Automatically targeting the dorsolateral subthalamic nucleus for functional connectivity-guided rTMS therapy
Abstract
Aim: Many resting-state functional magnetic resonance imaging (rs-fMRI) studies have provided evidence that repetitive transcranial magnetic stimulation (rTMS) exerts treatment effects via functional connectivity (FC) from a superficial stimulation target to a deep effective region. The dorsolateral subthalamic nucleus (DL-STN) is an effective target in deep brain stimulation surgery for Parkinson’s disease (PD), but its targeting highly depends on well-trained neurosurgeons and is not easily used for FC-guided rTMS. We aimed to devise a method for automatically localizing the DL-STN, and further develop a one-stop plug-in of rs-fMRI FC analysis to assist future individualized FC-guided rTMS.
Methods: Based on structural and iron-sensitive MRI of 78 participants, two raters defined the DL-STN coordinates with very high reliability. The averaged coordinates in the standard Montreal Neurological Institute (MNI) space were: left DL-STN, x: -11.89 ± 0.82, y: -14.51 ± 1.00, and z: -6.40 ± 1.01 and the right DL-STN, x: 12.53 ± 0.77, y:
Results: The AutoSTN seed showed significant FC with the motor cortices in all participants.
Conclusion: The AutoSTN-based rs-fMRI FC could guide future rTMS on PD. The one-stop plug-in CC-CAT can be used for any FC-guided rTMS treatment.
Keywords
INTRODUCTION
Parkinson’s disease (PD), one of the most frequent neurodegenerative disorders, is clinically characterized by akinesia, rigidity, resting tremor, and gait instability and is accompanied by various nonmotor complications, such as depression, cognitive decline, and sleeping problems, all of which could lead to significant family and social burdens[1,2]. Although dopaminergic drugs could effectively control motor symptoms at the early stage of PD, nonmotor symptoms might be worsened, and additional drug-related motor complications could emerge with long-term dopaminergic treatment[3,4]. Deep brain stimulation (DBS) surgery is a well-established and effective antiparkinsonian method for those patients who are refractory to medications, but it has a high cost and depends on strict patient selection criteria[5].
As a noninvasive technique, repetitive transcranial magnetic stimulation (rTMS) has been recommended for the treatment of PD[5-7]. While the commonly used figure “8” coil offers a focused stimulation approach, its focus could only reach 2~4 cm in depth[8-10], i.e., only the superficial cortex could be stimulated directly. However, the core pathology of most brain disorders, including PD[11], lies in the deep brain regions. Effectively modulating deep brain activity through the superficial cortex is essential for rTMS treatment. Wang et al. proposed a functional connectivity (FC)-guided rTMS approach with stimulation of the left parietal cortex which has FC with the hippocampus. The authors successfully modulated the FC of the hippocampus and significantly improved subjects’ associative memory performance[12]. This FC-guided rTMS approach might also be a promising therapy for patients with brain disorders.
The sensorimotor subdivision of the subthalamic nucleus (STN), a crucial node of the cortico-basal ganglia motor pathway[13,14], is an effective deep brain target in DBS surgery for PD patients[15-17]; the effectiveness of targeting this region indicates that the dorsolateral (DL)-STN might be a viable deep brain region in the FC-guided rTMS approach.
However, the small size, biconvex shape, oblique orientation in 3D planes, and special junctional position among deep brain regions of the DL-STN[18-20] make it a challenge to precisely target this region. To address the issue in DBS surgery, a method based on the anatomical landmarks of the red nucleus (RN) is widely used[21,22]. This method greatly depends on well-trained neurosurgeons and is not suitable for FC-guided rTMS.
Therefore, the current study developed an “AutoSTN” method to help automatically target DL-STN coordinates. Furthermore, a one-stop plug-in FC analytic toolbox named the “Connectivity and Coordinates Converting Assistant Toolbox (CC-CAT)” was developed to automatically target the DL-STN in native space and to serve as an individualized potential superficial stimulation target in rTMS therapy.
METHODS
Participants and data acquisition
The research was conducted at the Center for Cognition and Brain Disorders (CCBD) of Hangzhou Normal University (HZNU) and subjected to a rigorous review by the local ethics committee (No. 20140508). Recruited participants excluded those with a history of neurological and psychiatric disorders. For each subject, a written informed consent was signed before participation in this study. Eighty subjects were included in this study. Of these, two were excluded due to poor MRI image quality; thus, 78 subjects (60.9 ± 7.7 years old, 54 males) were finally included.
