Advances in radiomics applications for intrahepatic cholangiocarcinoma: a comprehensive review and future directions
Abstract
Radiomics was first introduced by Lambin et al. in 2012, and since then, research in this field has grown rapidly. Researchers have shown great interest in developing efficient methods for automatically extracting a large number of quantitative features from medical images, aiming to enhance diagnostic accuracy and predictive capability. Although there has been a rise in Radiomics studies focusing on intrahepatic cholangiocarcinoma (ICC) in recent years, comprehensive reviews are still relatively scarce. This study explores how Radiomics technology can be utilized in modeling analyses to predict lymph node metastasis, microvascular invasion, and early recurrence of ICC, as well as the application of deep learning in these analyses. This paper provides a brief overview of the current state of Radiomics research and offers references for future studies.
Keywords
INTRODUCTION
In the past decade, the dramatic increase in computing power and memory has enabled the development and implementation of advanced artificial intelligence (AI) technologies for processing radiological images, particularly in the area of tumor imaging. Since its introduction in 2012, radiomics has attracted considerable attention[1]. Traditional imaging is limited to capturing basic semantic features and has a constrained capability to accurately reflect tumor characteristics. However, radiomics methods can provide rich and significant supplementary data. After more than a decade of development, AI-based diagnostic tools have been continually improved. In many cases, their diagnostic performance has been proven to match or even surpass that of human experts across various types of cancer[2,3]. These successes have encouraged the continued use of AI methods for more complex decision-making tasks, such as disease prediction, predicting responses to different treatment modalities, identifying treatment-related changes, and discovering imaging-based representations of phenotypic (e.g., gender, age, or race) and genotypic features associated with prognosis.
Intrahepatic cholangiocarcinoma (ICC) ranks as the second most common type of primary liver cancer[4]. Based on different classification methods, the most common type of cholangiocarcinoma is ICC, which is a malignant tumor in the epithelial cells of the intrahepatic bile ducts, occurring in small intrahepatic bile ducts or bile ducts near the bifurcation of the hepatic duct[5]. Epidemiological studies have shown an increase in the incidence and mortality rates of ICC globally in recent years[6]. Due to the absence of specific clinical manifestations in the early stages of the disease, most patients are incidentally diagnosed during routine physical examinations[7]. A minority of patients who have symptoms present with non-specific features, leading to over 70% of patients being diagnosed in the late stages of the disease [American Joint Committee on Cancer (AJCC) stage III or IV], resulting in an average 1-year and 5-year survival rate of only 30% and 18%, respectively. Due to the increasing incidence of ICC, several studies have focused on improving patient diagnosis, prognosis, and treatment[8-11]. The diagnosis of ICC is typically achieved through serum markers [CA 19-9, Carcinoembryonic Antigen(CEA)] and imaging examinations. However, in atypical cases, differential diagnosis remains challenging, making biopsy the only definitive tool for accurate diagnosis[12].
Currently, the most widely used staging system for ICC is the AJCC tumor, lymph node, and metastasis (TNM) staging system, which relies on surgical pathology data and is therefore applicable only in a postoperative context. Regarding clinical characteristics, imaging manifestations, and treatment approaches, ICC stands out as a distinct and habitual malignant tumor, differing from perihilar and distal bile duct cancers. Therefore, a special prognostic prediction model is necessary. Many researchers have been able to establish models that can objectively and accurately predict the prognosis of ICC patients based on preoperative clinical or imaging data such as CA199 levels, tumor volume under computed tomography (CT) and magnetic resonance imaging (MRI), the presence of intrahepatic multifocal metastasis, distant organ metastasis, and invasion of major blood vessels in the liver. These models demonstrate superior discriminative ability and accuracy compared to the 8th edition of the AJCC TNM staging system.
For preoperative tumor staging and the assessment of resectability in ICC, cross-sectional CT and MRI are the most commonly utilized imaging techniques. Physicians can leverage this imaging information to refine and optimize treatment plans. However, many details in medical imaging data cannot be identified and utilized by the naked eye. The full potential of these details in clinical diagnosis and treatment has not been fully exploited. Nevertheless, the rapid development of high-throughput methods in recent years makes this idea increasingly feasible. High-throughput methods, which can automatically extract numerous quantitative imaging features from medical imaging data, have garnered significant interest recently due to their potential to enhance predictive and diagnostic performance. In the field of ICC, radiomics has been widely applied. When combined with various machine learning techniques, radiomics demonstrates excellent predictive capabilities regarding prognosis, recurrence, and survival in ICC patients. This review aims to discuss the practical applications of radiomics in the clinical practice of ICC. The included studies mainly address the following topics: prediction of lymph node metastasis (LNM), microvascular invasion, early postoperative recurrence, survival, and differentiation of ICC from other diseases.
MATERIALS AND METHODS
To systematically review the application of radiomics in ICC, this study conducted a literature search in databases such as PubMed, Embase, and Google Scholar. The search terms included “ICC” combined with “radiomics”, “texture analysis”, and “imaging features”. Only studies published before May 30, 2024, were included. Duplicate records were first removed, followed by a screening process based on titles and abstracts. Non-English full texts, studies unrelated to the research topic, preclinical studies with no translational significance, case reports, and editorials were excluded. For studies involving multiple tumor types, only those presenting radiomics results specific to ICC were retained. Relevant full-text articles were then retrieved and further reviewed.
For quality control of the included studies, we used the Diagnostic Accuracy Study Quality Assessment Tool 2 (QUADAS-2) for independent evaluation. The assessment covered “patient selection”, “index test”, “reference standard”, and “flow and timing”. Each study’s risk of bias and applicability were rated as “high”, “low” or “unclear”. Two independent reviewers performed the ratings, with a third reviewer involved in resolving disagreements until a consensus was reached. Additionally, to further enhance the credibility of the results, we conducted a backward reference search of all included studies’ reference lists to ensure no significant studies were missed.
RADIOMICS WORKFLOW
The earliest applications of radiomics can be traced back to a study[13] that demonstrated the correlation between patient time to progression in lung cancer, and another study by Segal et al., which derived 28 imaging features that could reconstruct 78% of the global gene expression profile, revealing cell proliferation, liver synthesis function, and patient prognosis[14]. Lambin et al. published a landmark paper that formally coined the term “radiomics” thus garnering significant attention for the concept[1].
Image acquisition
High-quality and standardized images are typically obtained through imaging modalities such as MRI, CT, positron emission tomography - computed tomography(PET-CT), and ultrasound. In the clinical management of nearly all patients with ICC, CT or MRI imaging is routinely performed to acquire pertinent diagnostic images. Ensuring the quality and standardization of these images is crucial.
Region of interest segmentation
In most cases, experienced radiologists or radiation oncologists manually delineate the tumors. However, there has been development in automatic and semi-automatic segmentation methods, with some automatic approaches achieving results comparable to those of manual segmentation by radiologists. In the field of medical imaging, a variety of techniques are available for both semi-automatic and fully automatic segmentation, each employing tools of different levels of sophistication. Due to the inherently hypovascular nature of ICC, the tumor often exhibits distinct contrast with the surrounding normal liver tissue at certain stages, making the delineation of its boundaries relatively straightforward. Consequently, the performance of automated segmentation methods can even surpass that of manual tumor delineation by experienced radiologists. Basic segmentation methods primarily analyze the intensity and contrast within images, with a popular method being segmentation based on predefined thresholds. Alternatively, region-based segmentation identifies groups of similar and connected voxels using criteria based on uniformity[15]. These methods are typically semi-automatic because they require human intervention to set thresholds or validate the uniformity criteria.
Further, some segmentation research incorporates models of uncertainty and algorithms for optimization to pinpoint specific areas within an image. Techniques such as statistical pattern recognition, which automatically identifies and categorizes patterns in images, c-clustering, which sorts images into distinct groups without supervision, and graph-based segmentation, which visualizes images as networks of voxels connected by edges optimized through a cost function, are frequently applied in the study of radiomics[16,17].
Extraction and analysis of radiomics features
Multi-phase contrast-enhanced computed tomography (CE-CT) is regarded as the standard imaging modality for diagnosing ICC. ICC typically manifests as peripheral arterial phase hyperenhancement (APHE), peripheral washout, and delayed central enhancement. Consequently, the arterial phase of CT is considered the optimal timing for obtaining imaging data.
Radiomics features are divided into two categories: semantic features and agnostic features[18]. Semantic features involve visible characteristics such as the size, shape, location, and degree of necrosis of tumor lesions. Although the analysis of these features is relatively easy to implement in clinical practice, they typically rely on the diagnostician’s experience, which can lead to variability in assessments both between different observers and within the same observer over time. In contrast, agnostic features are quantitative descriptors extracted from tissues of interest using mathematical methods and are not included in standard radiological reports. Agnostic features utilize advanced mathematical algorithms and are divided into morphological and statistical features. Morphological features describe the shape and physical structure of the segmented volume. Statistical features are further subdivided into first-order, second-order, and higher-order features. First-order features focus on the attributes of individual voxels, such as brightness or intensity, and include average intensity, maximum and minimum intensity, standard deviation, and histogram analysis, revealing the distribution and variation of pixel intensities within the image[19]. Second-order features, also known as texture features, describe the texture patterns of an image by analyzing the spatial relationships between voxels. Commonly used second-order features include the gray-level co-occurrence matrix (GLCM), gray-level run-length matrix (GLRLM), and gray-level size zone matrix (GLSZM), which explore the spatial correlations of voxel intensities. Higher-order features employ more complex mathematical models and algorithms, such as wavelet transforms or deep learning technologies, to analyze the intricate relationships between voxels and extract deeper layers of image data. In ICC, morphological and statistical features are crucial for identifying texture patterns and heterogeneity within tumors. Features like the GLCM and wavelet transforms are particularly useful in discerning subtle differences that may indicate aggressive tumor behavior.
Predictive model building
Radiomics modeling primarily encompasses feature selection, modeling methodologies, and validation[20]. This intricate process initiates with the extraction of pertinent quantitative features from a plethora of imaging attributes, encompassing aspects such as morphology, texture, and statistical properties. During the feature selection phase, sophisticated statistical analyses and advanced machine learning algorithms are employed to discern the most diagnostic or prognostic features. Commonly utilized techniques include principal component analysis (PCA), least absolute shrinkage and selection operator (LASSO) regression, and Random Forests, all of which are vital for mitigating feature redundancy and augmenting model efficacy.
