The use of restricted activity to identify global decline in multimorbidity: current evidence and the potential of a connected health approach
Abstract
Keywords
INTRODUCTION
The number of people living with multiple long-term conditions (MLTCs) is growing, almost becoming the “norm” amongst many adults[1]. MLTCs exist when an individual has two or more chronic conditions. These can be persistent physical, mental, non-communicable or infectious diseases[2]. The long-term nature of these conditions means that they accumulate with age, and with the ageing population[1,3], this can create unsustainable pressure on healthcare resources.
Problems with typical care pathways for multiple long-term conditions
Western healthcare is typically arranged around a series of specialities, each of which has developed around treating a single disease or disease complex[4]. Such a system is not well-placed to manage MLTCs. Even when generalists lead care, they often revert to single disease pathways[4]. There is a need to design forward-thinking and innovative changes in the delivery of care, including addressing operational, logistical, and medical issues [Figure 1]. Difficulties include patients experiencing problematic polypharmacy including drug-drug or drug-disease interactions, follow-up by multiple different doctors, departments, or hospitals, with little communication between them, along with problems associated with their social settings[2] [Figure 1].
Current multiple long-term conditions care
In England, more than half of people over 65 years old suffer from MLTCs, with this predicted to reach two-thirds by 2035[5]. Previously increasing life expectancy has flattened in some countries and led to increased years lived with poor health[6]. A significant cause of this is the well-established positive association between age and the prevalence of MLTCs[1]. Measures addressing the care of those with MLTCs may, therefore, ultimately improve the quality of life for older adults.
People with MLTCs represent a significant proportion of primary and secondary care workload (and therefore costs) yet are prone to poorer outcomes[7]. They may also straddle physical and mental health: depression prevalence increases with increasing numbers of chronic conditions[8]. Subsequently, deterioration may be missed or detected late, potentially due to patients falling between specialities or care providers through not fitting within single-condition healthcare models[7].
Patients with MLTCs may require extensive monitoring for their different conditions (e.g., mood, peak flow, blood pressure, and weight) and multiple treatments, yet the more conditions a patient has, the less likely they are to be able to manage complex monitoring and/or treatment regimens[1]. Higher burdens of care cause additional psychological struggles, forming a vicious cycle of decline[1]. Any novel monitoring solution should therefore be simple and require minimal input from the individual. Connected technology could help with this.
CONNECTED HEALTH FOR LONG-TERM CONDITIONS
Healthcare innovation typically moves in waves, where advances in technology and knowledge drive changes in systems operation[9]. At present, there is a shift towards a more population-focused, connected health approach using remote monitoring[10]. Connected health can be described as technology-enabled healthcare delivery[11]. To help meet the challenges of an aging population with an increase in MLTCs, connected health might facilitate enhanced care delivery in an efficient, proactive, and timely manner, along with effective data sharing between patients and professionals. One example of connected health interventions for MLTCs are those aimed at hypertension, where self-monitoring has been shown to result in significant reductions in blood pressure[12]. A systematic review of digital telemedicine solutions in MLTCs found six eligible studies, with three using blood pressure as the primary outcome[13]. While encouraging, this emphasises a continued focus on single disease processes.
Restricted activity as a general measure of health
Self-monitoring of existing clinical markers might encompass multiple disease domains for people with MLTCs placing a significant burden on frail populations[14]. Combining a series of potentially complex steps will likely result in reduced adherence, especially for a person who is deteriorating and therefore may miss early signs of declining health. A general health measure could enable a wider group of patients, especially those with MLTCs or with frailty, to monitor their health, allowing subsequent targeting of monitoring and/or treatment in response to the detection of deterioration.
Restricted activity could be such a marker[15]. It has been defined in various ways in the literature but can be broadly described as a reduction in a person’s usual activities, ultimately leading to being unable to get out of bed. This can be applied to all, regardless of condition, including frailer patients with greater health burdens. There is no universal method of measuring restricted activity. Previously proposed measures included changes in the ability to perform activities of daily living (ADL)[16-18], time in bed during set time periods[19,20], or how respondents spent most of their day running up to an illness[21] [Table 1].
