MASLD-mimicking microenvironment drives an aggressive phenotype and represses IDH2 expression in hepatocellular carcinoma
Abstract
Aim: Hepatocellular carcinoma (HCC) in patients with Metabolic dysfunction-associated steatotic liver disease (MASLD, formerly NAFLD) is expected to be a significant public health issue in the near future. Therefore, understanding the tumor microenvironment interactions in MASLD-induced HCC is crucial, and the development of relevant preclinical models is needed. Hence, we aimed to determine the effects of a MASLD-mimicking microenvironment (ME) on the aggressiveness of HCC cells and identify target genes that drive HCC by developing a 3D-in vitro co-culture system.
Methods: A 3D co-culture system mimicking the MASLD-ME was created with LX-2 liver stellate cells embedded in 3D collagen gel in the lower and SNU-449 HCC cells on the upper parts of Boyden chambers, and cells were grown in an optimized metabolic medium (MM). The effects of MASLD-ME on motility, sphere formation, proliferation, and cell cycle of SNU-449 cells were tested by Boyden chamber, 3D sphere formation, XTT, and Flow cytometry, respectively. The protein expression/activation profiles of motile SNU-449 cells that passed the membrane toward MASLD-ME or control condition were investigated using a multiplex protein profiling system DigiWest and confirmed with RT-PCR, WB, and Flow cytometry. IDH2 levels were examined in primary human HCC and adjacent liver tissues by IHC and in TCGA and CPTAC cohorts by bioinformatics tools.
Results: MM treatment increased fat accumulation, motility, and spheroid formation of both SNU-449 and LX-2 cells. MASLD-ME induced activation of LX2 cells, leading to the formation of bigger colonies with many intrusions compared to related controls. DigiWest analysis showed that metabolism-related proteins such as IDH2 were the most affected molecules in SNU-449 cells that migrated toward the MASLD-ME compared to those that migrated toward the control condition. Downregulation of IDH2 expression was confirmed in SNU-449 cells grown in MASLD-ME, in primary HCC tumor samples by IHC, and in HCC patient cohorts by bioinformatics analysis.
Conclusion: This study reports the potential involvement of MASLD-ME in the downregulation of IDH2 expression and promoted motility and colonization capacity of HCC cells. The 3D MASLD model presented in this study may be useful in investigating the mechanistic roles of MASLD-ME in HCC.
Keywords
INTRODUCTION
HCC is among the most common and deadly cancers worldwide. The prognosis remains dismal due to the difficulties in early diagnosis and the high level of recurrence, metastasis, and development of therapy resistance. HCC has an increasing incidence that is reflected by the rise in the incidence of NAFLD, especially in the Western World[1,2]. NAFLD includes a diverse range of pathologic conditions, covering hepatic steatosis and non-alcoholic steatohepatitis (NASH) that can evolve to fibrosis, cirrhosis, and HCC[3]. Currently, NAFLD affects nearly 25% of the global population, and NASH is the fastest-rising cause of HCC worldwide[4]. The term MASLD (Metabolic dysfunction-associated steatotic liver disease) has now replaced NAFLD[5,6].
MASLD is characterized by the presence of hepatic steatosis along with at least one cardiometabolic risk factor, such as elevated body mass index, disrupted glucose metabolism, high blood pressure, high triglyceride, and low high-density cholesterol (HDL-C) levels. The development and progression of MASLD involves multiple risk factors, including age, sex, ethnicity, hormonal status, genetic predisposition, epigenetic factors, and dietary habits[7-11]. Patients with MASLD generally have a hypercaloric and unhealthy diet characterized by high consumption of fat esterified with saturated and polyunsaturated free fatty acids (FFAs), cholesterol, fructose, and low antioxidant vitamins[12]. MASLD is defined as an increase in liver fat content with a threshold of more than 5% and regularly coexists with metabolic disorders such as insulin resistance, type 2 diabetes, obesity, dyslipidemia, hypertension, and cardiovascular disease[7,8]. When hepatic steatosis is observed on liver histology without a history of alcohol consumption,it can be classified as metabolic dysfunction-associated steatotic liver (MASL)[7,8]. However, when there is steatosis, hepatocyte ballooning and lobular inflammation, with or without perisinusoidal fibrosis, it is sub-categorized as metabolic dysfunction-associated steatohepatitis (MASH)[13]. Hepatic fat accumulation (e.g., steatosis) appears to be rather benign, whereas hepatocyte lipotoxicity results mainly from the accumulation of FFAs, free cholesterol, and other lipid metabolites[14,15]. These metabolic alterations in the liver exert additional stress on many organelles, especially the endoplasmic reticulum and mitochondria, trigger stress-induced responses, and generate reactive oxygen species (ROS). The induction of inflammatory signaling pathways by releasing pro-inflammatory cytokines by Kupffer cells or adipokines from adipocytes, deregulated hepatocyte apoptosis, and hepatic stellate cells (HSCs) activation drives the progress of macrovesicular steatosis to MASH (reviewed by Kamm and McCommis)[16]. This progression covers continuous liver injury that is associated with hepatocyte apoptosis, liver inflammation, fibrogenesis, and eventually cirrhosis with liver failure, and, finally, HCC[17]. In this scenario, HSCs seem to be the main cells responsible for fibrosis (reviewed by Kamm and McCommis)[16]. The accumulated evidence suggests that HSCs are induced by inflammation and are involved in the regulation of liver inflammation. Therefore, it is necessary to investigate the involvement of HSCs in MASLD from the viewpoint of inflammation and fibrosis. Tumors that arise in MASH appear to be larger and less amendable to curative therapies than those with other properties[18]. The cellular mechanisms and signaling cascade are still largely unknown, and no effective treatments are available. Thus, the proper understanding of the MASLD spectrum may help develop new approaches to managing MASLD-associated diseases, including HCC.
The development of experimental models accurately representing MASLD to study its underlying mechanism remains challenging. In this study, we aimed to design and develop a versatile in vitro
METHODS
Patient tissue specimens
A total of 26 resected tissue samples [paired with HCC tissue and adjacent non-tumor tissue (NT)] were analyzed for this study. In all 26 samples, 12 were with vascular invasion, while the other 14 were not. All specimens were histologically and clinically diagnosed at the Ege University Hospital Department of Pathology by FY, DN, and BS. Approval from the Institutional Research Ethics Committee was obtained with the number 19-3T/39. The histopathological analysis of all patients was analyzed by the WHO histopathological classification of liver and intrahepatic bile ducts.
