Advancing cardiovascular disease prediction: portable or wearable devices for automatic and rapid blood sample collection for biomarker detection with simultaneous physiological marker measurement
INTRODUCTION
Cardiovascular diseases (CVDs) are a significant global health burden and the number one killer worldwide for the past many decades. The best way to overcome them is through early prediction, detection and intervention. Recent advances in health engineering and medical technology have generated numerous exciting portable and wearable devices capable of automatic and rapid blood sample collection for biomarker detection, in conjunction with simultaneous physiological marker measurement[1]. This commentary explores the potential of these innovative devices and highlights their significance in advancing early prediction strategies of CVD.
METHODS
Biomarker detection
In the past, biomarker detection through blood samples often involved invasive procedures or visits to healthcare facilities, which was time-consuming, costly, and inconvenient. However, as shown in Figure 1A, recent advances in portable and wearable devices have transformed this process to allow for automatic and rapid blood sample collection for biomarker detection[2] .
Figure 1. Recent advances in portable or wearable devices for automatic and rapid blood sample collection for biomarker detection. (A) Microneedle-based skin patch for rapid and wearable blood test[2]; (B) Quantitative detection of blood cardiac troponin I and creatine kinase-MB level using surface-enhanced Raman scattering (SERS) with nanoparticle-based immunoassay[3].
One innovative approach is surface-enhanced Raman scattering (SERS), which utilizes nanoparticle-based immunoassay to enhance the Raman signal of molecules in blood samples. This enables the detection of highly sensitive and specific biomarker. As shown in Figure 1B, Cheng et al.[3] and Gao et al.[4] utilized SERS active noble metal nanoparticles (e.g., gold and silver) functionalized with antibodies to selectively bind to CVD biomarkers. By amplifying the Raman signal, the sensitivity of the CVD biomarkers can be significantly enhanced[5]. The integration of SERS with nanoparticles presents tremendous potential in clinical diagnostics, not only for CVDs, but also for cancers and other infectious diseases.
Moreover, the blood biomarker detection devices can be miniaturized via microelectromechanical systems (MEMS) technologies, e.g., microneedles that induce minimal discomfort during blood sample collection. Blicharz et al.[6] invented a compact and self-contained device consisting of solid microneedles, a high-velocity custom-designed insertion mechanism, a vacuum chamber, and a microfluidic system that contains lithium heparin anticoagulant to achieve safe and painless 100 µL blood sampling and collection, shedding lights on the decentralization of healthcare. Their commercial solution TAP™ from Seventh Sense Biosystems, Inc., has recently gone to the market to provide portable blood sampling solutions for home-usage.
On the other hand, in recent years artificial intelligence (AI) technologies have been widely applied in SERS for CVD biomarker detection. The accuracy, sensitivity, and efficiency of the analysis have all been improved through the help of AI algorithms[7]. Specifically, AI algorithms are making differences through data preprocessing, noise reduction, feature extraction, classification and prediction, as well as calibration and quantification[8]. For example, coupled with machine learning algorithms, including the random forest and support vector machine method, Dixon et al.[9] utilized SERS to characterize complex biofluids and categorize five CVD samples (including c-reactive protein, troponin I, low-density lipoprotein, natriuretic peptide, and creatinine) with an accuracy of 90%.
Through the application of AI algorithms and the seamless integration of them into our daily lives, the emerging portable and wearable devices present great opportunities for advancing blood testing and biomarker detection. They pave the way for improved disease diagnosis, personalized medicine, and early prediction and intervention of CVDs.
