Applications of artificial intelligence in benign prostatic hyperplasia
Abstract
The advancement of computational abilities has taken us from the days of machines performing simple, one-dimensional tasks to themselves learning and applying knowns to unknowns. Artificial intelligence (AI) has become integral in daily life, yet there is vast room for application in surgery. Cancer research can divert attention from more prevalent benign diseases which may equally cause a significant impact on quality of life. Here we review recent advancements in the field of AI for diagnostics, management, and prognostication of benign prostatic hyperplasia, evaluating the strengths and limitations of these approaches with implications for future research.
Keywords
INTRODUCTION
Artificial intelligence (AI) is a broad term that describes the ability of a computer or machine to perform tasks that would normally require human intelligence. One area of AI which has garnered significant recent interest is machine learning (ML). It involves the incorporation of algorithms into a model, and then training the model on existing data sets to allow learning and prediction of outcomes from unseen data sets. Deep learning (DL) is a subset of ML that encompasses several layers of processing [e.g., convolutional neural networks (CNNs)] to create predictive and analytical models [Figure 1], which often produce superior results in practice.
Figure 1. An overview of AI, ML and DL (image adapted with color changes from[1]).
Examples of AI in surgery include image analysis for diagnostics, optimizing management techniques, and prognostication[2,3]. However, while recent advances have been made in urological oncology, applications of AI in benign conditions have been underexplored[3].
While prostate cancer (PCa) is the second most commonly diagnosed cancer in men worldwide, the incidence of benign prostatic hyperplasia (BPH) far exceeds this, with a prevalence of 50% and 80% in men by the 6th and 9th decade of life, respectively[4,5]. BPH and its treatment are associated with urinary dysfunction and retention (features of bladder outlet obstruction), increased risk of urinary tract infections, sexual dysfunction, and even fatal consequences as a result of complications[6-10]. Therefore, further research is vital for advancing patient care in this field, and indeed, the high incidence and prevalence of BPH provide the large data sets necessary to train powerful AI models.
SEARCH STRATEGY AND ARTICLE SELECTION
The initial article collection strategy comprised a simple search on PubMed using the following phrases: “(artificial intelligence benign prostatic hyperplasia) OR (artificial intelligence benign prostatic enlargement)” since 2021, yielding 35 results.
Inclusion criteria:
Studies including data on benign prostatic hyperplasia/enlargement (BPH/BPE)
Primary research articles
Use of AI in diagnosis/management options/treatment outcomes
Human participants
Studies comparing prostate Ca with BPH/BPE
Full-text articles
Exclusion criteria:
Animal studies
Reviews/case studies/meta-analyses
Studies comparing AI in robotic surgery
Studies on prostate Ca purely
Studies outlining homeopathy-based diagnostics and/or treatments
Eight papers were selected for this review [Table 1].
Summary of research discussed in the article
Study | No. of participants | Applications | AI system used | Key findings | Main limitations |
Gao et al. Nov 2021[18] | 89 | Diagnosis | PSSNET segmentation method in CNN. | Segmenting prostate MRIs to delineate relevant parts for PCa and BPH diagnosis. PSSNet was a superior processing algorithm. CCN-based images increase diagnostic accuracy by 14%. | Small sample size. Comparison is made to cancer prostates and not normal prostates, so CNN is less likely to diagnose BPH solely. |
Zhang et al. Jan 2023[22] | 136 | Diagnosis | DL algorithm - CLSTM network vs ML algorithm (radiomics). | Found that a 20% increase in ROI from peritumoral tissue gives significant differences in diagnostic accuracy, with DL being superior to ML, when differentiating between PCa and BPH. | Single-center study, small sample size and the index suspicion lesion were initially outlined by radiologists who may harbor some bias. |
da Silva et al. Jun 2021[24] | 100 | Diagnosis | Paige Prostate. | AI applied to WSIs of TRUS prostate biopsies. Results showed that Paige Prostate had a sensitivity of 0.93 and a specificity of 0.98 for benign slides. | No inclusion of normal prostates. The focus of the study was not benign lesions. Unclear stats for benign diagnoses which had to be manually calculated. |
