Artificial intelligence streamlines diagnosis and assessment of prognosis in Brugada syndrome: a systematic review and meta-analysis
Abstract
Aim: The objective of this systematic review and meta-analysis was to determine the diagnostic and prognostic utility of artificial intelligence/machine learning (AI/ML) algorithms in Brugada Syndrome (BrS).
Methods: A systematic review and meta-analysis of the literature was conducted in accordance with the Preferred Reporting Items for Systematic reviews and Meta-Analyses (PRISMA) guidelines. MEDLINE, EMBASE, SCOPUS, and WEB OF SCIENCE databases were searched for relevant articles. Abstract and title screening, full-text review, and data extraction were conducted independently by two of the authors. Conflicts were resolved via discussion among authors. A risk-of-bias assessment was performed using the QUADAS-2 tool for diagnostic studies and the PROBAST tool for prognostic studies. Forest plots and the summary area under the receiver operating characteristic (SAUROC) curve were done in R.
Results: A total of 12 papers were included in our study. Among the best-performing diagnostic algorithms from each study, the sensitivity and specificity ranged from 0.80 to 0.89 and 0.74 to 0.97, respectively. In overall studies, sensitivity was 0.845 ± 0.014 and specificity was 0.892 ± 0.062 using a random effects model. A pooled analysis of the summary area under the receiver operating characteristic curve (SAUROC) was 0.77 for diagnostic studies. Prognostic studies showed good performance as well, with the AUC of the best-performing prognostic algorithms ranging from 0.71 to 0.90.
Conclusions: Overall, AI/ML algorithms had high diagnostic and prognostic accuracy. These results highlight the potential of AI/ML algorithms for the diagnosis and prognosis of BrS and permit a choice of the best-performing ML algorithms.
Keywords
INTRODUCTION
Brugada syndrome (BrS) is a rare inherited cardiac channelopathy that can lead to sudden cardiac death (SCD) and/or ventricular tachycardia/fibrillation (VT/VF) in persons with structurally normal hearts[1]. Genetically, it is attributed to loss of function mutations in the SCN5A gene, present in 20% of diagnosed patients. BrS can result in myocardial fibrosis and expression of gap junction proteins, which may be mediated by inflammation[2-4].
BrS is a challenging entity from the perspective of its diagnosis and prediction of the development of serious, potentially fatal arrhythmias. BrS is diagnosed on the basis of a 12-lead ECG in addition to clinical findings. The typical findings of the Brugada pattern on ECG are a pseudo-right bundle branch block and persistent ST-segment elevation in V1 and/or V2[5]. Other ECG findings characteristic of BrS include “J” waves, QT interval prolongation, and increased S wave voltage and duration. Since 40% of patients with BrS present with a normal or non-diagnostic ECG, a drug challenge using a sodium-channel-blocker
Another challenging problem in the management of BrS is the identification of patients at high risk of sudden death who might benefit from the implantation of a cardioverter-defibrillator (ICD). Most individuals with BrS are asymptomatic and have a low risk of sudden death. However, sudden death in BrS occurs in individuals who had been previously asymptomatic. The development of algorithms that would improve the assessment of prognosis is an urgent need. In addition, these models may aid clinicians in the risk stratification of BrS. This review aims to systematically evaluate current AI models for the diagnosis and risk stratification of BrS.
METHODS
Study selection
This review was directed in accordance with the 2020 Preferred Reporting Items for Systematic Reviews and Meta-Analyses[12]. Electronic searches were conducted in MEDLINE, EMBASE, SCOPUS, and WEB OF SCIENCE from database inception to November 6, 2023, with keywords “artificial intelligence” OR
The inclusion criteria were all primary research papers published in English that examined the utility of AI, machine learning, and ECG data in diagnosing or predicting adverse cardiac events in patients with Brugada syndrome. The exclusion criteria were: pediatric patients and non-human studies. Abstracts, editorials, case reports, and reviews were also excluded.
All references were uploaded to Covidence and were electronically merged to remove duplicates[14]. Two authors (CL and SS) individually reviewed studies to determine their inclusion or exclusion. The data extracted from each study were: study design, country in which the study was conducted, AI training cohort size, Brugada sample size, and control sample size. In addition, the following algorithm characteristics were extracted from each study: sensitivity, specificity, positive predictive value, negative predictive value, accuracy, area under the curve (AUC), and F1 score. Two reviewers (CL, JS) examined each paper independently to determine whether they fit the inclusion or exclusion criteria. Data extraction was conducted by two reviewers (CL, JS) and a consensus was reached for any conflicts.
Data analysis
Risk of bias assessment was conducted by CL. The QUADAS-2 tool was used to assess the risk of bias in diagnostic algorithm accuracy studies, whereas the PROBAST tool was used to assess the risk of bias in prognostic algorithm accuracy studies[15,16]. Diagnostic studies were assessed based on the domains of patient selection, index test(s), reference standard, and flow and timing. Prognostic studies were assessed based on the domains of participants, predictors, outcomes, and analysis.
Statistical analysis
Forest plots were used to quantify results and depict the standard difference of means, 95% confidence interval, and P-value. Data analysis was conducted in R using the Meta-Analysis of Diagnostic Accuracy (mada) package[17]. Forest plots and the summary area under the receiver operating characteristic (SAUROC) curve were done in R. The meta-analysis was carried out using Comprehensive Meta-Analysis (Biostat Inc., NJ, USA) by fitting the random effects model with inverse-variance weighting.