Subsequently, in the modeling methodologies phase, the curated features are leveraged to construct predictive or classification models. Frequently employed modeling techniques encompass linear regression, logistic regression, support vector machines (SVM), Random Forests, and neural networks. Each technique possesses unique merits and specific applications. For instance, linear regression is apt for predicting continuous variables, logistic regression is utilized for binary classification challenges, whereas SVMs and Random Forests excel in managing high-dimensional data and non-linear problems. Neural networks, particularly deep learning models such as Convolutional Neural Networks, are remarkably proficient in processing intricate imaging data by autonomously extracting and amalgamating features, thereby enhancing model precision.
Finally, during the validation phase, the model’s performance and reliability are meticulously evaluated through both internal and external validation. Internal validation commonly adopts methods such as cross-validation and leave-one-out by partitioning the dataset into multiple subsets, sequentially utilizing one subset as the validation set while the remainder as the training set, to comprehensively assess the model’s performance across varying subsets. External validation employs an independent dataset to rigorously test the model, appraising its generalization capability and practical application value. Common performance metrics include accuracy, sensitivity, specificity, and the area under the ROC curve (AUC-ROC).
By adhering to these rigorous steps, radiomics is capable of extracting a copious amount of valuable quantitative data from medical images, unveiling potential correlations between imaging features and tumor heterogeneity. This capability not only facilitates a more precise assessment of the biological behavior and prognosis of tumors but also provides robust support for clinicians in diagnostic and therapeutic decision-making, thereby propelling the advancement of personalized medicine. By integrating radiomic features with clinical, pathological, and genetic data to construct multimodal predictive models, radiomics is progressively becoming an indispensable tool for enhancing clinical practice[21-23][Figure 1].
APPLICATIONS OF RADIOMICS IN ICC
Differentiating ICC from other diseases
The preoperative diagnosis of ICC currently relies mainly on imaging studies and CA19-9 levels[7,24]. However, conventional imaging often provides limited information, and some patients’ CA19-9 levels remain within the normal range, making it challenging to accurately differentiate ICC from other liver lesions[25]. Enhanced imaging offers some diagnostic differentiation value, with ICC typically showing progressive, centripetal enhancement, which can be distinguished from certain liver lesions. Nevertheless, observation of enhanced imaging is subjective, and due to the heterogeneity of ICC, some ICC imaging presentations are atypical and can overlap with other lesions, necessitating a precise and objective method to overcome these shortcomings. Radiomics, capable of mining and extracting information on intratumoral heterogeneity at high throughput, holds promise for differentiating ICC from intrahepatic bile duct stones[26], other primary liver cancers[27-33], etc.[34,35].
In one study, Wang et al. delineated tumor region of interest (ROIs) and expanded them to create multiple ROI expansion areas (-2, 0, 2, 4, 6, and 8 mm) in MRI images of 87 hepatocellular carcinoma (HCC) patients and 75 pathologically confirmed ICC patients, establishing several predictive models[36]. By combining convolutional features, the model’s performance was further improved. Compared to the standard ROI, expanded areas achieved better predictive performance, with the 6 mm expansion area exhibiting the best prediction ability [area under the curve (AUC): 0.84 vs. 0.86 vs. 0.88 vs. 0.90 vs. 0.94 vs. 0.82]. To differentiate HCC and ICC, Huang et al. developed a deep learning model based on channel and spatial attention mechanisms, called CSAM-Net, outperforming traditional radiomics models (RMs) constructed with logistic regression, LASSO regression, SVM, and random forests (AUC = 0.987 vs. 0.913)[37]. Another study by Liu et al. indicated that using machine learning to analyze MRI and CT radiomics features for differentiating cHCC-ICC from HCC and ICC showed good predictive performance, potentially impacting treatment decisions[28]. Additionally, Jiang et al. established a predictive model composed of 2 PET and 1 CT radiomics feature, finding that PET and CT radiomics features could effectively differentiate HCC and ICC, with an AUC of 0.86; clinical factors did not significantly enhance discrimination, with clinical models and combined models of radiomics features and clinical features yielding AUCs of 0.56 and 0.80, respectively[38]. These studies demonstrate that radiomics can provide molecular-based imaging features and intratumoral heterogeneity information. When combined with clinical information, it can effectively differentiate HCC and ICC, suggesting that future radiomics should integrate various clinical factors to more effectively distinguish ICC from HCC. Differentiation between ICC and CHC is also a significant direction in clinical practice. Zhou et al. developed radiomics features based on MRI images, selecting 11 radiomics features and integrating alpha-fetoprotein, background liver disease (cirrhosis or chronic hepatitis) to construct a nomogram, showing strong calibration and discrimination performance, with training and validation cohort AUCs of 0.945 and 0.897, respectively[39]. The radiomics nomogram proved superior to radiomics features and individual clinical models (P < 0.05). Xue et al., based on arterial phase CT images of 131 patients with intrahepatic bile duct stones and concurrent tumors, extracted four radiomics features, combined with three clinical features (fever, CA 19-9, and CEA), establishing a combined model[26]. This model showed the best performance in predicting ICC concurrent with intrahepatic bile duct stones, with an AUC of 0.902. Another study by Xue et al. extracted radiomics features from arterial and venous phase enhanced CT images, building a model with phase-specific radiomics scores and two clinical factors (CEA and CA19-9), achieving the best model performance, with training and validation group AUCs of 0.864 and 0.843, respectively, surpassing simple RMs (training group AUC: 0.809, validation group AUC: 0.790) and clinical variable models (training group AUC: 0.801, validation group AUC: 0.830), indicating the combined model can distinguish ICC from inflammatory tumors with intrahepatic bile duct stones, improving diagnostic accuracy[40]. Xu et al. utilized enhanced CT to extract texture features, identifying 38 eligible texture features[32]. Through five feature selection methods (distance correlation, random forest, LASSO, etc.) and nine feature classification methods (linear discriminant analysis, SVM, logistic regression, etc.), 45 predictive models were established to differentiate ICC from liver lymphoma. Most models performed well (AUC > 0.85), with the random forest linear discriminant analysis showing the best performance among the 45 models (AUC = 0.997), suggesting that combining enhanced CT texture features with multiple machine learning models can effectively distinguish ICC from liver lymphoma. Xu et al., based on multiparametric MRI images, extracted radiomics features using the LASSO algorithm and selected clinical variables (tumor diameter and CEA level) and MRI results to construct a clinical model[34]. The final radiomics nomogram model, which integrates both the clinical and RMs, demonstrated superior performance in differentiating intrahepatic mass-forming cholangiocarcinoma (IMCC) from colorectal liver metastasis (CRLM). The model achieved AUCs of 0.94 (95%CI: 0.90-0.97) for the training cohort, 0.93 (95%CI: 0.86-1.00) for the internal validation cohort, and 0.92 (95%CI: 0.84-1.00) for the external validation cohort. Table 1 summarizes the models and results of the analyzed studies.
Differentiating ICC from other diseases
Author | Years | Image | Differential diagnosis | Number of cases | AUC |
Wang et al.[36] | 2024 | MRI | HCC-ICC | HCC(n = 87) ICC(n = 75) | 0.96 |
Liu et al.[30] | 2023 | MRI | HCC-ICC | HCC(n = 129) ICC(n = 48) | 0.977 |
Xue et al.[40] | 2021 | CT | Inflammatory mass-ICC | Training (IM = 66, IMCC = 44) Validation (IM = 18, ICC = 17) | 0.864 |
Huang et al.[37] | 2023 | CT | HCC-ICC | HCC(n = 395) ICC (n = 99) | 0.987 |
Ren et al.[41] | 2021 | US | HCC-ICC | Training: 149 External validation: 38 test: 39 | 0.936 |
Xue et al.[26] | 2021 | CT | Intrahepatic lithiasis-ICC | Training (IBI = 60, ICC = 36) Validation (IBI = 18, ICC = 17) | 0.829 |
Xu et al.[34] | 2023 | MRI | Resectable colorectal liver metastases-ICC | Training (CRLM = 69, ICC = 64) Internal validation (CRLM = 28, ICC = 29) External validation (CRLM = 28, ICC = 23) | 0.92 |
Zhou et al.[39] | 2022 | MRI | CHC-ICC | Training (CHC = 45, ICC = 106) Validation (CHC = 19, ICC = 46) | 0.945 |
Xu et al.[32] | 2021 | CT | Hepatic lymphoma-ICC | HL = 28 ICC = 101 | 0.997 |
Chen et al.[27] | 2023 | MRI | HCC-ICC | HCC (n = 83) ICC (n = 51) | 0.9 |
Liu et al.[28] | 2021 | MRI/CT | HCC-CHC-ICC | HCC (n = 38) CHC (n = 24) ICC (n = 24) | 0.79/0.81/0.71 |
Predicting LNM
LNM is significantly associated with poor prognosis in patients with ICC and is a crucial determinant of the tumor’s resectability[24,42]. However, current methods for assessing lymph node status offer unstable predictive accuracy and limited discriminative capability[43]. As a result, some patients initially deemed operable during pre-surgical assessments are later found to have metastasis during surgery, leading to abortive operations. Fine-needle aspiration, despite being invasive, has limited effectiveness in detecting minor lymph node metastases and carries a risk of tumor spread[44,45]. The data from the papers are summarized in Table 2.
