Content
Special Interview with Prof. Kelly Cohen & Dr. Jon Ander Martin: Unmanned Aerial Vehicles
On October 14, 2022, the Editorial Office of Complex Engineering Systems (CES) was great honored to interview Prof. Kelly Cohen and Dr. Jon Ander Martin, Department of Aerospace Engineering & Engineering Mechanics, University of Cincinnati, Cincinnati, OH, USA. Prof. Kelly Cohen is also a Subject Editor of CES.
Attracted by their recent research interests and published article, “Comparison of battery modeling regression methods for application to unmanned aerial vehicles”.
In this interview, Dr. Jon Ander Martin gives us a detailed explanation about his article and Prof. Kelly Cohen provides a broader perspective on the impact of AI/ML on real-time prognostics and health management of autonomous vehicles (ground or air). Let us take a look at the details:
Q1: How correlated is the sizing of a battery in UAV performance?
A1: It's a very important matter to correctly decide the battery and I think there's mainly two reasons, the early the most obvious one, the first one we all think about is more weight in an aircraft, the performance is going to be worse, and you need that trade of between how many batteries you put in there, how large they are and what range do you want to achieve, so you need to reach that optimum point for whatever your application is of, okay this is my range, this is how much capacity I need, so you need to really balance that trade of and I would say that another very important reason is we are used to gen engines that consume gas, and so we're used to planes where the take off weight is not equal to the landing weight and the landing weight is lower because we burn fuel when we're flying, so the requirements for landing are let the weight of the aircraft is lower when it's landing so that means you can dimension your landing gear for a lower weight, you can half a lower approach speed and so on, which doesn't happen with an electric aircraft so it's really important for me to start two main reasons why it's really important to correctly size the battery.
Q2: From the real-time implementation viewpoints, which method [Support Vector Machine (SVM), Relevance Vector Machine (RVM), and Fuzzy Inference System (FIS)] presents good potential as a battery management method in UAV?
A2: So first thing I want to say we are not that far in the research yet, it's one of the next steps that we want to apply or not that far yet, but I'll share my thoughts of what I think, it's the best method anyway, so I think there's two things to consider here, one is the memory distorted capacity for the model, these are complex models, there needs to be not memory to store all these data, the date and the algorithm on board, that's the first thing to consider. The second one is the computational power, if the model is too complex, theseespecially the research we have conducted was all with multi-copter so they typically have single board computers which have just enough power to execute these methodologies, so the computational power is the second important thing, taking to count these two things I would say that both RVM and the Fuzzy Inference System are the best methodologies, you can reduce the model to a few data points and the execution is passed in both. If we need to put it in an aircraft, I would probably pick the FIS system, just because certification is going to be one of the next steps to do and the explainability of the fuzzy system is going to make it easier.
Q3: It is stated that the NASA Ames battery Lion dataset is obtained in a controlled area. What type of modifications are needed in SVM, RVM, and FIS methods for accurate estimation using the data set directly obtained from a UAV test?
A3: Again I have to say the comparison between lab testing and field testing is going to be part of the end of my pieces which I'm currently conducting, but again I can give you some ideas. So both RVM the three methodologies deal with the uncertain, with the additional and certainty that the field testing provides we have things that we cannot control as supposed to allow the problem with these two with RVM and SVM is that there is the parameter, the margin with that we need to estimate and we cannot estimate until we have some data and we look at results then we can estimate that parameter, and it's pretty important to have a good value for that otherwise we're gonna either overfit, if we don't get that parameter, we're going to overfit especially the SVM methodology, RVM being probably, it doesn't require such a good estimation but SVM especially needs a very good estimation of that parameter,the fuzzy system, the phase does not need a parameter, fuzzy systems naturally embrace the uncertainties, so I would say that the fuzzy system is the best one for that reason when we're dealing with uncertainty from field testingand I don't think it requires more modifications because it already embraces that uncertainty.
Q4: Can the proposed data-driven approach be also utilized for accurate and robust surface temperature estimation of a battery, which is an important degeneration factor in batteries?
A4: The short answer is yes, the methodologies allow for multi dimensional that I input, so the short answer is yes, we can include that the problem of course is as we add more variables into the model, we need one more data andto get the good model, it's the curse of dimensionality we are all familiar with it, and also we'll need a longer times to get the model like computationally and so on. I guess that it depends how accurate you want to estimate the temperature, I think that you can do simple models but accurate enough, following physical modeling are supposed to data driven modeling for a temperature the battery has very complex dynamics inside, but I think that the temperature if we want a simplest model, it is enough to get just a physical model, instead of the data driven model, now I think it would be interesting to study that trade of how accurate do we want it, and how much computational power are we adding to the equation if we want to get this.