For all participants, a resting-state functional magnetic resonance imaging (rs-fMRI), 3D-enhanced susceptibility-weighted angiography (ESWAN), and 3D T1-weighted imaging scanning were collected on a 3T GE scanner (MR750, GE Medical Systems, Milwaukee, WI).
During rs-fMRI collection, the subjects were instructed to keep their eyes closed, avoid falling asleep, and remain as still as possible. The rs-fMRI images were scanned with the following parameters: repetition time (TR) = 2,000 ms, echo time (TE) = 30 ms, interleaved acquisition with a total of 43 slices, flip angle (FA) = 90°, collecting matrix = 64 × 64, field of view (FOV) = 220 × 220 mm2, spatial resolution = 3.44 × 3.44 ×
Data preprocessing
Individual DL-STN coordinate targeting based on anatomical landmarks
Data preprocessing for DL-STN coordinate targeting for each subject was performed based on AFNI
DL-STN coordinate targeting was conducted on the coregistered ESWAN image in AC-PC aligned stereotactic space according to the steps of defining DL-STN in DBS surgery[21,22] using AFNI. The details are shown in Figure 1.
Figure 1. Targeting the DL-STN coordinates of a subject. (A) The axial slice of the red nucleus with the maximal diameter was determined. The x coordinate of the DL-STN was defined 3 mm lateral to the lateral edge of the RN. The y coordinate was defined as the coordinate of a line tangent to the anterior edge of the RN; (B) The z coordinate of the DL-STN was defined 2 mm inferior to the superior edge of the RN. (C) and (D) are the coronal and axial views of the DL-STN coordinates, respectively. DL-STN: Dorsolateral subthalamic nucleus; RN: red nucleus.
We estimated the intra- (i.e., test-retest) and inter-rater reliability of all the x, y, and z coordinates using the intra-class correlation (ICC) according to the following equation[23,24]:
where MSr represents between-subject variance, MSe represents the random error, MSc represents between-rater variance, n is the number of subjects, and K is the number of raters.
Additionally, the intra- and inter-rater Euclidean distances of the x, y, and z coordinates for each subject were calculated.
The coordinates of our results were compared with those of Lozano’s DL-STN[22]. It should be noted that the origin of the coordinates in their study was the midpoint of the AC-PC line, and we transformed their coordinate origin to the AC.
The AutoSTN method to define the DL-STN coordinates
All the images, including the labeled image with DL-STN coordinates, were transformed to Montreal Neurological Institute (MNI) space. To estimate the variation in the individual DL-STN coordinates
FC of the DL-STN at the group level and individual level
The x, y, and z coordinates displayed high intra- and inter-reliability with very small Euclidean distances
The intra- and inter-rater reliability of the DL-STN coordinates
Intra-rater | Rater | Left DL-STN | Right DL-STN | ||||
x | y | z | x | y | z | ||
R1 | 0.965 | 0.991 | 0.999 | 0.990 | 0.993 | 0.996 | |
R2 | 0.981 | 0.985 | 0.999 | 0.987 | 0.989 | 0.999 | |
Inter-rater | Time | Left DL-STN | Right DL-STN | ||||
x | y | z | x | y | z | ||
1st | 0.950 | 0.978 | 0.999 | 0.953 | 0.983 | 0.999 | |
2nd | 0.950 | 0.971 | 0.999 | 0.967 | 0.975 | 0.999 |
To validate our assumption that the DL-STN has significant FC with the motor cortices, we conducted group DL-STN FC analysis in MNI space and individual DL-STN FC analysis in native space. All functional data analyses were processed using the DPABI 4.3 toolbox (DPABI_V2.3, http://rfmri.org/dpabi) and validated by using the newly developed plug-in “CC-CAT” (please see “Automatic implementation using the ‘CC-CAT’ plug-in” section).