Liu et al. Jun 2022[26] | 315 | Diagnosis | Comparing 5 ML algorithms -RF, LR, GBDT, k-NN, and AdaBoost algorithms. | Used immunohistochemical markers P504 and P63 to develop an ML model that could predict P504/P63 status via MRI for pts with BPH, Pca and atypical hyperplasia. RF demonstrated the highest accuracy across all three labels. | Potential sampling errors due to biopsy sample vs whole prostatectomy sample. Different algorithms perform better/worse with differing sample sizes. Variation in IHC markers across racial cohorts. ROI not subdivided. |
Iwamura et al. Jul 2022[27] | 95 | Diagnosis | Tensor Flow Deep Learning Classifier algorithm. | Used immunoglobulin N-glycan signatures to aid in early diagnosis of BPH. Supervised ML-model training was undertaken with data from patients with known diagnoses of BPH. True positive/negative rates were 61.1%, with little overlap with non-prostatic diseases. | Considerable overlap of BPH with other prostatic diseases. Potential selection biases. |
Wang et al. Jan 2023[28] | 729 | Diagnosis | Used Pytorch to build a DL model (CNN). | Used SERS to create fingerprints of serum samples for patients with PCa vs. BPH. Applied CNN to analyze the SERS data. Results from CNN analysis evaluated through accuracy and ROC-AUC. Accuracy and ROC-AUC were highest when using SERS + PSA + age data for modeling. | Single-center study. Most limitations of the paper are relevant to differences between grades of PCa rather than the diagnosis of BPH. |
Tzelves et al. Jun 2022[32] | 153 | Management | Clustering analysis using k-means algorithm. | Used unsupervised methods of learning to identify whether patients with similarities/differences would be best managed through similar surgical techniques. No pattern of surgical choice was found based on patient pre-operative characteristics. | No significant outcomes using AI methods. |
Mourmouris et al. 2021[34] | 153 increased to 606 | Clinical outcomes after surgical management of BPE | Supervised regression algorithms employed in the WEKA data mining software for predictive modeling. | Compared different ML algorithms to see which is best for the prediction of outcomes of surgically managed BPE (IPSS score and Qmax changes). | Single-center study. Artificial increase in sample size. Lack of clarity whether test data sets used for predictive modeling was the same as the training data set - could lead to over-fitting. |
ARTIFICIAL INTELLIGENCE IN DIAGNOSTICS OF BPH
Diagnostics using non-invasive imaging techniques
Of the diagnostic methods used in the diagnosis of BPH, magnetic resonance imaging (MRI) is one of the most common[11,12]. Intra- and inter-variability of MRI interpretation by radiologists have led to attempts at standardization[13,14]. The application of AI to image analysis has the potential to eliminate human bias while significantly reducing the time required for interpretation[15]. The limitations of AI lie in its reduced accuracy and applicability to wide ranges of data sets compared to its human counterpart (i.e., radiologists)[16].
Radiologists will often manually segment prostate MRI images to facilitate diagnosis - a time-consuming process with variable outcomes[17]. Gao et al. aimed to produce high-quality prostate MRI segmentation by employing DL techniques[18]. The study sample comprised 89 patients with histologically confirmed PCa
Image analysis may provide enhanced results by extending the analysis area beyond solely the main region of interest (ROI). Zhang et al. conducted a comparative analysis between a DL algorithm, namely a bi-directional convolutional long short-term memory network (CLSTM), and a radiomics ML (non-DL) algorithm, for differentiating diagnoses of PCa versus BPH using dynamic contrast-enhanced MRI (DCE-MRI)[22]. The patient cohort consisted of 136 individuals who underwent prostate multi-parametric-MRI, with confirmed diagnoses of either PCa or BPH. While the importance of peritumoral tissue in diagnostics has been acknowledge, the extent to which the ROI should be increased has not been determined[23]. Researchers identified three different methods for extracting the peritumoral region and compared the diagnostic results obtained through examination of the tumor alone versus various ROIs, with altering amounts of peritumoral tissue.