Predicting LNM
Author | Year | Image modality | Number of cases | Number of features | Feature selection and modeling method | Model performance (AUC, sensitivity, specificity) |
Xu et al.[46] | 2023 | CT | Training: 86; External validation: 30 | 6 | LASSO; LR | Combination model AUC: 0.85, Radiomics model: 0.82, Clinical model: 0.75 |
Zhang et al.[45] | 2022 | CT | ALL: 296 | 24 | mRMR; DT; LR | Combination model AUC: 0.98, Radiomics model: 0.70, Clinical model: 0.87 |
Xu et al.[47] | 2019 | MRI | Training: 106; External validation: 42 | 5 | mRMR; SVM | Radiomics model AUC: 0.787, Combination model AUC: 0.842 |
Ji et al.[48] | 2019 | CT | Training: 103; External validation: 52 | 8 | LASSO; LR | Combination model AUC: 0.89, Imaging model: 0.64, Clinical model: 0.72 |
Radiomics prediction models built using features extracted from CT or MRI can be used to preoperatively predict LNM, thereby enabling clinicians to make better-informed clinical decisions[49,50]. In 2019, Ji et al. extracted eight features related to lymph node status from CT images and, together with CA19-9 levels, built a combined predictive model[48]. The model, tested on a training set of 103 patients and a validation set of 52 patients, showed AUCs of 0.8462 and 0.8921, respectively. It categorized LNM into high-risk and low-risk groups, revealing significant differences in overall survival (OS) and recurrence-free survival between the two groups. However, this study’s patient groups, both for training and validation, were from a single center and lacked external validation. In another study from 2019, Xu et al. constructed a SVM model based on five selected imaging features from MRI, creating a combined nomogram model with SVM scores, CA19-9 levels, and MRI characteristics[50]. Compared to the standalone SVM model, the combined nomogram demonstrated superior discriminative ability in distinguishing between predicting LNM and non-LNM patients (AUC: training set: 0.842 vs. 0.788; validation set: 0.870 vs. 0.787). Zhang et al. integrated radiomics features from multiple CT sequences to build a fusion model, which, across three cohorts (training, external validation, and internal validation), showed AUC values surpassing those of clinical RMs[45]. The constructed nomogram, validated independently, displayed good differentiation and prognostic value. In summary, employing radiomics prediction models based on features extracted from CT or MRI holds promise for accurately predicting preoperative lymph node status, potentially becoming a valuable tool for preoperative assessment and prognostic evaluation in ICC.
Predicting microvascular invasion
Microvascular invasion is an independent risk factor affecting the prognosis of patients undergoing radical resection for ICC[42,51], with those experiencing predicting microvascular invasion (MVI) often facing a poorer prognosis[52]. However, clinical detection of MVI is primarily conducted through microscopic histopathological examination, identifiable only postoperatively. Therefore, building models to predict MVI preoperatively, thus offering references for clinicians to adjust surgical methods and extents, has emerged as a hot topic in ICC research recently. The advent and development of radiomics, by enabling the extraction of features from the entire tumor and its surrounding areas, have continually improved the accuracy of preoperative MVI predictions. Table 3 summarizes the models and results of the analyzed studies.
Predicting MVI
Author | Year | Image modality | Number of cases | Number of features | Feature selection and modeling method | Model performance (AUC, sensitivity, specificity) |
Ma et al.[56] | 2023 | MRI | Training cohort (n = 111) Validation cohort (n = 49) | - | LASSO; LR; SVM | Imaging model AUC = 0.885, Radiomics model AUC = 0.987, Combination model AUC = 0.995 |
Qian et al.[57] | 2022 | MRI | Training: 130 External validation: 33; test: 24 | 2600 | LASSO; LR/RF/SVM | Imaging model AUC = 0.726, Radiomics model AUC = 0.950, Combination model AUC = 0.953 |
Fiz et al.[58] | 2023 | CT | 244 | - | LASSO; LR | Clinical model AUC = 0.75, Clinical + Radiomics model (tumor) AUC = 0.82, Clinical + Radiomics model (tumor + edge) AUC = 0.82 |
Jiang et al.[38] | 2022 | PET/CT | Training: 100 External validation: 27 | 1,815 | Hypothetical test; RF | Clinical model AUC = 0.67, PET model AUC = 0.88, CT model AUC = 0.67, PET + CT model AUC = 0.75, Clinical + PET + CT model AUC = 0.90 |
Fiz et al.[35] | 2022 | PET/CT | 74 | - | Backward stepwise/PCA | Clinical model AUC = 0.774, Clinical + Radiomics model AUC = 0.871, Tumor-/Margin + clinical + Radiomics model AUC = 0.882 |
Chen et al.[55] | 2023 | MRI | Training: 167 External validation: 68 | 1,132 | LASSO; LR; DT | Clinical model AUC = 0.787, Radiomics model AUC = 0.806, Clinical + Radiomics model AUC = 0.874 |
Zhou et al.[54] | 2021 | MRI | Training: 88 External validation: 38 | 788 | LASSO; LR | Radiomics model AUC = 0.873, External validation AUC = 0.850 |
Xiang et al.[53] | 2021 | CT | Training: 110 Validation: 47 | 157 | Hypothetical test; SVM | Imaging model AUC = 0.824, Radiomics model AUC = 0.802, Imaging + Radiomics model AUC = 0.886 |
In recent years, numerous studies utilizing radiomics to predict preoperative MVI have surfaced, trying various imaging modalities including ultrasound, CT, MRI, and PET-CT for model construction[38,53-56]. Qian et al. utilized the LASSO logistic regression to identify the optimal features from MRI images of patients in three cohorts (training cohort: n = 130, validation cohort: n = 33, test cohort: n = 24) and developed a radiomics model for preoperative MVI prediction[57]. This model showed excellent and consistent predictive performance in the training (AUC = 0.950), validation (AUC = 0.883), and test (AUC = 0.812) cohorts. Additionally, Qian et al. employed univariate and multivariate analyses to identify independent predictors of MVI status: larger tumor size (P = 0.003) and intrahepatic bile duct dilatation (P = 0.002)[57]. These factors were combined with the final radiomics model to create an MVI prediction nomogram, which also achieved strong predictive results across the training (AUC = 0.953), validation (AUC = 0.861), and test (AUC = 0.819) cohorts. In 2023, Ma similarly built a predictive model for MVI using MRI features. Unlike Qian et al.[57], Ma et al. compared the predictive capabilities of different MRI sequences and VOIs, selecting the three most optimal sequences (T1WI-D, T1WI, DWI) and one optimal VOI (including the tumor and a 10 mm peritumoral area), achieving desirable prediction outcomes (AUCTC = 0.987 and AUCVC = 0.859)[56]. The predictive ability further improved when combining tumor size, intrahepatic bile duct dilatation, and a multi-sequence fusion of VOI10 mm MRI in a radiomics model: TC (AUC = 0.995, 95%CI: 0.987-1.000) and VC (AUC = 0.867, 95%CI: 0.798-0.921). Additionally, Zhou et al. and Chen et al. developed RMs based on dynamic contrast-enhanced MRI images, achieving satisfactory results[54,55]. Beyond MRI, other imaging modalities also exhibited good predictive capabilities. In 2021, Xiang et al. constructed a portal phase image radiomics model with six features extracted from enhanced CT, displaying good predictive power in two cohorts (training group: n = 110, AUC = 0.804; validation group: n = 47, AUC = 0.769)[53]. The integration of significant clinical factors {satellite nodules [odds ratio (OR)=13.73], arterial hypointensity (OR = 4.31), and tumor contour (OR = 4.99)} with radiomics features into a nomogram achieved satisfactory predictive results, with AUCs of 0.886 in the training group and 0.80 in the validation group, significantly surpassing the simple radiomics model and the clinical model (training group AUC: 0.822, test group AUC: 0.756). Fiz et al. elucidated that preoperative CT portal phase extraction of tumor and peritumoral tissue imaging characteristics enhanced the prediction of ICC grading and MVI (clinical data model: AUC = 0.75; clinical data model + tumor-VOI: AUC = 0.82; clinical data model + tumor-/edge-VOI: AUC = 0.82), finding that combining tumor with peritumoral tissue imaging characteristics could improve predictive power[58]. Jiang et al., by extracting features from PET and CT to construct predictive models separately, showed that the PET group significantly outperformed the CT group in predicting MVI (PET: AUC = 0.88; CT: AUC = 0.67)[38]. However, the predictive ability of models combining PET and CT features decreased (PET + CT: AUC = 0.75). The best predictive results were achieved with a nomogram combining PET, CT, and clinical factors (PET + CT + Clinical: AUC = 0.90). It is evident that preoperative imaging-based prediction of MVI holds value, aiding doctors in making clinical decisions related to treatment plans and influencing.
Early recurrence post-surgery
Early recurrence is defined as recurrence within two years post-surgery or within one year postoperatively. It is a critical factor affecting overall patient survival[59,60]. Therefore, effectively identifying patients at high risk of early recurrence can help clinicians devise targeted treatment plans, thereby enhancing patient survival time. While some prediction models based on clinical features exist, their accuracy and applicability are limited, leading to an urgent need for new methods to identify ER patients. Medical imaging plays a crucial role in the preoperative assessment of ICC[61], making models built on image features for predicting ICC’s early recurrence a focus of research[62-66].
In 2018, Liang et al. were the first to use nine features extracted from MRI images to build a radiomics model to predict patients with ICC prone to early recurrence[67]. In the training cohort (n = 139) and the validation cohort (n = 70), the AUC of radiomics features was 0.82 (95%CI: 0.74-0.88) and 0.77 (95%CI: 0.65-0.86), respectively. Liang et al. then developed a radiomics nomogram that combined imaging features and tumor clinical staging, achieving better predictive capacity with AUCs of 0.90 (95%CI: 0.83-0.94) and 0.86 (95%CI: 0.76-0.93) across both cohorts[67]. Differing from Liang et al.[67], Xu et al. not only focused on the internal structure features of tumors in MRI images but also integrated peritumoral area features (3 and 5 mm), finding that models combining internal tumor structure and a 5 mm peritumoral area performed better than those that only considered internal tumor structure or combined it with a 3 mm peritumoral area (AUC: 0.852 vs. 0.835 vs. 0.760)[47].
Beyond MRI, enhanced CT has also been frequently used to construct models predicting postoperative recurrence. Studies by Song et al. utilizing CT-constructed radiomics prediction models for early recurrence optimized the model’s predictive capacity by integrating clinical features. Song et al.’s combined prediction model, incorporating 15 radiomics features and three clinical features (CA19-9 > 1,000 U/ml, vascular invasion, and tumor margin), achieved an AUC of 0.974, significantly outperforming standalone RMs (AUC = 0.877), clinical feature models (AUC = 0.733), and the AJCC 8th TNM staging system (AUC = 0.717)[66]. The studies by Bo et al. and Xu et al. also demonstrated that a combined model integrating radiomics and clinical features surpassed individual models (AUC: 0.873 vs. 0.872 vs. 0.685, 0.85 vs. 0.82 and 0.75)[46,68]. Thus, selecting and combining different models appropriately can potentially enhance the new model’s predictive capacity. Yang et al. developed three predictive models, composed of clinical factors (CA19-9), pathological factors (MVI, tumor differentiation grade), imaging features, and a combination of these factors[69]. The composite model, integrating clinical factors (CA19-9), pathological factors (MVI, tumor differentiation grade), and imaging features, achieved the best predictive capacity (AUC = 0.876 vs. 0.823 vs. 0.697). In another study, Zhao et al. established three sets of models for prediction, similarly to Yang, but incorporated postoperative immunohistochemical staining of tissue specimens for epidermal growth factor receptor (EGFR), vascular endothelial growth factor receptor (VEGFR), P53, and Ki67 into the pathological factors[65]. The results, akin to Yang’s et al. study[69], showcased the superiority of the composite model, which, compared to standalone radiomics and CRP models, demonstrated enhanced ER predictive performance with an AUC, sensitivity, and specificity of 0.949, 0.875, and 0.774, respectively. Observing the outcomes of these studies reveals that MRI and CT can effectively predict postoperative recurrence, and appropriately adding factors like clinical features, imaging features, postoperative pathology results, and immunological factors can significantly improve the predictive ability of the model. Studies are listed in Table 4.