Physiological marker measurement
In addition to blood sample collection for biomarker detection, wearable devices offer the unique capability of physiological marker measurement. As shown in Figure 2, by using optical sources or photoplethysmographic (PPG) sensors, these devices integrated with AI algorithms can continuously monitor physiological parameters such as heart rate[10-13], blood pressure[14-16], and blood oxygen saturation[17-19]. For example, by using PPG signals from a smartwatch, Han et al. developed a real-time peak detection algorithm for accurate estimation and determination of heart rate even under various cardiac rhythms. Moreover, by utilizing AI algorithms to extract blood glucose[20] levels from PPG signals, these portable devices can be implemented in wearable platforms such as a watch for unobtrusive measurements[21], eliminating the need for traditional invasive techniques. This real-time monitoring provides valuable insights into an individual’s cardiovascular health and complements the biomarker data, thereby enabling comprehensive assessment of cardiovascular risks at homes and hospitals.
Simultaneous biomarker detection and physiological marker measurement
Current research prototypes and commercial products range from Apple or Huawei watch for physiological parameters monitoring, to Omron, Roche or Yuwell home-usage glucose meter for blood sugar level detection, to NADAL® or Wondfo point-of-care lateral flow assay for troponin I or c-reactive protein blood biomarker test. However, these devices can only achieve separate and independent detection function, either for biomarkers or for physiological markers.
Therefore, we have been developing an integrated solution for simultaneous detection of biomarkers and physiological markers from a finger[1], as shown in Figure 3. This integrated method is based on PPG, SERS, and microfluidic chip. A PPG sensor can not only function as a physiological marker detector, but also a distance sensor to achieve close-looped control for blood sampling.
To promote the adoption in clinical settings, clinical trial and reliability test involving sufficient amount medical data and regulatory approval from relevant authorities such as the Food and Drug Administration (FDA) in the United States or the National Medical Products Administration (NPMA) in China or equivalent regulatory bodies in other countries are needed. New device needs to comply with regulatory requirements for safety, efficacy, manufacturing, and quality control.
The existing healthcare infrastructure is mainly composed of hospitals and community health care facilities (small clinics, pharmacies, etc.), as well as their relevant services. The new portable or wearable easy-to-use device with integrated biomarker and physiological marker detection function can be distributed to small clinics, especially clinics in remote or developing or less developed areas, where bulky professional medical equipment used in hospitals, are not available. Moreover, this device can also be used at home by families or individuals. Considering community usage and home care, this device can supplement and decentralize the functions of hospitals and large medical institutions by providing people with easy-to-access health monitoring and assessment. Hence, this device has great potential to fundamentally change the current health care system, and realize predictive, preemptive, preventive, personalized, pervasive healthcare.
AI empowered integration for CVD prediction and health management
AI, as an emerging technology, holds immense potential to facilitate the process of CVD prediction and health management. Figure 4 presents an AI empowered integration of biomarkers and physiological makers for CVD prediction and health management, using the framework[22] of convolutional neural network (CNN), fully convolutional network (FCN), and principal component analysis network (PCANet) as examples. AI can play important roles in three levels as shown in the diagram. On the one hand, at level 1 and level 2, with more and more machine learning algorithms emerging, AI algorithms can help with the denoising, signal interpretation, and quantification of both biomarker detection from SERS spectrum[7-9,23] and physiological marker measurement from PPG signal[10,14,17,20]. On the other hand, at level 3, AI algorithms can analyze and integrate the biomarker and physiological marker parameters, coupled with other factors including historical health records and demographic information to predict CVD risks. Learning from large-scale datasets, the AI algorithms can extract meaningful insights and develop accurate predictive models that can estimate an individual's likelihood of developing CVD, hence assessing individual health conditions as well as providing personalized healthcare suggestions[24,25]. This integration, empowered by AI, allows for more accurate risk stratification, enabling targeted interventions and preventive measures. Furthermore, AI-embedded portable especially wearable device can continuously monitor and analyze real-time data to detect anomalies or changes that may signify worsening cardiovascular health. This proactive approach to health management facilitates timely interventions, personalized treatment plans, and improved healthcare outcomes. Overall, the AI-enabled integration of biomarkers and physiological markers has the potential to revolutionize CVD prediction and health management, leading to more effective prevention, early intervention, and optimal care for individuals at risk of CVD.