Summary of the different methods of measuring restricted activity in the literature
Type of restricted activity measured | Method of capturing |
Restricted activities | Categorising participants into groups, dependant on how they perform the major activity: unable to perform the major activity, limited in the amount or kind of major activity, limited but not in the major activity, or not limited[22] Categorising participants by independent, partially dependent, or fully dependent based on: ● How able a participant was to walk across a room[16,17] ● How able a participant was to dress themselves[16,17] ● How able a participant was to transfer between a bed a chair[16,17] |
Activities of daily living (ADL) | ADL are an established set of questions used to identify physical independence; Categorising participants by independent, partially dependent, or fully dependent based upon 6 activities, eating, toileting, bathing, dressing, transferring, and walking across a room; An overall score was given[16-18,20,23] |
Restricted activities/Bed rest | Categorising participants based on their mobility patterns: those who could walk independently; those who walked with the help of caregivers or with the use of walking aids; those who were bedridden or confined to a wheelchair[24] Questionnaire, asking how participants spend most of their day: on feet, sitting, or in bed[21] |
Bed rest | Questionnaire looking at daily hours spent in bed: less than 10 h, 10-16 h, or more than 16 h[23] Questionnaire on 1/2 days in bed per fortnight[15] |
Restricted activity could manifest earlier than other typically used signs and can capture declining health due to a variety of underlying causes. Gill’s work in the United States highlighted that restricted activity occurs for older people around 6 months before death leading to increased bed rest approximately 3 months before death[15]. Gill used a set of questions to examine the underlying cause of restricted activity, firstly asking about any restriction in activity and then, if present, asking about detailed reasons. Their findings suggest that restricted activity could be a robust measure of deterioration regardless of cause and could therefore have potential as a general health measure. Other studies have further suggested associations between restricted activity measured in various ways and higher rates of hospitalisation[22] and mortality[15,19,20]. As far as the authors are aware, no previous research has considered restricted activity as a marker of declining health, no previous research has considered restricted activity as a marker of declining health for use in routine clinical practice to trigger further intervention, but in combination with connected solutions, this appears worthy of consideration.
Using a general measure of health, such as restricted activity, would not aim to make new diagnoses, but rather to highlight the start of a decline and a point to intervene with additional, personalised checks. Restricted activity as a prognostic measure does have its limitations, including a bias towards physical activity, which may be skewed by a person’s baseline mobility or external factors such as the weather. Flexibility would be fundamental as each user’s daily activities would differ, and this would need to be considered when designing different features and alerts. For example, it would be important to calibrate the measure to an individual’s baseline movements. Patient involvement prior to conducting this review highlighted some wheelchair users were already conscious of physical activity changes during episodes of decline, supporting the validity of this particular marker but further highlighting the importance of flexibility to suit an individual’s circumstances.
DIGITAL INTERVENTIONS AND WELLBEING TRACKERS IN HEALTHCARE
Wellbeing apps are increasing in popularity, particularly accompanying technology such as smartwatches and Fitbits, where users log drivers of health such as activity, sleep, and dietary intake. Despite this, the market for apps aimed at older people is still lacking. A systematic review of mobile apps for older people highlighted that the needs of the population had not been met sufficiently, specifically focussing on overall app quality, usability, and data protection[23].
Technological advances have empowered patients to take on more responsibility for their health[24]. These advances, provided they are focussed on older populations, have the potential to drive improvements in MLTC care. They might include both passive and active monitoring of activity as well as symptoms. Exploiting the full potential of technology will require attention to issues caused by visual, dexterity, and cognitive decline[23]. Interventions to date have considered monitoring gait changes[25], fall monitoring[26], and sleep tracking[27]. Similar technology could identify restricted activity in the context of MLTCs.
Connected health in disease monitoring
The COVID-19 pandemic brought rapid advances in population symptom monitoring, including the Zoe COVID-19 symptom tracker[28]. It measured self-reported symptoms, identifying that anosmia, fatigue, persistent cough, and loss of appetite could predict COVID-19 infection before rapid self-tests were readily available[28]. This proved extremely useful in tracking numbers as well as the characteristics of new variants. Zoe was downloaded by 2.6 million people in 28 days[29] and received overall consumer feedback, with an average of 4.7 stars on both the Apple and Google Play stores[30,31].
Another successful symptom-tracking app is TrueColours, which is used to monitor mental health in people with bipolar disorder. TrueColours works by self-reporting symptoms through texts, web-interface or smartphone apps on an agreed personal schedule of weekly, daily or specific set times. Symptoms are reported on a user-friendly interface, with access for supervising clinicians. It has been shown to detect episodes earlier and to monitor the user’s condition[32]. TrueColours was shown to be effective in reducing long-term mood variability[33]. The app is embedded into clinical systems but can also be used for collecting data in new trials. It has now also been translated into use for other conditions with fluctuating symptoms, such as ulcerative colitis[34].