RESULTS
One hundred forty-one studies were identified from our search and uploaded to Covidence for screening [Figure 1]. Sixty-one references were marked as duplicates and removed. Seventy-four studies were screened for relevance by title and abstract independently by two authors (CL and SS), and of these, 53 were excluded. 21 studies were eligible for full-text review and screened independently by CL and SS. Nine studies were excluded at this stage for reasons specified in Figure 1. In total, 12 studies were included in our review.
Study characteristics
Studies were conducted in 7 different countries/regions including Italy (n = 3), Switzerland (n = 1),
Diagnostic study characteristics
BrS | Control (BrS negative) | ||||||||
Study ID | Country | Diagnosis | Training sample size | % male | Mean age (± SD) | Training sample size | % male | Mean age (± SD) | |
Micheli et al.[24] (2023) | Italy | Physician Diagnosed | 123 ECGs | NR | NR | 183 ECGs | NR | NR | |
Melo et al.[9] (2023) | Italy | Physician Diagnosed | 596 ECGs | NR | NR | 558 ECGs | NR | NR | |
Zanchi et al.[25] (2023) | Switzerland | 79 were physician diagnosed, 44 underwet ajmaline challenge | 79 | 69.6% | 47 ± 14 | 44 | 63.60% | 36 ± 14 | |
Liu et al.[11] (2022) | Taiwan | Physician verified (cardiologist) | 138 ECGs | NR | NR | 138 ECGs | NR | NR | |
Liao et al.[10] (2022) | Canada | Procainamide or Brugada type 1 ECG pattern in the standard precordial | 105 | 77% | NR | 76 | 53% | NR |
AUC of diagnostic study algorithm
Risk of bias assessment
Diagnostic studies were assessed based on the domains of patient selection, index test(s), reference standard, and flow and timing. Two diagnostic studies were determined to be at high or unclear risk of bias. Prognostic studies were assessed on the domains of participants, predictors, outcomes, and analysis. Four prognostic studies were determined to be at high or unclear risk of bias. Detailed results are provided in Supplementary Tables 3 and 4.
Model testing and validation
ML algorithms were evaluated based on the area under the curve (AUC) of the receiver operating characteristic curve (ROC). Accuracy, positive predictive value (PPV), negative predictive value (NPV), sensitivity, specificity, and F1 were also used as evaluation metrics. Accuracy is defined as the number of overall cases correctly identified, but it may be misleadingly high if the model is trained on an imbalanced dataset. PPV (i.e., precision) is the ratio of predicted positives to true positives. NPV is the ratio of predicted negatives to true negatives. Sensitivity is the model’s ability to identify true positive cases, whereas specificity is the probability that a predicted negative is truly negative. F1 score is the harmonic mean of precision and recall, balancing the two metrics.
Notably, these metrics are dependent on a defined threshold value, which determines the classification boundary between positive and negative cases. A higher threshold may increase sensitivity at the cost of specificity. Conversely, a lower threshold may decrease sensitivity at the cost of specificity. Threshold selection techniques varied between selecting an optimal value based on the ROC curve, using optimal precision vs recall, Youden’s J statistic, and using predefined sensitivity values [Supplementary Table 4].
The AUC of the best-performing diagnostic study algorithms ranged from 0.934-0.976. The AUC of the best-performing prognostic study algorithms ranged from 0.7092 to 0.942. However, the included studies did not consistently report all of the metrics, with several studies not reporting both AUC and a 95%CI.
Different studies used a variety of machine learning algorithms. Lee et al. trained a random forest model to predict spontaneous VT/VF on latent risk factors extracted by non-negative matrix factorization (NMF)[18]. The total sample size was 516 and included 314 asymptomatic patients. Liao et al. trained several convolutional neural networks (CNN) to identify and diagnose the type 1 Brugada ECG pattern[10]. The highest-performing algorithm was the convolutional deep neural network (DNN) trained on 12-lead ECG data, which had an AUC of 0.976 (96%CI: 0.973-0.979). Liu et al. used a learning transfer strategy on a model originally used to classify right bundle branch block (RBBB) and adapted it to classify the type 1 Brugada pattern[11]. Melo et al. trained a DNN on 12-lead ECG data in a cohort of 1,154 patients (596 BrS positive, 558 controls)[9]. Only a small fraction of patients showed a type 1 Brugada pattern and these patients were identified with 100% accuracy. Randazzo et al. trained two models, a multi-layer perceptron neural network (MLP) and a boosted decision tree (BDT), on ECG features extracted manually by cardiologists to predict retrospective arrhythmic events[20]. Tse et al. trained a regression model with latent variables extracted by NMF to predict spontaneous VT/VF incidence[21]. These included clinical variables such as syncope and AF as well as ECG variables such as type 1 Brugada pattern, QRS duration, QTc interval and others. When validated on an external cohort from multiple different countries, they found that the model’s performance was optimal when trained on five latent variables. Romero et al. trained an ensemble classifier to distinguish BrS patients according to symptomatology using features extracted from the QRS complex, HRV markers, or both[22]. Romero et al. utilized a multivariate ensemble classifier trained on ECG data for risk stratification in 110 BrS patients, of which 25 showed symptoms[23]. Lee et al. compared the performance of 7 different machine learning models with respect to the prognosis of VT/VF[19].