For the FC in MNI space, we first preprocessed the images through the following steps: (1) removal of the first 10 time frames; (2) slice-timing correction to correct the slice-dependent delays; and (3) head motion correction. According to the realignment parameter of head movement, the subjects who presented more than 2 mm displacement or 2-degree translation in any of the x, y, or z direction were excluded. Then, we performed: (4) co-registration of fMRI images to the AC-PC aligned T1 images as mentioned in “Individual DL-STN coordinate targeting based on anatomical landmarks” section; (5) normalized images to MNI space using EPI normalization; (6) bandpass filtering of 0.01-0.08 Hz; (7) removal of linear trends and nuisance covariates with head motion (Friston-24), white matter (WM), and cerebrospinal fluid (CSF) parameters; and (8) spatial smoothing using a Gaussian kernel with full-width half maximum (FWHM) = 6 mm. After preprocessing, the time frame of the voxel of the DL-STN (DL-STN on the left, x: -11.89 ± 0.82, y: -14.51 ± 1.00, and z: -6.40 ± 1.01 and DL-STN on the right, x: 12.53 ± 0.77, y:
Considering that FC-guided rTMS would be performed in the native space, we transformed the individual FC maps within the motor cortex mask from MNI space to native space.
Automatic implementation using the “CC-CAT” plug-in
We have developed a one-stop solution, a plug-in named “CC-CAT” based on RESTplus (http://restfmri.net/forum/), for automatic implementation.
In this plug-in, users only need to perform one single click and input a few parameters, i.e., the TR and slice numbers, and the program will automatically perform all the steps we mentioned above.
As Supplementary Figure 2 shows, users need to select the region of interest (ROI) (the DL-STN for this paper), type in the TR and the number of slices in the fMRI data that are included, set the input/output directory, and then just perform a single click on the button labeled “Press to Convert”.
The program will write the following results to the output directory [Supplementary Figure 3]:
1. Left and right STN coordinates in native space (text file, i.e., rev_LROI_Coordinate.txt, rev_RROI_Coordinate.txt).
2. Left and right DL-STN masks in native space (NIFTI files with prefixes “rev_LSTN_MNI” and “rev_RSTN_MNI”).
3. Left and right STN-based FC map in native space (NIFTI files with prefixes “rev_LROI1FC_” and “rev_RROI2FC_”).
4. Left and right STN-based FC map in MNI space (NIFTI files with prefixes “ROI1FC_” and “ROI2FC_”).
All the FC analyses mentioned above were validated by using the newly developed plug-in toolbox.
RESULTS
The DL-STN coordinates in the native AC-PC aligned space
The DL-STN coordinates in the native AC-PC aligned space displayed high intra- and inter-rater reliability (ICCs > 0.95, Table 1). The overall intra- and inter-rater Euclidean distances of the x, y, and z coordinates were very small [Supplementary Figure 1]. Three subjects displayed intra-rater distances of 3-4 mm in any direction (x, y, and z) [Table 2], and 8 subjects presented inter-rater distances of 3-4 mm in any direction
The subjects had an intra-rater distance of 3-4 mm by both raters
Rater | DL-STN | Subject ID | Intra-rater distance | ||
x | y | z | |||
R1 | Left | e319 | 3 | 0 | 0 |
R2 | Left | e281 | 0 | 4 | 1 |
R2 | Right | e340 | 0 | -3 | 0 |
The subjects had an inter-rater distance of 3-4 mm at both rating times
Time | DL-STN | Subject ID | Inter-rater distance | ||
x | y | z | |||
1st | Right | e327 | -3 | -2 | -1 |
2nd | Left | e249 | 2 | -3 | -2 |
2nd | Left | e281 | 0 | 4 | 1 |
2nd | Left | e325 | 0 | -3 | -2 |
2nd | Left | e420 | 1 | -3 | -2 |
2nd | Right | e249 | -1 | -3 | 0 |
2nd | Right | e340 | -1 | -4 | -1 |
2nd | Right | e416 | -1 | -3 | -1 |
In native AC-PC aligned space, the DL-STN coordinates (x, y, and z) of our study were consistent with those of Lozano et al., with only the z coordinate being approximately 1 mm inferior to that of Lozano’s DL-STN [Supplementary Table 1][22].
The DL-STN coordinates in standard MNI space
Overall, the average coordinates of the DL-STN in standard MNI space were as follows: the left DL-STN, x: -11.89 ± 0.82, y: -14.51 ± 1.00, and z: -6.40 ± 1.01 and the right DL-STN, x: 12.53 ± 0.77, y: -13.97 ± 0.86, and z: -6.57 ± 0.99 [Table 4].