The results revealed that employing the +/-20% region-growing method increased the mean area under the curve (AUC) for both DL and radiomics (0.89 and 0.79, respectively). The performance of CLSTM was overall superior to the radiomics model. Further expansion of the region-growing method reduced the AUC for both. These findings suggest that the accuracy of diagnosis can be improved by incorporating a slight increase in ROI outside the tumor zone, and the application of DL can further enhance this improvement.
Diagnostics using invasive biopsies
Biopsy sampling often follows imaging of prostatic lesions if deemed suspicious by radiologists. A 2021 study aimed to evaluate the accuracy of a CNN DL model called Paige Prostate in classifying transrectal ultrasound (TRUS) biopsy slides from 100 patients as either benign, suspicious, or malignant[24]. Paige Prostate identified 379 out of the total 579 samples as benign; this included one slide which after further analysis, had been labeled incorrectly by pathologists. There were two false positive and 27 false negative results. Overall, Paige Prostate demonstrated a sensitivity of 0.93 and a specificity of 0.98 for B. This demonstrates a potentially useful screening application for AI - the identification and exclusion of benign lesions prior to analysis by histopathologists.
It is widely acknowledged that the process of biopsy acquisition may engender varying degrees of anxiety in patients[25]. However, leveraging historical data on significant tumor markers and correlating it with imaging data presents an opportunity to predict the relationship between the two, and subsequently facilitate diagnosis without invasive procedures.
To this end, a recent 2022 study utilized immunohistochemical (IHC) markers P504s (positive in PCa and negative in BPH) and P63 (positive in BPH and negative in PCa)[26]. The authors aimed to develop a ML model that could apply MRI data as a surrogate to predict P504s/P63 status. A cohort of 351 patients with different diagnoses and IHC-staining patterns was divided into three groups, one with benign lesions
The RF model produced the highest ROC curve (0.92) for BPH, while k-NN demonstrated the highest specificity (0.886) and RF achieved the second-best specificity (0.857). GBDT showed the highest accuracy for BPH, with a value of 0.847, while RF’s accuracy was 0.831. All models displayed low sensitivity for atypical prostatic hyperplasia but high specificity. With such promising results, the need for invasive biopsy procedures may potentially be minimized in the future.
Diagnosis through analysis of serum components
Another non-invasive diagnostic technique is the analysis of serum components. In a recent study conducted by Iwamura et al., supervised ML models were trained on serum samples collected from a diverse cohort of 1,312 patients with nine different urological diagnoses[27]. The diagnoses included prostatic (BPH: n = 95) and non-prostatic diseases such as urosepsis. The task was to identify Ig N-glycan signatures (N-glycosylation patterns on the serum protein “Ig”) for each condition and diagnose it accurately. A cross-validation analysis identified the TensorFlow Deep Learning Classifier algorithm as the optimum model.
The study revealed that a combination of N-glycans A1FB, G1FB, G1[6], and G0FB is crucial for the detection of BPH. Upon testing, the model achieved a true positive/negative rate of 61.1% in BPH identification, with little overlap with non-prostatic diseases, as evidenced by an AUC and specificity at 90% sensitivity greater than 0.95% and 84.2%, respectively. However, the study also revealed a reduction in AUC/specificity when BPH was compared to other prostatic diseases.
Despite these limitations, the study represents a significant step forward in the development of a reliable diagnostic tool for BPH. By utilizing the power of supervised ML and Ig N-glycan signatures, the model shows promise in the early detection and accurate diagnosis of this common urological condition, potentially improving patient outcomes and quality of life. However, it is important to note that early detection in a pre-symptomatic setting is unlikely to be of clinical benefit to patients, and it is important to identify symptomatic cohorts for clinically applicable research that has positive effects on disease outcomes.
Similarly, Wang et al. explored the differences in blood composition between PCa and BPH using serum samples from 729 patients[28]. This approach was motivated by the fact that prostate-specific antigen (PSA) has reduced specificity when differentiating between similar prostatic diagnoses (e.g., PCa versus BPH versus prostatitis)[29,30]. The authors employed surface-enhanced Raman spectroscopy (SERS) to create spectra of multiple different biomarkers/metabolites that are differentially released in BPH and PCa. The technique generates complex fingerprints and peaks, making interpretation with traditional methods challenging. To address this, they utilized a CNN to analyze the data. The diagnostic precision of the CNN model was measured using percentage accuracy and ROC-AUC.