Diagnostic algorithm performance
Five studies used ML algorithms for the diagnosis of BrS [Tables 3 and 4]. Micheli et al. used a CNN trained on ECG data for the diagnosis of BrS on a dataset of 306 ECGs from the BrAID (Brugada syndrome and Artificial Intelligence applications to Diagnosis) project[24]. The model showed excellent performance with a sensitivity of 0.8773 and a specificity of 0.9234. Melo et al. trained a DNN on a cohort of 596 BrS-positive and 558 control patients[9]. On an external validation cohort of 370 ECGs, the model demonstrated good performance in diagnosing BrS without the use of a SCB (0.934 AUC, 95%CI: 0.973-0.979). Zanchi et al. compared various ML models trained on P-wave features for the diagnosis of BrS in a cohort of 123 patients[25]. The worst-performing model was the K-nearest neighbors’ model, with a reasonable sensitivity (0.843) but poor specificity (0.513). The best-performing model was the AdaBoost model, with a sensitivity of 0.865 and specificity of 0.738. Liu et al. compared the performance of a deep-learning model with that of two cardiologists in the diagnosis of BrS based on ECG[11]. The model showed higher sensitivity
Test accuracy of diagnostic algorithms study
Algorithm | F1 | Sensitivity (aka recall) | Specificity | NPV | PPV (aka precision) | Accuracy | |
Micheli et al.[24] (2023) | Convolutional neural network (6 blocks V2) | NR | 0.8773 | 0.9234 | NR | NR | 0.9053 |
CNN (6 blocks V1) | NR | 0.8536 | 0.8581 | NR | NR | 0.8562 | |
CNN (6 blocks V1, V2) | NR | 0.8987 | 0.8943 | NR | NR | 0.902 | |
Melo et al.[9] (2023) | Deep Neural Network | NR | 0.796 | 0.936 | 0.813 | 0.609 | 0.884 |
Zanchi et al.[22] (2023) | K nearest neighbors | MF1 = 0.681, WF1 = 0.711 | 0.843 | 0.513 | NR | NR | 0.725 |
Decision tree (with Adasyn) | MF1 = 0.661, WF1 = 0.668 | 0.562 | 0.855 | NR | NR | 0.663 | |
Random forest (with SMOTE) | MF1 = 0.765, WF1 = 0.784 | 0.824 | 0.721 | NR | NR | 0.783 | |
Stacking (with SMOTE) | MF1 = 0.780, WF1 = 0.799 | 0.902 | 0.498 | NR | NR | 0.798 | |
Support vector machining (with SMOTE) | MF1 = 0.704, WF1 = 0.722 | 0.717 | 0.734 | NR | NR | 0.716 | |
Majority voting | MF1 = 0.692, WF1 = 0.721 | 0.799 | 0.581 | NR | NR | 0.723 | |
Bagging | MF1 = 0.780, WF1 = 0.799 | 0.836 | 0.736 | NR | NR | 0.798 | |
AdaBoost (with Weighted class) | MF1 = 0.795, WF1 = 0.814 | 0.865 | 0.738 | NR | NR | 0.814 | |
GBoost (with SMOTE) | MF1 = 0.771, WF1 = 0.789 | 0.811 | 0.754 | NR | NR | 0.788 | |
Liu et al.[11] (2022) | Deep learning model | 0.887 (0.899-0.940) | 0.884 (0.819-0.942) | 0.891 | NR | NR | NR |
Liao et al.[10] (2022) | Convolutional deep neural network (12-lead ECG) | 0.672 | 0.5 | 1 | 0.905 | 1 | NR |
Convolutional deep neural network (12-lead ECG) | 0.833 | 0.8 | 0.972 (0.95-0.994) | 0.96 (0.959-0.960) | 0.862 (0.762-0.954) | NR | |
Convolutional deep neural network (12-lead ECG) | 0.77 | 0.9 | 0.905 | 0.973 | 0.672 | NR | |
Convolutional deep neural network (12-lead Holter) | 0.629 | 0.5 | 0.993 | 0.973 | 0.817 | NR | |
Convolutional deep neural network (12-lead Holter) | 0.694 | 0.8 | 0.968 | 0.989 | 0.603 | NR | |
Convolutional deep neural network (12-lead Holter) | 0.632 | 0.9 | 0.942 | 0.995 | 0.482 | NR |
Prognostic study characteristics
Outcome | Control | |||||||||||
Study ID | Country | Outcome predicted | Training sample size | % male | Mean age (± SD) | Sample size | % male | Mean age (± SD) | ||||
Tse et al.[21] (2020) | Hong Kong | VT/VF | 32 | 95% | 49 (35-68) Median, LQ, UQ | 117 | 81% | 50 (39-59) Median, LQ, UQ | ||||
Romero et al.[22] (2016) | France | Syncope, VF, or SCD | 14 | NR | NR | 48 | NA | NA | ||||
Randazzo et al.[20] (2023) | Italy | SCD or VF | 41 ECGs | NR | NR | 168 ECGs | NR | NR | ||||
Lee et al.[18] (2021) | Hong Kong | VT/VF | 516 | 92% | 50 ± 16 | NA | NA | NA | ||||
Romero et al.[23] (2022) | France | Syncope, VF, or SCD | 25 | Total training: 74.5% male, 25.5% female (not reported for individual groups) | Total training: 44.6 ± 13.7 | 85 | NA | NA | ||||
Lee et al.[19] (2022) | Hong Kong | VT/VF | 548 | 92.70% | 49.9 ± 16.3 | NA | NA | NA | ||||
Nakamura et al.[26] (2023) | Japan | Fatal arrhythmia | 157 | 90.40% | 44.8 ± 14.8 | NA | NA | NA |
Overall, ML algorithms for the diagnosis of BrS via ECG data showed good performance with regard to sensitivity and specificity. We performed a pooled analysis of the best-performing algorithm from each study. The sensitivity and specificity of the best-performing diagnostic algorithms ranged from 0.80 to 0.89 and 0.74 to 0.97, respectively. A meta-analysis showed that overall studies sensitivity was 0.848 ± 0.015 (SEM, z = 57.3 m, P < 0.0001) [Figure 2] and specificity was 0.892 ± 0.061 (SEM, z = 14.5, P < 0.0001) using a random effects model [Figure 3]. An analysis for publication bias using the classic Failsafe-N test would require over 7,000 negative studies to invalidate the result for sensitivity and over 2,000 negative studies to invalidate the result for specificity.