The mean and SD of the DL-STN coordinates
Rater | Time | Left DL-STN | Right DL-STN | ||||||||||
x | y | z | x | y | z | ||||||||
Mean | SD | Mean | SD | Mean | SD | Mean | SD | Mean | SD | Mean | SD | ||
R1 | 1st | -12.23 | 0.88 | -14.29 | 0.84 | -6.21 | 1.07 | 13.03 | 0.70 | -13.71 | 0.74 | -6.31 | 1.00 |
2nd | -12.01 | 0.75 | -14.24 | 0.87 | -5.95 | 1.01 | 12.91 | 0.76 | -13.74 | 0.81 | -6.21 | 0.93 | |
R2 | 1st | -11.65 | 0.83 | -14.76 | 1.08 | -6.68 | 0.99 | 12.05 | 0.84 | -14.15 | 0.87 | -6.87 | 0.99 |
2nd | -11.65 | 0.83 | -14.76 | 1.20 | -6.77 | 0.95 | 12.12 | 0.77 | -14.28 | 1.01 | -6.88 | 1.03 | |
Overall | -11.89 | 0.82 | -14.51 | 1.00 | -6.40 | 1.01 | 12.53 | 0.77 | -13.97 | 0.86 | -6.57 | 0.99 |
The distances for x, y, and z between the individual DL-STN coordinates and the average DL-STN coordinates were less than 2 mm for 93.6% of subjects [Figure 2]. Five (6.4%) subjects displayed 2-3 mm from the average DL-STN coordinates in the x, y, or z coordinates targeted by both raters [Figure 2, Supplementary Table 2]. The coordinates of these outliers still displayed high intra- and inter-rater reliability (ICCs 0.797-0.998) [Supplementary Table 3].
The FC of the DL-STN
Overall, the DL-STN has high FC with the motor cortices, including the premotor area (PMA), primary motor cortex (M1), and supplementary motor area (SMA), at both the group level [Figure 3] and the individual level [Figure 4]. All individuals showed significant FC between the DL-STN seed ROI and the lateral motor cortices [Figure 4], with a peak r > 0.4 for most subjects [Supplementary Table 4]. For the five subjects who displayed coordinates 2-3 mm from the average DL-STN, the peak r was 0.3-0.9
Figure 3. One sample t-tests of the FC of the bilateral DL-STN (GRF correction, individual P < 1E-09, cluster P < 1E-07). (A) The right DL-STN; (B) the left DL-STN. FC: Functional connectivity; DL-STN: dorsolateral subthalamic nucleus; GRF: Gibbs random field.
Figure 4. The FC of the DL-STN within the motor cortex in native AC-PC space of one subject; (A) and (B) the FC of the left DL-STN within the left and right hemisphere motor cortices of one subject; (C)and (D) the FC of the right DL-STN within the left and right hemisphere motor cortices of the same subject. The high FC areas indicated by arrows might be potential therapeutic targets for future FC-guided rTMS. FC: Functional connectivity; DL-STN: dorsolateral subthalamic nucleus; AC-PC: anterior commissure-posterior commissure; rTMS: repetitive transcranial magnetic stimulation.
DISCUSSION
We developed an approach, i.e., AutoSTN, to help automatically target individual DL-STNs in the current study. The obtained DL-STN coordinates demonstrated significant FC with motor areas at both the group level and individual level.
The STN and motor pathway
As a crucial nucleus of both the hyper-direct and indirect motor circuits of the cortico-basal ganglia[13,14], the STN mediates inhibitory output from the basal ganglia to motor cortices. Abnormal increased FC between the STN and motor cortices[25,26] existed in PD patients compared with healthy subjects. This abnormally high connectivity may lead to excessive inhibitory outflow from the base ganglia to motor cortices, resulting in hypokinetic movement disorders in PD patients[27].
Automatic targeting of the DL-STN for FC-guided TMS
STN-DBS surgery is a well-established therapy for drug-refractory PD patients. However, it is an invasive approach with a high cost and depends on strict patient screening criteria[5]. Alternatively, rTMS is a noninvasive and low-cost technique and has been recommended for the treatment of PD patients[5-7]. Usually, the conventional stimulation target for rTMS located within the motor cortices[6,7,28] was defined according to “Hotspot”, a brain area corresponding to hand movement. As an individualized variation, the “Hotspot” is not always located within the M1 area[29]. Furthermore, the distance of the “Hotspot” defined according to different sessions would be varied from several millimeters to centimeters[30-32]. Additionally, this defined stimulation method ignores impaired activity because of brain lesions in PD patients. Therefore, individualized stimulation targets are essential for improving the therapeutic efficacy of rTMS for PD patients.