Predicting early recurrence post-surgery
Author | Year | Image modality | Number of cases | Number of features | Feature selection & modeling method | Model performance (AUC, sensitivity, specificity) |
Song et al.[66] | 2023 | CT | Training: 160 Validation: 36 External validation1: 74 External validation2: 61 | 15 | mRMR; GBM | Radiomics model AUC = 0.877, Clinical model AUC = 0.733, Combination clinical-radiomics model AUC = 0.974 |
Bo et al.[68] | 2023 | CT | Training: 90 Validation: 37 | 10 | LR; SVM; Neural network; RF; XGBoost; LightGBM; Bayes | LR model AUC = 0.87, SVM model AUC = 0.89, Neural network model AUC = 0.89, RF model AUC = 0.89, XGBoost model AUC = 0.85, LightGBM model AUC = 0.84, Bayes model AUC = 0.88, Clinical model AUC = 0.685 |
Liang et al.[67] | 2018 | CT | Training: 139 Validation: 70 | 9 | LASSO; LR | Radiomics model AUC = 0.82, Radiomics + Clinical stage model AUC = 0.90 |
Xu et al.[47] | 2021 | CT | Training: 159 Validation: 50 | 2268 | mRMR; Bootstrap | RS (IA) AUC = 0.778, RS (IA and 3 mm PA) AUC = 0.793, RS (IA and 5 mm PA) AUC = 0.804, CFM AUC = 0.788, CM (IA) AUC = 0.829, CM (IA and 3 mm PA) AUC = 0.819, CM (IA and 5 mm PA) AUC = 0.825 |
Yang et al.[69] | 2022 | CT | Training: 87 Validation: 37 | - | mRMR; RF; LR | Clinical model AUC = 0.697, Radiomics model AUC = 0.823, Clinical + Radiomics model AUC = 0.876 |
Chen et al.[62] | 2023 | CT | 136 | - | LR; RF; Neural network; Bayes; SVM; XGBoost | LR AUC = 0.7594, RF AUC = 0.8914, Neural network AUC = 0.7386, Bayes AUC = 0.6818, SVM AUC = 0.7396, XGBoost AUC = 0.8026 |
Zhu et al.[63] | 2021 | MRI | Training: 125 Validation: 87 | - | LASSO; LR | Preoperative model AUC = 0.844, Pathological model AUC = 0.741, Combination model AUC = 0.917 |
Zhao et al.[65] | 2019 | CT | 47 | - | Hypothetical test; LR | Radiomics model AUC = 0.889, Clinical model AUC = 0.798, Combined clinical-radiomics model AUC = 0.949 |
OS
The most commonly used staging system for ICC currently is the TNM staging, based on the tumor, lymph nodes, and metastasis. Due to tumor heterogeneity, the prognosis of ICC patients varies significantly among individuals, making the prediction accuracy somewhat limited[70,71]. In clinical practice and future clinical trials, effective prognostic prediction tools, such as radiomics-based predictive models and nomograms, have been proposed[35,61,72-74]. This represents a pressing need for formulating treatment strategies.
Deng et al. conducted a radiomics analysis of venous and arterial phase CT images of 82 ICC patients, combining radiomics features with clinical factors to establish a composite model[75]. This model included the psoas muscle index, radiomics score, intrahepatic bile duct stones, carcinoembryonic antigen, and the neutrophil-to-lymphocyte ratio as five indicators. The results showed a C-index of 0.768, with the AUC for predicting 1-year and 3-year OS rates being 0.809 and 0.886, respectively, significantly higher than other models. Park et al. aimed to develop and validate a preoperative model that could predict postoperative outcomes[76]. They constructed three different models using clinical, radiological, and radiomics features, with the focus on predicting recurrence-free survival. The clinical-radiological-radiomics model showed the best performance, with a training group C-index of 0.75 (0.72-0.79). This assists in assessing the postoperative outcomes of ICC patients with tumor formations preoperatively, allowing for the selection of the best treatment plan at the initial decision-making stage.
Fiz et al. extracted radiomics features from 18F-FDG PET/CT images and, along with clinical data, established clinical models, clinical plus intratumoral RMs, and clinical plus intratumoral and peritumoral (5 mm) RMs to predict tumor grading, MVI, OS, and progression-free survival (PFS)[35]. The results indicated that adding peritumoral radiomics features could optimize predictions for ICC tumor grading and survival rates (AUC = 0.834 vs. 0.783 vs. 0.718), but did not improve MVI predictions (AUC = 0.881 vs. 0.871 vs. 0.773). Thus, ICC radiomics based on PET can also predict pathological data, allowing for a reliable preoperative prognostic assessment. Tang et al. conducted a radiomics analysis of CT images and used the LASSO method to select three imaging features[77]. Multivariate Cox analysis identified three independent prognostic factors: cirrhosis, CA19-9 levels ≥ 35 U/mL, and tumor size > 5 cm. A nomogram combining imaging features and clinical factors was constructed. The radiomics nomogram demonstrated significant prognostic value for OS. Tang et al.’s study revealed notable differences in 1-year and 3-year survival rates between high-risk and low-risk patients: 30.4% vs. 56.4% and 13.0% vs. 30.6%, respectively (P = 0.018). Additionally, ultrasound could also be utilized to construct models[77]. Li et al. were the first to extract radiomics features from baseline ultrasound (US) and contrast-enhanced ultrasound (CEUS) images (four sequences) to build a preoperative model predicting OS in patients with ICC[73]. The nomogram, including CA 19-9, gender, ascites, radiomics features, and radiological characteristics, achieved a higher C-index than the 8th TNM staging system. In another study, Yang et al. used an MRI-based radiomics model to predict preoperative survival outcomes[78]. Integrating radiomics features into the TNM staging system significantly improved prognostic accuracy (validation set C-index 0.745 vs. 0.649, P = 0.039, NRI improved by 39.9%-43.8%, IDI improved by 16.1%-19.4%). In summary, as a method of extracting quantitative and high-dimensional features from medical imaging data, radiomics can accurately describe tumor biological characteristics, predicting the prognosis of ICC. Studies are listed in Table 5.
Survival data: overall survival
Author | Years | Image | Number of cases | Feature selection classifier | C-index |
Silva et al.[79] | 2021 | CT | 78 | PCA | 0.81 |
Tang et al.[72] | 2021 | CT | 101 | LASSO | 0.781 |
Park et al.[76] | 2021 | CT | 345 | LASSO | 0.75 |
Deng et al.[75] | 2021 | CT | 82 | Cox + AIC | 0.768 |
Li et al.[73] | 2022 | US | 170 | LASSO | 0.72 |
Fiz et al.[35] | 2022 | PET/CT | 74 | Correlation/PCA | 0.8 |
Yang et al.[78] | 2022 | MRI | 163 | LASSO | 0.75 |
FUTURE DIRECTIONS OF RADIOMICS IN ICC
Beyond the applications discussed, the use of radiomics in ICC patients has many potential avenues for expansion, such as predicting pathological features typically diagnosed postoperatively, and even certain genotypes[80-87]. In 2017, Rios Velazquez et al. confirmed the association between imaging phenotypes captured using radiological features and EGFR-mutated tumors in four independent lung adenocarcinoma cohorts[88]. This association may have clinical implications for selecting patients for targeted therapy. The correlation between imaging phenotypes and other molecular subtypes of NSCLC is also being further investigated in prospective genotyping analysis cohorts[89].
Qian et al., for example, utilized a nomogram combining features extracted from MRI images with clinical factors to predict Ki-67 expression, achieving promising predictive capability in the test cohort (AUC = 0.815)[80]. Members of the immune checkpoint pathway, such as Programmed Death Protein 1 (PD-1) and its ligand PD-L1, have garnered increasing attention in recent years[90-92]. Currently, immunohistochemistry staining on needle biopsies is a common method to assess tumor PD-1/PD-L1 expression. However, the heterogeneous expression of these markers often confuses results, limiting the clinical utility of measuring PD-1/PD-L1 expression. Molecular imaging can uncover the tumor microenvironment (TME) and allow for real-time visualization of target molecule and cell expression using specific radioactive isotopes or optical probes. Zhang et al., based on MRI, established a predictive model for PD-1/PD-L1 expression, employing Radscores (arterial phase), clinical-radiological factors, and clinical factors both individually and combined[93]. The models predicting PD-1 and PD-L1 expression achieved the highest area under the curve of 0.897 and 0.890, respectively. MRI radiomics can potentially act as a non-invasive biomarker to evaluate PD-1/PD-L1 expression and predict ICC patient prognosis. The research conducted by Zhang et al. has also yielded similar results[93].
Apart from PD-1 and PD-L1, various ICIs[94], such as Cytotoxic T-lymphocyte antigen 4 ( CTLA4)[95,96], EGFR[87,97], and anaplastic lymphoma kinase (ALK)[98], which have not yet been explored in ICC, have potential research value. He et al. constructed a radiomics model predicting CTLA4 expression levels using seven radiomics features, with AUCs of 0.769 for the training set and 0.724 for the validation set[95].
To improve the survival rate of ICC patients, a combination of local and systemic treatment strategies is usually employed. Multiple treatments may include resection, thermal ablation, radiation therapy, transarterial embolization, and systemic therapy[99-101]. The timing and intent of systemic therapy depend on the tumor stage and the pathological condition of the specimen. Systemic therapy is often aimed at palliative care. However, systemic therapy or chemoradiotherapy can also serve as neoadjuvant therapy to shrink the tumor size, allowing for local treatment. Considering the potential adverse reactions from the treatment, patient selection and monitoring are crucial for optimal treatment outcomes.
