Meeting abstracts of the 1st Surgical AI Conference
1. Artificial Intelligence-Assisted Analysis of tumor-targeted robotic surgery using molecular guidance
Samaneh Azargoshasb1,2, Hilda A de Barros2, Daphne D. D. Rietbergen1,3, Paolo Dell’Oglio1,4, Pim J van Leeuwen2, Christian Wagner5, Phillip Stricker6,7, Sergi Vidal-Sicart8,9, Alberto Briganti10, Tobias Maurer11,12, Henk G. van der Poel2,13, Matthias N. van Oosterom1,2, Fijs W. B. van Leeuwen1,2
1Interventional Molecular Imaging Laboratory, Department of Radiology, Leiden University Medical Center, Leiden 2333 ZA, the Netherlands.
2Department of Urology, Netherlands Cancer Institute-Antoni van Leeuwenhoek Hospital, Amsterdam 1066 CX, the Netherlands.
3Section of Nuclear Medicine, Department of Radiology, Leiden University Medical Center Leiden 2333 ZA, the Netherlands.
4Department of Urology, ASST Grande Ospedale Metropolitano Niguarda, Milan 20162, Italy.
5St. Antonius - Hospital Gronau, Head of Robotic Urology, Gronau 48599, Germany.
6St. Vincent’s Prostate Cancer Research Centre, Darlinghurst NSW 2010, Australia.
7Department of Urology, St Vincents Hospital and Campus, Darlinghurst NSW 2010, Australia.
8Nuclear Medicine Department, Hospital Clinic Barcelona, Barcelona 08036, Spain.
9Institut d’Investigació Biomèdica August Pi I Sunyer (IDIBAPS), Barcelona 08036, Spain.
10Department of Urology, University Vita-Salute, San Raffaele Scientific Institute, Via Olgettina, 58, Milan 20132, Italy.
11Martini-Klinik Prostate Cancer Center, University Hospital Hamburg-Eppendorf, Martinistraße 52, Hamburg 20251, Germany.
12Department of Urology, University Hospital Hamburg-Eppendorf, Martinistraße 52, Hamburg 20251, Germany.
13Department of Urology, Amsterdam University Medical Centers, De Boelelaan 1117, Amsterdam 1081 HV, the Netherlands.
Correspondence to: Dr. Fijs W. B. van Leeuwen, Interventional Molecular Imaging Laboratory, Department of Radiology, Leiden University Medical Center, Albinusdreef 2, Leiden 2333 ZA, the Netherlands. E-mail: f.w.b.van_leeuwen@lumc.nl
Abstract
Aim: The DROP-IN gamma probe enables robotic radioguided surgery using radioactive tracers for molecular guidance. In prostate cancer, it supports surgical guidance towards sentinel lymph nodes (SLN) and prostate-specific membrane antigen (PSMA)-avid lesions. Despite both procedures using 99mTc isotopes and DROP-IN gamma probe, PSMA resections are more challenging due to tracer pharmacokinetics differences. To study the impact of different levels of image guidance on surgical decision-making, deep learning algorithms were used to analyze surgical performance using DROP-IN probe kinematics.
Methods: 44 prostate cancer patients underwent robot-assisted procedures (25 SLN and 19 PSMA-targeted). SPECT/CT and PSMA-PET/CT were used as preoperative roadmaps, and intraoperative probe readouts were recorded. A frame-by-frame detection method using a ResNet algorithm was employed to track the DROP-IN probe tip in surgical videos (2,200 frames training, 577 frames test, and 100 frames evaluation set). The multiparametric kinematics extracted from probe trajectories were used to generate decision-making scores.
Results: PSMA-targeted resections show significantly lower nodal signal intensities in preoperative SPECT-CT scans (three-fold; P = 0.01), intraoperative probe readouts (eight-fold; P < 0.001), and signal-to-background ratios (SBR; two-fold; P < 0.001). Our custom AI tracking algorithm proved accurate enough for kinematic assessment, revealing that challenges encountered during PSMA-targeted procedures result in longer target identification times and increased probe pick-ups (both five-fold; P < 0.001), leading to a four-fold reduction in decision-making score (P < 0.001).