FC-guide rTMS approach could connect a superficial cortex with a core deep brain effective target in DBS surgery, such as the STN or the pallidus pars interna (GPi)[16,33], realizing individualized precise stimulation. Indeed, these effective deep brain targets for DBS were functionally connected with motor cortices. Indeed, these effective deep brain targets for DBS were functionally connected with motor cortices[34]. Furthermore, retrospective studies found that the FC strength between the subgenual anterior cingulate (sgACC) and the dorsolateral prefrontal cortex (DLPFC) was correlated with rTMS clinical efficacy for major depressive disorder (MDD)[35-37]. Moreover, fMRI-guided rTMS has been demonstrated to be effective in the treatment of MDD patients, and this individualized neuronavigational method was represented by Stanford Neuronavigational Therapy (SNT) which has been approved by FDA[38]. In line with numerous previous studies[39-41], we found significant FC between the STN and motor cortices (including M1, SMA, and premotor area). On the basis of these findings, although there was no evidence about FC-guided TMS utilization in PD, we presume that the FC peak within the motor cortices could be used as the superficial stimulation target in rTMS therapy for patients with PD.
However, the STN can be functionally divided into three subregions, i.e., the medial limbic, ventromedial associative, and dorsolateral motor subregions (DL-STN)[42-44]. The DL-STN was the region that provided the maximum therapeutic effect with the least adverse events during DBS surgery[45-47]. Although image-based DL-STN targeting in DBS surgery has been well established with modern MRI techniques, e.g., ESWAN, it is a challenge to manually localize multiple anatomical landmarks.
Here, we provided an AutoSTN method to automatically localize DL-STN coordinates. The AutoSTN method was performed based on the image-based DL-STN targeting method by manually localizing the DL-STN targets of 78 healthy subjects to obtain the average coordinates. It has been shown that the image-based DL-STN targeting method displayed the smallest variance compared with the indirect targeting method based only on AC and PC landmarks or the image-only direct targeting method, which was closely associated with the optimal electrode contact with satisfactory clinical outcome[21,22]. Our results showed that the DL-STN coordinates had both high intra- and inter-rater reliability (ICC > 0.95). The overall DL-STN coordinate targeting was in agreement with that of Lozano et al.[22] [Supplementary Table 1]. Moreover, most subjects displayed less than 2 mm of the intra- and inter-rater distances of the x, y, and z coordinates
Only 5 (6.4%) subjects displayed distances in the x, y, and z directions between the individual and the averaged DL-STN coordinates higher than 2 mm but less than 3 mm [Supplementary Table 2]. These five subjects did not display high intra- and inter-rater distances of more than 3 mm. Furthermore, all five subjects showed high FC between the DL-STN and the motor cortices. Collectively, these findings further implied that the average DL-STN coordinates can be used as the coordinates for most people, which allowed for the development of AutoSTN coordinates.
One-stop plug-in for “AutoSTN” implementation
Based on the software RESTplus, we developed a one-stop plug-in toolkit named “CC-CAT” for automatic seed-based FC analysis [Supplementary Figure 2]. As shown in our FC analysis results [Figure 4], future rTMS studies could consider the peak FC coordinates in the motor cortices as stimulation targets for rTMS therapy. We validated the one-stop plug-in and obtained the same results as those of DPABI
Limitations and cautions
We strongly suggest that researchers double-check the quality of spatial normalization by visual inspection of the plug-in “CC-CAT”, especially for rTMS treatment. The final coordinates should also be double-checked on the structural images. Furthermore, we could not exclude the variations resulting from different MRI machines and settings, and its robustness and adaptability should be explored in the future. Finally, as this study represents only an initial exploration of individualized FC-guide TMS therapy for PD, it did not include any patients with PD. Therefore, the effectiveness of DL-STN FC-guided rTMS as a therapy needs to be investigated in future clinical studies.
Conclusions
In our study, we developed an AutoSTN method to help automatically target the DL-STN, which corresponds to the average coordinates of a group of healthy subjects. Furthermore, based on the AutoSTN coordinates, a one-stop plug-in FC analysis was developed, which could be integrated into future FC-guided rTMS therapeutic procedures.
All original code has been deposited at GitHub and is publicly available at GitHub - Hongy1836/ccat-toolbox.
DECLARATIONS
Authors’ contributions
Made substantial contributions to conceptualization, data analysis, and manuscript writing: Zhao N, Qiao Y, Yue J, Jing Y
Made major contributions to manuscript review, editing, and made major contributions to study design: Zhen Y, Xiang YT, Wang J, Zang YF
Performed data acquisition, as well as providing technical and material support: Ge Q, Zhang J, Zhang M
Availability of data and materials
The data that support the findings of this study are available from the corresponding author upon reasonable request.