New prognostic variables have led to the development of predictive models for treatment response. Over the past decade, studies using advanced analytical techniques like radiomics have significantly expanded and achieved promising predictive results in various cancers[102-106]. However, studies on using radiomics to predict treatment outcomes in ICC are relatively few. In 2020, Mosconi et al. investigated the link between structural features on pre-transarterial radioembolization (pre-TARE) CT and objective response (OR), PFS, and OS[107]. The findings showed that post-TARE, there was higher iodine uptake in the arterial phase (higher mean histogram value, P < 0.001) and a more uniform distribution (low kurtosis, P = 0.043; GLCM contrast, P = 0.004; GLCM variance, P = 0.005; GLCM homogeneity, P = 0.005; and GLCM correlation, P = 0.030). Good radiomic features were found in 15 out of the 55 patients. In 2023, Ballı et al.[108] conducted a similar TARE-based study and found that a radiomics model established based on pre-treatment MRI could accurately predict the radiographic response to Yttrium-90 TARE in ICC patients. Combining radiomics with clinical features can enhance the predictive power of the model.
In other cancers, radiomics has been widely used to predict treatment response[109,110], especially for predicting responses to targeted therapy combined with immunotherapy[111,112]. However, current articles on using radiomics to predict treatment response in ICC are few and all are related to TARE, indicating significant potential in this field for the future.
When assessing ICC staging and resectability with current imaging methods, some cases are discovered to be unresectable intraoperatively, referred to as futile resections. The primary cause of futile resections is the discrepancy between preoperative assessments and intraoperative findings, including peritoneal metastasis, intrahepatic multifocal metastasis, and extensive tumor infiltration. Chu et al. used enhanced CT imaging to extract radiomics features and, using postoperative pathology as the gold standard, established clinical models, RMs, and combined models to predict futile resection in ICC patients[113]. The results showed that the radiomics model and the combined model predicted ICC futile resection with similar efficacy, both outperforming the clinical model, with AUCs of 0.838, 0.864, and 0.716 for the training group and 0.804, 0.800, and 0.590 for the validation group, respectively.
Additionally, Cai et al. utilized retrospective data from breast cancer (BC) patients undergoing ultrasound and tomography synthesis, developing a SVM algorithm to predict pCR status (ypT0 and ypN0) using preprocessed ultrasound and tumor synthesis radiomics features along with patient and tumor variables, achieving good results (AUC 0.72-0.81; P = 0.007)[114]. Han et al. also demonstrated superior diagnostic performance using radiomics methods in predicting preoperative multiplanar reconstruction (MPR) to neoadjuvant chemotherapy in non-small cell lung cancer[115]. Predicting the effectiveness of systemic chemotherapy in patients using radiomics has not yet been applied in ICC patients but may become a potential research focus in the future.
In ICC, Immune cells are vital components of the tumor immune microenvironment[116,117], with a high density of immune cells associated with improved survival rates and considered to exert local antitumor activity[118]. Currently, there is no non-invasive method to assess the quantity and distribution of immune cells in ICC. However, advancements have been made in some cancers using radiomics technology[119-122]. Jeon et al. extracted radiomics features from four phases of dynamic contrast-enhanced MRI and combined features from all four phases to build RMs for predicting CD8+ T cell infiltration and its spatial structure represented by immune phenotype[123]. The model showed high performance in both training (AUC = 0.973) and validation cohorts (AUC = 0.985), accurately predicting the BC immune phenotype based on CD8+ T cell spatial distribution. This method could be used for non-invasive stratification of patients based on the state of the tumor immune microenvironment. Similar to Jeon et al.’s study[123], Jiang et al. focused on generating an image-driven biomarker (Rad_score) to predict tumor-infiltrating regulatory T lymphocytes (Treg) in BC[124]. Jiang et al. extracted 108 radiological features from MRI images, four of which were used for model construction. The SVM model’s area under the curve (AUCs) for the training and validation sets were 0.744 (95%CI: 0.622-0.867) and 0.733 (95%CI: 0.535-0.931), respectively[124]. Sun et al. developed and validated two CT imaging biomarkers [lymph radiomics score (LRS) and marrow radiomics score (MRS)] to assess the immunohistochemistry (IHC)-derived lymphatic and marrow immune environments[119]. The non-invasive imaging biomarkers accurately assessed the immune environment and provided information for gastric cancer prognosis and immunotherapy.
Currently, most predictive nomograms based on radiomics are formed by combining radiomics features with clinical factors or by combining features from different imaging sequences[22,125]. Feng et al., aiming to forecast the pathological complete response to neoadjuvant chemoradiotherapy in locally advanced rectal cancer, established a radiopathomics model[126]. The Radiology Pathology Integrated Prediction System (aripes) was built on three feature sets related to pathological complete response: radiomics MRI features, pathomics nuclear features, and pathomics microenvironment features. The prediction system showed AUCs of 0.868 (95%CI: 0.825-0.912) for the training cohort, 0.860 (0.860-0.828-0.892) for validation cohort 1, and 0.872 (0.810-0.934) for validation cohort 2. Thus, aripes integrates radiomics MRI features, pathomics nuclear features, and pathomics microenvironment features, which could also be applied in ICC in the future, such as predicting the effectiveness of chemotherapy, postoperative recurrence, etc.
Beyond predicting responses to chemotherapy, radiomics is increasingly applied to the comprehensive evaluation of the TME and cancer prognosis. The TME constitutes a highly dynamic and spatially heterogeneous multicellular ecosystem, encompassing diverse cell types and molecular components. It plays a pivotal role in modulating tumor characteristics, including its plasticity, invasiveness, and metastatic potential. Radiomics can elucidate the relationships between TME features and imaging characteristics, thereby facilitating predictions of tumor progression. For instance, Yu et al. demonstrated the efficacy of MRI radiomics in predicting preoperative axillary LNM, identifying correlations between radiomic features and TME characteristics, such as immune cell populations, long-chain non-coding RNAs, and methylation sites, thus uncovering potential biological insights[127].
Nevertheless, radiomics has inherent limitations. The highly dynamic and heterogeneous nature of the TME poses challenges for single-modal imaging approaches in assessing the TME and predicting cancer prognosis. The development of multiomics methodologies, including radiogenomics, radiotranscriptomics, and radiopathomics, represents a significant advancement, offering novel strategies for evaluating the TME and cancer prognosis. Future research is poised to integrate transcriptomics, proteomics, metabolomics, and other multiomics data to achieve a more comprehensive understanding of the TME’s complexity and heterogeneity, thus enhancing prognostic accuracy and clinical utility.
DISCUSSION
Recent studies have demonstrated that while radiomics analysis has significant potential in various tumor applications, variability in feature extraction and lack of reproducibility remain major limitations[128,129]. Future radiomics research can benefit from standardizing imaging protocols related to dose administration, consistent acquisition parameters, and using reconstruction kernels with lower noise levels[130].
As radiomics progresses, an increasing number of studies have demonstrated its broad application prospects in the early diagnosis, treatment, prognostication, and recurrence assessment of ICC. However, as an emerging technology, the application of radiomics to ICC still faces challenges and limitations: (1) Many current studies are conducted at single centers with limited sample sizes and lack prospective research. Small sample sizes can cause model overfitting and inadequate validation sets when numerous radiomic features are extracted, increasing the risk of selection bias. Future studies require larger-scale, multicenter prospective research; (2) RMs generally perform better than clinical models, but combining radiomic models with clinical indicators to build models will yield reliable results; (3) Tumor image segmentation methods can be manual, semi-automatic, or automatic. However, current studies predominantly rely on manual segmentation, which is labor-intensive and subject to subjective bias, awaiting the emergence of various automatic segmentation methods; (4) Beyond the method of delineation, the outlines are not confined to the tumor itself but can also encompass subregions, such as the peritumoral area, which contains valuable information; (5) ICC can be categorized into three distinct morphological subtypes: mass-forming, periductal infiltrating, and intraductal growth. Among these, the mass-forming subtype is the most prevalent. However, the application of radiomics techniques to the study of the periductal infiltrating and intraductal growth subtypes remains challenging due to the complexities in capturing their imaging characteristics; (6) In the future, there should be active exploration of integrating radiomics with other omics, such as pathomics or genomics, to form an omics-integrated predictive system, thereby enhancing predictive capabilities.
Although research in ICC radiomics has made some progress in various aspects, there is still a lack of study volume. Future research should incorporate multi-phase image features, histopathology, immunohistochemical markers, genomics, and metabolomics to advance the realization of personalized clinical treatment.
In addressing the challenges currently encountered by radiomics in early diagnosis, treatment, prognosis prediction, and recurrence assessment of tumors, we should take appropriate actions. Accurate identification and segmentation are essential for selectively collecting radiomic features of tumors. Manual and semi-automatic segmentation techniques are labor-intensive and exhibit high inter- and intra-regional variability due to factors such as shape, size, poor contrast with adjacent organs, and surrounding structures[131]. Studies have demonstrated that automated segmentation methods in pelvic organs, including feature detection, edge or intensity-based methods, clustering techniques, shape and/or location priors, thresholding, and deformable models, enhance the reproducibility of radiomic studies[132]. Robust machine learning techniques for training reliable models have become an essential part of radiomics. These methods can learn from data, thereby automating and improving the prediction process, which enhances the performance of radiomics-based predictive models. Parmar et al. evaluated the predictive performance and stability of 14 feature selection methods and 12 classification methods for NSCLC patients, demonstrating the importance of selecting appropriate machine learning methods for each tumor type[133]. However, too many features may contain redundant and irrelevant information, leading to overfitting. The number of features can be reduced before being used as input for machine learning training. This can be done by performing repeat analysis on patients to identify the most reliable/reproducible features and assess redundancy[134]. Finally, before being applied in clinical settings, the stability and reproducibility of prognostic/predictive models should be evaluated[135]. Many studies have presented findings based on relatively small datasets, and internal validation might not be adequate to predict performance on external datasets. Therefore, it is necessary to conduct such external validation in large, multicenter environments before implementing these predictive models in clinical practice[136].