Cell lines
The Human HCC cell line (SNU-449, HuH-7) and human hepatic stellate cell line (LX2) were gifted from Mehmet Öztürk and Zeynep Fırtına Karagonlar, respectively. THLE-2 cells were purchased from ATCC and cultured as described by the manufacturer. SNU-449 and HuH-7 cells were cultured in Dulbecco’s modified Eagle’s medium with 1 g/L glucose and L-Glutamine (DMEM, Invitrogen, Carlsbad, CA,11880028 USA) supplemented with 10% fetal bovine serum (FBS; Biological Industries), 1% penicillin/streptomycin (15140122, Invitrogen), and 1% MEM non-essential amino acid solution (11140035, Invitrogen.). LX-2 cells were cultured in Dulbecco’s modified Eagle’s medium with 4.5 g/L glucose and L-Glutamine (DMEM, Invitrogen, Carlsbad, CA, 41966029, USA) supplemented with 2% fetal bovine serum (FBS; Sigma-Aldrich, St Louis, MO, USA) and 1% penicillin/streptomycin. Cells were maintained at 37 °C in a humidified chamber with 5% CO2. THLE-2 cells were harvested in BEGM medium (CC-3170, Lonza) supplemented with 10% fetal bovine serum (FBS from Sigma-Aldrich), 1% Penicillin-Streptomycin (PS from Invitrogen), and 50 μg/mL gentamycin (Sigma-Aldrich) in PureCol/fibronectin-coated T-75 flasks in a humidified
Preparation and treatment of metabolic medium
Long-chain fatty acids (FA), palmitic acid (PA) (P0500, Sigma-Aldrich), and oleic acids (OA) (27728, Sigma Aldrich) were purchased. The mixture of PA and OA was prepared in ethanol (1.08543, Sigma-Aldrich). The stock solution was kept at + 4 °C, and the working solution was prepared just before the experiments. Fructose stock solution was prepared in water (M105323.0250, Merck). BSA stock solution (A1391, Applichem) was prepared in PBS. BSA is used to deliver FFA to cells. The final optimized Metabolic Medium (MM) contained free fatty acids (FFAs) dissolved in ethanol, fructose, and insulin (Roche, 11376497001). The carrier medium, which was control condition (control), contained ethanol, BSA, and PBS. Ethanol concentration did not exceed 0.05% in the cell culture medium, as suggested by
Establishment of 3D co-culture system
LX-2 cells were embedded in three-dimensional collagen I gel (354236, Corning) using 2% FBS high glucose complete DMEM using low-attached 24-well plates. The following day, cells were treated with either MM or control conditions. The culture medium was replaced with freshly prepared MM and control conditions every two days. On day 5, upper chambers were integrated into the system and loaded with SNU-449 cells. On day 8, SNU-449 cells that passed the membrane were collected and transferred to a new culture dish. Meanwhile, conditioned media were collected and used to treat SNU-449 cells that were transferred to new dishes. On day 8, RNA was obtained from LX-2 cells. When SNU-449 cells reached 70% confluency, RNA and protein isolations were performed.
3D colony formation assay in matrigel
SNU-449 cells and LX-2 cells were embedded in three-dimensional collagen I gel (354236, corning) as previously described[20]. SNU-449 cells were also embedded in 60% Growth Factor Reduced Matrigel (354230, Corning) prepared in DMEM. Cells were treated with control condition and a metabolic medium. Cultures were fixed with 4% paraformaldehyde after indicated periods. Images were captured at magnifications 20x and 40x using phase contrast objective using Olympus BX71. For quantification of the colony number, the entire area per experimental condition in each of the three independent cultures was photographed using 40x magnification.
Western blot
Total proteins were isolated and prepared by using a modified RIPA buffer as described previously[21]. Antibodies against IDH2 (sc-374476, Santa Cruz Biotechnology) and Calnexin (PA5-34754, Invitrogen) were used as described before[21]. Equal loading and transfer were confirmed by repeat probing for calnexin (house-keeping gene).
Nile red staining
Cells seeded on coverslips were grown for 24 h and treated with conditions as indicated in the figure legends for indicated periods. Cells were fixed with 4% paraformaldehyde solution, washed with PBS, and stained with Nile Red (19123, Sigma Aldrich). Images were taken using Olympus BX71.
Motility assay
In vitro motility assays were performed using Boyden chambers (353097, Falcon) as described previously[21]. Briefly, overnight starved SNU-449 cells with 2% DMEM were placed into upper chambers, and control condition or metabolic medium in DMEM was added to the lower chambers. After 24 h incubation at
Immunohistochemical staining
IDH2 (sc-374476) expression profiles were analyzed by using immunoperoxidase staining in the serial liver sections. Standard 5-mm tissue sections were taken on lysine-coated slides. Sections were deparaffinized and then rehydrated. Immunostaining was performed using an automated immunohistochemical stainer according to the manufacturer’s guidelines (IVIEW DAB, BenchMarkXP, and Ventana, USA). The antigen retrieval step was included in the automated program. Endogenous peroxidase activity was blocked using 3% H2O2 for 4 min at 37 °C. Endogenous avidin-biotin activity was blocked by using the avidin-biotin block solution (DAKO, Denmark), and then primary antibodies, anti-IDH2, were applied. The sections were stained with 3,3’-diaminobenzidine tetrahydrochloride (DAB), a chromogen stain (brown), and counterstained with hematoxylin.
IDH2 expression was defined as membranous and/or cytoplasmic when more than 10% of the hepatocytes stained positive for IDH2. The extent of staining was scored as one positive (10% to 25% of cells were positively stained), two positive (26% to 50% of cells were positively stained), and three positive (more than 50% of the cells were positively stained). The intensity of IDH2 immunostaining was semiquantitatively graded as follows: none (0), weak (+1), moderate (+2), and intense (+3).
RNA isolation
Total RNA from LX-2 and SNU-449 cells were purified using NucleoZOL reagent (Macherey-Nagel) following the manufacturer's instructions. RNAs were eluted in DNase/RNase-free ultrapure water, and their concentration was analyzed by NanoDrop and stored either at -80 °C or reverse transcribed in subsequent cDNA preparation steps.
Quantitative RT-PCR
Expression of mRNA levels of various targeted genes was quantified using specific primers designed specifically for genes of interest and SYBR green-based real-time quantitative PCR assay. Primers were designed with the NCBI Primer designing tool (https://www.ncbi.nlm.nih.gov /tools/primer-blast/), and their specificity was controlled by UCSC In-Silico PCR (https://genome.ucsc.edu/cgi-bin/hgPcr )[22]. The sequences of primers used for quantitative RT-PCR (RT-qPCR) studies are listed in Supplementary Table 1. Oligonucleotide primer pairs were synthesized by Oligomer (TR).
Briefly, total RNAs were transcribed into cDNA using RevertAid First Strand cDNA Synthesis Kit (Thermo Scientific™). qPCR was performed in 10 µL reaction volumes (5 µL Master Mix, 0.3 µL primers, 2 µL 5 ng/µL cDNA, 4.4 µL H2O) using Luminaris Color HiGreen High ROX qPCR Master Mix (Thermo Scientific™).
Real-time PCR amplification was performed with The Applied Biosystems 7500 Fast Real-Time PCR Systems. Cycling conditions were as follows: 2 min at 50 °C, 10 min at 95 °C, followed by 40 cycles of 15 s at 95 °C, 30 s at 60 °C, and 30 s at 72 °C.
