The pivotal role of data harmonization in revolutionizing global healthcare: a framework and a case study
Abstract
Aim: Data harmonization standardizes healthcare information, enhancing accessibility and interoperability, which is crucial for improving patient outcomes and driving medical research and innovation. It enables precise diagnoses and personalized treatments, and boosts AI model efficiency. However, significant challenges such as ethical concerns, technical barriers in the data lifecycle, AI biases, and varied regional regulations impede progress, underscoring the need for solutions like adopting universal standards such as HL7 FHIR, where the lack of generalized harmonization efforts is significant.
Methods: We propose an advanced, holistic framework that utilizes FAIR-compliant reference ontologies (based on the FAIRplus and FAIR CookBook criteria) to make data findable, accessible, interoperable, and reusable enriched with terminologies from OHDSI (Observational Health Data Sciences and Informatics) vocabularies and word embeddings to identify lexical and conceptual overlaps across heterogeneous data models.
Results: The proposed approach was applied to autoimmune diseases, cardiovascular diseases, and mental disorders using unstructured data from EU cohorts involving 7,551 patients with primary Sjogren’s Syndrome, 25,000 patients with cardiovascular diseases, and 3,500 patients with depression and anxiety. Metadata from these datasets were structured into dictionaries and linked with three newly developed reference ontologies (ROPSS, ROCVD, and ROMD), which are accessible on GitHub. These ontologies facilitated data interoperability across different systems and helped identify common terminologies with high precision within each domain.
Conclusion: Through the proposed framework, we aim to urge the adoption of data harmonization as a priority, emphasizing the need for global cooperation, investment in technology and infrastructure, and adherence to ethical data usage practices toward a more efficient and patient-centered global healthcare system.
Keywords
INTRODUCTION
The need for data harmonization in healthcare is a multidimensional challenge with profound implications for the future of healthcare[1-3]. The primary objective of harmonizing medical data is to enhance patient outcomes. “When data from various healthcare sources are harmonized, it enables more accurate and comprehensive diagnoses, personalized treatment plans, and overall improved patient care”. This is because consistent and comparable data from different sources provide healthcare professionals with a more holistic view of a patient's health, leading to better-informed medical decisions. Moreover, the role of harmonized and FAIRified data in advancing medical research and innovation cannot be overstated. Researchers require large, diverse datasets to conduct robust studies. “Harmonized data facilitate the amalgamation and analysis of information from diverse sources, thereby accelerating the discovery of new treatments and healthcare technologies”. It also ensures that the findings of such research are more representative and widely applicable. Moreover, harmonized data can facilitate collaborative international research efforts to address global health challenges. The ability to analyze data from global regions offers invaluable insights into the discovery of effective treatment strategies. According to Figure 1, the major challenges in data harmonization include: (i) ethical and privacy concerns; (ii) data sharing and interoperability; (iii) data preparation; (iv) international standards and cooperation; (v) biases during AI modeling, and (vi) global health implications.
The integration of FAIR (Findability, Accessibility, Interoperability, and Reusability)-compliant reference ontologies with Observational Health Data Sciences and Informatics (OHDSI) vocabularies can significantly enhance medical data harmonization, addressing interoperability, consistency, and usability gaps. The FAIR principles ensure that data are well-documented, structured for both human and computational use, and efficiently integrated across different systems. OHDSI’s vocabularies can enhance these principles by providing a standardized framework that facilitates data integration from diverse healthcare systems. Standardization of data representation is another critical advantage. Utilizing FAIR-compliant ontologies and OHDSI vocabularies enables the mapping of diverse healthcare data to a unified set of terms and definitions, reducing ambiguity and enhancing data accuracy. These ontologies can ensure consistent data modeling across various sources, which is crucial for effective data aggregation. They also promote scalable data integration through semantic enrichment, which enhances querying and data analysis capabilities. This integration, in turn, enhances data quality and usability. Ontologies facilitate data validation by enforcing adherence to predefined relationships and constraints, ensuring data integrity, quality, and reusability, thus fostering efficiency in research and reducing redundancies in data collection, which is particularly valuable in healthcare.