Figure 4. AI empowered integration of biomarkers and physiological makers for CVD prediction and health management, using the framework[22] of convolutional neural network (CNN), fully convolutional network (FCN), and principal component analysis network (PCANet) as examples.
Advantages
Convenience and user-friendliness: One of the key advantages of portable or wearable devices capable of simultaneous detection of blood and physiological markers is their convenience and user-friendliness. These devices are designed to be compact, lightweight, and easy to use, allowing individuals to monitor their cardiovascular health conveniently and effortlessly at home. By removing barriers and simplifying the process, these devices equipped with AI algorithms encourage proactive engagement in preventive care, empowering individuals to take charge of their cardiovascular well-being.
Real-time insights, early prediction and risk mitigation: The integration of automatic blood sample collection with CVD biomarker detection and simultaneous physiological marker measurement in portable or wearable devices provides real-time insights into an individual's cardiovascular health. This real-time information facilitates early prediction of CVDs, allowing for timely intervention, risk mitigation and emergency care for acute CVDs. Healthcare professionals can use these devices connected with telemedicine and mHealth systems to identify abnormal biomarker levels, track trends, and monitor physiological markers, enabling personalized interventions tailored to each individual's needs.
CHALLENGES AND FUTURE DIRECTIONS
While portable or wearable devices for automatic blood sample collection and biomarker detection with simultaneous physiological marker measurement show immense potential, several challenges need to be addressed:
1. Unobtrusive and Continuous Monitoring of Multimodal CVD Markers: developing methods for unobtrusive and continuous monitoring of multimodal biomarkers at the same time remains a significant challenge. Technologies need to be further developed to ensure long-term wearability, user comfort, and accuracy in capturing real-time multimodal data of CVD markers and blood glucose level together with vital signs such as BP, ECG, etc. In addition, advances in sensor miniaturization, power efficiency, and wireless connectivity are key areas to focus on to enable seamless and continuous monitoring without interfering with daily activities. Besides, possible solutions also include developing ultrathin integrated flexible sensor to enhance wearability and enable continuous monitoring.
2. Standardization of Sample Collection and Biomarker Detection Protocols: establishing standardized protocols for sample collection and biomarker detection is crucial for ensuring consistent and reliable results. Lack of standardization can lead to inconsistent in results, making it difficult to compare and validate findings across different studies or establish reliable diagnostic or prognostic biomarkers and their detection methods. The main challenge lies in the variability that can occur across different research studies, clinical settings, and laboratories. Addressing the challenge of standardization requires a multi-faceted approach and coordinated effort among researchers, clinicians, stakeholders from industries, and regulatory bodies. Collaboration, consensus-building, and the establishment of guidelines, reference materials, and quality control measures are key to achieving standardized sample collection and biomarker detection protocols.
3. Validation of Novel Biomarkers and Physiological markers: as new CVD markers emerge, rigorous validation studies are needed to confirm their clinical CVD relevance, accuracy, and predictive value. To tackle this challenge, large-scale rigorously designed studies involving diverse populations with different cardiovascular conditions should be conducted to assess the CVD predictive, diagnostic, and prognostic capabilities of these novel biomarkers.
4. Ensuring Accuracy and Reliability of Results: accuracy and reliability are critical aspects in biomarker detection and physiological marker measurement. Enhancing the performance of sensing technologies, refining algorithms for data analysis and interpretation, and ensuring calibration and quality control measures are essential for accurate and reliable results. More specifically, the performance of sensing technologies can be influenced by various factors such as interferences and signal-to-noise ratio. To overcome this problem, continuous improvement and optimization of sensing technologies, including sensor design, signal amplification, noise reduction, and interference elimination, can enhance accuracy and reliability. Robust testing and validation of the technology in different conditions and populations are essential to ensure consistent performance. Besides, for calibration and quality control measures, implementing standardized calibration protocols and regular quality control checks can help calibrate measurement devices and ensure accurate and consistent results. Reference materials and certified controls with known concentrations or values can be used to validate measurement accuracy and monitor instrument performance over time. In additional, robust validation studies on large clinical databases and adherence to regulatory standards are necessary to instill confidence in the accuracy of these novel devices and technologies.
