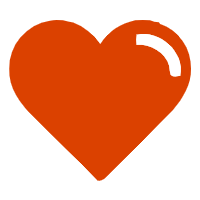
Comments
Comments must be written in English. Spam, offensive content, impersonation, and private information will not be permitted. If any comment is reported and identified as inappropriate content by OAE staff, the comment will be removed without notice. If you have any queries or need any help, please contact us at support@oaepublish.com.