Melting curve analysis was used to assure the specificity of primers. RPL41 was used as an internal control and for normalization. 2-ΔCt and 2-ΔΔCt method was used to indicate the relative expression level of corresponding genes. At least two biological replicates were performed for RT-qPCR experiments.
Flow cytometry
SNU-449 cells treated with control condition or metabolic medium for 72 h were harvested, fixed, and permeabilized in cold 70% ethanol for 2 h on ice. All steps of flow cytometric staining were performed in FACS buffer containing PBS with 1% BSA and 2 mM EDTA. Non-specific antibody binding was blocked by incubating the permeabilized cells in the FACS buffer for 30 min at room temperature. Subsequently, cells were stained with 50 µL/sample of 1:50 diluted mouse IDH2 Antibody (B-6) (Santa Cruz Biotechnology) for 30 min at 4 °C. Cells were washed in FACS buffer by centrifugation, resuspended in 50 µL/sample of Alexa Fluor 488-conjugated goat anti-mouse secondary antibody solution (1:500, A-11001, Invitrogen), and incubated for 30 min at 4 °C in the dark. Samples were acquired using the CytoFlex flow cytometer and analyzed by CytExpert software (Beckman Coulter).
Cell cycle analysis
Cell cycle analysis was performed using propidium iodide (PI) staining and flow cytometry. SNU-449 cells (1 × 105 cells/well) were seeded in 6-well tissue culture plates and incubated at 37 °C overnight, followed by treatment of control condition or metabolic medium for an additional 48 h. At the end of the incubation, cells were harvested, fixed in cold 70% ethanol for 2 h on ice, and stained with PI solution containing
Cell proliferation (XTT)
For cell proliferation assay, SNU-449 cells were seeded at 2,000 cells/well density in 96-well flat bottom tissue culture plates. The next day, cells were treated with either control condition or a metabolic medium and incubated at 37 °C, 5% CO2 for 24, 48, and 72 h. At indicated time points, 10 µL of Cell Proliferation Reagent WST-1 (Roche) was added into each well, and the plate was incubated at 37 °C for an additional
MTT
THLE-2 cells were plated on 96-well plates and harvested for 24, 48, and 72 h. MTT analysis was performed as described previously[23]. Measured absorbances were evaluated and plotted by Prism. Three biological replicates were done to perform statistical analysis.
Bioinformatics analysis and in silico validations
Analysis of IDH2 expression in TCGA and CPTAC datasets
Analysis of IDH2 expression in normal and tumor tissues was conducted with The Cancer Genome Atlas (TCGA) and Clinical Proteomic Tumor Analysis Consortium (CPTAC) liver hepatocellular carcinoma cohorts (LIHC) with The University of ALabama at Birmingham CANcer data analysis Portal (UALCAN)[24,25] that is an extensive, user-friendly web resource for accessing and analyzing open-access OMICS data in both mRNA and protein levels. In mRNA expression, values were represented as normalized TPM (transcript per million) values. In protein expression analysis, Z-values represented standard deviations from the median across samples for liver hepatocellular carcinoma. Protein expression analysis Z-values represent standard deviations from the median across samples for the cancer type. Log2 count ratios from CPTAC were first normalized within each sample profile and then normalized across samples.
Analysis of differentially expressed (DE) genes and clinical characterizations in Low-IDH2 vs. High-IDH2 tumors in TCGA
In this analysis, cBioPortal (https://www.cbioportal.org/)[26-28] is an open-access resource for interactive exploration of cancer datasets such as TCGA. Normalized (RSEM, batch normalized from Illumina HiSeq_RNASeqV2) mRNA expression data of HCC tissues in the TCGA-LIHC (https://gdc.cancer.gov/about-data/publications/pancanatlas)[29] dataset was grouped into quartiles according to the level of normalized IDH2 expression values. Differentially expressed (DE) gene lists between Low-IDH2 quartile (Q1) and High-IDH2 quartile (Q4) were obtained and visualized as a volcano graph. Pathway analyses of DE genes were performed using KEGG: Kyoto Encyclopedia of Genes and Genomes database (https://www.genome.jp/kegg/)[30]. Then, we analyzed the expression profile of IDH2 based on clinicopathological factors such as histological grade, aneuploidy, hypoxia, and fraction genome altered scores and survival status, and we drew Kaplan-Meier survival graphs and clinicopathological factors-related graphs.
Re-analyzing of open-access datasets
Expression studies related to NASH/NAFLD-based HCC were searched in the open-access datasets in Gene Expression Omnibus (GEO, www.ncbi.nlm.nih.gov/geo/)[31] and ArrayExpress (www.ebi.ac.uk/biostudies/arrayexpress)[32] by using the search terms “NASH” or “NAFLD” and “HCC”. The datasets generated, except human tissue samples, were excluded. The rest of the datasets were evaluated according to the presence of their NASH/NAFLD and HCC information. E-MEXP-3291[33] dataset (n: 9 NASH, 19 control samples) was normalized and analyzed using BRB-ArrayTools (v4.61 stable) developed by Dr. Richard Simon and the BRB-ArrayTools Development Team. It is an integrated package for the visualization and statistical analysis of Microarray gene expression, copy number, methylation, and RNA-Seq data. In the GSE164441[34] dataset (n: 10 NAFLD-based HCC, 10 control samples), the mean of normalized FPKM (Fragments Per Kilobase of transcript per Million mapped reads) values was used for NAFLD-based HCC and control comparison. These datasets were used for the in silico analysis of the genes of our interest, such as IDH2 in related datasets. [Terms are used as defined in the datasets (NASH and NAFLD)].
Statistical analysis
Most of the statistical analyses were performed using GraphPad Prism 8.0 software (San Diego, California USA, https://www.graphpad.com). The statistical difference among tested groups was analyzed using unpaired t-test, two-tailed t-test, Mann-Whitney test, Two-Way ANOVA (with Sidak’s post-test),
RESULTS
Designing and optimization of the composition of a metabolic medium
To mimic MASLD-ME, we used LX-2 cells to simulate a fibrotic microenvironment and used fructose, free fatty acids (OA and PA), insulin, and high glucose medium to reproduce bad diet habits. A variety of cell lines, including THLE-2 (primary hepatocyte), LX-2 (hepatic stellate cells), SNU-449, and HuH-7 (HCC cell lines), were used to optimize components and concentrations for the culture conditions to mimic hepatic steatosis. HuH-7 cells were exposed to different ratios and concentrations of OA and/or PA. A combination of the two free fatty acids at a ratio 2:1 ratio (OA: PA) was found to be the most effective in cytoplasmic lipid formation (data not shown). Simultaneously, we tested the maximum concentrations of FFA that can be conjugated with BSA [Supplementary Figure 1A and B]. BSA has 6-7 high-affinity binding sites for fatty acids and thus is an effective carrier serving to substantially increase the solubility of fatty acids in an aqueous solution[35]. Cells were treated overnight with different ratios of BSA: FFA combinations, and as shown by Nile red staining, with increasing concentrations of FFA and BSA, lipid accumulation in the HuH-7 cells increased substantially [Supplementary Figure 1A and B]. Moreover, 2.5% BSA was found to bind and deliver more FFA to cells than 1.25% BSA [Supplementary Figure 1A and B ]. We tested the degree of fat accumulation in the THLE-2 cell line with a similar approach. We observed that the accumulation of fat droplets within the cells was improved with the increasing concentration of FFA
Figure 1. Representative images obtained after treatment with MM. SNU-449 cells (A) and LX-2 (B) cells were analyzed with Nile Red to observe fat accumulation. Fat droplets stained in red. Bright Field (BF) microscopy images of control groups treated with control condition (AX, BX) and fluorescence micrographs (AY, BY). BF microscopy images of MM-treated groups (AW, BW) and fluorescence micrographs (AZ, BZ). Scale bar: 50 µM. MM: metabolic medium; BF: Bright Field.