Notably, PCa and BPH groups showed distinct peaks and fingerprint patterns when analyzed using SERS. The accuracy achieved solely with SERS data was 85.14%, accompanied by an ROC-AUC of 0.87. By adding PSA and age data into the model, diagnostic accuracy increased to 88.55% with an ROC-AUC of 0.91. These findings hold promise for improved diagnostics without reliance on invasive techniques such as biopsies.
Artificial intelligence in the management of BPH
Several management options for BPH exist, creating the opportunity for potentially personalized surgical management for patients[31]. One study utilized unsupervised learning techniques to investigate whether patients (with BPE) with similar characteristics were chosen to undergo similar surgical techniques[32]. The study compared three surgical techniques: monopolar transurethral resection of the prostate (mTUR-P), bipolar transurethral resection of the prostate (bTUR-P), and bipolar transurethral vaporization of the prostate (bTUVis). The k-means clustering analysis algorithm utilized patterns in pre-operative variables (e.g., age, PSA, prostate volume, etc.) to identify groups of similar patients for whom particular surgical techniques may be preferred. However, due to the high variation of patient factors, surgeon’s preferences and experiences, skill, equipment availability, and other practical factors, no standard pattern of surgical technique selection was found. These results suggest that the choice of surgical technique may be more based on practical considerations rather than pre-operative patient factors analyzed in this study.
Artificial intelligence in predicting outcomes for BPH management
Improvement in quality of life following treatment is of great importance to patients[33]. In a study by Mourmouris et al., 153 men with benign prostatic enlargement (BPE) were treated using one of three methods - transurethral vaporization (TUVis), transurethral resection using bipolar energy (TURis), or transurethral resection using monopolar energy (TUR)[34]. The authors used the synthetic minority oversampling technique (SMOTE) to increase the size of the data set from 153 to 606 patients. Several supervised learning algorithms, including RF, k-NN, LR, and others, were employed in the WEKA data mining software to build predictive models.
Correlation coefficient (R), Mean Absolute Error (MAE), and Root Mean-Squared Error (RMSE) were used as performance metrics when predicting an increase in maximum urinary flow rate (Qmax) and reduction in international prostate symptom score (IPSS). RF outperformed all others in predicting Qmax increase and was superior for R and RMSE in predicting IPSS reduction.
DISCUSSION
Ultimately, AI aims to mimic clinicians in diagnostics, managing BPH, and outcome prediction. It has proven to be relatively accurate and rapid when tested on particular sets of data taking into account a small number of variables, as demonstrated in this review. AI can process massive amounts of data rapidly, and distinguish between similarly presenting conditions, such as BPH and PCa.
However, even with the use of layers of analyses and algorithms (such as in CNNs), there is little research that integrates patient factors more holistically, such as combining serum analyses and imaging, to create predictive models. Taking multiple patient and diagnostic factors into consideration is the bread and butter of a clinician/surgeon during decision-making. One study in this review demonstrated that adding a layer of this information can improve predictive accuracy, displaying promise for further research[28].
Much of the research presented in this paper is primarily based on PCa, with applications to BPH simply being a by-product or an interpretation from the studies, rather than the focus. A limitation of such research is the lack of control cohorts when training AI models. BPH appears to be compared to PCa rather than being treated as a stand-alone diagnosis. This reduces the applicability of such research to daily diagnostics, where the requirement to identify “normal” is key. Furthermore, research concentrating on the benign prostate would encourage the recruitment of patients with symptomatic BPH, and pave the way for the identification of applications of AI in this cohort more specifically[35]. This should be the ultimate goal when striving for the best clinical practice; the benefit of applying AI to BPH cannot be reliably ascertained unless the results are applicable to issues faced by patients as opposed to academic diagnoses.