Figure 2. Forest plot of sensitivity of diagnostic studies. Error bars represent 95% confidence intervals.
Figure 3. Forest plot of specificity of diagnostic studies. Error bars represent 95% confidence intervals.
Since the majority of studies did not explicitly report 2 × 2 contingency tables, these were imputed algebraically from their data, where necessary, using sensitivity, specificity, sample size, and number of condition-positive patients. The heterogeneity of studies was assessed using Chi-squared tests for equality of sensitivities and specificities (Test for equality of sensitivities: X-squared = 7.4429, df = 4, P-value = 0.114; Test for equality of specificities: X-squared = 79.9133, df = 4, P-value ≤ 2 × 10-16). This suggests that there are significant differences in specificity but not sensitivity among diagnostic studies. Next, a bivariate approach was used to calculate the pooled SROC. Using the mada package in R, we fit a bivariate diagnostic random-effects meta-analysis[17]. Among five diagnostic studies, the overall pooled summary area under the receiver operator characteristic curve (SAUROC) for diagnosis of BrS was 0.877 [Figure 4]. The SAUROC represents the pooled AUC of all the included studies. It was calculated by combining the true positive rates and false positive rates from the included studies and plotting them against each other. A higher SAUROC represents greater diagnostic/prognostic accuracy across several ML models and datasets.
Prognostic algorithm performance
The AUC of the best-performing prognostic algorithms ranged from 0.71-0.90 for five of seven studies that reported it [Tables 5 and 6]. Unlike the diagnostic studies, the sensitivity and specificity were only reported for four of the seven studies, so a pooled analysis was not possible. Tse et al. utilized a logistic regression model trained on latent variables extracted via a non-negative matrix factorization method to predict
AUC of prognostic study algorithm
Study | Algorithm | AUC | 95%CI |
Tse et al.[21] (2020) | Benchmark using logistic regression (# latent variables = 0) | 0.6383 | NR |
NMF (# latent variables = 2) | 0.6759 | NR | |
NMF (# latent variables = 3) | 0.6809 | NR | |
NMF (# latent variables = 4) | 0.6993 | NR | |
NMF (# latent variables = 5) | 0.7092 | NR | |
NMF (# latent variables = 6) | 0.6856 | NR | |
Romero et al.[22] (2016) | Ensemble classifier (HRV-based model) | 0.87 | NR |
Ensemble classifier (QRS-based model) | 0.73 | NR | |
Ensemble classifier (HRV + QRS combination based model) | 0.9 | NR | |
Randazzo et al.[20] (2023) | NR | NR | NR |
Lee et al.[18] (2021) | Model 1 (multivariate classifier with 9 features) | 0.819 | 0.756-0.882 |
Model 2 (multivariate classifier with 7 features) | 0.817 | 0.741-0.893 | |
Romero et al.[23] (2022) | Model 3 (multivariate classifier with 3 features) | 0.796 | 0.719-0.873 |
Lee et al.[19] (2022) | Random survival forest | 0.942 | 0.913-0.964 |
Ada boost classifier | 0.872 | 0.831-0.923 | |
Gaussian naive Bayes | 0.832 | 0.803-0.861 | |
Light gradient boosting machine | 0.812 | 0.781-0.831 | |
Random forest classifier | 0.783 | 0.764-0.821 | |
Gradient boosting classifier | 0.762 | 0.751-0.802 | |
Decision tree classifier | 0.683 | 0.651-0.713 | |
Nakamura et al.[26] (2023) | CNN (Average of 5-fold cross validation on an ECG basis) | 0.8 | 0.73-0.87 |
CNN (Average of 5-fold cross validation on an patient basis) | 0.81 | 0.72-0.90 |
Test accuracy of prognostic algorithms
Study | Algorithm | F1 | Sensentivity (aka recall) | Specificity | NPV | PPV (aka precision) | Accuracy |
Tse et al.[21] (2020) | Benchmark using logistic regression (# latent variables = 0) | 0.6056 | 0.6131 | NR | NR | 0.5983 | NR |
NMF (# latent variables = 2) | 0.6559 | 0.6552 | NR | NR | 0.6567 | NR | |
NMF (# latent variables = 3) | 0.6769 | 0.6567 | NR | NR | 0.6984 | NR | |
NMF (# latent variables = 4) | 0.6973 | 0.6899 | NR | NR | 0.7048 | NR | |
NMF (# latent variables = 5) | 0.7048 | 0.696 | NR | NR | 0.7139 | NR | |
NMF (# latent variables = 6) | 0.6925 | 0.6738 | NR | NR | 0.7123 | NR | |
Romero et al.[22] (2016) | Ensemble classifier (HRV-based model) | NR | 1 | 0.67 | NR | NR | NR |
Ensemble classifier (QRS-based model) | NR | 0.75 | 0.67 | NR | NR | NR | |
Ensemble classifier (HRV + QRS combination based model) | NR | 1 | 0.83 | NR | NR | NR | |
Randazzo et al.[20] (2023) | Boosted decision tree (BDT) | 0.67 | NR | NR | 0.8947 | 1 | 0.9048 |
Multi-layer perceptron neural network (MLP) | 0.27 | NR | NR | 0.8333 | 0.5 | 0.8095 | |
MLP opt. threshold | 0.43 | NR | NR | 0.8979 | 0.3143 | 0.6547 | |
Decision tree | 0.35 | NR | NR | 0.839 | 0.9 | 0.842 | |