Metabolic-medium activated LX-2 cells
When HSCs are activated, they transdifferentiate into α-smooth muscle actin (αSMA) positive myofibroblasts with star-like shapes, which produce an increased amount of extracellular matrix (ECM), profibrogenic cytokines, and result in liver fibrosis (reviewed by Kamm and McCommis)[16]. Thus, we investigated whether the MM that we optimized has the potential to activate LX-2 cells. We treated LX-2 cells with MM and observed that LX-2 cells adopted a fibroblast-like shape [Figure 2E] and induced αSMA expression upon MM treatment compared to cells treated with control condition [Figure 3A]. Importantly, LX-2 cells embedded in collagen gel formed bigger colonies with many protrusions under MM treatment compared to the control [Figure 3B]. Next, we assessed αSMA gene expression in these LX-2 colonies using RT-qPCR. As expected, αSMA expression was significantly higher in MM-treated LX-2 cells embedded in collagen gel, compared to the control counterparts [Figure 3C]. In conclusion, our results show that MM activated LX-2 cells growing both in 2D and 3D cultures.
Figure 3. MM induced LX-2 activation. αSMA expression analysis of the MM treated and control cells with WB (A) and RT-qPCR (C). Morphological analysis of the cell and colony formations in 3D-collagen culture (B) (Unpaired t-test. ***P < 0.001). Scale bar: 50 µM. αSMA: α-smooth muscle actin; MM: metabolic medium.
Metabolic medium promoted the acquisition of aggressive phenotypes in SNU-449
We next investigated whether treatment with MM promoted the tumorigenic behavior of SNU-499 cells. Firstly, we assessed the proliferation and cell cycle progression of the cells treated with control or MM using XTT assay and propidium iodide DNA staining, respectively. Our results showed that the proliferation of SNU-449 cells significantly increases upon 24 h of MM treatment, compared to the control [Figure 4A]. Interestingly, the proliferative effect of MM vanished at later time points [Figure 4A]. To reveal if MM affects the cell cycle progression of HCC cells, we treated cells either with the control or MM for 48 hours and determined the cell cycle distribution by staining the DNA of the fixed cells with propidium iodide. We observed that the percentage of SNU-499 cells in the G0/G1 phase was significantly diminished, while those in the S phase and G2/M phase were significantly increased after MM treatment [Figure 4B], suggesting that MM treatment retards the cell cycle in S - G2/M phases. Furthermore, there was no difference in the percentage of sub-G1 apoptotic cell fraction among the treatments confirming the non-cytotoxic behavior of MM [Figure 4B]. On the other hand, SNU-449 cells treated with MM exhibited promoted motility
Figure 4. Effects of MM treatment on cellular behaviors of SNU-449 cells. (A) Proliferation assay of SNU-449 cells treated with either control condition or MM for indicated time points. XTT proliferation assay was performed in quintuplicate and data were depicted as mean ± standard deviation; (B) Cell cycle analysis of SNU-449 cells treated with either control condition or metabolic medium (MM) for 48 h. (Top) Representative PI cell cycle histogram graphs of triplicate samples showing similar results. (Bottom) Percentage of cells in each cell cycle phase determined by PI intensity indicating the DNA content of SNU-449 cells at 48 h post control or MM treatment; (C-F) Motility (C and D) and spheroid formation (E and F) analysis of MM and control SNU-449 cells. The significance of differences between groups in (A) and (B) was analyzed by Two-way ANOVA test and in (D) and (F) by unpaired t-test. (ns: non-significant,
Developing an in vitro co-culture system to investigate the association between MASLD-related liver microenvironment and metastasis
To investigate the molecular mechanism of the MASLD on the metastatic behaviors, we developed a 3D
Figure 5. Schematic representation of the novel-designed 3D co-culture system and our experimental design (A) Created with BioRender.com. Expression analysis of inflammation- and fibrogenesis-related markers CXCR4 (B) IL1B (C) FAS (D) and TGFB1 (E) upon MM treatment compared to the control group (Unpaired t-test, * P < 0.05, **P < 0.01, ***P < 0.001). CXCR4: C-X- Motif Chemokine Receptor 4; IL1B: Interleukin 1 Beta; FAS: fas Cell Surface Dearth Receptor; TGFB1: Transforming Growth Factor Beta 1.
Novel-designed 3D co-culture system mimicked the pro-inflammatory and pro-fibrotic MASLD microenvironment
To evaluate the effects of MASLD-associated factors and the crosstalk between co-cultured cells in our 3D MASLD-ME, we performed RT-qPCR analysis using LX-2 cells. We assessed the expression levels of genes known to have a role in inflammation and fibrogenesis. CXCR4 receptor and interleukin 1beta (IL1B) gene expression was significantly upregulated upon MM treatment compared to the control group
SNU-449 cells that preferentially migrated to MASLD-mimicking microenvironment had diminished IDH2 expression
DigiWest protein profiling analysis was performed to determine the protein expression/activation profiles of motile SNU-449 cells that passed the membrane toward MASLD-mimicking microenvironment in the experimental system shown in Figure 5A. Briefly, proteins from SNU-449 cells that migrated toward (motile) the MM or control were purified and sent to NMITT Pharmaservice for DigiWest analysis. The DigiWest approach is based on transferring the western blot to a bead-based microarray platform and extending traditional western blotting to reach proteomic scales[37]. Using this service, we were able to dissect cellular signaling pathways and have hundreds of specific proteins and protein modification information in our system[37]. DigiWest results and subsequent bioinformatics analyses showed that, as expected, the expression of metabolism-regulating proteins is mainly altered in SNU-449 cells upon MM induction. Among them, we identified IDH2 for further analysis.
We performed WB analysis using the same protein samples that were sent to DigiWest analysis and observed that IDH2 expression decreased in the group treated with MM [Figure 6A]. Similarly, IDH2 gene expression and intracellular protein levels were found to be diminished in MM-treated SNU-449 cells compared to control counterparts assessed by RT-qPCR [Figure 6B] and Flow Cytometry [Figure 6C]. As IDH2 expression decreased in the MM group in SNU-449 cells, protein expression decreased in HuH-7 cells [Figure 6D]. Densitometric analysis (IDH2/Calnexin expression) revealed that the protein expression of both SNU-449 and HuH-7 nearly decreased in half in MM condition [Figure 6A and D].