Additionally, in an attempt to incorporate malignant and benign prostatic conditions, limitations in the applicability of research emerge. This is exemplified by Liu et al., where the 3rd category of patients (aside from benign prostate and PCa) included all of the following diagnoses: high-grade prostatic intraepithelial neoplasia (HGPIN) + atypical adenomatous hyperplasia (AAH) + atypical small acinar proliferation (ASAP). The former two are P504s positive/P63 positive and the latter is P504s negative/P63 negative[26]. Grouping premalignant and benign conditions together, without considering differences in their IHC-staining patterns, diminishes the clinical relevance of the research[36]. Concentrating on establishing a strong base for applications of AI to BPH initially would create a platform for building comparisons between benign and malignant cases; this is currently difficult due to the lack of a well-established presence of AI in both sectors. It is crucial to note that the studies mentioned in this review have highlighted the sensitivity and specificity of their AI models, whereas PPV (positive predictive value) and NPV (negative predictive value) are arguably more important[37]. With the high pre-test probability of BPH due to its high prevalence in the population, the PPV and NPV can be distorted and cloud a clinician’s interpretation of model outcomes. Taking this into account is key and further highlights the need for testing symptomatic cohorts to maximize clinical appropriateness.
Standardization of reporting methodology, techniques, and results is a natural step forward when research in a particular sector progresses in numbers. AI in medicine/surgery is still relatively novel; therefore, quality control of relevant studies has not yet been established, creating concerns with transparency of algorithms and systems of calculating/presenting results. This creates a bottleneck when attempting to implement this research in clinical practice and health systems[38]. A systematic review by Nagendran et al. illustrated the issues related to the prospective study designs, methodology, use of small sample sizes, transparency of AI models, and adherence to existing reporting standards found in most AI studies[39]. As a result, the outcomes can be difficult for clinicians to interpret and rely upon, thereby preventing the implementation of the technology. Standardization in this field, combined with open-source sharing, would massively accelerate the employment of AI in clinical systems.
The accuracy of AI predictions can be enhanced when sample sizes (input) are augmented appropriately[40,41]. Several studies in this review have relatively low patient numbers, largely due to being single-center studies. Liu et al. discussed that different algorithms have varying performances based on sample size[26]. Therefore, the superior models in these studies may not be the correct model of choice when applied to the large sample size faced in daily practice. Advances in prostate segmentation for PCa using AI versus expert radiologists have been demonstrated in recent times, but the lack of external validation using larger sample sizes has prevented clinical implementation[42]. Multicenter studies allowing the accumulation of larger patient cohorts could overcome this limitation with improved results. The quality of output of any AI model is primarily based on the quality and quantity of the input; case-based reasoning strengthens as the number of stored cases increases[1]. The cases of benign disease far outweigh that of cancer, reinforcing the idea that AI is better applied in the former sector than the latter.
Going even further than multicenter studies, the inclusion of patient cohorts across the globe would further enhance the applicability of AI models. Racial bias in such studies is common, reducing the accuracy of results when applied to ethnic minorities[26,43,44]. Widening the diversity of patients has already proven to overcome this challenge, and will allow AI to be utilized in places where it is most needed[45-47]. It would also be paramount to conduct these studies using BPH cohorts rather than PCa ones, with the aim of starting to use AI to distinguish between features of symptomatic versus asymptomatic BPH, eventually predicting suitable intervention options.
CONCLUSION
In summary, this review presents potential applications of AI in the field of BPH when considering diagnostics, management, and outcome prediction. As expected, much of the advancement has been in image analysis, which utilizes the abundant data sets currently available. However, the development of non-invasive diagnostic techniques to replace invasive ones might be most impactful for patients. Enhancement of this technology for driving the choice of management would move us closer to personalized medicine/surgery and likely minimize the number of ineffective procedures a patient experiences before positive results.
While we have discussed the advantages of this research, gaps and limitations of current approaches have inevitably been highlighted. The most glaring deficit noted is the lack of investigation of AI primarily in BPH, with most studies focusing on PCa with applications to BPH simply being an extrapolation. Moreover, the clinical significance of conditions should be central to studies for high impact and applicability; studying symptomatic BPH is far more relevant practically. The design and reporting standards of AI studies have also been briefly criticized, which may affect the validity of their results.