Financial support and sponsorship
This work was supported by the National Nature Science Foundation of China (NSFC) [81520108016].
Conflicts of interest
All authors declared that there are no conflicts of interest. Zang YF is an Editorial Board member of the journal Ageing and Neurodegenerative Diseases.
Ethical approval and consent to participate
The experiment was performed in accordance with the Declaration of Helsinki and approved by the Ethics Committee of the Center for Cognition and Brain Disorders (CCBD) at Hangzhou Normal University (HZNU) (No. 20140508). A written informed consent was obtained from each subject prior to participation in this study.
Consent for publication
Written informed consent for publication was obtained from all participants.
Copyright
© The Author(s) 2024.
Supplementary Materials
REFERENCES
1. Wirdefeldt K, Adami HO, Cole P, Trichopoulos D, Mandel J. Epidemiology and etiology of Parkinson’s disease: a review of the evidence. Eur J Epidemiol 2011;26 Suppl 1:S1-58.
3. Rascol O, Payoux P, Ory F, Ferreira JJ, Brefel-Courbon C, Montastruc JL. Limitations of current Parkinson’s disease therapy. Ann Neurol 2003;53 Suppl 3:S3-12; discussion S12.
4. Ceravolo R, Rossi C, Del Prete E, Bonuccelli U. A review of adverse events linked to dopamine agonists in the treatment of Parkinson’s disease. Expert Opin Drug Saf 2016;15:181-98.
5. Heumann R, Moratalla R, Herrero MT, et al. Dyskinesia in Parkinson’s disease: mechanisms and current non-pharmacological interventions. J Neurochem 2014;130:472-89.
6. Goodwill AM, Lum JAG, Hendy AM, et al. Using non-invasive transcranial stimulation to improve motor and cognitive function in Parkinson’s disease: a systematic review and meta-analysis. Sci Rep 2017;7:14840.
7. Li S, Jiao R, Zhou X, Chen S. Motor recovery and antidepressant effects of repetitive transcranial magnetic stimulation on Parkinson disease: a PRISMA-compliant meta-analysis. Medicine 2020;99:e19642.
8. Rossi S, Hallett M, Rossini PM, Pascual-Leone A; Safety of TMS Consensus Group. Safety, ethical considerations, and application guidelines for the use of transcranial magnetic stimulation in clinical practice and research. Clin Neurophysiol 2009;120:2008-39.
9. Rossini PM, Burke D, Chen R, et al. Non-invasive electrical and magnetic stimulation of the brain, spinal cord, roots and peripheral nerves: basic principles and procedures for routine clinical and research application. An updated report from an I.F.C.N. Committee. Clin Neurophysiol 2015;126:1071-107.
10. Deng ZD, Lisanby SH, Peterchev AV. Coil design considerations for deep transcranial magnetic stimulation. Clin Neurophysiol 2014;125:1202-12.
11. Obeso JA, Marin C, Rodriguez-Oroz C, et al. The basal ganglia in Parkinson’s disease: current concepts and unexplained observations. Ann Neurol 2008;64 Suppl 2:S30-46.
12. Wang JX, Rogers LM, Gross EZ, et al. Targeted enhancement of cortical-hippocampal brain networks and associative memory. Science 2014;345:1054-7.
13. DeLong MR, Wichmann T. Circuits and circuit disorders of the basal ganglia. Arch Neurol 2007;64:20-4.
14. Nambu A, Tokuno H, Takada M. Functional significance of the cortico-subthalamo-pallidal ‘hyperdirect’ pathway. Neurosci Res 2002;43:111-7.
15. Xie CL, Shao B, Chen J, Zhou Y, Lin SY, Wang WW. Effects of neurostimulation for advanced Parkinson’s disease patients on motor symptoms: a multiple-treatments meta-analysas of randomized controlled trials. Sci Rep 2016;6:25285.
16. Peng L, Fu J, Ming Y, Zeng S, He H, Chen L. The long-term efficacy of STN vs GPi deep brain stimulation for Parkinson disease: a meta-analysis. Medicine 2018;97:e12153.
17. Parsons TD, Rogers SA, Braaten AJ, Woods SP, Tröster AI. Cognitive sequelae of subthalamic nucleus deep brain stimulation in Parkinson’s disease: a meta-analysis. Lancet Neurol 2006;5:578-88.