Figure 6. Expression analysis of IDH2 in SNU-449 cells by (A) WB (B) RT-qPCR (C) Flow Cytometry in control and MM conditions. Protein expressions of IDH2 in HuH-7 cells by (D) WB in control and MM conditions. In silico validation/analysis of IDH2 expression in open-access datasets; (E) GSE164441 and (F) E-MEXP-3291. IDH2 expression of motile and non-motile SNU-449 cells in control (G) and MM conditions (H) (Unpaired t-test, ns: nonsignificant, *P < 0.05, **P < 0.01). IDH2:Isocitrate Dehydrogenase (NADP(+))2; MM: metabolic medium; NAFLD: non-alcoholic fatty liver disease; HCC: hepatocellular carcinoma; NASH: non-alcoholic steatohepatitis
We also performed in silico validation/analysis with open-access transcriptome datasets related to NASH/NAFLD-based HCC. E-MEXP-3291[33] and GSE164441[34] were re-analyzed for NASH or NAFLD-based HCC and control comparison. We signified that Isocitrate dehydrogenase 2 (IDH2) expression is significantly low in NASH and NAFLD-based patients compared to control samples in independent datasets [Figure 6E and F]. [Terms are used as defined in the datasets (NASH and NAFLD)].
We also analyzed the expression level of IDH2 in RNA samples obtained from non-motile cells. When we compared IDH2 expression level both in control and MM conditions, cells that were motile expressed IDH2 less than cells that were non-motile [Figure 6G and H].
Expression of IDH2 is significantly low in tumors and related to aggressive phenotype in HCC patients
We performed bioinformatics analysis to investigate the effect of IDH2 expression level in open-access patient datasets: TCGA and CPTAC. Firstly, we analyzed IDH2 mRNA and protein levels in TCGA and CPTAC liver hepatocellular carcinoma cohorts (LIHC) using UALCAN[24-25]. IDH2 expression was found to be significantly low in tumors compared to normal samples in terms of mRNA and protein levels
Figure 7. (A and B) Expression analysis of IDH2 in mRNA and protein level (A) TCGA-LIHC; P = 7.11 E-01; n: tumor = 371, normal = 50 and (B) CPTAC-LIHC; P = 4.52 E-34 n: tumor = 165 normal = 165 Graphs were generated by UALCAN. (C-H) Analysis of differentially expressed (DE) genes and clinical characterizations in Low-IDH2 vs. High-IDH2 tumors in TCGA cohort (C)Volcano graph of DE genes. Statistically significant DE genes are represented by blue dots (P < 0.05, FC > 2) (D) Progress-free survival of patients in Low-IDH2 and High-IDH2 expressed groups. Low-IDH2 patients’ (E) Histologic grade; (F) Aneuploidy score; (G) Hypoxia score; and (H) Fraction genome altered of tumors are higher than High-IDH2 patients. Graphs were generated by cBioPortal.
We also analyzed TCGA-LIHC data by splitting the tumor samples into quartiles according to their IDH2 normalized transcript levels. Then, we performed differential expression (DE) analysis between Low-IDH2 expression quartile (Q1) and High-IDH2 expression quartile (Q4) via using cBioPortal[26-28]. 1795 genes were found to be upregulated and 1,573 genes were downregulated in the Low-IDH2 group and listed as DE gene list [Figure 7C]. When we performed KEGG pathway analysis to find pathways associated with DE gene lists, the results showed that most of the DE genes were taking part in important pathways such as the “Metabolic pathways, Pathways in cancer, PI3K-Akt signaling pathway, Cell cycle, Insulin signaling pathway, Hepatocellular carcinoma, Focal adhesion, Non-alcoholic fatty liver disease” (data not shown).
Survival analysis with Low-IDH2 and High-IDH2 groups showed that HCC patients in the Low-IDH2 group had significantly shorter progress-free survival time [Figure 7D] and shorter overall survival (data not shown) in 5 years/60 months.
We performed further analysis to characterize the relation of the IDH2 expression pattern with the clinicopathological features of HCC tumors. We observed that IDH2 expression level is negatively correlated with histological grade status. Low-IDH2 patients were significantly clustered in a higher “histological grade” group [Figure 7E]. Low-IDH2 patients had significantly higher aneuploidy, hypoxia, and fraction genome altered scores [Figure 7F-H].
We also analyzed IDH2 expression using immunoperoxidase staining [Figure 8]. Expressions of IDH2 were examined in HCC tissue samples (n = 26) and in parenchyma representing normal-adjacent tissue (n = 26) [Figure 8C]. In HCC tissues, we observed 15% positive staining, and in parenchyma, 23% positive staining for IDH2 (P > 0.05). When we analyzed the expression status of IDH2 according to microvascular invasion, we observed that IDH2 was expressed in 25% of the cases that had microvascular invasion, but 75% of the cases without microvascular invasion had IDH positivity [Figure 8D].
Figure 8. Immunohistochemical staining of IDH2 in HCC patient tissues and parenchyma. When more than 10% of the hepatocytes are stained (brown), defined as positive for IDH2 (A); others were defined as negative (B). Comparison of the ratios of positive staining for IDH2 in parenchyma and HCC tumor tissues (C); and in tumor samples of patients with/without microvascular invasion (D)
DISCUSSION
MASLD is a growing cause of chronic liver disease and is the dominant cause of HCC, particularly in developed countries. Due to its complicated and multifactorial nature, there are still no FDA-approved drugs for the treatment of MASLD, and therapeutic targets and drug therapies are being actively investigated. A number of preclinical and clinical studies have made rapid progress. However, there is still limited information on natural history and key pathogenic drivers. Furthermore, the validation of predictive biomarkers of disease risk and response to therapy is absent and critically needed. Moreover, the lack of optimal in vitro and in vivo models to mimic the disease makes it difficult to discover druggable targets and new therapeutic agents. Regarding the unmet challenges, we first developed a relevant in vitro model to examine the process and mechanism of MASLD on the progression of HCC. After validating the model, we tested the novel 3D co-culture system mimicking MASLD-ME on the aggressive phenotype of HCC
One of the crucial events occurring in MASLD progression to NASH and then HCC is lipotoxicity resulting from an excessive FFA influx to liver cells. Cellular effects of FFA accumulation causing negative cellular responses (lipotoxicity) are generally studied in vitro; however, it is a challenging step to manage. FFA are poorly soluble, and they are required to be dissolved in an appropriate solvent and to be complexed with a suitable carrier to manage their delivery to the cells without any side effects. In this study, to achieve optimum FFA concentrations in the cells, we first determined the types and concentrations of fatty acids, and the ratio of BSA to bound FFA. In addition to excessive caloric intake in the form of a high-fat diet, excessive fructose-and glucose-contained drinks or foods are also causes of hepatic insulin resistance. They promote de novo lipogenesis, impair fatty acid oxidation, induce endoplasmic reticulum stress, and trigger hepatic inflammation. Thus, we incorporated insulin, glucose, and fructose into the system to mimic MASLD-inducing diet. After optimization studies given in Supplementary Figure 1, we determined optimum concentrations for each component and named it “metabolic medium (MM)”. We observed that MM induced migration and spheroid formation in the tested HCC cells. Intriguingly, MM treatment increased proliferation for 24 h, but later, it led to an accumulation of cells in the S-G2/M phases of cell cycle, implying that cells were arrested. We have previously reported that hepatocyte growth factor induction increased motility, invasion, and branching-tubulogenesis formation of HCC cells, including SNU-449, do not alter proliferation[38,39]. Cancer cell dissemination, which is a critical aspect of metastasis, requires a switch from a proliferative to an invasive state. Cell cycle arrest has been reported for HCC, breast, and lung cancers (reviewed by Kohrman and Matus)[40]. It seems that MM induced the acquisition of less proliferative but more invasive and motile characteristics in cells.