Q5: Due to the complicated electrochemical dynamics, battery aging is an important phenomenon to properly model and estimate state of health(SOH), especially in ground vehicles. How can this phenomenon be addressed in UAV applications?
A5: As the question says it is a complicated problem, the dynamics are very complicated and I think that right now there's two ways to deal with it, one is go for a very complicated physical model which will requires a lot of knowledge about the internal chemistry of the battery, about the reactions happening in their, they are very heavy models which I don't think that can be run in a single board computer as we're doing right now with small quadcopters multicopters and so on. The other option is a lot of testingn, if you want to study the dynamics of a complex system, you need a lot of testing to do it and that's the other way we have, they both have their pros and cons but I would say those two are the main approaches.
Q6: Can the proposed techniques be extended to estimate other internal states such as State of Energy (SOE), State of Power (SOP), and State of Temperature (SOT) for effective charge management of batteries?
A6: Sure answer yes, in a previous publication to this one, we showed how to apply RVM to the state of health problem which is very closely related to the state of energy problem, so yes and then generally speaking, these methodologies are all suitable we've shown it in this paper, all these methodologies are suitable to model strongly known linear viables and problems, both the state of temperature and the state of power are going to be non linear progressions, so we have not tested, we have not applied the methodologies to them, but my prediction is that they should work because it's more or less the same kind of non linear variable.
Then we invite Prof. Kelly Cohen to provide a broader perspective on the impact of AI/ML on real-time prognostics and health management of autonomous vehicles (ground or air).
A: So we are in this industry 4.0 period where there's a lot of data and lot of technologies that can allow us to see an increase in autonomous intelligence systems both ground and air, also with the advanced mobility which has an economic global impact of 1.9 trillion dollars, you can see that the world of aerospace is changing with many new players coming in for this vision to be realized, you need to be able to guarantee safety, you need to be able to guarantee performance, and this assurance is only possible when you include the techniques of what happens when there are malfunctions, what are you fall tolerant, and in order to be able to control and guarantee safety when things are not nominal, when things have been degraded, we need to be able to have a very good prognostic, so given the rise of machine learning and the opportunities that we see the capabilities of lot of the AI techniques to be able to deliver accurate predictions, we will be able to realize this field and we think in the next decade or two, we would be able to get there now, not all AI systems and algorithms are the same, there are those that are more trustworthy than others, there are those that are more brittle than others, so we've got to be careful to ensure because when we release these autonomous systems into the public, they have to perform and the public needs to trust them, so if I'm going to have my children, my family be on an autonomous car or an autonomous air taxi, I've got to make sure that I'm not putting them in harm's way, for that to happen I have to trust the system, to be able to trust the system I need to have a set of very trustworthy autonomous systems that will make that happen, and the techniques that we are building the ability to monitor the health of a vehicle would be crucial and critical where it comes to guaranteing that safety.
Biography of the interviewees
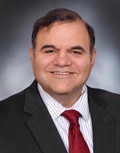
Prof. Kelly Cohen, the Brian H. Rowe Endowed Chair in aerospace engineering, has been a faculty member since 2007 in the Department of Aerospace Engineering and Engineering Mechanics at UC's College of Engineering and Applied Science. His career is marked by achievement in the field of aerospace engineering and education, including the SOCHE (Strategic Ohio Council for Higher Education) Excellence Award, UC George Barbour Award for Good Faculty-Student Relations, UC Faculty Core Values Award, UC Dolly Cohen Award for Excellence in Teaching, the American Institute of Aeronautics and Astronautics Outstanding Technical Contribution Application Award, the CEAS Distinguished Researcher Award and the Greater Cincinnati Consortium of Colleges and Universities Excellence in Teaching Award, among many others. Current Work: Explainable AI for aerospace and bio-medical applications, Collaborative UAV control and task planning, multi-agent decision making, UAV operations for enhanced situational awareness and resource allocation, disaster management using UAVs, UAV flight control design and testing, UAVs for package delivery, estimation and control for feedback aerodynamic flow control, system identification/control of rotorcraft/VTOL aircraft.
Dr. Jon Ander Martin, Industrial Engineer. PhD candidate Aerospace Engineering at University of Cincinnati. Test Engineer at Electric Power Systems. Aug 2017 - May 2018, Electronic safety device for working zone delimitation in high voltage electrical substations, associated with UPV/EHU, designed a portable electronic device from scratch & learned first-hand the development of a new product. Jun 2017- Aug 2019, Formula Student Bizkaia, Electronics Group Manager, achievements: 2nd place in Cost & Manufacturing, FSUK 2019; 3rd place in Design presentation, FSUK 2019; 2nd best Electric Vehicle, FSUK 2019, 10th place overall classification, FSUK 2019.