The regulatory landscape significantly impacts data harmonization efforts. Regulations, such as the Health Insurance Portability and Accountability Act (HIPAA)[4] in the United States or the General Data Protection Regulation (GDPR)[5] in the European Union, define how patient data can be collected, stored, shared, and used. Achieving compliance with these regulations is complex and poses barriers to harmonizing data across different regions. HIPAA primarily focuses on the privacy and security protections of personal health information in the United States, setting standards for how such data should be handled to ensure data confidentiality and integrity. It outlines specific conditions under which protected health information (PHI) can be used or disclosed, mandating physical, administrative, and technical safeguards. This affects the architecture and security features of data management systems used in harmonization, necessitating features such as data encryption, secure access controls, and audit capabilities to comply with HIPAA’s strict safeguards. GDPR, on the other hand, emphasizes the protection of personal data and the privacy of EU citizens. It introduces comprehensive rights for data subjects, such as the right to access, rectify, and erase their data, which influences how data are collected, stored, and utilized in harmonization processes. GDPR also requires explicit consent for data processing activities, impacting how consent is captured and managed in data integration projects. Additionally, it imposes stringent conditions on the transfer of personal data outside the EU, affecting global data harmonization efforts by requiring compliance with specific legal frameworks before data can be transferred internationally. Both HIPAA and GDPR establish a framework that ensures data are handled responsibly and ethically, helping to establish trust among stakeholders, which is essential for successful data harmonization initiatives. However, their stringent requirements also pose challenges, including compliance costs and operational complexities.
Another critical challenge lies in the technical barriers that are introduced during data sharing, including data standardization and interoperability. The first major technical obstacle is data interoperability. Medical data are stored in different formats, creating significant challenges for seamless data exchange and integration. Developing and maintaining universal standards that facilitate global data sharing requires extensive coordination among a myriad of stakeholders, alongside continuous technological updates. Another substantial technical challenge is maintaining data quality and integrity. Inconsistencies, errors, and incomplete data can severely impact patient care and the validity of research. Implementing thorough data validation and cleaning processes, though crucial, is resource-intensive and technically demanding. Furthermore, the scalability and infrastructure required to manage the vast amounts of data generated globally pose their own set of challenges. Establishing the necessary infrastructure for large-scale data harmonization requires significant financial investment and sophisticated technological solutions, including the utilization of cloud computing and high-performance computing platforms. On the ethical front, patient privacy and data security are critical. The risk of data breaches and unauthorized access increases as data are shared across borders, complicating compliance with diverse and sometimes conflicting data protection regulations (GDPR, HIPAA). Another ethical concern is ensuring proper consent and autonomy. The varying cultural norms and legal frameworks around consent across different countries complicate the process of informing patients and obtaining their consent regarding data use. Ensuring patients are
Various semi-automated tools have been proposed to harmonize diverse data in biobanks, clinical registries, and cohorts. These include the DataSHaPER[8,9], which was successfully used to align 53 epidemiological databases with a 36% rate of compatibility, the SORTA system[10], yielding a 97% recall rate in correlating 5,120 entries within a single biobank, and the BiobankConnect[11], which demonstrated a 74% precision in integrating data across six biobanks. Semantic matching approaches were also proposed to map cohort data to reference model elements[12], yielding 67 different mapping scenarios to model all possible associations between 8 EU cohorts and a reference data model. Statistical methods like Item Response Theory (IRT) analysis[13] have also been applied to investigate the influence of various factors on certain items, such as psychiatric phenotypes, to achieve uniformity in scale. However, these methods face significant limitations in their applicability across different clinical areas. They rely on a semi-automated approach that necessitates close cooperation between clinical experts and technical professionals to establish specific lexical matching rules. The efficiency of these existing systems is often compromised, either by the intricate nature of the clinical domain being studied or by the absence of computational techniques for automated terminology matching.