Persistent hepatic lipotoxicity following hepatic injury and chronic inflammation activates quiescent HSCs[41,42]. HSCs can be activated by inflammation and participate in the regulation of liver inflammation[16]. They are the main cells that play a significant role in liver fibrosis which is the consequence of the accumulation of ECM, including type I collagen. Thus, to investigate the molecular mechanism of HCC associated with MASLD, we designed our 3D model, using (i) HCC cell line SNU-449 and HSC cell line
DigiWest protein profiling analysis using SNU-449 protein lysates showed that, particularly, “mTOR signaling, PI3K-Akt signaling pathway, RTK/MAPK signalling pathways were affected by MM treatment. This finding implies that metabolic pathways and pathways regulating tumorigenesis and mobility are predominantly affected. Since metabolic reprogramming in MASLD and HCC development is an important factor, including alterations of activity and expression of tricarboxylic acid (TCA) cycle enzymes, we chose IDH2 for further analysis.
IDH2 is a mitochondrial key metabolic enzyme that catalyzes the conversion of isocitrate to
Further, analyses of IDH2 expression in normal liver and HCC tissues using TCGA and CPTAC patient datasets were conducted through bioinformatics analysis. These analyses also revealed that IDH2 expression significantly downregulated in HCC compared to normal counterparts. Strikingly, our analysis to find pathways associated with IDH2 expression levels was related to “Metabolic pathways, Pathways in cancer, Cell cycle, PI3K-Akt signaling pathway, Insulin signaling pathway, Hepatocellular carcinoma, Focal adhesion, Non-alcoholic fatty liver disease”. These pathways are closely related to the DigiWest results that we obtained with our 3D model. Low-IDH2 patients had poorer prognosis compared to the patients with High-IDH2. It has been known that an aggressive phenotype is related to poor prognosis in HCC[39]. Moreover, when we characterized clinicopathological features of HCC, we observed that the Low-IDH2 patients had significantly higher aneuploidy, hypoxia, and fraction genome altered scores, which are associated with aggressive phenotype and drug resistance (reviewed by Lukow et al. and Tang et al.)[52,53]. The Low-IDH2 group had a significantly shorter survival time and higher grade than High-IDH2 ones. In addition, Zhang et al. reported that IDH gene polymorphisms can be an independent prognostic marker for HCC patients treated with TACE[54]. Our IHC analysis also demonstrated that IDH2 expression was downregulated in HCC samples compared to parenchyma and its expression was lower in the tissues that had microvascular invasions. Overall, these data imply that, as has been shown in the literature, the downregulation of IDH2 expression is an indicator of a more aggressive phenotype. However, to clarify the effects of MASLD microenvironment on the regulation of IDH2 level with underlying mechanisms, further studies involving a big cohort of MASLD-induced HCC patients, along with silencing or overexpression studies, are needed. Interestingly, in opposed to cholongiocarcinoma, our in silico analysis showed that HCC cell lines do not have IDH2 mutations (data not shown). Thus, it seems that IDH2 acts as a tumor suppressor in HCC and the loss of its function leads to the acquisition of a more aggressive phenotype. Thus, a different approach is needed to target MASLD-mediated decrease in IDH2 levels for HCC patients than treatment with current IDH1/IDH2 inhibitors that target IDH2 mutated tumors.
The major limitation of this study is the small sample size and lack of detailed medical records of patients regarding MAFLD history. Further studies are needed with larger cohorts, including metabolic characteristics of patients. Another limitation is using condition-medium instead of 3D co-culture systems containing hepatic stellate cells and/or immune system cells with HCC cell lines that reflect cell-cell contacts occurring during disease progression. Although we established that IDH2 expression is downregulated in MAFLD-related HCC, it is noteworthy that the precise function of mutation-independent decrease of IDH2 in HCC needs to be confirmed using in vivo animal models.
In conclusion, the 3D MASLD model presented in this study represents the pro-inflammatory and pro-fibrotic MASLD microenvironment. This 3D MASLD model resembles the pathophysiology of HCC induced by MASLD and is considerably low-cost, with easily changeable components including cell lines and ECM molecules, as well as medium. Thus, it is a promising model for investigating the molecular mechanisms of MASLD-inducing HCC and testing new drug targets. Using this 3D model, we also demonstrate for the first time that MASLD-ME causes a mutation-independent decrease in IDH2 levels and promotes aggressive behaviors in HCC. Additionally, we confirmed the role of low IDH2 levels in poor prognosis in HCC by bioinformatics analysis of publicly available datasets and IHC stainings of primary HCC tissues.
DECLARATIONS
Acknowledgments
We thank Prof. Dr. Mehmet Öztürk and Prof. Dr. Zeynep Fırtına Karagonlar for providing cell lines. We thank Assoc. Prof. Duygu Sağ and Assoc. Prof. Şerif Şentürk for providing TGFB1 and IL1B primers. During the preparation of this work, the authors used BioRender to create Figure 5A and the graphical abstract.
Authors’ contributions
Conceptualization, methodology, investigation, formal analysis, visualization, writing the original draft & editing: Korhan P
Methodology, investigation, formal analysis, visualization, writing the original draft & editing: Bağırsakçı E, Solmaz G
Bioinformatics data analysis, methodology, investigation, formal analysis, visualization, writing the original draft & editing: Öztemur Islakoğlu Y
Histological evaluations, IHC stainings and evaluation, editing the original draft: Sarıkaya B, Nart D, Yılmaz F
Conceptualization, investigation, methodology, writing-review & editing, supervision, funding acquisition, project management: Atabey N
Discussed the results and contributed to the final manuscript: Korhan P, Bağırsakçı E, Öztemur Islakoğlu Y, Solmaz G, Sarıkaya B, Nart D, Yılmaz F, Atabey N
Availability of data and materials
Not applicable.
Financial support and sponsorship
This work was supported by “Scientific and Technological Research Council of Turkey (TUBITAK)” with the grant number 119S698.