Conclusion: AI-based DROP-IN probe tracking enabled objective and quantitative kinematic assessment during two image-guided procedures. While the DROP-IN probe facilitates both procedures, the PSMA-targeted approach, with lower signal intensities and higher background, resulted in reduced surgical performance.
Keywords: Image-guided surgery, artificial intelligence, robot-assisted surgery, surgical instrument tracking, surgical performance evaluation
2. Enhancing surgery in endometriosis laparoscopy: training neural networks to segment incision boundaries
Saman Noorzadeh1, Giuseppe Giacomello2, Filippo A. Ferrari3, Jean-Luc Pouly4, Julien Peyras1, Adrien Bartoli1,5, Julie Desternes1, Antoine Netter6, Fanny Duchateau6, Henrique Abrão7, Mauricio S. Abrão7, Attila Bokor8, Michel Canis4, Nicolas Bourdel1,4
1SURGAR Surgical Augmented Reality, Clermont-Ferrand 63000, France.
2Department of Clinical and Experimental Sciences, University of Brescia, Brescia 25121, Italy.
3Division of Obstetrics and Gynecology, Azienda Ospedaliera Universitaria Integrata, Verona 37126, Italy.
4Department of Obstetrics Gynecology and Reproductive Medicine, CHU Clermont-Ferrand, Clermont-Ferrand 63000, France.
5Department of Clinical Research and Innovation, CHU Clermont-Ferrand, Clermont-Ferrand 63000, France.
6Department of Obstetrics and Gynecology, Marseille hospital, Marseille 13006, France.
7Gynecologic Division, Beneficência Portuguesa de São Paulo, São Paulo 01323-001, Brazil.
8Department of Obstetrics and Gynecology, Semmelweis University, Budapest 1088, Hungary.
Correspondence to: Dr. Saman Noorzadeh, SURGAR Surgical Augmented Reality, 22 allée Alan Turing, Clermont-Ferrand 63000, France. E-mail: saman.noorzadeh@surgar-surgery.com
Abstract
Aim: This study addresses inter-surgeon variability and the lack of standardized surgical procedures during laparoscopic surgery for endometriosis. We propose a neural network for automated incision boundary segmentation.
Methods: The dataset includes 210 laparoscopic surgeries from five centers worldwide, adhering to legal regulations. We created a guidebook for data annotations. Two junior and two senior surgeons annotated ~8 K zones across 1,150 images. Our annotation process involved over 55 person-hours of discussions to achieve consensus on ontology and on defining reference segmentations. We used DeepLabV3 and trained four neural networks on data annotated by experts of varying proficiency. We use Intersection-over-Union (IoU) as an evaluation metric.
Results: Firstly, the best- and worst-performing surgeons achieved 42% and 48% IoU with the reference segmentations, with 0.24 and 0.26 standard deviation (std), respectively. In contrast, the best neural network achieved a mean-IoU of 36% (0.26 std). However, all experts visually assessed these results to be on par with theirs. Additionally, the neural network’s specificity consistently exceeded 97%, ensuring a low number of false signals. Secondly, our consensus-based annotation process significantly improved (P < 0.05) the initial inter-surgeon agreement observed across all annotator pairs, except one.
Conclusion: Artificial Intelligence is promising at assisting endometriotic surgery. We plan to expand our dataset to improve performance, design a clinically meaningful evaluation metric to replace the unadapted IoU and conduct a clinical impact study to measure concrete applicability.