Suggestions for future research would include the incorporation of multiple layers of information in CNNs, increased diversity of patient cohorts, research on decision making for invasive methods such as biopsies using AI predictions, and inclusion of normal controls in model training. With further advancements in this field, standardization of methodology, reporting and measuring outcomes are a natural progression and would lead to a more robust setup. We conclude that recent research presents AI as a promising adjunct for applications in BPH, and discussed avenues with great potential to mature the field from its current nascent stages to the clinic.
DECLARATIONS
AcknowledgementWe would like to thank Camillo Moschner, Robert Smyth and Roy Zhang for proofreading the article.
Authors’ contributionsMade substantial contributions to the conception and design of the study, data analysis and interpretation, and creation of the manuscript: Bansal S
Performed data acquisition, as well as provided administrative, technical, and material support: Chuluunbaatar Y, Brodie A, Vasdev N
Availability of data and materialsNot applicable.
Financial support and sponsorshipNone.
Conflicts of interestAll authors declared that there are no conflicts of interest.
Ethical approval and consent to participateNot applicable.
Consent for publicationNot applicable.
Copyright© The Author(s) 2023.
REFERENCES
1. Sarker IH. AI-based modeling: techniques, applications and research issues towards automation, intelligent and smart systems. SN Comput Sci 2022;3:158.
2. Gumbs AA, Alexander F, Karcz K, et al. White paper: definitions of artificial intelligence and autonomous actions in clinical surgery. Art Int Surg 2022;2:93-100.
3. Brodie A, Dai N, Teoh JY, Decaestecker K, Dasgupta P, Vasdev N. Artificial intelligence in urological oncology: An update and future applications. Urol Oncol 2021;39:379-99.
5. GBD 2019 Benign Prostatic Hyperplasia Collaborators. The global, regional, and national burden of benign prostatic hyperplasia in 204 countries and territories from 2000 to 2019: a systematic analysis for the Global Burden of Disease Study 2019. Lancet Healthy Longev 2022;3:e754-76.
6. Gratzke C, Bachmann A, Descazeaud A, et al. EAU Guidelines on the assessment of non-neurogenic male lower urinary tract symptoms including benign prostatic obstruction. Eur Urol 2015;67:1099-109.
8. Behcet M, Avcioglu F. Causative Agents of Urinary Tract infections in elderly men with benign prostatic hyperplasia: a microbiological evaluation. Clin Lab 2021;67.
9. Yang CY, Chen GM, Wu YX, et al. Clinical efficacy and complications of transurethral resection of the prostate versus plasmakinetic enucleation of the prostate. Eur J Med Res 2023;28:83.
10. Launer BM, McVary KT, Ricke WA, Lloyd GL. The rising worldwide impact of benign prostatic hyperplasia. BJU Int 2021;127:722-8.
12. Wasserman NF. Benign prostatic hyperplasia: a review and ultrasound classification. Radiol Clin North Am 2006;44:689-710, viii.
13. Stabile A, Giganti F, Kasivisvanathan V, et al. Factors influencing variability in the performance of multiparametric magnetic resonance imaging in detecting clinically significant prostate cancer: a systematic literature review. Eur Urol Oncol 2020;3:145-67.
14. Brembilla G, Dell’Oglio P, Stabile A, et al. Interreader variability in prostate MRI reporting using Prostate Imaging Reporting and Data System version 2.1. Eur Radiol 2020;30:3383-92.
15. Berlin C, Adomeit S, Grover P, et al. Novel AI-based algorithm for the automated computation of coronal parameters in adolescent idiopathic scoliosis patients: a validation study on 100 preoperative full spine X-rays. Global Spine J 2023; doi: 10.1177/21925682231154543.
16. Syer T, Mehta P, Antonelli M, et al. Artificial intelligence compared to radiologists for the initial diagnosis of prostate cancer on magnetic resonance imaging: a systematic review and recommendations for future studies. Cancers 2021;13:3318.
17. Montagne S, Hamzaoui D, Allera A, et al. Challenge of prostate MRI segmentation on T2-weighted images: inter-observer variability and impact of prostate morphology. Insights Imaging 2021;12:71.