Conflicts of interest
All authors declared that there are no conflicts of interest.
Ethical approval and consent to participate
Research involving human subjects, human material or human data was performed in accordance with the Declaration of Helsinki and approved by The Ethics Board of Ege University on March 6, 2019 and number 19-3T/39. Written broad informed consents were obtained from patients before liver transplantation or liver biopsy sampling.
Consent for publication
Not applicable.
Copyright
© The Author(s) 2024.
Supplementary Materials
REFERENCES
1. Riazi K, Azhari H, Charette JH, et al. The prevalence and incidence of NAFLD worldwide: a systematic review and meta-analysis. Lancet Gastroenterol Hepatol 2022;7:851-61.
2. Teng ML, Ng CH, Huang DQ, et al. Global incidence and prevalence of nonalcoholic fatty liver disease. Clin Mol Hepatol 2023;29:S32-42.
3. Younossi ZM, Koenig AB, Abdelatif D, Fazel Y, Henry L, Wymer M. Global epidemiology of nonalcoholic fatty liver disease-Meta-analytic assessment of prevalence, incidence, and outcomes. Hepatology 2016;64:73-84.
4. Tan DJH, Setiawan VW, Ng CH, et al. Global burden of liver cancer in males and females: Changing etiological basis and the growing contribution of NASH. Hepatology 2023;77:1150-63.
5. Rinella ME, Lazarus JV, Ratziu V, et al. NAFLD Nomenclature consensus group. A multisociety Delphi consensus statement on new fatty liver disease nomenclature. J Hepatol 2023;78:1966-86.
6. Rinella ME, Neuschwander-Tetri BA, Siddiqui MS, et al. AASLD practice guidance on the clinical assessment and management of nonalcoholic fatty liver disease. Hepatology 2023;77:1797-835.
7. Chalasani N, Younossi Z, Lavine JE, et al. The diagnosis and management of nonalcoholic fatty liver disease: practice guidance from the American association for the study of liver diseases. Hepatology 2018;67:328-57.
8. European Association for the Study of the Liver (EASL); European Association for the Study of Diabetes (EASD); European Association for the Study of Obesity (EASO). EASL-EASD-EASO clinical practice guidelines for the management of non-alcoholic fatty liver disease. J Hepatol 2016;59:1121-40.
9. Bessone F, Razori MV, Roma MG. Molecular pathways of nonalcoholic fatty liver disease development and progression. Cell Mol Life Sci 2019;76:99-128.
11. Buzzetti E, Pinzani M, Tsochatzis EA. The multiple-hit pathogenesis of non-alcoholic fatty liver disease (NAFLD). Metabolism 2016;65:1038-48.
12. Musso G, Gambino R, De Michieli F, et al. Dietary habits and their relations to insulin resistance and postprandial lipemia in nonalcoholic steatohepatitis. Hepatology 2003;37:909-16.
13. Leoni S, Tovoli F, Napoli L, Serio I, Ferri S, Bolondi L. Current guidelines for the management of non-alcoholic fatty liver disease: a systematic review with comparative analysis. World J Gastroenterol 2018;24:3361-73.
14. Donnelly KL, Smith CI, Schwarzenberg SJ, Jessurun J, Boldt MD, Parks EJ. Sources of fatty acids stored in liver and secreted via lipoproteins in patients with nonalcoholic fatty liver disease. J Clin Invest 2005;115:1343-51.
15. Mota M, Banini BA, Cazanave SC, Sanyal AJ. Molecular mechanisms of lipotoxicity and glucotoxicity in nonalcoholic fatty liver disease. Metabolism 2016;65:1049-61.
16. Kamm DR, McCommis KS. Hepatic stellate cells in physiology and pathology. J Physiol 2022;600:1825-37.
17. Brunt EM. Grading and staging the histopathological lesions of chronic hepatitis: the Knodell histology activity index and beyond. Hepatology 2000;31:241-6.
18. Piscaglia F, Svegliati-Baroni G, Barchetti A, et al. HCC-NAFLD Italian study group. clinical patterns of hepatocellular carcinoma in nonalcoholic fatty liver disease: a multicenter prospective study. Hepatology 2016;63:827-38.
19. Siddiqui RA, Jenski LJ, Neff K, Harvey K, Kovacs RJ, Stillwell W. Docosahexaenoic acid induces apoptosis in Jurkat cells by a protein phosphatase-mediated process. Biochim Biophys Acta 2001;1499:265-75.
20. Atabey N, Gao Y, Yao ZJ, et al. Potent blockade of hepatocyte growth factor-stimulated cell motility, matrix invasion and branching morphogenesis by antagonists of Grb2 Src homology 2 domain interactions. J Biol Chem 2001;276:14308-14.
21. Bozkaya G, Korhan P, Cokaklı M, et al. Cooperative interaction of MUC1 with the HGF/c-Met pathway during hepatocarcinogenesis. Mol Cancer 2012;11:64.
22. UCSC in-silico PCR. Available from: https://genome.ucsc.edu/cgi-bin/hgPcr [Last accessed on 17 May 2024].
23. Bağırsakçı E, Şahin E, Atabey N, Erdal E, Guerra V, Carr BI. Role of albumin in growth inhibition in hepatocellular carcinoma. Oncology 2017;93:136-42.
24. Chandrashekar DS, Bashel B, Balasubramanya SAH, et al. UALCAN: a portal for facilitating tumor subgroup gene expression and survival analyses. Neoplasia 2017;19:649-58.
25. Chandrashekar DS, Karthikeyan SK, Korla PK, et al. UALCAN: an update to the integrated cancer data analysis platform. Neoplasia 2022;25:18-27.
26. Cerami E, Gao J, Dogrusoz U, et al. The cBio cancer genomics portal: an open platform for exploring multidimensional cancer genomics data. Cancer Discov 2012;2:401-4.
27. Gao J, Aksoy BA, Dogrusoz U, et al. Integrative analysis of complex cancer genomics and clinical profiles using the cBioPortal. Sci Signal 2013;6:pl1.
28. de Bruijn I, Kundra R, Mastrogiacomo B, et al; AACR Project GENIE BPC Core Team, AACR Project GENIE Consortium. Analysis and visualization of longitudinal genomic and clinical data from the AACR project GENIE biopharma collaborative in cBioPortal. Cancer Res 2023;83:3861-7.
29. TCGA-PanCanAtlas publications. Available from: https://gdc.cancer.gov/about-data/publications/pancanatlas [Last accessed on 17 May 2024].
30. Kanehisa M, Goto S. KEGG: kyoto encyclopedia of genes and genomes. Nucleic Acids Res 2000;28:27-30.
31. Clough E, Barrett T. The gene expression omnibus database. In: Mathé E, Davis S, editors. Statistical Genomics. New York: Springer; 2016. pp. 93-110.
32. Sarkans U, Gostev M, Athar A, et al. The biostudies database-one stop shop for all data supporting a life sciences study. Nucleic Acids Res 2018;46:D1266-70.