Naive bayes | 0.45 | NR | NR | 0.857 | 0.577 | 0.823 | |
Support vector machine | 0.18 | NR | NR | 0.819 | 1 | 0.823 | |
Lee et al.[18] (2021) | Cox model | 0.742 | 0.728 | NR | NR | 0.7565 | NR |
RSF model | 0.8433 | 0.8531 | NR | NR | 0.8338 | NR | |
RSF-NMF model | 0.8769 | 0.8881 | NR | NR | 0.8712 | NR | |
Romero et al.[23] (2022) | Model 1 (multivariate classifier with 9 features) | NR | 0.791 ± 0.087 | 0.796 ± 0.0103 | NR | NR | NR |
Model 2 (multivariate classifier with 7 features) | NR | 0.850 ± 0.111 | 0.777 ± 0.076 | NR | NR | NR | |
Model 3 (multivariate classifier with 3 features) | NR | 0.853 ± 0.106 | 0.724 ± 0.096 | NR | NR | NR | |
Lee et al.[19] (2022) | NR | ||||||
Nakamura et al.[26] (2023) | CNN (Average of 5-fold cross validation on an ECG basis) | 0.75 ± 0.09 | 0.73 ± 0.09 | NR | 0.87 ± 0.06 | 0.49 ± 0.22 | 0.73 ± 0.09 |
CNN (Average of 5-fold cross validation on an patient basis) | 0.81 ± 0.11 | 0.77 ± 0.14 | NR | 0.94 ± 0.11 | 0.44 ± 0.29 | 0.77 ± 0.14 |
Nakamura et al. trained a CNN for the prediction of fatal arrhythmia. The model performed the best when trained on a per-patient basis, showing an AUC of 0.81 (95%CI: 0.72-0.90)[26].
DISCUSSION
In this systematic review and meta-analysis, we evaluated the performance of ML algorithms in diagnosing BrS and predicting adverse cardiac events. Overall, the pooled estimation showed that ML algorithms performed well in diagnosing BrS and predicting adverse cardiac events, but there are meaningful differences between different algorithms.
Considering the high accuracy of ML algorithms in diagnosing BrS and the shortcomings associated with SCBs, implementing ML algorithms in a clinical setting could streamline diagnosis and help identify ECGs with Brugada patterns. The diagnostic algorithm with the highest performance as measured by AUC and combination of sensitivity and specificity was the convolutional DNN based on 12-lead ECG proposed by Liao et al.[10]. This algorithm had an AUC of 0.976 (95%CI: 0.973-0.979) and sensitivity and specificity of 0.8 and 0.972, respectively. In a follow-up random sample of patients from the 50 ECGs testing cohort, the ML model performed just as well as cardiologists, scoring a sensitivity and specificity of 96% and 90% compared with cardiologist 1 (sensitivity = 88.9%, specificity = 88.0%) and cardiologist 2 (sensitivity = 92.5%,
One of the most challenging aspects for clinicians in the management of BrS patients is risk stratification, as many cases are asymptomatic and present with a Brugada pattern on ECG. Patients with a previous history of syncope or aborted cardiac arrest have a high risk for sustained VT/VF. The risk of VT is 1.9%-8.8% and 7.7%-13.8% for VF[27,28]. However, risk stratification in patients with no previous history of cardiac events is less clear. Thus, AI may be a valuable tool to aid clinicians in assessing prognosis and deciding which patients need an ICD. Regarding prognostic algorithms, the Ensemble classifier trained on QRS and HRV data was the top performer with an AUC of 0.90, sensitivity of 1, and specificity of 0.83 in determining the risk of VF, SCD, or syncope[22]. This suggests that a combination of QRS morphology and HRV markers is suitable for the classification of BrS patients based on symptomatology. The second best-performing prognostic algorithm was the Gaussian naïve Bayes model used by Lee et al.[19], with an AUC of 0.832 (95%CI: 0.803-0.861) in its prediction of VT/VF. Sensitivity and specificity values were not reported in that study.
Integration of clinical factors and ECG patterns in AI models
Clinical factors can play a valuable role in enhancing the prognostic accuracy of ML algorithms. An interesting approach was that of Tse et al. who used an NMF method to extract latent features, which are relationships between clinical variables that were only discoverable after applying a dimensionality reduction technique[21]. These latent features were then incorporated into the training of their ML model. Clinical factors associated with spontaneous VT/VF included syncope, AF, QRS duration, and QTc interval prolongation. Additionally, Lee et al. found that symptoms on initial presentation were statistically significant predictors of VT/VF during follow-up[18]. Patients presenting with syncope or VT/VF were at increased risk for spontaneous VT/VF during follow-up at every time point. Lastly, Lee et al. performed a Cox regression using a multivariate model and found that syncope, initial VT/VF, other arrhythmias, and significant S wave in lead I were statistically significant predictors of VT/VF during follow-up[19].