5. Sensitivity and Specificity: improving the sensitivity and specificity of unobtrusive sensing for biomarker and physiological marker detection poses several challenges, especially under the circumstance that thousands of different biomarkers and substances existing in human blood. One of the key challenges is to ensure that the sensing technology can capture subtle changes in biomarker levels or physiological parameters accurately and consistently. Moreover, minimizing the interference from external factors, such as motion artifacts, ambient light, and environmental conditions, is essential to improve the sensitivity and specificity of the measurements. Possible solutions to this challenge include developing advanced signal processing algorithms and machine learning techniques to differentiate true biomarker signals from noise and artifacts and improving the selectivity of blood biomarker sensors using necessary antibodies or pretreatment method.
6. Data Security and Privacy: with the integration of portable and wearable devices from individuals to healthcare systems at hospitals, data security and privacy concerns, including data encryption, data storage and retention, data governance and compliance, and user education and awareness, must be effectively addressed. Specifically, it is very important and challenging to ensure the confidentiality of sensitive health data transmitted among devices and healthcare systems. To address the problem, data encryption techniques can be employed to secure the data during transmission, making it unreadable to unauthorized parties. Robust encryption algorithms and secure communication protocols, such as Transport Layer Security (TLS), can be implemented to protect data integrity and confidentiality. As for data storage and retention, healthcare organizations, hospitals, and government should establish secure storage systems for the collected data. This system includes implementing measures, such as encryption at rest, firewall protection, regular backups, and secure data disposal methods. Moreover, to facilitate the protection of personal health information, healthcare systems could establish comprehensive data governance policies and procedures to ensure compliance with relevant regulations, such as the Health Insurance Portability and Accountability Act (HIPAA) in the United States or the General Data Protection Regulation (GDPR) in the European Union.
Future directions should focus on improving the accuracy and reliability of rapid multimodal and unobtrusive CVD marker detection. Importantly, efforts should be made on effectively extracting pertinent and important information from noisy, long-term, and continuous biomarkers and physiological signals through the application of big data analysis technology. Portable or wearable devices offer the advantage of collecting real-time multimodal data over extended periods, providing a wealth of information for CVD prediction. However, the data often contains various sources of noise and require advanced analytical techniques to identify meaningful signals, patterns, and relationships. Big data analysis methods, such as machine learning algorithms and data-mining techniques, play a vital role in processing, denoising, and analyzing this extensive and complex data. By leveraging these technologies, hidden patterns, correlations, and predictive models that enable accurate and early detection of CVDs can be identified, leading to timely intervention and improved personalized health care.
In addition, the integration of unobtrusive blood sugar and blood pressure measurement devices into the existing healthcare infrastructure should also be the focus of future efforts. This involves seamless data integration with electronic health records, interoperability with other medical devices, and integration into clinical decision support systems.
Lastly, advancements in AI and machine learning algorithms can enhance risk prediction models and enable more accurate and personalized assessments of cardiovascular health. Collaborations among researchers, healthcare professionals, technology developers, and policymakers will be the key to drive advancements, standardization, and adoption of these technologies for improved CVD prediction and management.
CONCLUSION
Portable and wearable devices that enable automatic and rapid blood sample collection for biomarker detection in conjunction with simultaneous physiological marker measurement represent major advances for the prediction of CVDs. These devices provide convenience, real-time insights, and early prediction capabilities to empower individuals and healthcare professionals to proactively monitor and manage cardiovascular health. As technologies continues to evolve and major challenges being gradually addressed, the synergistic integration of these devices in clinical practice holds great promise for revolutionizing early prediction strategies, ultimately leading to transform the landscape of cardiovascular disease detection and prevention.
DECLARATIONS
AcknowledgmentsWe thank Professor Yuanting Zhang, Editor-in-Chief for CHATmed, for the insightful discussions with him on the future direction of CVDs.