To address these challenges, we propose a cutting-edge, holistic data harmonization approach that goes beyond the current state of the art. Our approach is based on the development of a “smart”
METHODS
Overview
The proposed framework is executed in the form of a web service through a secure, GDPR-compliant, federated cloud computing environment[24]. The workflow of the framework is depicted in Figure 2. It has been designed to support different types of unstructured data and consists of five stages, including: (i) metadata extraction and relational mapping stage; (ii) data modeling stage; (iii) “smart” knowledge-based mapping stage; and (iv) lexical and semantic analysis stage. Stage (i) is automated and uses as input a . JSON file which represents the input tabular dataset and automatically extracts the feature names (terminologies), the range values (minimum and maximum values per feature), and the data types (integer, float, string, etc.). Stage (ii) is manual and involves the definition of a reference ontology (i.e., a hierarchical data model) to represent the domain knowledge of a disease of interest using Protégé to map the features into entities (classes, subclasses) and to define object properties (relationships among them). This stage requires close collaboration between the clinical experts in the field and the technical experts to map each entity into codes from international data models like SNOMED-CT, RxNorm, LOINC, etc., to promote data interoperability following the FAIR CookBook principles. Stage (iii) is also automated and involves the definition of a large corpus as described in A1. Stage (iv) is automated and involves the hybrid application of lexical and semantic analysis, where the Levenshtein distance and the Jaro distance are used to identify lexically relevant features and the extracted information from the word embeddings is used to identify conceptually relevant features. The output of the workflow is a data harmonization report which summarizes the main findings of the harmonization process. At the heart of our framework lies the robust definition and development of HL7 compliant data models. Our approach adheres to and is inspired by the principles outlined in the FAIR CookBook[22] for making data FAIR, and our methodology extends these principles into practical application. We focus on ensuring that the resulting harmonized data are not only standardized but also semantically enriched and globally accessible (the first three stages in Figure 2 are related to data FAIRification).
Input
The proposed workflow can support a variety of unstructured tabular data which are stored in the form of csv, xlsx, SQL, and txt formats. Data sharing/processing agreements are distributed to the data providers to fulfill all necessary GDPR and HIPAA requirements for data sharing.
Functionalities/stages
Metadata extraction and relational mapping stage
Useful metadata are extracted from the input raw data, including feature names, range values, and data types. This information is stored in the form of a JSON (JavaScript Object Notation) structure. Relational modeling is then applied to transform the JSON structure into an XML (Extensible Markup Language) format for the application of the data modeling stage.
Data modeling stage
A reference ontology is constructed for the domain of interest to encapsulate the disease’s knowledge using the Protégé open source ontology editor[25]. This process involves a detailed mapping and modeling of disease-specific terminologies and concepts from the metadata extraction stage, ensuring that the ontologies serve as robust, accurate representations of medical knowledge. The reference ontology serves as a
“Smart” knowledge-based mapping stage
To further enhance the interconnectivity of the reference ontology, we introduce a linguistic layer by incorporating synonyms from NLTK[14]. This integration broadens the semantic scope of the ontologies, allowing for more comprehensive data interpretation. We define a “smart” knowledge-based repository in the form of a large corpus that is built on top of the OHDSI Athena vocabulary[15] including terminologies from globally recognized healthcare data models. This integration ensures that our reference ontologies are not only detailed but also aligned with global healthcare data practices. The corpus offers the basis for the lexical and semantic analysis stage in Section Lexical and semantic analysis stage. A key innovative aspect of our methodology is the application of word embeddings using the Word2Vec language modeling method[26]. These embeddings are computed to augment the terminologies, adding semantic and contextual layers based on the CBOW (Continuous Bag of Words) architecture[27]. This augmentation yields even more dynamic and interpretive vocabularies, which are essential for effective data harmonization. For example, the terminologies “blood tests” and “hematological tests” are not lexically relevant but are conceptually the same. Therefore, if we calculate the word embeddings for the terminology “blood test”, we can capture all the conceptually relevant terminologies and thus significantly improve the precision of the data harmonization process.
Lexical and semantic analysis stage
Moving beyond the conventional lexical analysis, we propose a hybrid semantic analysis process, utilizing a combination of the Levenshtein and the Jaro distances on top of extracted object properties and entity relations from the reference ontology[28]. This analytical phase is critical in identifying and mapping lexical and conceptual overlaps between our reference ontologies and the terminologies from various clinical centers. Through the examination of the object properties, entity relationships are extracted and word embeddings are calculated for each entity and included in the lexical analysis to further reduce information loss.