BrS and multi-modal training in medical AI models
Recently, there has been much progress in the integration of different data modalities in the training of diagnostic algorithms. For instance, Contrastive Language-Image Pre-Training (CLIP) models connect medical imaging (X-ray, MRI, CT, etc.) to medical descriptions and notes[29]. This integration allows CLIP models to assist in automated diagnosis and medical research - both pertinent to the diagnosis of BrS. There are two types of CLIP models: (1) Medical Vision-Language Pre- Training (MED-VLP) with Frozen Language Models and Latent Space Geometry Optimization (M-FLAG) and (2) Unifying Cross-Lingual Medical Vision-Language Pre-Training by Diminishing Bias (MED-UniC). M-FLAG frozen language models are pre-trained on large data sets and then are fine-tuned to accomplish specific tasks[30]. This approach makes it easier to train the model on specific functions. M-FLAG utilizes Latent Space Geometry Optimization, a technique that optimizes the space in which data are projected. Effective space manipulation leads to improved model performance by ensuring representations of both text and image modalities are compatible and can be efficiently combined to make diagnostic predictions. In contrast, MED-UniC models involve medical data streams from multiple sources of data, such as imaging (e.g., radiography) and text data (e.g., consult notes)[31]. Medical vision and language pre-training (MED-VLP) hopes to integrate and jointly process these data to generalize representations from large-scale medical image-text data. Subsequently, it enables a vision-and-language model to address a wide range of medical vision-and-language tasks, which can be crucial for mitigating the data scarcity problem in the medical field and aid in integrating the knowledge from pictures and text. The current literature on AI algorithms for the diagnosis and prognosis of BrS mainly incorporates only ECG data (and sometimes clinical data). The high accuracy of multi-modal AI models highlights the potential of integrating CLIP models in the
AI in the diagnosis of other cardiac diseases
The utility of AI and ML in diagnosing other cardiac conditions strengthens the case for using AI models in the diagnosis and prognosis of BrS. AI and ML have helped characterize different types of heart failure with preserved ejection fraction[32], AI-enabled ECG-based screening tool for the diagnosis of left ventricular systolic dysfunction[33], and prediction of atrial fibrillation[34]. ECG-based ML algorithms are being used for the diagnosis of other inherited arrhythmias, such as long QT syndrome (LQTS)[35]. They found that among eight studies, the pooled SAUROC was 0.95 (95%CI: 0.31-1.00), sensitivity was 0.87 (95%CI: 0.83-0.90), and specificity was 0.91 (95%CI: 0.88-0.93), indicating good diagnostic performance. These metrics were slightly higher than the SAUROC calculated in our review, suggesting that ML algorithms may perform better for diagnosing LQTS compared to BrS. Another interpretation of this comparison is that algorithms for the diagnosis of BrS may not yet be well optimized, and further work must be done with larger datasets to attain higher diagnostic accuracy.
Strengths and limitations
Our study had several strengths. A thorough search of the literature and in-depth analysis was conducted. Included studies explored a variety of different machine learning algorithms. A pooled analysis was conducted to evaluate the SROC of diagnostic studies, which indicated that ML algorithms perform well in the diagnosis of BrS.
The primary limitations of our study are outlined. (1) Prognostic studies did not consistently report both sensitivity and specificity, so a pooled analysis of prognostic studies was not possible; (2) Several studies displayed an unclear or high risk of bias. There were a few reasons for this conclusion; primarily, these studies did not report patient inclusion/exclusion criteria, demographic characteristics of the patients, or the method used to diagnose BrS. Additionally, although most models underwent internal cross-validation, few were externally validated with other datasets. Therefore, there is the possibility of overfitting of the models; (3) Since BrS is a relatively rare disease, the total sample size of all included studies was not high (n = 1,868 for diagnostic studies and n = 1,859 for prognostic studies), which may limit the generalizability of our findings; (4) Clinically, BrS is often diagnosed after a drug challenge with a SCB which can sometimes unmask the type-1 BrS ECG pattern, aiding in diagnosis[36]. Our meta-analysis was unable to stratify patients based on whether or not they received a drug challenge as there were insufficient data reported on whether this procedure was used; (5) Some studies explored the utility of ML algorithms for diagnosis/prognosis of BrS mainly based on ECG data alone. Other non-ML risk score models that incorporate clinical risk factors and ECG features exist, but these were not employed. For instance, the Shanghai scoring system incorporates ECG features, clinical history, and family history[37]. The Sieira score is based on ECG pattern, in addition to family history of SCD and clinical presentation (e.g., syncope or aborted SCD)[38]. Future ML models should be trained on ECG data and clinical risk factors to achieve optimal performance; (6) Another limitation is the lack of reporting on survival data according to age and time of diagnosis. Thus, we were not able to construct survival curves merging data from all studies; (7) A major concern with the use of ML algorithms as a diagnostic/prognostic tool is the potential bias in data collection for training of the model. Overrepresentations of specific demographic groups, such as by ethnic/racial groups, age groups, or gender, may also lead to overfitting of the model and loss of generalizability to other populations. For instance, the vast majority of our sample consisted of patients who were males of European descent. Therefore, caution may be necessary when interpreting the accuracy or applicability of models trained on datasets that lack diversity. Additionally, all included studies trained models on retrospective cohorts, which could serve as another source of bias. Performance metrics of models should be interpreted cautiously until the models can be validated on more robust, prospectively collected validation datasets. In the development of future models, care should be taken to address bias in data collection for model training in terms of population and data quality; (8) Lastly, since models are usually trained on high-quality databases and ECGs of
CONCLUSION
This systematic review and meta-analysis demonstrated the utility of AI/ML algorithms for the diagnosis and prognosis of BrS. Pooled analysis of AUC demonstrated good diagnostic performance of BrS according to ECG algorithms. These findings have clinical relevance because they suggest that the use of AI/ML in a care setting may help clinicians streamline diagnosis and risk stratification in BrS patients. Future research is needed to directly compare the performance of each AI/ML algorithm using the same robust dataset and ascertain their clinical utility.