3. Artificial intelligence 3D augmented reality guided RARP vs. cognitive MRI intervention: preliminary analysis of RIDERS trial
Daniele Amparore1, Enrico Checcucci2, Michele Sica1, Sabrina De Cillis1, Gabriele Volpi2, Paolo Alessio2, Federico Piramide1, Alberto Piana3, Edoardo Cisero1, Michele Ortenzi1, Stefano De Luca1, Pasquale Rescigno2, Matteo Manfredi1, Ilaria Stura4, Giuseppe Migliaretti4, Pietro Piazzolla2, Cristian Fiori1, Francesco Porpiglia1
1Department of Oncology, Division of Urology, University of Turin, San Luigi Gonzaga Hospital, Orbassano 10043, Italy.
2Department of Surgery, Candiolo Cancer Institute, FPO-IRCCS, Candiolo 10060, Italy.
3Romolo Hospital, Rocca di Neto 88821, Italy.
4Department of Public Health and Pediatric Sciences, School of Medicine, University of Turin, Turin 10126, Italy.
Correspondence to: Dr. Daniele Amparore, Department of Oncology, Division of Urology, University of Turin, San Luigi Gonzaga Hospital, Regione Gonzole 10, Orbassano 10043, Italy. E-mail: danieleamparore@hotmail.it
Abstract
Aim: The aim of this study was to compare oncological and functional outcomes of 3D Artificial Intelligence (AI) Augmented Reality (AR) RARP vs. 2D RARP.
Methods: From June 2022, candidates with suspicious extracapsular extension at preoperative mp-MRI were enrolled in the study and randomized with a ratio of 1:3 in 3D and no-3D Groups. At the end of the extirpative phase with a nerve-sparing (NS) approach, a selective excisional biopsy on the neurovascular bundle (NVB) was performed (thanks to overlap with AI AR in the 3D group while in a cognitive manner in the 2D group). Biopsy findings, perioperative, oncological, and functional outcomes of the two groups were analyzed and compared. Positive surgical margins (PSM) were first evaluated and completed with biopsy assessment.
Results: 30 and 48 patients were enrolled in 3D and no-3D Groups. No differences were found in terms of perioperative variables. PSM were 53.3% (16/30) and 54.1% (26/48) (P = 0.45), respectively. Selective excisional biopsies were positive in 43.75% (7/16) and 11.53% (3/26) of the cases in 3D and no-3D Groups
Conclusions: 3D AI AR imaging assistance enables the accurate identification of tumors at the level of the NVBs, permitting the execution of a NS procedure.
4. Pixel tracks and pseudo-depth maps from monocular laparoscopic video clips using implicit neural representations
Beerend Gerats1,2, Seb Mol1,3, Jelmer M. Wolterink3,4, Ivo Broeders1,2
1AI & Data Science Center, Meander Medical Center, Amersfoort 3813 TZ, the Netherlands.
2Robotics and Mechatronics, University of Twente, Enschede 7522 NB, the Netherlands.
3Technical Medical Center, University of Twente, Enschede 7522 NB, the Netherlands.
4Department of Applied Mathematics, University of Twente, Enschede 7522 NB, the Netherlands.
Correspondence to: Beerend Gerats, AI & Data Science Center, Meander Medical Center, Maatweg 3, Amersfoort 3813 TZ, the Netherlands. E-mail: bga.gerats@meandermc.nl
Abstract
Aim: The reconstruction of surgical scenes from laparoscopic video has many potential applications, from education to intra-operative context awareness. Recently, the use of implicit neural representations (INRs) was proposed for the reconstruction of surgical scenes. However, pseudo-depth maps from stereoscopic imaging are required, limiting the application of these methods to robotic surgery. In this research, we propose the application of an INR-based method to monocular video clips for the reconstruction of surgical scenes and the generation of pseudo-depth maps.
Methods: We use “OmniMotion”, a novel method for tracking pixels through a video clip by reconstruction of a 3D virtual scene with INRs. We evaluate its applicability on monocular laparoscopic videos by evaluating pixel tracking accuracy and pseudo-depth map correctness. For our experiments, we use video clips from the SCARED dataset that was specifically designed for depth estimation in laparoscopic video.