18. Gao W, Zhang P, Wang H, Tuo P, Li Z. Magnetic resonance imaging image feature analysis algorithm under convolutional neural network in the diagnosis and risk stratification of prostate cancer. J Healthc Eng 2021;2021:1034661.
19. Gandhi J, Weissbart SJ, Kim AN, Joshi G, Kaplan SA, Khan SA. Clinical considerations for intravesical prostatic protrusion in the evaluation and management of bladder outlet obstruction secondary to benign prostatic hyperplasia. Curr Urol 2018;12:6-12.
20. Awaisu M, Ahmed M, Lawal AT, et al. Correlation of prostate volume with severity of lower urinary tract symptoms as measured by international prostate symptoms score and maximum urine flow rate among patients with benign prostatic hyperplasia. Afr J Urol 2021;27:16.
21. Wasserman NF, Spilseth B, Golzarian J, Metzger GJ. Use of MRI for lobar classification of benign prostatic hyperplasia: potential phenotypic biomarkers for research on treatment strategies. AJR Am J Roentgenol 2015;205:564-71.
22. Zhang Y, Li W, Zhang Z, et al. Differential diagnosis of prostate cancer and benign prostatic hyperplasia based on DCE-MRI using bi-directional CLSTM deep learning and radiomics. Med Biol Eng Comput 2023;61:757-71.
23. Algohary A, Alhusseini M, Breto AL, et al. Longitudinal changes and predictive value of multiparametric MRI features for prostate cancer patients treated with MRI-guided lattice extreme ablative dose (LEAD) boost radiotherapy. Cancers 2022;14:4475.
24. da Silva LM, Pereira EM, Salles PG, et al. Independent real-world application of a clinical-grade automated prostate cancer detection system. J Pathol 2021;254:147-58.
25. Mao J, Dai Y, Wang L, Pan S, Wang W, Yu H. 'Is it painful'? A qualitative study on experiences of patients before prostate needle biopsy. BMJ Open 2022;12:e056619.
26. Liu YF, Shu X, Qiao XF, et al. Radiomics-based machine learning models for predicting P504s/P63 immunohistochemical expression: a noninvasive diagnostic tool for prostate cancer. Front Oncol 2022;12:911426.
27. Iwamura H, Mizuno K, Akamatsu S, et al. Machine learning diagnosis by immunoglobulin N-glycan signatures for precision diagnosis of urological diseases. Cancer Sci 2022;113:2434-45.
28. Wang Y, Qian H, Shao X, et al. Multimodal convolutional neural networks based on the Raman spectra of serum and clinical features for the early diagnosis of prostate cancer. Spectrochim Acta A Mol Biomol Spectrosc 2023;293:122426.
29. Ezenwa EV, Tijani KH, Jeje EA, et al. The value of percentage free prostate specific antigen (PSA) in the detection of prostate cancer among patients with intermediate levels of total PSA (4.0-10.0 ng/mL) in Nigeria. Arab J Urol 2012;10:394-400.
30. Lenzer J, Brownlee S. US expert panel recommends against prostate cancer screening. BMJ 2011;343:d6479.
31. Pandolfo SD, Del Giudice F, Chung BI, et al. Robotic assisted simple prostatectomy versus other treatment modalities for large benign prostatic hyperplasia: a systematic review and meta-analysis of over 6500 cases. Prostate Cancer Prostatic Dis 2022;1-16.
32. Tzelves L, Feretzakis G, Kalles D, et al. Cluster analysis assessment in proposing a surgical technique for benign prostatic enlargement. Stud Health Technol Inform 2022;295:466-9.
33. Oswald N, Hardman J, Kerr A, et al. Patients want more information after surgery: a prospective audit of satisfaction with perioperative information in lung cancer surgery. J Cardiothorac Surg 2018;13:18.
34. Mourmouris P, Tzelves L, Feretzakis G, et al. The use and applicability of machine learning algorithms in predicting the surgical outcome for patients with benign prostatic enlargement. Which model to use? Arch Ital Urol Androl 2021;93:418-24.