DECLARATIONS
Authors’ contributions
Concept and design of the review: Yu D
Literature collection, data analysis, and drafting the manuscript: Xu J
Revising the manuscript: Li B
Read and approved the final version of the manuscript: Yu D, Xu J, Li B
Availability of data and materials
Not applicable.
Financial support and sponsorship
This work was supported by grants from the National Natural Science Foundation of China (No. 82372834, 82173129, and 82002509).
Conflicts of interest
All authors declared that there are no conflicts of interest.
Ethical approval and consent to participate
Not applicable.
Consent for publication
Not applicable.
Copyright
© The Author(s) 2024.
REFERENCES
1. Lambin P, Rios-Velazquez E, Leijenaar R, et al. Radiomics: extracting more information from medical images using advanced feature analysis. Eur J Cancer 2012;48:441-6.
2. McKinney SM, Sieniek M, Godbole V, et al. International evaluation of an AI system for breast cancer screening. Nature 2020;577:89-94.
3. Bejnordi B, Veta M, Johannes van Diest P, et al; the CAMELYON16 Consortium. Diagnostic assessment of deep learning algorithms for detection of lymph node metastases in women with breast cancer. JAMA 2017;318:2199-210.
4. Sung H, Ferlay J, Siegel RL, et al. Global cancer statistics 2020: GLOBOCAN estimates of incidence and mortality worldwide for 36 cancers in 185 countries. CA Cancer J Clin 2021;71:209-49.
5. Choi JH, Thung SN. Recent advances in pathology of intrahepatic cholangiocarcinoma. Cancers (Basel) 2024;16:1537.
6. Sirica AE, Gores GJ, Groopman JD, et al. Intrahepatic cholangiocarcinoma: continuing challenges and translational advances. Hepatology 2019;69:1803-15.
7. Ilyas SI, Gores GJ. Pathogenesis, diagnosis, and management of cholangiocarcinoma. Gastroenterology 2013;145:1215-29.
8. Krenzien F, Nevermann N, Krombholz A, et al. Treatment of intrahepatic cholangiocarcinoma-a multidisciplinary approach. Cancers (Basel) 2022;14:362.
9. Wang K, Zhang H, Xia Y, Liu J, Shen F. Surgical options for intrahepatic cholangiocarcinoma. Hepatobiliary Surg Nutr 2017;6:79-90.
10. Capuozzo M, Santorsola M, Landi L, et al. Evolution of treatment in advanced cholangiocarcinoma: old and new towards precision oncology. Int J Mol Sci 2022;23:15124.
11. Shen X, Zhao H, Jin X, et al. Development and validation of a machine learning-based nomogram for prediction of intrahepatic cholangiocarcinoma in patients with intrahepatic lithiasis. Hepatobiliary Surg Nutr 2021;10:749-65.
12. Granata V, Fusco R, Belli A, et al. Conventional, functional and radiomics assessment for intrahepatic cholangiocarcinoma. Infect Agent Cancer 2022;17:13.
13. Nwogu I, Corso JJ. Exploratory identification of image-based biomarkers for solid mass pulmonary tumors. Med Image Comput Comput Assist Interv 2008;11:612-9.
14. Segal E, Sirlin CB, Ooi C, et al. Decoding global gene expression programs in liver cancer by noninvasive imaging. Nat Biotechnol 2007;25:675-80.
15. Buch K, Li B, Qureshi MM, Kuno H, Anderson SW, Sakai O. Quantitative assessment of variation in CT parameters on texture features: pilot study using a nonanatomic phantom. AJNR Am J Neuroradiol 2017;38:981-5.
16. Qiu Q, Duan J, Duan Z, et al. Reproducibility and non-redundancy of radiomic features extracted from arterial phase CT scans in hepatocellular carcinoma patients: impact of tumor segmentation variability. Quant Imaging Med Surg 2019;9:453-64.
17. Lecler A, Duron L, Balvay D, et al. Combining multiple magnetic resonance imaging sequences provides independent reproducible radiomics features. Sci Rep 2019;9:2068.
18. Gillies RJ, Kinahan PE, Hricak H. Radiomics: images are more than pictures, they are data. Radiology 2016;278:563-77.
19. Just N. Improving tumour heterogeneity MRI assessment with histograms. Br J Cancer 2014;111:2205-13.
20. Park JE, Park SY, Kim HJ, Kim HS. Reproducibility and generalizability in radiomics modeling: possible strategies in radiologic and statistical perspectives. Korean J Radiol 2019;20:1124-37.
21. Saha A, Harowicz MR, Grimm LJ, et al. A machine learning approach to radiogenomics of breast cancer: a study of 922 subjects and 529 DCE-MRI features. Br J Cancer 2018;119:508-16.
22. Mayerhoefer ME, Materka A, Langs G, et al. Introduction to radiomics. J Nucl Med 2020;61:488-95.
23. Park JE, Kim HS. Radiomics as a quantitative imaging biomarker: practical considerations and the current standpoint in neuro-oncologic studies. Nucl Med Mol Imaging 2018;52:99-108.
24. Bridgewater J, Galle PR, Khan SA, et al. Guidelines for the diagnosis and management of intrahepatic cholangiocarcinoma. J Hepatol 2014;60:1268-89.
25. Xu X, Mao Y, Tang Y, et al. Classification of hepatocellular carcinoma and intrahepatic cholangiocarcinoma based on radiomic analysis. Comput Math Methods Med 2022;2022:5334095.
26. Xue B, Wu S, Zheng M, et al. Development and validation of a radiomic-based model for prediction of intrahepatic cholangiocarcinoma in patients with intrahepatic lithiasis complicated by imagologically diagnosed mass. Front Oncol 2020;10:598253.
27. Chen X, Chen Y, Chen H, et al. Machine learning based on gadoxetic acid-enhanced MRI for differentiating atypical intrahepatic mass-forming cholangiocarcinoma from poorly differentiated hepatocellular carcinoma. Abdom Radiol (NY) 2023;48:2525-36.
28. Liu X, Khalvati F, Namdar K, et al. Can machine learning radiomics provide pre-operative differentiation of combined hepatocellular cholangiocarcinoma from hepatocellular carcinoma and cholangiocarcinoma to inform optimal treatment planning? Eur Radiol 2021;31:244-55.
29. Deng X, Liao Z. A machine-learning model based on dynamic contrast-enhanced MRI for preoperative differentiation between hepatocellular carcinoma and combined hepatocellular-cholangiocarcinoma. Clin Radiol 2024;79:e817-25.
30. Liu N, Wu Y, Tao Y, et al. Differentiation of hepatocellular carcinoma from intrahepatic cholangiocarcinoma through MRI radiomics. Cancers (Basel) 2023;15:5373.
31. Mahmoudi S, Bernatz S, Ackermann J, et al. Computed tomography radiomics to differentiate intrahepatic cholangiocarcinoma and hepatocellular carcinoma. Clin Oncol (R Coll Radiol) 2023;35:e312-8.
32. Xu H, Zou X, Zhao Y, et al. Differentiation of intrahepatic cholangiocarcinoma and hepatic lymphoma based on radiomics and machine learning in contrast-enhanced computer tomography. Technol Cancer Res Treat 2021;20:15330338211039125.
33. Peng Y, Lin P, Wu L, et al. Ultrasound-based radiomics analysis for preoperatively predicting different histopathological subtypes of primary liver cancer. Front Oncol 2020;10:1646.
34. Xu Y, Ye F, Li L, et al. MRI-based radiomics nomogram for preoperatively differentiating intrahepatic mass-forming cholangiocarcinoma from resectable colorectal liver metastases. Acad Radiol 2023;30:2010-20.
35. Fiz F, Masci C, Costa G, et al. PET/CT-based radiomics of mass-forming intrahepatic cholangiocarcinoma improves prediction of pathology data and survival. Eur J Nucl Med Mol Imaging 2022;49:3387-400.
36. Wang S, Wang X, Yin X, Lv X, Cai J. Differentiating HCC from ICC and prediction of ICC grade based on MRI deep-radiomics: using lesions and their extended regions. Phys Med 2024;120:103322.
37. Huang JL, Sun Y, Wu ZH, et al. Differential diagnosis of hepatocellular carcinoma and intrahepatic cholangiocarcinoma based on spatial and channel attention mechanisms. J Cancer Res Clin Oncol 2023;149:10161-8.
38. Jiang C, Zhao L, Xin B, Ma G, Wang X, Song S. 18F-FDG PET/CT radiomic analysis for classifying and predicting microvascular invasion in hepatocellular carcinoma and intrahepatic cholangiocarcinoma. Quant Imaging Med Surg 2022;12:4135-50.
39. Zhou Y, Zhou G, Zhang J, Xu C, Zhu F, Xu P. DCE-MRI based radiomics nomogram for preoperatively differentiating combined hepatocellular-cholangiocarcinoma from mass-forming intrahepatic cholangiocarcinoma. Eur Radiol 2022;32:5004-15.
40. Xue B, Wu S, Zhang M, et al. A radiomic-based model of different contrast-enhanced CT phase for differentiate intrahepatic cholangiocarcinoma from inflammatory mass with hepatolithiasis. Abdom Radiol (NY) 2021;46:3835-44.
41. Ren S, Li Q, Liu S, et al. Clinical value of machine learning-based ultrasomics in preoperative differentiation between hepatocellular carcinoma and intrahepatic cholangiocarcinoma: a multicenter study. Front Oncol 2021;11:749137.
43. Songthamwat M, Chamadol N, Khuntikeo N, et al. Evaluating a preoperative protocol that includes magnetic resonance imaging for lymph node metastasis in the cholangiocarcinoma screening and care program (CASCAP) in Thailand. World J Surg Oncol 2017;15:176.
44. Malikowski T, Levy MJ, Gleeson FC, et al. Endoscopic ultrasound/fine needle aspiration is effective for lymph node staging in patients with cholangiocarcinoma. Hepatology 2020;72:940-8.
45. Zhang S, Huang S, He W, et al. Radiomics-based preoperative prediction of lymph node metastasis in intrahepatic cholangiocarcinoma using contrast-enhanced computed tomography. Ann Surg Oncol 2022;29:6786-99.
46. Xu Y, Li Z, Yang Y, et al. A CT-based radiomics approach to predict intra-tumoral tertiary lymphoid structures and recurrence of intrahepatic cholangiocarcinoma. Insights Imaging 2023;14:173.