Authors’ contributionsMade a substantial contribution to the conception and writing of the manuscript: Wang X
Contributed to the manuscript's writing and editing: Wang X, Khoo BL, Chen SC
All authors approved the final manuscript for submission.
Availability of data and materialsNot applicable.
Financial support and sponsorshipThis work was supported by the Hong Kong Centre for Cerebro-cardiovascular Health Engineering, funded by the Innovation and Technology Commission (COCHE-1.5).
Conflicts of interestAll authors declared that there are no conflicts of interest.
Ethical approval and consent to participateNot applicable.
Consent for publicationNot applicable.
Copyright© The Author(s) 2023.
REFERENCES
1. ZhangY, Liu Z, Wang X, et al. A blood sampling instrument integrating biomarker detection and physiological signal monitoring. 2023. Publication number CN115969374A. (In Chinese). Available from https://patents.google.com/patent/CN115969374A/zh?q=%E4%B8%80%E7%A7%8D%E9%9B%86%E7%94%9F%E7%89%A9%E6%A0%87%E5%BF%97%E7%89%A9%E6%A3%80%E6%B5%8B%E5%92%8C%E7%94%9F%E7%90%86%E4%BF%A1%E5%8F%B7%E7%9B%91%E6%B5%8B%E4%B8%80%E4%BD%93%E5%8C%96%E7%9A%84%E9%87%87%E8%A1%80%E4%BB%AA [Last accessed on 26 Sep 2023].
2. Jiang X, Lillehoj PB. Microneedle-based skin patch for blood-free rapid diagnostic testing. Microsyst Nanoeng 2020;6:96.
3. Cheng Z, Wang R, Xing Y, Zhao L, Choo J, Yu F. SERS-based immunoassay using gold-patterned array chips for rapid and sensitive detection of dual cardiac biomarkers. Analyst 2019;144:6533-40.
4. Gao R, Chen F, Yang D, et al. Simultaneous SERS-based immunoassay of dual cardiac markers on pump-free hybrid microfluidic chip. Sensors and Actuators B: Chemical 2022;369:132378.
5. Khlebtsov BN, Bratashov DN, Byzova NA, Dzantiev BB, Khlebtsov NG. SERS-based lateral flow immunoassay of troponin I by using gap-enhanced Raman tags. Nano Res 2019;12:413-20.
6. Blicharz TM, Gong P, Bunner BM, et al. Microneedle-based device for the one-step painless collection of capillary blood samples. Nat Biomed Eng 2018;2:151-7.
7. Liu Z, Meng D, Su G, et al. Ultrafast early warning of heart attacks through plasmon-enhanced raman spectroscopy using collapsible nanofingers and machine learning. Small 2023;19:e2204719.
8. Huang X, Liu B, Guo S, et al. SERS spectroscopy with machine learning to analyze human plasma derived sEVs for coronary artery disease diagnosis and prognosis. Bioeng Transl Med 2023;8:e10420.
9. Dixon K, Bonon R, Ivander F, et al. Using machine learning and silver nanoparticle-based surface-enhanced raman spectroscopy for classification of cardiovascular disease biomarkers. ACS Appl Nano Mater 2023;6:15385-96.
10. Temko A. Accurate heart rate monitoring during physical exercises using PPG. IEEE Trans Biomed Eng 2017;64:2016-24.
11. Han D, Bashar SK, Lázaro J, et al. A real-time PPG peak detection method for accurate determination of heart rate during sinus rhythm and cardiac arrhythmia. Biosensors (Basel) 2022;12:82.
12. Pribadi EF, Pandey RK, Chao PC. Optimizing a novel PPG sensor patch via optical simulations towards accurate heart rates. Microsyst Technol 2020;26:3409-20.
13. Passler S, Müller N, Senner V. In-ear pulse rate measurement: a valid alternative to heart rate derived from electrocardiography? Sensors (Basel) 2019;19:3641.