Output
The output of the proposed approach is a data harmonization report which summarizes the matched terminologies between the reference ontology and the input set of terminologies along with the level of lexical or conceptual overlap and the standardized value ranges according to the predefined value ranges in the reference ontology.
RESULTS
The proposed approach was applied in three different domains, including the: (i) autoimmune diseases, using unstructured data (clinical, laboratory tests, medical conditions, demographic, therapies) from 21 EU cohorts with 7,551 patients who have been diagnosed with primary Sjogren’s Syndrome (pSS)[29]; (ii) cardiovascular diseases (CVD), using unstructured data (clinical, genomics, laboratory tests, medical conditions, demographic) from 7 EU cohorts with 25,000 patients who were diagnosed with cardiovascular diseases[30]; and (iii) mental disorders (MD; particularly depression and anxiety), using unstructured data (clinical, laboratory tests, medical conditions, demographic) from three EU cohorts with 3,500 patients[30].
Our main findings are summarized in Table 1. Metadata were extracted from each unstructured raw dataset and were stored in dictionaries. Three reference ontologies were constructed upon clinical guidance to reflect the minimum requirements that describe the domain knowledge for each case (i.e., pSS, CVD, MD). We refer to those ontologies as ROPSS, ROCVD, and ROMD, respectively. The ontologies are open (the ROCVD and ROMD can be found under the following GitHub link: https://github.com/vpz4/TO_AITION; the ROPSS is located in the following GitHub link: https://github.com/vpz4/PSS-Ontology) and are expressed into RDF (Resource Description Framework)/OWL (Web Ontology Language) formats to facilitate interoperability between different systems and applications.
Data harmonization results
Domain | Reference ontology | Number of patients | Number of entities (terminologies) | Final set of terminologies | Precision(%) |
AD | ROPSS | 7,551 | 35 (150) | 48 | 45/48 (93.75%) |
CVD | ROCVD | 25,000 | 10 (792) | 71 | 62/71 (87.32%) |
MD | ROMD | 3,800 | 9 (34) | 14 | 12/14 (85.71%) |
Synonyms and word embeddings were calculated for the terminologies per reference ontology, yielding three large corpora, namely CPSS, CCVD, and COMD. The lexical and semantic analyzers were applied to identify overlapping terminologies between CPSS, CCVD, COMD, and the extracted metadata per cohort. Our analysis revealed a set of: (i) 45 common terminologies with 93.75% precision across the 21 EU cohorts in pSS; (ii) 62 common terminologies with 87.32% precision across the 7 EU cohorts in CVD; and (iii) 12 common terminologies with 85.71% precision across the 3 EU cohorts in MD.
CONCLUSIONS AND FUTURE DIRECTIONS
The proposed framework’s compliance with the FAIR CookBook[22] (and FAIRplus[23]) criteria is comprehensive. To facilitate data access and retrieval, it shares and stores data in a secure, GDPR/HIPAA-compliant cloud computing environment, which requires data sharing/processing agreements for access. In modeling the domain, it utilizes metadata extraction, relational mapping, and data modeling stages, employing identifiers from widely recognized HL7 data models such as SNOMED-CT, LOINC, OMOP,
According to Table 2, although the DataSHaPER framework[8,9] offers a data model for mapping biobank data, it faces limitations in diverse clinical domains and relies heavily on expert cooperation. Although the SORTA system[10] is notable for its high recall rate in a single biobank, it is limited to specific biobank mapping scenarios. The BiobankConnect software[11] provides a customized solution to overcome biobank data complexities that are limited to a single biobank. Semantic matching[13] is useful but requires close cooperation between clinical and technical experts. IRT analysis[13] requires context-specific mapping to remove influences of covariates for data standardization. The proposed framework builds on FAIR CookBook (and FAIRplus) principles by utilizing the OHDSI Athena vocabulary to create a “smart” knowledge-based repository, yielding overlapping terminologies with more than 85% precision across three different clinical domains. However, this framework faces challenges in implementation complexity and needs more real-world applications to enhance its generalizability. In future work, we plan to include functionalities to reduce bias and enhance cross-lingual capabilities to ensure that medical data are more universally usable, contributing to equitable healthcare delivery across different linguistic and regional boundaries[31]. As the healthcare sector progresses, straightforward strategies like the proposed one are essential in addressing today’s challenges.