18. Schaltenbrand G, Wahren W. Atlas for stereotaxy of the human brain. Georg Thieme. 1977. Available from: https://www.semanticscholar.org/paper/Atlas-for-Stereotaxy-of-the-Human-Brain-Schaltenbrand-Wahren/d181ed3002e0d5c503be5a565e500d1aa181220b. [Last accessed on 8 May 2024].
19. Chandran AS, Bynevelt M, Lind CRP. Magnetic resonance imaging of the subthalamic nucleus for deep brain stimulation. J Neurosurg 2016;124:96-105.
20. Ashkan K, Blomstedt P, Zrinzo L, et al. Variability of the subthalamic nucleus: the case for direct MRI guided targeting. Br J Neurosurg 2007;21:197-200.
21. Andrade-Souza YM, Schwalb JM, Hamani C, et al. Comparison of three methods of targeting the subthalamic nucleus for chronic stimulation in Parkinson’s disease. Neurosurgery 2008;62 Suppl 2:875-83.
22. Lozano CS, Ranjan M, Boutet A, et al. Imaging alone versus microelectrode recording-guided targeting of the STN in patients with Parkinson’s disease. J Neurosurg 2018;130:1847-52.
23. Koo TK, Li MY. A guideline of selecting and reporting intraclass correlation coefficients for reliability research. J Chiropr Med 2016;15:155-63.
24. Shrout PE, Fleiss JL. Intraclass correlations: uses in assessing rater reliability. Psychol Bull 1979;86:420-8.
25. Fernández-Seara MA, Mengual E, Vidorreta M, et al. Resting state functional connectivity of the subthalamic nucleus in Parkinson’s disease assessed using arterial spin-labeled perfusion fMRI. Hum Brain Mapp 2015;36:1937-50.
26. Baudrexel S, Witte T, Seifried C, et al. Resting state fMRI reveals increased subthalamic nucleus-motor cortex connectivity in Parkinson’s disease. Neuroimage 2011;55:1728-38.
27. Vitek JL, Giroux M. Physiology of hypokinetic and hyperkinetic movement disorders: model for dyskinesia. Ann Neurol 2000;47:S131-40.
28. Chen KHS, Chen R. Invasive and noninvasive brain stimulation in Parkinson’s disease: clinical effects and future perspectives. Clin Pharmacol Ther 2019;106:763-75.
29. Ahdab R, Ayache SS, Brugières P, Farhat WH, Lefaucheur JP. The hand motor hotspot is not always located in the hand knob: a neuronavigated transcranial magnetic stimulation study. Brain Topogr 2016;29:590-7.
30. Cincotta M, Giovannelli F, Borgheresi A, et al. Optically tracked neuronavigation increases the stability of hand-held focal coil positioning: evidence from “transcranial” magnetic stimulation-induced electrical field measurements. Brain Stimul 2010;3:119-23.
31. Julkunen P, Säisänen L, Danner N, et al. Comparison of navigated and non-navigated transcranial magnetic stimulation for motor cortex mapping, motor threshold and motor evoked potentials. Neuroimage 2009;44:790-5.
32. Weiss C, Nettekoven C, Rehme AK, et al. Mapping the hand, foot and face representations in the primary motor cortex - retest reliability of neuronavigated TMS versus functional MRI. Neuroimage 2013;66:531-42.
33. Wong JK, Cauraugh JH, Ho KWD, et al. STN vs. GPi deep brain stimulation for tremor suppression in Parkinson disease: a systematic review and meta-analysis. Parkinsonism Relat Disord 2019;58:56-62.
34. Fox MD, Buckner RL, Liu H, Chakravarty MM, Lozano AM, Pascual-Leone A. Resting-state networks link invasive and noninvasive brain stimulation across diverse psychiatric and neurological diseases. Proc Natl Acad Sci U S A 2014;111:E4367-75.
35. Fox MD, Buckner RL, White MP, Greicius MD, Pascual-Leone A. Efficacy of transcranial magnetic stimulation targets for depression is related to intrinsic functional connectivity with the subgenual cingulate. Biol Psychiatry 2012;72:595-603.
36. Weigand A, Horn A, Caballero R, et al. Prospective validation that subgenual connectivity predicts antidepressant efficacy of transcranial magnetic stimulation sites. Biol Psychiatry 2018;84:28-37.