14. Elgendi M, Fletcher R, Liang Y, et al. The use of photoplethysmography for assessing hypertension. NPJ Digit Med 2019;2:60.
15. Haddad S, Boukhayma A, Caizzone A. Continuous PPG-based blood pressure monitoring using multi-linear regression. IEEE J Biomed Health Inform 2022;26:2096-105.
16. Ibtehaz N, Mahmud S, Chowdhury MEH, et al. PPG2ABP: translating photoplethysmogram (PPG) signals to arterial blood pressure (ABP) waveforms. Bioengineering (Basel) 2022;9:692.
17. Mohan PM, Nisha AA, Nagarajan V, Jothi ESJ. Measurement of arterial oxygen saturation (SpO2) using PPG optical sensor. In: 2016 International Conference on Communication and Signal Processing (ICCSP), Melmaruvathur, India, 2016, pp. 1136-1140.
18. Koteska B, Mitrova H, Bogdanova AM, Lehocki F. Machine learning based SpO2 prediction from PPG signal's characteristics features. In: 2022 IEEE International Symposium on Medical Measurements and Applications (MeMeA), Messina, Italy, 2022, pp. 1-6.
19. Gastel M, Stuijk S, de Haan G. New principle for measuring arterial blood oxygenation, enabling motion-robust remote monitoring. Sci Rep 2016;6:38609.
20. Zhang G, Mei Z, Zhang Y, et al. A noninvasive blood glucose monitoring system based on smartphone PPG signal processing and machine learning. IEEE Trans Ind Inf 2020;16:7209-18.
21. Zheng YL, Ding XR, Poon CC, et al. Unobtrusive sensing and wearable devices for health informatics. IEEE Trans Biomed Eng 2014;61:1538-54.
22. Weng S, Yuan H, Zhang X, et al. Deep learning networks for the recognition and quantitation of surface-enhanced Raman spectroscopy. Analyst 2020;145:4827-35.
23. Wang X, Yin Y, Chen S, et al. 2023. Methods, devices, and equipment for intelligent protein detection based on Raman spectral signals. Publication number CN116297400A. (In Chinese). Available from https://patents.google.com/patent/CN116297400A/zh?q=%E5%9F%BA%E4%BA%8E%E6%8B%89%E6%9B%BC%E5%85%89%E8%B0%B1%E4%BF%A1%E5%8F%B7%E7%9A%84%E8%9B%8B%E7%99%BD%E6%99%BA%E8%83%BD%E6%A3%80%E6%B5%8B%E6%96%B9%E6%B3%95%E3%80%81%E8%A3%85%E7%BD%AE%E5%8F%8A%E8%AE%BE%E5%A4%87#similarDocuments [Last accessed on 26 Sep 2023].
24. Sahu M, Gupta R, Ambasta RK, Kumar P. Artificial intelligence and machine learning in precision medicine: A paradigm shift in big data analysis. Precision Medicine. Elsevier; 2022. pp. 57-100.
Cite This Article

How to Cite
Download Citation
Export Citation File:
Type of Import
Tips on Downloading Citation
Citation Manager File Format
Type of Import
Direct Import: When the Direct Import option is selected (the default state), a dialogue box will give you the option to Save or Open the downloaded citation data. Choosing Open will either launch your citation manager or give you a choice of applications with which to use the metadata. The Save option saves the file locally for later use.
Indirect Import: When the Indirect Import option is selected, the metadata is displayed and may be copied and pasted as needed.
About This Article
Copyright
Data & Comments
Data
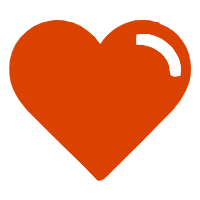
Comments
Comments must be written in English. Spam, offensive content, impersonation, and private information will not be permitted. If any comment is reported and identified as inappropriate content by OAE staff, the comment will be removed without notice. If you have any queries or need any help, please contact us at [email protected].