Preface for “renewable power generation, energy storage, and resource management challenges and solutions”
Renewable energy sources offer many benefits, such as reducing carbon footprint, diversifying the energy mix, and enhancing energy security. However, they simultaneously pose some challenges, such as intermittency, variability, unpredictability, and low power density[1]. The high penetration of renewable energy integration will profoundly change the system form, architecture characteristics, and operation mechanism of traditional power systems, which increases the complexity of reliable power supply, economical operation, and the safety and stability of power systems[1,2]. First of all, improving energy conversion efficiency, power prediction level, and other measurements can help to achieve the grid friendliness, power support ability, and capability to withstand power system disturbances of renewable energy generation stations[3]. Secondly, the prediction of renewable energy generation power output is closely combined with external factors such as meteorological conditions, and it is necessary to enhance the real-time state collection, perception, and processing capabilities, thereby realizing the flexible scheduling of the power system. In addition, the large-scale application of multi-time scale energy storage should be promoted to achieve intelligent dispatch and control of the power system, which could be adopted to the solution of multiple interactions among renewable energy generation, the power grid, load demand, and energy storage systems[4,5].
Energy storage is an essential technology combination for new energy grid connection and green transportation, which has become one of the key elements in building a new type of power system[6]. Although the cost of energy storage has decreased, it still belongs to asset-intensive equipment, and its cost issue is a key factor restricting the promotion of energy storage[7]. In addition, the instability of energy storage policies and the market environment severely limits the application scenarios of energy storage and the retirement treatment issues caused by large-scale deployment of energy storage devices, which are also worthy of attention. Energy storage has a promising future supported by the strategic goal of “double carbon”. We need to deepen the construction of the electricity auxiliary service market, improve market varieties such as frequency regulation, peak shaving, and backup, and support energy storage entities to participate in energy trading mechanisms. In addition, combined with the characteristics of new energy resources, network architecture, and load demand, the combination configuration of energy storage is reasonably formulated, the new business model of promoting centralized construction of energy storage equipment and energy storage sharing operation is promoted, and the measures of increasing scientific and technological innovation and operation and maintenance management to improve the safety and water equality of energy storage operation are widely concerned by scholars[8].
Resource Management involves operational issues such as economic dispatch and planning, including location and capacity determination[9,10]. The challenges of resource management include precise prediction of parameter changes, the establishment of system models in advance, and online scheduling. With regard to parameter changes, linear programming, quadratic programming, and other models usually assume that the optimization model parameters are given/stable[11]. The characteristics of these methods are intuitive modeling ideas and complete theories such as duality and approximation, but wind and solar power generation rely on intermittent and random meteorological conditions, and loads are affected by various complex factors, making it difficult to predict[12]. In order to analyze the influence of parameter perturbation of the optimization model, the random optimization assumes that the probability distribution of the parameters is given or can be obtained, and the changing scenario is constructed through the sampling average approximate solution strategy of the Monte Carlo method. The difficulty of this method lies in the fact that the probability distribution of uncertain parameters is unknown or difficult to estimate, which increases the complexity of the stochastic optimization model. Robust optimization is oriented towards the worst-case scenario, making the optimal solution sufficiently robust to all changes in parameters in a bounded uncertain set. In order to achieve online scheduling, reinforcement learning uses resource management as an energy system to learn the optimal decision and maximize benefits in continuous interaction with renewable power generation, energy storage, and users without establishing a system model in advance.
DECLARATIONS
Authors’ contributionsThe author contributed solely to the article.
Availability of data and materialsNot applicable.
Financial support and sponsorshipNone.
Conflicts of interestAll authors declared that there are no conflicts of interest.
Ethical approval and consent to participateNot applicable.
Consent for publicationNot applicable.
Copyright© The Author(s) 2023.
REFERENCES
1. Nehrir MH, Wang C, Strunz K, et al. A review of hybrid renewable/alternative energy systems for electric power generation: configurations, control, and applications. IEEE Trans Sustain Energy 2011;2:392-403.
2. Aaslid P, Korpas M, Belsnes MM, Fosso OB. Stochastic optimization of microgrid operation with renewable generation and energy storages. IEEE Trans Sustain Energy 2022;13:1481-91.
3. Du E, Zhang N, Hodge B, et al. The role of concentrating solar power toward high renewable energy penetrated power systems. IEEE Trans Power Syst 2018;33:6630-41.
4. Byers C, Botterud A. Additional capacity value from synergy of variable renewable energy and energy storage. IEEE Trans Sustain Energy 2020;11:1106-9.
5. Ma Y, Hu Z, Song Y. Hour-ahead optimization strategy for shared energy storage of renewable energy power stations to provide frequency regulation service. IEEE Trans Sustain Energy 2022;13:2331-42.
6. Zhu J, Li S, Borghetti A, et al. Review of demand-side energy sharing and collective self-consumption schemes in future power systems[J]. iEnergy 2023. (in press)
7. Li S, Zhu J, Chen Z, Luo T. Double-layer energy management system based on energy sharing cloud for virtual residential microgrid. Applied Energy 2021;282:116089.
8. Gür TM. Review of electrical energy storage technologies, materials and systems: challenges and prospects for large-scale grid storage. Energy Environ Sci 2018;11:2696-767.
9. Li S, Zhu J, Dong H. A novel energy sharing mechanism for smart microgrid. IEEE Trans Smart Grid 2021;12:5475-8.
10. Naderipour A, Abdul-malek Z, Nowdeh SA, Ramachandaramurthy VK, Kalam A, Guerrero JM. Optimal allocation for combined heat and power system with respect to maximum allowable capacity for reduced losses and improved voltage profile and reliability of microgrids considering loading condition. Energy 2020;196:117124.
11. Zhu J, Dong H, Zheng W, Li S, Huang Y, Xi L. Review and prospect of data-driven techniques for load forecasting in integrated energy systems. Applied Energy 2022;321:119269.
Cite This Article
How to Cite
Zhu, J. Preface for “renewable power generation, energy storage, and resource management challenges and solutions”. . J. Smart. Environ. Green. Comput. 2023, 3, 44-6. http://dx.doi.org/10.20517/jsegc.2023.16
Download Citation
Export Citation File:
Type of Import
Tips on Downloading Citation
Citation Manager File Format
Type of Import
Direct Import: When the Direct Import option is selected (the default state), a dialogue box will give you the option to Save or Open the downloaded citation data. Choosing Open will either launch your citation manager or give you a choice of applications with which to use the metadata. The Save option saves the file locally for later use.
Indirect Import: When the Indirect Import option is selected, the metadata is displayed and may be copied and pasted as needed.
About This Article
Copyright
Data & Comments
Data
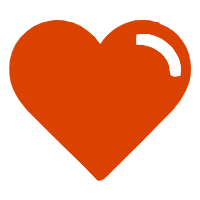
Comments
Comments must be written in English. Spam, offensive content, impersonation, and private information will not be permitted. If any comment is reported and identified as inappropriate content by OAE staff, the comment will be removed without notice. If you have any queries or need any help, please contact us at support@oaepublish.com.