Results: We find that OmniMotion can provide accurate pixel tracks through short monocular laparoscopic video clips. The method performs particularly well on anatomy-related pixels, while its performance on tool-related pixels is fragile. In video clips that provide a clear view of the abdomen and involve camera movement, the method is able to generate useful pseudo-depth maps.
Conclusion: Our results show the potential for INR-based methods on monocular laparoscopic video clips for the reconstruction of surgical scenes, pixel tracking, and the generation of pseudo-depth maps. Further development of the method is necessary to make its application computationally efficient and reliable for 3D tracking of surgical tools.
Keywords: Pixel tracks, pseudo-depth maps, surgical scene reconstruction, monocular laparoscopic videos, implicit neural representations, OmniMotion
5. Sequence-based imitation learning for robot-assisted surgical operations
Gabriele Furnari, Cristian Secchi, Federica Ferraguti
Department of Sciences and Methods for Engineering, University of Modena and Reggio Emilia, Reggio Emilia 42122, Italy.
Correspondence to: Gabriele Furnari, Department of Sciences and Methods for Engineering, University of Modena and Reggio Emilia, Reggio Emilia 42122, Italy. E-mail: gabriele.furnari@unimore.it
Abstract
Aim: The proposed study aims to advance research in the field of autonomous surgical operations through imitation learning from video demonstrations and enhance the performance obtained through state-of-the-art approaches. The evaluation is executed by comparing the results on the JIGSAW dataset.
Methods: To address this objective, we exploited an encoder-decoder structure with a ResNet18 Convolutional Neural Network (CNN) and a Long Short-Term Memory (LSTM)-based Recurrent Neural Network (RNN), handling surgical motion sequences with a sliding window mechanism. A self-generating sequence approach exploits each predicted pose iteratively as input for the next prediction. This model processes video frames and prior poses to predict the poses for the robotic arms. This methodology effectively modeled the sequential nature of surgical operations. In terms of parameters, a learning rate of 0.5 × 10-6, RMSProp optimizer, MAE loss function, and 50 epochs were used. The hidden layer size (Hsize) was set to 100, balancing model complexity and efficiency.
Results: The model achieved promising results, exhibiting an average loss of 0.18 cm per position, significantly surpassing the state-of-the-art Motion2Vec model’s performance (0.94 cm average loss). This highlights the sequence-based approach’s efficacy in capturing and predicting surgical trajectories with higher precision.
Conclusion: The proposed study supports imitation learning’s viability for acquiring complex task execution policies in surgical robotics. The sequence-based model, combining CNN and RNN architectures, successfully handles intricate surgical trajectories, obtaining an average loss of 0.18 cm. This work emphasizes imitation learning’s potential in enhancing the precision of robot surgical procedures and advocates for the adoption of sequence-based models in trajectory prediction for robotic systems.
Keywords: Autonomous surgical robot, imitation learning, sequence-based
6. The role of contextual chronological cues in phase classification accuracy in laparoscopic cholecystectomy
André Pita1, Pedro Bargão1, Paulo Mira2, AS Soares2
1Department of Urology, Hospital Professor Doutor Fernando da Fonseca, Amadora 2720-276, Portugal.
2Department of General Surgery, Hospital Professor Doutor Fernando da Fonseca, Amadora 2720-276, Portugal.
Correspondence to: Dr. André Pita, Department of Urology, Hospital Professor Doutor Fernando da Fonseca, Hospital IC19 276, Amadora 2720-276, Portugal. E-mail: andre.pita@hff.min-saude.pt
Abstract
Aim: To better design computer vision algorithms to classify surgical phases accurately, it is necessary to define the role of contextual data in accurate phase identification. We aimed to define the role of chronological order in the phase classification accuracy in laparoscopic cholecystectomy procedures.
Methods: A survey was created to present two sets of images to every rater: a set containing 7 chronologically ordered images of phases and a set containing 7 randomized ones from a total of 80 procedures. Data and ground truth were retrieved from the Cholec80 dataset. Each participant had a randomized allocation of one ordered and one random set of images to classify. Raters were surgeons with varying levels of expertise in performing laparoscopic cholecystectomy.