DECLARATIONS
Authors’ contributions
Conceptualization, data extraction and analysis, and manuscript writing: Leong CJ
Data extraction and manuscript writing: Sharma S
Data extraction and manuscript writing: Seth J
Conceptualization, supervision, and manuscript writing: Rabkin SW
Availability of data and materials
Not applicable.
Financial support and sponsorship
Not applicable.
Conflicts of interest
All authors declared that there are no conflicts of interest.
Ethical approval and consent to participate
Not applicable.
Consent for publication
Not applicable.
Copyright
© The Author(s) 2024.
Supplementary Materials
REFERENCES
1. Sarquella-Brugada G, Campuzano O, Arbelo E, Brugada J, Brugada R. Brugada syndrome: clinical and genetic findings. Genet Med 2016;18:3-12.
2. Nademanee K, Raju H, de Noronha SV, et al. Fibrosis, connexin-43, and conduction abnormalities in the brugada syndrome. J Am Coll Cardiol 2015;66:1976-86.
3. Pieroni M, Notarstefano P, Oliva A, et al. Electroanatomic and pathologic right ventricular outflow tract abnormalities in patients with brugada syndrome. J Am Coll Cardiol 2018;72:2747-57.
4. Kapplinger JD, Tester DJ, Alders M, et al. An international compendium of mutations in the SCN5A-encoded cardiac sodium channel in patients referred for Brugada syndrome genetic testing. Heart Rhythm 2010;7:33-46.
5. Antzelevitch C, Brugada P, Borggrefe M, et al. Brugada syndrome: report of the second consensus conference: endorsed by the heart rhythm society and the European heart rhythm association. Circulation 2005;111:659-70.
6. Batchvarov VN. The brugada syndrome - diagnosis, clinical implications and risk stratification. Eur Cardiol 2014;9:82-7.
7. Poli S, Toniolo M, Maiani M, et al. Management of untreatable ventricular arrhythmias during pharmacologic challenges with sodium channel blockers for suspected Brugada syndrome. Europace 2018;20:234-42.
8. Conte G, Sieira J, Sarkozy A, et al. Life-threatening ventricular arrhythmias during ajmaline challenge in patients with Brugada syndrome: incidence, clinical features, and prognosis. Heart Rhythm 2013;10:1869-74.
9. Melo L, Ciconte G, Christy A, et al. Deep learning unmasks the ECG signature of Brugada syndrome. PNAS Nexus 2023;2:pgad327.
10. Liao S, Bokhari M, Chakraborty P, et al. Use of wearable technology and deep learning to improve the diagnosis of brugada syndrome. JACC Clin Electrophysiol 2022;8:1010-20.
11. Liu CM, Liu CL, Hu KW, et al. A deep learning-enabled electrocardiogram model for the identification of a rare inherited arrhythmia: brugada syndrome. Can J Cardiol 2022;38:152-9.
12. Page MJ, McKenzie JE, Bossuyt PM, et al. The PRISMA 2020 statement: an updated guideline for reporting systematic reviews. PLoS Med 2021;18:e1003583.
13. Campbell S, Kung J. Filter to retrieve studies related to artificial intelligence from the OVID EMBASE database. Available from: https://docs.google.com/document/d/1eWyO0jv9_6FYsxyC5LUYwFe9eH_3h83-tPNZ6wmos18/edit#heading=h.ldbxqb34y1kj [Last accessed on 5 Jun 2024].
14. Innovation VH. Covidence systematic review software. Available from: http://www.covidence.org [Last accessed on 5 Jun 2024]
15. Whiting PF, Rutjes AW, Westwood ME, et al. QUADAS-2 Group. QUADAS-2: a revised tool for the quality assessment of diagnostic accuracy studies. Ann Intern Med 2011;155:529-36.
16. Wolff RF, Moons KGM, Riley RD, et al. PROBAST Group†. PROBAST: a tool to assess the risk of bias and applicability of prediction model studies. Ann Intern Med 2019;170:51-8.
17. Doebler P, Holling H. Meta-analysis of diagnostic accuracy with mada. Available from: https://cran.r-project.org/web/packages/mada/vignettes/mada.pdf [Last accessed on 5 Jun 2024].
18. Lee S, Zhou J, Li KHC, et al. Territory-wide cohort study of brugada syndrome in Hong Kong: predictors of long-term outcomes using random survival forests and non-negative matrix factorisation. Open Heart 2021;8:e001505.
19. Lee S, Zhou J, Chung CT, et al. Comparing the performance of published risk scores in brugada syndrome: a multi-center cohort study. Curr Probl Cardiol 2022;47:101381.
20. Randazzo V, Marchetti G, Giustetto C, et al. Learning-based approach to predict fatal events in brugada syndrome. In: Esposito A, Faundez-Zanuy M, Morabito FC, Pasero E, eds. Applications of Artificial Intelligence and Neural Systems to Data Science. Smart Innovation, Systems and Technologies. Springer Nature; 2023:63-72.
21. Tse G, Zhou J, Lee S, et al. Incorporating latent variables using nonnegative matrix factorization improves risk stratification in brugada syndrome. J Am Heart Assoc 2020;9:e012714.
22. Romero D, Calvo M, Behar N, Mabo P, Hernandez A. Ensemble classifier based on linear discriminant analysis for classifying Brugada syndrome patients according to symptomatology. Available from: https://ieeexplore.ieee.org/document/7868715 [Last accessed on 5 Jun 2024].