37. Cash RFH, Cocchi L, Lv J, Fitzgerald PB, Zalesky A. Functional magnetic resonance imaging-guided personalization of transcranial magnetic stimulation treatment for depression. JAMA Psychiatry 2021;78:337-9.
38. Paychiatric News. FDA clears accelerated TMS protocol for depression. Available from: https://psychnews.psychiatryonline.org/doi/full/10.1176/appi.pn.2022.10.10.40. [Last accessed on 8 May 2024].
39. Isaacs BR, Forstmann BU, Temel Y, Keuken MC. The connectivity fingerprint of the human frontal cortex, subthalamic nucleus, and striatum. Front Neuroanat 2018;12:60.
40. Shen B, Gao Y, Zhang W, et al. Resting state fMRI reveals increased subthalamic nucleus and sensorimotor cortex connectivity in patients with Parkinson’s disease under medication. Front Aging Neurosci 2017;9:74.
41. Wang Z, Chen H, Ma H, Ma L, Wu T, Feng T. Resting-state functional connectivity of subthalamic nucleus in different Parkinson’s disease phenotypes. J Neurol Sci 2016;371:137-47.
42. Arnold Anteraper S, Guell X, Whitfield-Gabrieli S, et al. Resting-state functional connectivity of the subthalamic nucleus to limbic, associative, and motor networks. Brain Connect 2018;8:22-32.
43. Brunenberg EJL, Moeskops P, Backes WH, et al. Structural and resting state functional connectivity of the subthalamic nucleus: identification of motor STN parts and the hyperdirect pathway. PLoS One 2012;7:e39061.
44. Lambert C, Zrinzo L, Nagy Z, et al. Confirmation of functional zones within the human subthalamic nucleus: patterns of connectivity and sub-parcellation using diffusion weighted imaging. Neuroimage 2012;60:83-94.
45. Guehl D, Edwards R, Cuny E, et al. Statistical determination of the optimal subthalamic nucleus stimulation site in patients with Parkinson disease. J Neurosurg 2007;106:101-10.
46. Avecillas-Chasin JM, Alonso-Frech F, Nombela C, Villanueva C, Barcia JA. Stimulation of the tractography-defined subthalamic nucleus regions correlates with clinical outcomes. Neurosurgery 2019;85:E294-303.
Cite This Article
Export citation file: BibTeX | RIS
OAE Style
Zhao N, Qiao Y, Yue J, Jing Y, Ge Q, Zhang M, Zhang J, Zhen Y, Xiang YT, Wang J, Zang YF. Automatically targeting the dorsolateral subthalamic nucleus for functional connectivity-guided rTMS therapy. Ageing Neur Dis 2024;4:8. http://dx.doi.org/10.20517/and.2023.31
AMA Style
Zhao N, Qiao Y, Yue J, Jing Y, Ge Q, Zhang M, Zhang J, Zhen Y, Xiang YT, Wang J, Zang YF. Automatically targeting the dorsolateral subthalamic nucleus for functional connectivity-guided rTMS therapy. Ageing and Neurodegenerative Diseases. 2024; 4(2): 8. http://dx.doi.org/10.20517/and.2023.31
Chicago/Turabian Style
Zhao, Na, Yang Qiao, Juan Yue, Ying Jing, Qiu Ge, Meng Zhang, Jianguo Zhang, Yuan Zhen, Yu-Tao Xiang, Jue Wang, Yu-Feng Zang. 2024. "Automatically targeting the dorsolateral subthalamic nucleus for functional connectivity-guided rTMS therapy" Ageing and Neurodegenerative Diseases. 4, no.2: 8. http://dx.doi.org/10.20517/and.2023.31
ACS Style
Zhao, N.; Qiao Y.; Yue J.; Jing Y.; Ge Q.; Zhang M.; Zhang J.; Zhen Y.; Xiang Y.T.; Wang J.; Zang Y.F. Automatically targeting the dorsolateral subthalamic nucleus for functional connectivity-guided rTMS therapy. Ageing. Neur. Dis. 2024, 4, 8. http://dx.doi.org/10.20517/and.2023.31
About This Article
Copyright
Data & Comments
Data
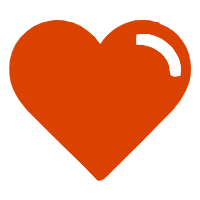

Comments
Comments must be written in English. Spam, offensive content, impersonation, and private information will not be permitted. If any comment is reported and identified as inappropriate content by OAE staff, the comment will be removed without notice. If you have any queries or need any help, please contact us at support@oaepublish.com.