Comparison of the proposed framework with similar ones
Study | Key points | Advantages | Disadvantages |
[8,9] | 36% compatibility rate in aligning 53 epidemiological databases | A comprehensive data model for mapping the domain knowledge of complex biobank data | Significant limitations in different clinical domains, require close cooperation between clinical and technical experts, detailed mapping of terminologies to ontologies, absence of computational techniques for fully automated matching |
[10] | 97% recall rate in correlating 5,120 entries within a single biobank | Proven effectiveness in specific mapping scenarios across biobanks | |
[11] | 74% precision in integrating data across six biobanks | A customized solution that is fine-tuned to the needs and complexities of biobank data | |
[12] | Mapped cohort data to reference model elements for eight EU cohorts | Data modeling is based on terminologies from HL7 data models | |
[13] | Models the influence of various factors on psychiatric phenotypes | Particularly effective in scenarios where detailed, context-specific mapping and analysis are required | |
Current | Terminologies with more than 85% precision across three different clinical domains | Builds on FAIR CookBook and FAIRplus principles, introduces linguistic layers based on word embeddings | Increased complexity of implementation, more real-world cases to increase the generalizability of the framework |
DECLARATIONS
Acknowledgments
The research work has received funding from the European Commission under GA 101135932 (FAITH Project).
Authors’ contributions
Concept and design of the study: Pezoulas VC, Fotiadis DI
Data analysis and interpretation: Pezoulas VC, Fotiadis DI
Final review and recommendations for improvement: Fotiadis DI
Availability of data and materials
Not applicable.
Financial support and sponsorship
The research work has received funding from the European Commission under GA 101135932 (FAITH Project).
Conflicts of interest
Both authors declared that there are no conflicts of interest.
Ethical approval and consent to participate
Not applicable.
Consent for publication
Not applicable.
Copyright
© The Author(s) 2024.
REFERENCES
1. Schmidt BM, Colvin CJ, Hohlfeld A, Leon N. Definitions, components and processes of data harmonisation in healthcare: a scoping review. BMC Med Inform Decis Mak 2020;20:222.
2. Kumar G, Basri S, Imam AA, Khowaja SA, Capretz LF, Balogun AO. Data harmonization for heterogeneous datasets: a systematic literature review. Applied Sciences 2021;11:8275.
3. Kourou KD, Pezoulas VC, Georga EI, et al. Cohort harmonization and integrative analysis from a biomedical engineering perspective. IEEE Rev Biomed Eng 2019;12:303-18.
4. Moore W, Frye S. Review of HIPAA, part 1: history, protected health information, and privacy and security rules. J Nucl Med Technol 2019;47:269-72.
5. Zaeem RN, Barber KS. The effect of the GDPR on privacy policies: recent progress and future promise. ACM Trans Manage Inf Syst 2021;12:1-20.
6. Duda SN, Kennedy N, Conway D, et al. HL7 FHIR-based tools and initiatives to support clinical research: a scoping review. J Am Med Inform Assoc 2022;29:1642-53.
7. Wagner AH, Babb L, Alterovitz G, et al. The GA4GH variation representation specification: a computational framework for variation representation and federated identification. Cell Genom 2021;1:100027.
8. Fortier I, Burton PR, Robson PJ, et al. Quality, quantity and harmony: the DataSHaPER approach to integrating data across bioclinical studies. Int J Epidemiol 2010;39:1383-93.
9. Fortier I, Doiron D, Little J, et al. International Harmonization Initiative. Is rigorous retrospective harmonization possible? Application of the dataSHaPER approach across 53 large studies. Int J Epidemiol 2011;40:1314-28.