Results: 30 raters (10 consultants, 20 trainees) from a convenience sample rated 60 sets of images. Our statistical analysis, conducted using IBM SPSS Statistics, involved a paired samples t-test to compare the accuracy rates between the ordered and randomized image sets. No significant difference was found when comparing average accuracy in the ordered versus randomized sets (97.1% vs. 96.4% respectively, P > 0.05) [Table 1]. No significant difference was found when specific phases were analyzed [Table 2].
Conclusion: According to our data, surgeons relying only on visual cues achieved a near-perfect accuracy in phase identification, independent of seeing the phases in chronological order. Therefore, we hypothesize that computer vision algorithms based on visual cues with minimal contextual information can achieve similar phase classification accuracy in laparoscopic cholecystectomy.
Paired samples t-test between the ordered and randomized sets
Paired samples test | ||||||||||
Paired differences | t | df | Significance | |||||||
Mean | Std. deviation | Std. error Mean | 95% Confidence interval of the difference | One-sided p | Two-sided p | |||||
Lower | Upper | |||||||||
Pair 1 | q_correct_ordered q_correct_random | .00476 | .05912 | .01079 | -.01731 | .02684 | .441 | 29 | .331 | .662 |
Paired samples t-test between specific phases
Paired samples test | ||||||||||
Paired differences | t | df | Significance | |||||||
Mean | Std. deviation | Std. error mean | 95% Confidence interval of the difference | One-sided p | Two-sided p | |||||
Lower | Upper | |||||||||
Pair 1 | F1O - F1R | .00000 | .37139 | .06781 | -.13868 | .13868 | .000 | 29 | .500 | 1.000 |
Pair 2 | F2O - F2R | -.03333 | .31984 | .05839 | -.15276 | .08610 | -.571 | 29 | .286 | .573 |
Pair 3 | F3O - F3R | -.03333 | .18257 | .03333 | -.10151 | .03484 | -1.000 | 29 | .163 | .326 |
Pair 4 | F4O - F4R | .03333 | .18257 | .03333 | -.03484 | .10151 | 1.000 | 29 | .163 | .326 |
Pair 6 | F6O - F6R | .00000 | .26261 | .04795 | -.09806 | .09806 | .000 | 29 | .500 | 1.000 |
Pair 7 | F7O - F7R | .06667 | .25371 | .04632 | -.02807 | .16140 | 1.439 | 29 | .080 | .161 |
Keywords: Contextual classification, surgical phase, laparoscopic cholecystectomy
Cite This Article
Export citation file: BibTeX | RIS
OAE Style
Orsi Academy. Meeting abstracts of the 1st Surgical AI Conference. Art Int Surg 2024;4:1-6. http://dx.doi.org/10.20517/ais.2024.02
AMA Style
Orsi Academy. Meeting abstracts of the 1st Surgical AI Conference. Artificial Intelligence Surgery. 2024; 4(1): 1-6. http://dx.doi.org/10.20517/ais.2024.02
Chicago/Turabian Style
Orsi Academy, . 2024. "Meeting abstracts of the 1st Surgical AI Conference" Artificial Intelligence Surgery. 4, no.1: 1-6. http://dx.doi.org/10.20517/ais.2024.02
ACS Style
Orsi, Academy. Meeting abstracts of the 1st Surgical AI Conference. Art. Int. Surg. 2024, 4, 1-6. http://dx.doi.org/10.20517/ais.2024.02
About This Article
Special Issue
Copyright
Data & Comments
Data
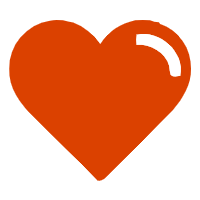

Comments
Comments must be written in English. Spam, offensive content, impersonation, and private information will not be permitted. If any comment is reported and identified as inappropriate content by OAE staff, the comment will be removed without notice. If you have any queries or need any help, please contact us at support@oaepublish.com.