35. Lee CL, Kuo HC. Current consensus and controversy on the diagnosis of male lower urinary tract symptoms/benign prostatic hyperplasia. Ci Ji Yi Xue Za Zhi 2017;29:6-11.
36. Armah HB, Parwani AV. Atypical adenomatous hyperplasia (adenosis) of the prostate: a case report with review of the literature. Diagn Pathol 2008;3:34.
37. Trevethan R. Sensitivity, specificity, and predictive values: foundations, pliabilities, and pitfalls in research and practice. Front Public Health 2017;5:307.
38. Aristidou A, Jena R, Topol EJ. Bridging the chasm between AI and clinical implementation. Lancet 2022;399:620.
39. Nagendran M, Chen Y, Lovejoy CA, et al. Artificial intelligence versus clinicians: systematic review of design, reporting standards, and claims of deep learning studies. BMJ 2020;368:m689.
40. Hu X, Li B, Mo Y, Alselwi O. Progress in artificial intelligence-based prediction of concrete performance. ACT 2021;19:924-36.
41. Nebot JA. A review of artificial intelligent approaches applied to part accuracy prediction. IJMMM 2010;8:6.
42. Bhattacharya I, Khandwala YS, Vesal S, et al. A review of artificial intelligence in prostate cancer detection on imaging. Ther Adv Urol 2022;14:17562872221128791.
43. Seyyed-Kalantari L, Zhang H, McDermott MBA, Chen IY, Ghassemi M. Underdiagnosis bias of artificial intelligence algorithms applied to chest radiographs in under-served patient populations. Nat Med 2021;27:2176-82.
44. Hong C, Pencina MJ, Wojdyla DM, et al. Predictive Accuracy of stroke risk prediction models across black and white race, sex, and age groups. JAMA 2023;329:306-17.
45. Wang R, Chaudhari P, Davatzikos C. Bias in machine learning models can be significantly mitigated by careful training: evidence from neuroimaging studies. Proc Natl Acad Sci U S A 2023;120:e2211613120.
46. Istasy P, Lee WS, Iansavichene A, et al. The impact of artificial intelligence on health equity in oncology: scoping review. J Med Internet Res 2022;24:e39748.
47. Applicability of Artificial Intelligence in Healthcare in Resource-Poor Settings. Harvard Health Policy Review2022. Available from: https://dimesociety.org/journal/applicability-of-artificial-intelligence-in-healthcare-in-resource-poor-settings/. [Last accessed on 1 Aug 2023].
Cite This Article
Export citation file: BibTeX | RIS
OAE Style
Bansal S, Chuluunbaatar Y, Brodie A, Vasdev N. Applications of artificial intelligence in benign prostatic hyperplasia. Art Int Surg 2023;3:129-39. http://dx.doi.org/10.20517/ais.2023.07
AMA Style
Bansal S, Chuluunbaatar Y, Brodie A, Vasdev N. Applications of artificial intelligence in benign prostatic hyperplasia. Artificial Intelligence Surgery. 2023; 3(2): 129-39. http://dx.doi.org/10.20517/ais.2023.07
Chicago/Turabian Style
Bansal, Saakshi, Yanjinlkham Chuluunbaatar, Andrew Brodie, Nikhil Vasdev. 2023. "Applications of artificial intelligence in benign prostatic hyperplasia" Artificial Intelligence Surgery. 3, no.2: 129-39. http://dx.doi.org/10.20517/ais.2023.07
ACS Style
Bansal, S.; Chuluunbaatar Y.; Brodie A.; Vasdev N. Applications of artificial intelligence in benign prostatic hyperplasia. Art. Int. Surg. 2023, 3, 129-39. http://dx.doi.org/10.20517/ais.2023.07
About This Article
Special Issue
Copyright
Data & Comments
Data
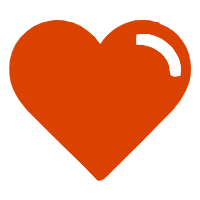

Comments
Comments must be written in English. Spam, offensive content, impersonation, and private information will not be permitted. If any comment is reported and identified as inappropriate content by OAE staff, the comment will be removed without notice. If you have any queries or need any help, please contact us at support@oaepublish.com.