10. Pang C, Sollie A, Sijtsma A, et al. SORTA: a system for ontology-based re-coding and technical annotation of biomedical phenotype data. Database 2015;2015:bav089.
11. Pang C, Hendriksen D, Dijkstra M, et al. BiobankConnect: software to rapidly connect data elements for pooled analysis across biobanks using ontological and lexical indexing. J Am Med Inform Assoc 2015;22:65-75.
12. Chondrogiannis E, Karanastasis E, Andronikou V, Varvarigou T. National Technical University of Athens; Athens; Greece. Bridging the gap among cohort data using mapping scenarios. J Assoc Inf Tech 2021;12:179-88.
13. Van Hauwaert SM, Schimpf CH, Azevedo F. The measurement of populist attitudes: testing cross-national scales using item response theory. Politics 2020;40:3-21.
14. Available from: https://www.nltk.org/ [Last accessed on 24 May 2024].
15. Available from: https://athena.ohdsi.org/search-terms/start [Last accessed on 24 May 2024].
16. Available from: https://www.snomed.org/ [Last accessed on 24 May 2024].
17. Available from: https://www.nlm.nih.gov/research/umls/rxnorm/index.html [Last accessed on 24 May 2024].
18. Available from: https://loinc.org/kb/abbreviations/ [Last accessed on 24 May 2024].
19. Available from: https://icd.who.int/en [Last accessed on 24 May 2024].
20. Available from: https://bioportal.bioontology.org/ontologies/ATC [Last accessed on 24 May 2024].
21. Available from: https://www.ohdsi.org/data-standardization/ [Last accessed on 24 May 2024].
22. Rocca-Serra P, Gu W, Ioannidis V, et al. FAIR Cookbook Contributors. The FAIR cookbook - the essential resource for and by FAIR doers. Sci Data 2023;10:292.
23. Available from: https://fairplus-project.eu/about/ [Last accessed on 24 May 2024].
24. Available from: https://www.preciouscloud.eu/ [Last accessed on 24 May 2024].
25. Available from: https://protege.stanford.edu/ [Last accessed on 24 May 2024].
27. Wang, Qi, Xu J, Chen H, He B. Two improved continuous bag-of-word models. 2017 International Joint Conference on Neural Networks (IJCNN): 2017 May 2851-6 Anchorage, AK, USA.
28. Pezoulas VC, Exarchos TP, Fotiadis DI. Medical data harmonization. Medical data sharing, harmonization and analytics. Elsevier; 2020. pp. 137-83.
29. Pezoulas VC, Goules A, Kalatzis F, et al. Addressing the clinical unmet needs in primary Sjögren's Syndrome through the sharing, harmonization and federated analysis of 21 European cohorts. Comput Struct Biotechnol J 2022;20:471-84.
30. Pezoulas VC, Kourou KD, Kalatzis F, et al. Overcoming the barriers that obscure the interlinking and analysis of clinical data through harmonization and incremental learning. IEEE Open J Eng Med Biol 2020;1:83-90.
31. Wan Z, Liu C, Zhang M, et al. Med-unic: Unifying cross-lingual medical vision-language pre-training by diminishing bias. Available from: https://arxiv.org/abs/2305.19894 [Last accessed on 24 May 2024].
Cite This Article
How to Cite
Download Citation
Export Citation File:
Type of Import
Tips on Downloading Citation
Citation Manager File Format
Type of Import
Direct Import: When the Direct Import option is selected (the default state), a dialogue box will give you the option to Save or Open the downloaded citation data. Choosing Open will either launch your citation manager or give you a choice of applications with which to use the metadata. The Save option saves the file locally for later use.
Indirect Import: When the Indirect Import option is selected, the metadata is displayed and may be copied and pasted as needed.
About This Article
Copyright
Data & Comments
Data
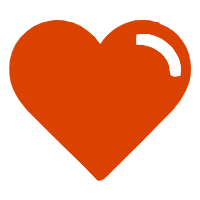
Comments
Comments must be written in English. Spam, offensive content, impersonation, and private information will not be permitted. If any comment is reported and identified as inappropriate content by OAE staff, the comment will be removed without notice. If you have any queries or need any help, please contact us at [email protected].