47. Xu L, Wan Y, Luo C, et al. Integrating intratumoral and peritumoral features to predict tumor recurrence in intrahepatic cholangiocarcinoma. Phys Med Biol 2021;66:125001.
48. Ji GW, Zhu FP, Zhang YD, et al. A radiomics approach to predict lymph node metastasis and clinical outcome of intrahepatic cholangiocarcinoma. Eur Radiol 2019;29:3725-35.
49. Pan YJ, Wu SJ, Zeng Y, et al. Intra- and peri-tumoral radiomics based on dynamic contrast enhanced-MRI to identify lymph node metastasis and prognosis in intrahepatic cholangiocarcinoma. J Magn Reson Imaging 2024:Online ahead of print.
50. Xu L, Yang P, Liang W, et al. A radiomics approach based on support vector machine using MR images for preoperative lymph node status evaluation in intrahepatic cholangiocarcinoma. Theranostics 2019;9:5374-85.
51. Khan SA, Tavolari S, Brandi G. Cholangiocarcinoma: epidemiology and risk factors. Liver Int 2019;39 Suppl 1:19-31.
52. Chen Y, Liu H, Zhang J, et al. Prognostic value and predication model of microvascular invasion in patients with intrahepatic cholangiocarcinoma: a multicenter study from China. BMC Cancer 2021;21:1299.
53. Xiang F, Wei S, Liu X, Liang X, Yang L, Yan S. Radiomics analysis of contrast-enhanced CT for the preoperative prediction of microvascular invasion in mass-forming intrahepatic cholangiocarcinoma. Front Oncol 2021;11:774117.
54. Zhou Y, Zhou G, Zhang J, Xu C, Wang X, Xu P. Radiomics signature on dynamic contrast-enhanced MR images: a potential imaging biomarker for prediction of microvascular invasion in mass-forming intrahepatic cholangiocarcinoma. Eur Radiol 2021;31:6846-55.
55. Chen S, Zhu Y, Wan L, Zou S, Zhang H. Predicting the microvascular invasion and tumor grading of intrahepatic mass-forming cholangiocarcinoma based on magnetic resonance imaging radiomics and morphological features. Quant Imaging Med Surg 2023;13:8079-93.
56. Ma X, Qian X, Wang Q, et al. Radiomics nomogram based on optimal VOI of multi-sequence MRI for predicting microvascular invasion in intrahepatic cholangiocarcinoma. Radiol Med 2023;128:1296-309.
57. Qian X, Lu X, Ma X, et al. A multi-parametric radiomics nomogram for preoperative prediction of microvascular invasion status in intrahepatic cholangiocarcinoma. Front Oncol 2022;12:838701.
58. Fiz F, Rossi N, Langella S, et al. Radiomic analysis of intrahepatic cholangiocarcinoma: non-invasive prediction of pathology data: a multicenter study to develop a clinical-radiomic model. Cancers (Basel) 2023;15:4204.
59. Spolverato G, Kim Y, Alexandrescu S, et al. Management and outcomes of patients with recurrent intrahepatic cholangiocarcinoma following previous curative-intent surgical resection. Ann Surg Oncol 2016;23:235-43.
60. Zhang XF, Beal EW, Bagante F, et al. Early versus late recurrence of intrahepatic cholangiocarcinoma after resection with curative intent. Br J Surg 2018;105:848-56.
61. King MJ, Hectors S, Lee KM, et al. Outcomes assessment in intrahepatic cholangiocarcinoma using qualitative and quantitative imaging features. Cancer Imaging 2020;20:43.
62. Chen B, Mao Y, Li J, et al. Predicting very early recurrence in intrahepatic cholangiocarcinoma after curative hepatectomy using machine learning radiomics based on CECT: a multi-institutional study. Comput Biol Med 2023;167:107612.
63. Zhu Y, Mao Y, Chen J, et al. Radiomics-based model for predicting early recurrence of intrahepatic mass-forming cholangiocarcinoma after curative tumor resection. Sci Rep 2021;11:18347.
64. Qin H, Hu X, Zhang J, et al. Machine-learning radiomics to predict early recurrence in perihilar cholangiocarcinoma after curative resection. Liver Int 2021;41:837-50.
65. Zhao L, Ma X, Liang M, et al. Prediction for early recurrence of intrahepatic mass-forming cholangiocarcinoma: quantitative magnetic resonance imaging combined with prognostic immunohistochemical markers. Cancer Imaging 2019;19:49.
66. Song Y, Zhou G, Zhou Y, et al. Artificial intelligence CT radiomics to predict early recurrence of intrahepatic cholangiocarcinoma: a multicenter study. Hepatol Int 2023;17:1016-27.
67. Liang W, Xu L, Yang P, et al. Novel nomogram for preoperative prediction of early recurrence in intrahepatic cholangiocarcinoma. Front Oncol 2018;8:360.
68. Bo Z, Chen B, Yang Y, et al. Machine learning radiomics to predict the early recurrence of intrahepatic cholangiocarcinoma after curative resection: a multicentre cohort study. Eur J Nucl Med Mol Imaging 2023;50:2501-13.
69. Yang Y, Zou X, Zhou W, et al. DWI-based radiomic signature: potential role for individualized adjuvant chemotherapy in intrahepatic cholangiocarcinoma after partial hepatectomy. Insights Imaging 2022;13:37.
70. Mavros MN, Economopoulos KP, Alexiou VG, Pawlik TM. Treatment and prognosis for patients with intrahepatic cholangiocarcinoma: systematic review and meta-analysis. JAMA Surg 2014;149:565-74.
71. Lee AJ, Chun YS. Intrahepatic cholangiocarcinoma: the AJCC/UICC 8th edition updates. Chin Clin Oncol 2018;7:52.
72. Tang YY, Zhao YN, Zhang T, Chen ZY, Ma XL. Comprehensive radiomics nomogram for predicting survival of patients with combined hepatocellular carcinoma and cholangiocarcinoma. World J Gastroenterol 2021;27:7173-89.
73. Li MD, Lu XZ, Liu JF, et al. Preoperative survival prediction in intrahepatic cholangiocarcinoma using an ultrasound-based radiographic-radiomics signature. J Ultrasound Med 2022;41:1483-95.
74. Fiz F, Rossi N, Langella S, et al. Radiomics of intrahepatic cholangiocarcinoma and peritumoral tissue predicts postoperative survival: development of a CT-based clinical-radiomic model. Ann Surg Oncol 2024;31:5604-14.
75. Deng L, Chen B, Zhan C, et al. A novel clinical-radiomics model based on sarcopenia and radiomics for predicting the prognosis of intrahepatic cholangiocarcinoma after radical hepatectomy. Front Oncol 2021;11:744311.
76. Park HJ, Park B, Park SY, et al. Preoperative prediction of postsurgical outcomes in mass-forming intrahepatic cholangiocarcinoma based on clinical, radiologic, and radiomics features. Eur Radiol 2021;31:8638-48.
77. Tang Y, Zhang T, Zhou X, et al. The preoperative prognostic value of the radiomics nomogram based on CT combined with machine learning in patients with intrahepatic cholangiocarcinoma. World J Surg Oncol 2021;19:45.
78. Yang Y, Zou X, Zhou W, et al. Multiparametric MRI-based radiomic signature for preoperative evaluation of overall survival in intrahepatic cholangiocarcinoma after partial hepatectomy. J Magn Reson Imaging 2022;56:739-51.
79. Silva M, Maddalo M, Leoni E, et al. Integrated prognostication of intrahepatic cholangiocarcinoma by contrast-enhanced computed tomography: the adjunct yield of radiomics. Abdom Radiol (NY) 2021;46:4689-700.
80. Qian X, Zhou C, Wang F, et al. Development and validation of combined Ki67 status prediction model for intrahepatic cholangiocarcinoma based on clinicoradiological features and MRI radiomics. Radiol Med 2023;128:274-88.
81. Zhan PC, Lyu PJ, Li Z, et al. CT-based radiomics analysis for noninvasive prediction of perineural invasion of perihilar cholangiocarcinoma. Front Oncol 2022;12:900478.
82. Brunese MC, Fantozzi MR, Fusco R, et al. Update on the applications of radiomics in diagnosis, staging, and recurrence of intrahepatic cholangiocarcinoma. Diagnostics (Basel) 2023;13:1488.
83. Liu Z, Luo C, Chen X, et al. Noninvasive prediction of perineural invasion in intrahepatic cholangiocarcinoma by clinicoradiological features and computed tomography radiomics based on interpretable machine learning: a multicenter cohort study. Int J Surg 2024;110:1039-51.
84. Aherne EA, Pak LM, Goldman DA, et al. Intrahepatic cholangiocarcinoma: can imaging phenotypes predict survival and tumor genetics? Abdom Radiol (NY) 2018;43:2665-72.
85. Saini A, Breen I, Pershad Y, et al. Radiogenomics and radiomics in liver cancers. Diagnostics (Basel) 2018;9:4.
86. Jeong WK, Jamshidi N, Felker ER, Raman SS, Lu DS. Radiomics and radiogenomics of primary liver cancers. Clin Mol Hepatol 2019;25:21-9.
87. Rizzo S, Petrella F, Buscarino V, et al. CT radiogenomic characterization of EGFR, K-RAS, and ALK mutations in non-small cell lung cancer. Eur Radiol 2016;26:32-42.
88. Rios Velazquez E, Parmar C, Liu Y, et al. Somatic mutations drive distinct imaging phenotypes in lung cancer. Cancer Res 2017;77:3922-30.
89. Brastianos PK, Horowitz PM, Santagata S, et al. Genomic sequencing of meningiomas identifies oncogenic SMO and AKT1 mutations. Nat Genet 2013;45:285-9.
90. Kornepati AVR, Vadlamudi RK, Curiel TJ. Programmed death ligand 1 signals in cancer cells. Nat Rev Cancer 2022;22:174-89.
91. Daassi D, Mahoney KM, Freeman GJ. The importance of exosomal PDL1 in tumour immune evasion. Nat Rev Immunol 2020;20:209-15.
92. Dermani FK, Samadi P, Rahmani G, Kohlan AK, Najafi R. PD-1/PD-L1 immune checkpoint: potential target for cancer therapy. J Cell Physiol 2019;234:1313-25.