33. Lake AD, Novak P, Fisher CD, et al. Analysis of global and absorption, distribution, metabolism, and elimination gene expression in the progressive stages of human nonalcoholic fatty liver disease. Drug Metab Dispos 2011;39:1954-60.
34. Yang W, Feng Y, Zhou J, et al. A selective HDAC8 inhibitor potentiates antitumor immunity and efficacy of immune checkpoint blockade in hepatocellular carcinoma. Sci Transl Med 2021;13:eaaz6804.
35. Spector AA, John K, Fletcher JE. Binding of long-chain fatty acids to bovine serum albumin. J Lipid Res 1969;10:56-67.
36. Jensen T, Abdelmalek MF, Sullivan S, et al. Fructose and sugar: a major mediator of non-alcoholic fatty liver disease. J Hepatol 2018;68:1063-75.
37. Treindl F, Ruprecht B, Beiter Y, et al. A bead-based western for high-throughput cellular signal transduction analyses. Nat Commun 2016;7:12852.
38. Korhan P, Erdal E, Atabey N. MiR-181a-5p is downregulated in hepatocellular carcinoma and suppresses motility, invasion and branching-morphogenesis by directly targeting c-Met. Biochem Biophys Res Commun 2014;450:1304-12.
39. Korhan P, Erdal E, Kandemiş E, et al. Reciprocal activating crosstalk between c-Met and caveolin 1 promotes invasive phenotype in hepatocellular carcinoma. PLoS One 2014;9:e105278.
40. Kohrman AQ, Matus DQ. Divide or conquer: cell cycle regulation of invasive behavior. Trends Cell Biol 2017;27:12-25.
41. Yamaguchi K, Yang L, McCall S, et al. Inhibiting triglyceride synthesis improves hepatic steatosis but exacerbates liver damage and fibrosis in obese mice with nonalcoholic steatohepatitis. Hepatology 2007;45:1366-74.
42. Feldstein AE, Werneburg NW, Canbay A, et al. Free fatty acids promote hepatic lipotoxicity by stimulating TNF-alpha expression via a lysosomal pathway. Hepatology 2004;40:185-94.
43. Cheng B, Huang Z, Wu L, Lin Y, Lin W, Zhu Q. Activation of human hepatic stellate cells enhances the metastatic ability of hepatocellular carcinoma cells via up-regulation of interleukin-1β. J BUON 2021;26:435-43.
44. Hong F, Tuyama A, Lee TF, et al. Hepatic stellate cells express functional CXCR4: role in stromal cell-derived factor-1alpha-mediated stellate cell activation. Hepatology 2009;49:2055-67.
45. Cubero FJ, Woitok MM, Zoubek ME, de Bruin A, Hatting M, Trautwein C. Disruption of the FasL/Fas axis protects against inflammation-derived tumorigenesis in chronic liver disease. Cell Death Dis 2019;10:115.
46. Peterová E, Mrkvicová A, Podmolíková L, Řezáčová M, Kanta J. The role of cytokines TGF-beta1 and FGF-1 in the expression of characteristic markers of rat liver myofibroblasts cultured in three-dimensional collagen gel. Physiol Res 2016;65:661-72.
47. Bronsard J, Savary C, Massart J, et al. 3D multi-cell-type liver organoids: a new model of non-alcoholic fatty liver disease for drug safety assessments. Toxicol In Vitro 2024;94:105728.
48. Guo J, Zhang R, Yang Z, Duan Z, Yin D, Zhou Y. Biological roles and therapeutic applications of IDH2 mutations in human cancer. Front Oncol 2021;11:644857.
49. Liu WR, Tian MX, Jin L, et al. High expression of 5-hydroxymethylcytosine and isocitrate dehydrogenase 2 is associated with favorable prognosis after curative resection of hepatocellular carcinoma. J Exp Clin Cancer Res 2014;33:32.
50. Tian GY, Zang SF, Wang L, Luo Y, Shi JP, Lou GQ. Isocitrate dehydrogenase 2 suppresses the invasion of hepatocellular carcinoma cells via matrix metalloproteinase 9. Cell Physiol Biochem 2015;37:2405-14.
51. Pan JH, Kim HS, Beane KE, et al. IDH2 deficiency aggravates fructose-induced NAFLD by modulating hepatic fatty acid metabolism and activating inflammatory signaling in female mice. Nutrients 2018;10:679.
52. Lukow DA, Sausville EL, Suri P, et al. Chromosomal instability accelerates the evolution of resistance to anti-cancer therapies. Dev Cell 2021;56:2427-39.e4.
53. Tang M, Bolderson E, O'Byrne KJ, Richard DJ. Tumor hypoxia drives genomic instability. Front Cell Dev Biol 2021;9:626229.
Cite This Article
Export citation file: BibTeX | EndNote | RIS
OAE Style
Korhan P, Bağırsakçı E, Öztemur Islakoğlu Y, Solmaz G, Sarıkaya B, Nart D, Yılmaz F, Atabey N. MASLD-mimicking microenvironment drives an aggressive phenotype and represses IDH2 expression in hepatocellular carcinoma. Hepatoma Res 2024;10:22. http://dx.doi.org/10.20517/2394-5079.2023.140
AMA Style
Korhan P, Bağırsakçı E, Öztemur Islakoğlu Y, Solmaz G, Sarıkaya B, Nart D, Yılmaz F, Atabey N. MASLD-mimicking microenvironment drives an aggressive phenotype and represses IDH2 expression in hepatocellular carcinoma. Hepatoma Research. 2024; 10: 22. http://dx.doi.org/10.20517/2394-5079.2023.140
Chicago/Turabian Style
Peyda Korhan, Ezgi Bağırsakçı, Yasemin Öztemur Islakoğlu, Gülhas Solmaz, Burcu Sarıkaya, Deniz Nart, Funda Yılmaz, Neşe Atabey. 2024. "MASLD-mimicking microenvironment drives an aggressive phenotype and represses IDH2 expression in hepatocellular carcinoma" Hepatoma Research. 10: 22. http://dx.doi.org/10.20517/2394-5079.2023.140
ACS Style
Korhan, P.; Bağırsakçı E.; Öztemur Islakoğlu Y.; Solmaz G.; Sarıkaya B.; Nart D.; Yılmaz F.; Atabey N. MASLD-mimicking microenvironment drives an aggressive phenotype and represses IDH2 expression in hepatocellular carcinoma. Hepatoma. Res. 2024, 10, 22. http://dx.doi.org/10.20517/2394-5079.2023.140
About This Article
Special Issue
Copyright
Data & Comments
Data
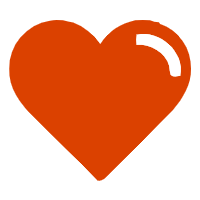
Comments
Comments must be written in English. Spam, offensive content, impersonation, and private information will not be permitted. If any comment is reported and identified as inappropriate content by OAE staff, the comment will be removed without notice. If you have any queries or need any help, please contact us at support@oaepublish.com.