23. Romero D, Calvo M, Le Rolle V, Béhar N, Mabo P, Hernández A. Multivariate ensemble classification for the prediction of symptoms in patients with Brugada syndrome. Med Biol Eng Comput 2022;60:81-94.
24. Micheli A, Natali M, Pedrelli L, et al. Analysis and interpretation of ECG time series through convolutional neural networks in Brugada syndrome diagnosis. In: Iliadis L, Papaleonidas A, Angelov P, Jayne C, editors. Artificial Neural Networks and Machine Learning – ICANN 2023. Cham: Springer Nature Switzerland; 2023. pp. 26-36.
25. Zanchi B, Faraci FD, Gharaviri A, et al. Identification of Brugada syndrome based on P-wave features: an artificial intelligence-based approach. Europace 2023:25.
26. Nakamura T, Aiba T, Shimizu W, Furukawa T, Sasano T. Prediction of the presence of ventricular fibrillation from a Brugada electrocardiogram using artificial intelligence. Circ J 2023;87:1007-14.
27. Brugada J, Brugada R, Antzelevitch C, Towbin J, Nademanee K, Brugada P. Long-term follow-up of individuals with the electrocardiographic pattern of right bundle-branch block and ST-segment elevation in precordial leads V1 to V3. Circulation 2002;105:73-8.
28. Kusano KF, Taniyama M, Nakamura K, et al. Atrial fibrillation in patients with Brugada syndrome relationships of gene mutation, electrophysiology, and clinical backgrounds. J Am Coll Cardiol 2008;51:1169-75.
29. Radford A, Kim JW, Hallacy C, et al. Learning transferable visual models from natural language supervision. Available from: https://arxiv.org/abs/2103.00020v1 [Last accessed on 5 Jun 2024].
30. Liu C, Cheng S, Chen C, et al. M-FLAG: medical vision-language pre-training with frozen language models and latent space geometry optimization. Available from: https://arxiv.org/abs/2307.08347 [Last accessed on 5 Jun 2024].
31. Zhang K, Yang Y, Yu J, et al. Multi-task paired masking with alignment modeling for medical vision-language pre-training. IEEE Trans Multimedia 2024;26:4706-21.
32. Rabkin SW. Evaluating the adverse outcome of subtypes of heart failure with preserved ejection fraction defined by machine learning: a systematic review focused on defining high risk phenogroups. EXCLI J 2022;21:487-518.
33. Yao X, McCoy RG, Friedman PA, et al. ECG AI-guided screening for low ejection fraction (EAGLE): rationale and design of a pragmatic cluster randomized trial. Am Heart J 2020;219:31-6.
34. Khurshid S, Friedman S, Reeder C, et al. ECG-based deep learning and clinical risk factors to predict atrial fibrillation. Circulation 2022;145:122-33.
35. Wu MJ, Wang WQ, Zhang W, Li JH, Zhang XW. The diagnostic value of electrocardiogram-based machine learning in long QT syndrome: a systematic review and meta-analysis. Front Cardiovasc Med 2023;10:1172451.
36. Monasky MM, Micaglio E, D'Imperio S, Pappone C. The mechanism of ajmaline and thus brugada syndrome: not only the sodium channel! Front Cardiovasc Med 2021;8:782596.
37. Priori SG, Wilde AA, Horie M, et al. Document Reviewers; Heart Rhythm Society; European Heart Rhythm Association; Asia Pacific Heart Rhythm Society. Executive summary: HRS/EHRA/APHRS expert consensus statement on the diagnosis and management of patients with inherited primary arrhythmia syndromes. Europace 2013;15:1389-406.
38. Sieira J, Conte G, Ciconte G, et al. A score model to predict risk of events in patients with brugada syndrome. Eur Heart J 2017;38:1756-63.
Cite This Article
Export citation file: BibTeX | EndNote | RIS
OAE Style
Leong CJ, Sharma S, Seth J, Rabkin SW. Artificial intelligence streamlines diagnosis and assessment of prognosis in Brugada syndrome: a systematic review and meta-analysis. Conn Health Telemed 2024;3:300005. http://dx.doi.org/10.20517/chatmed.2024.03
AMA Style
Leong CJ, Sharma S, Seth J, Rabkin SW. Artificial intelligence streamlines diagnosis and assessment of prognosis in Brugada syndrome: a systematic review and meta-analysis. Connected Health And Telemedicine. 2024; 3(2): 300005. http://dx.doi.org/10.20517/chatmed.2024.03
Chicago/Turabian Style
Cameron J. Leong, Sohat Sharma, Jayant Seth, Simon W. Rabkin. 2024. "Artificial intelligence streamlines diagnosis and assessment of prognosis in Brugada syndrome: a systematic review and meta-analysis" Connected Health And Telemedicine. 3, no.2: 300005. http://dx.doi.org/10.20517/chatmed.2024.03
ACS Style
Leong, CJ.; Sharma S.; Seth J.; Rabkin SW. Artificial intelligence streamlines diagnosis and assessment of prognosis in Brugada syndrome: a systematic review and meta-analysis. Conn. Health. Telemed. 2024, 3, 300005. http://dx.doi.org/10.20517/chatmed.2024.03
About This Article
Special Issue
Copyright
Data & Comments
Data
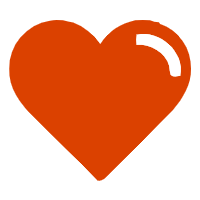
Comments
Comments must be written in English. Spam, offensive content, impersonation, and private information will not be permitted. If any comment is reported and identified as inappropriate content by OAE staff, the comment will be removed without notice. If you have any queries or need any help, please contact us at support@oaepublish.com.