93. Zhang J, Wu Z, Zhang X, et al. Machine learning: an approach to preoperatively predict PD-1/PD-L1 expression and outcome in intrahepatic cholangiocarcinoma using MRI biomarkers. ESMO Open 2020;5:e000910.
94. Prelaj A, Miskovic V, Zanitti M, et al. Artificial intelligence for predictive biomarker discovery in immuno-oncology: a systematic review. Ann Oncol 2024;35:29-65.
95. He H, Jin Z, Dai J, Wang H, Sun J, Xu D. Computed tomography-based radiomics prediction of CTLA4 expression and prognosis in clear cell renal cell carcinoma. Cancer Med 2023;12:7627-38.
96. Ter Maat LS, van Duin IAJ, Elias SG, et al. CT radiomics compared to a clinical model for predicting checkpoint inhibitor treatment outcomes in patients with advanced melanoma. Eur J Cancer 2023;185:167-77.
97. Ge X, Gao J, Niu R, et al. New research progress on 18F-FDG PET/CT radiomics for EGFR mutation prediction in lung adenocarcinoma: a review. Front Oncol 2023;13:1242392.
98. Chang C, Sun X, Wang G, et al. A machine learning model based on PET/CT radiomics and clinical characteristics predicts ALK rearrangement status in lung adenocarcinoma. Front Oncol 2021;11:603882.
99. Kelley RK, Bridgewater J, Gores GJ, Zhu AX. Systemic therapies for intrahepatic cholangiocarcinoma. J Hepatol 2020;72:353-63.
100. Greten TF, Schwabe R, Bardeesy N, et al. Immunology and immunotherapy of cholangiocarcinoma. Nat Rev Gastroenterol Hepatol 2023;20:349-65.
101. Ilyas SI, Affo S, Goyal L, et al. Cholangiocarcinoma - novel biological insights and therapeutic strategies. Nat Rev Clin Oncol 2023;20:470-86.
102. Shin J, Seo N, Baek SE, et al. MRI radiomics model predicts pathologic complete response of rectal cancer following chemoradiotherapy. Radiology 2022;303:351-8.
103. Chetan MR, Gleeson FV. Radiomics in predicting treatment response in non-small-cell lung cancer: current status, challenges and future perspectives. Eur Radiol 2021;31:1049-58.
104. Liu C, Zhao W, Xie J, et al. Development and validation of a radiomics-based nomogram for predicting a major pathological response to neoadjuvant immunochemotherapy for patients with potentially resectable non-small cell lung cancer. Front Immunol 2023;14:1115291.
105. Wesdorp NJ, Hellingman T, Jansma EP, et al. Advanced analytics and artificial intelligence in gastrointestinal cancer: a systematic review of radiomics predicting response to treatment. Eur J Nucl Med Mol Imaging 2021;48:1785-94.
106. Huynh E, Coroller TP, Narayan V, et al. CT-based radiomic analysis of stereotactic body radiation therapy patients with lung cancer. Radiother Oncol 2016;120:258-66.
107. Mosconi C, Cucchetti A, Bruno A, et al. Radiomics of cholangiocarcinoma on pretreatment CT can identify patients who would best respond to radioembolisation. Eur Radiol 2020;30:4534-44.
108. Ballı HT, Pişkin FC, Püren Yücel S, Sözütok S, Özgül D, Aikimbaev K. Predictability of the radiological response to Yttrium-90 transarterial radioembolization by dynamic magnetic resonance imaging-based radiomics analysis in patients with intrahepatic cholangiocarcinoma. Diagn Interv Radiol 2024;30:193-9.
109. Mirza-Aghazadeh-Attari M, Srinivas T, Kamireddy A, Kim A, Weiss CR. Radiomics features extracted from pre- and postprocedural imaging in early prediction of treatment response in patients undergoing transarterial radioembolization of hepatic lesions: a systematic review, meta-analysis, and quality appraisal study. J Am Coll Radiol 2024;21:740-51.
110. Jiang M, Li CL, Luo XM, et al. Ultrasound-based deep learning radiomics in the assessment of pathological complete response to neoadjuvant chemotherapy in locally advanced breast cancer. Eur J Cancer 2021;147:95-105.
111. Xu B, Dong SY, Bai XL, et al. Tumor radiomic features on pretreatment MRI to predict response to lenvatinib plus an anti-PD-1 antibody in advanced hepatocellular carcinoma: a multicenter study. Liver Cancer 2023;12:262-76.
112. Chen M, Lu H, Copley SJ, et al. A novel radiogenomics biomarker for predicting treatment response and pneumotoxicity from programmed cell death protein or ligand-1 inhibition immunotherapy in NSCLC. J Thorac Oncol 2023;18:718-30.
113. Chu H, Liu Z, Liang W, et al. Radiomics using CT images for preoperative prediction of futile resection in intrahepatic cholangiocarcinoma. Eur Radiol 2021;31:2368-76.
114. Cai L, Sidey-Gibbons C, Nees J, et al. Can multi-modal radiomics using pretreatment ultrasound and tomosynthesis predict response to neoadjuvant systemic treatment in breast cancer? Eur Radiol 2024;34:2560-73.
115. Han X, Wang M, Zheng Y, et al. Delta-radiomics features for predicting the major pathological response to neoadjuvant chemoimmunotherapy in non-small cell lung cancer. Eur Radiol 2024;34:2716-26.
116. Xia L, Oyang L, Lin J, et al. The cancer metabolic reprogramming and immune response. Mol Cancer 2021;20:28.
117. Sivori S, Pende D, Quatrini L, et al. NK cells and ILCs in tumor immunotherapy. Mol Aspects Med 2021;80:100870.
118. Petitprez F, de Reyniès A, Keung EZ, et al. B cells are associated with survival and immunotherapy response in sarcoma. Nature 2020;577:556-60.
119. Sun Z, Zhang T, Ahmad MU, et al. Comprehensive assessment of immune context and immunotherapy response via noninvasive imaging in gastric cancer. J Clin Invest 2024;134:e175834.
120. Liao H, Zhang Z, Chen J, et al. Preoperative radiomic approach to evaluate tumor-infiltrating CD8+ T cells in hepatocellular carcinoma patients using contrast-enhanced computed tomography. Ann Surg Oncol 2019;26:4537-47.
121. Sun R, Limkin EJ, Vakalopoulou M, et al. A radiomics approach to assess tumour-infiltrating CD8 cells and response to anti-PD-1 or anti-PD-L1 immunotherapy: an imaging biomarker, retrospective multicohort study. Lancet Oncol 2018;19:1180-91.
122. Aoude LG, Wong BZY, Bonazzi VF, et al. Radiomics biomarkers correlate with CD8 expression and predict immune signatures in melanoma patients. Mol Cancer Res 2021;19:950-6.
123. Jeon SH, Kim SW, Na K, Seo M, Sohn YM, Lim YJ. Radiomic models based on magnetic resonance imaging predict the spatial distribution of CD8+ tumor-infiltrating lymphocytes in breast cancer. Front Immunol 2022;13:1080048.
124. Jiang W, Wu R, Yang T, Yu S, Xing W. Profiling regulatory T lymphocytes within the tumor microenvironment of breast cancer via radiomics. Cancer Med 2023;12:21861-72.
125. Lambin P, Leijenaar RTH, Deist TM, et al. Radiomics: the bridge between medical imaging and personalized medicine. Nat Rev Clin Oncol 2017;14:749-62.
126. Feng L, Liu Z, Li C, et al. Development and validation of a radiopathomics model to predict pathological complete response to neoadjuvant chemoradiotherapy in locally advanced rectal cancer: a multicentre observational study. Lancet Digit Health 2022;4:e8-e17.
127. Yu Y, He Z, Ouyang J, et al. Magnetic resonance imaging radiomics predicts preoperative axillary lymph node metastasis to support surgical decisions and is associated with tumor microenvironment in invasive breast cancer: a machine learning, multicenter study. EBioMedicine 2021;69:103460.
128. Hatt M, Le Rest CC, Tixier F, Badic B, Schick U, Visvikis D. Radiomics: data are also images. J Nucl Med 2019;60:38S-44S.
129. Zhang YP, Zhang XY, Cheng YT, et al. Artificial intelligence-driven radiomics study in cancer: the role of feature engineering and modeling. Mil Med Res 2023;10:22.
130. Neri E, Del Re M, Paiar F, et al. Radiomics and liquid biopsy in oncology: the holons of systems medicine. Insights Imaging 2018;9:915-24.
131. Torres HR, Queirós S, Morais P, Oliveira B, Fonseca JC, Vilaça JL. Kidney segmentation in ultrasound, magnetic resonance and computed tomography images: a systematic review. Comput Methods Programs Biomed 2018;157:49-67.
132. Lenchik L, Heacock L, Weaver AA, et al. Automated segmentation of tissues using CT and MRI: a systematic review. Acad Radiol 2019;26:1695-706.
133. Parmar C, Rios Velazquez E, Leijenaar R, et al. Robust radiomics feature quantification using semiautomatic volumetric segmentation. PLoS One 2014;9:e102107.
134. Ruf J, von Wedel F, Furth C, et al. Significance of a single-time-point somatostatin receptor SPECT/multiphase CT protocol in the diagnostic work-up of gastroenteropancreatic neuroendocrine neoplasms. J Nucl Med 2016;57:180-5.
135. Rizzo S, Botta F, Raimondi S, et al. Radiomics: the facts and the challenges of image analysis. Eur Radiol Exp 2018;2:36.
Cite This Article

How to Cite
Download Citation
Export Citation File:
Type of Import
Tips on Downloading Citation
Citation Manager File Format
Type of Import
Direct Import: When the Direct Import option is selected (the default state), a dialogue box will give you the option to Save or Open the downloaded citation data. Choosing Open will either launch your citation manager or give you a choice of applications with which to use the metadata. The Save option saves the file locally for later use.
Indirect Import: When the Indirect Import option is selected, the metadata is displayed and may be copied and pasted as needed.
About This Article
Copyright
Data & Comments
Data
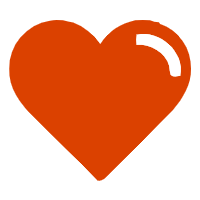
Comments
Comments must be written in English. Spam, offensive content, impersonation, and private information will not be permitted. If any comment is reported and identified as inappropriate content by OAE staff, the comment will be removed without notice. If you have any queries or need any help, please contact us at [email protected].