Some studies have explored tracking movement using sensors within a home setting, with the long-term goal of detecting early cognitive decline[35]. These Intelligent Systems for Assessing Aging Changes (ISAAC) demonstrated successful tracking of activity changes, including total activity, night-time activity, and walking speeds; however, the prognostic significance of these measures is not clear, nor when these technologies will be widely available.
HOW MIGHT CONNECTED HEALTH INTERVENTIONS MONITOR ACTIVITY?
The jump from health logging and disease monitoring in younger people to monitoring activity in an older population may not be straightforward, but there is considerable potential as smartphones become more prevalent across the whole population.
Key attributes of such an intervention might include a combination of monitoring activity passively and active collection of symptoms questionnaires to understand how an individual is feeling. An understanding of the patient’s feelings would be crucial to the success of such an intervention to gain better insight into who is at risk of declining health. This will be important in practice, as some patients may display reduced activity due to external factors not relating to health, such as bad weather.
If changes in disease states, from stable to unstable, can be identified, this could offer a point to add additional clinical monitoring, home visits from carers or medical staff, or a medication review to assess if intervention is needed. Such monitoring would aim to facilitate the management of patients with frailty or MLTCs by using restricted activity to detect early signs of declining health and to communicate with professionals as required[15,18,22,36].
Two important aspects of integrating such an intervention into future primary care workflows would be an understanding of different activity change thresholds and what these could mean, as well as downstream workload issues. This would allow the right data to be integrated into patient records, which would trigger time-sensitive tasks to action by an appropriate staff member.
An important aspect of this intervention is to alleviate the burden of monitoring for patients. At present, the burden on these already vulnerable patients is extremely high[1]. There is an argument that typical monitoring is done too frequently while patients are healthy[37,38]. At present, the burden on these patients is extremely high[1].Considering the nature of the likely target patient group, an important aspect of any intervention to identify functional deterioration would be to alleviate the burden of monitoring. Extensive monitoring has been observed to contribute to health inequalities, therefore having one “general” self-monitoring measure that could be self-administered or administered by a familial or formal caregiver might be more feasible and accessible to a wider range of people as a first-line of monitoring (i.e., a flag to initiate further action and/or monitoring).
Extensive monitoring has been observed to contribute to health inequalities, therefore having one “general” self-monitoring measure that could be self-administered, or administered by a familial or formal caregiver, might be more feasible and accessible to a wider range of people, as it could act as a first line of monitoring to detect changes from a stable state, and a trigger point for additional monitoring and/or intervention. This would also aim to encourage patients to take responsibility for their health, alleviating some of the burdens on the healthcare, and hopefully allow re-allocation of resources to patients who are less able to self-monitor.
EXPECTED BARRIERS TO IMPLEMENTATION
Like all new interventions, especially digital solutions, there will be barriers to uptake. The digital healthcare market is becoming more saturated; however, many available solutions are not used effectively[39]. Wang identified some key attributes of successful healthcare apps[40]. These were grouped into three categories: excitement, performance, and basic, impacting user satisfaction and dissatisfaction. While all 10 of the attributes play a role in active usage of an app, flexibility to fit around different people’s lives, and information support would be most crucial to a restricted activity intervention.
Technological retention is another difficult issue, with significantly more engagement during the initial period of use period. Global statistics from 2020 showed that healthcare app retention drops from 20% to 4% after 30 days[41]. To maintain usage, the reasons for using the app and the benefits from this should be clear.
A recent study identified the main barriers to engagement in digital health to be a lack of self-efficacy, knowledge, functionality, and understanding of the benefits[42]. To counteract this, the key enabler to uptake was support for the user to help overcome concerns, reinforce commitment, and enhance confidence in the value of its use. This is especially important when supporting vulnerable patient groups, such as older people with MLTCs.
Therefore, to overcome these expected barriers, it is important to design an intervention that is easy to use for all user groups; for example, if a patient and carer are both expected to use the intervention, it should be flexible to fit into the different everyday lives for both these users and potentially allow multiple users per individual being monitored. Any intervention should seek to be co-produced with patient and public contributors, as well as other stakeholder representatives including clinicians and technologists, to ensure their needs are properly addressed.
The end user of such interventions is still uncertain; however, identifying approaches that make these monitoring activities less intrusive would allow a broader range of people to monitor, which might be helpful as previously healthy people transition into a more frail state. This should be addressed in future research.
CONCLUSION
In summary, many healthcare structures are not currently designed to optimally monitor and manage MLTCs. One of the big challenges is to understand the general non-specific symptoms patients often display and know how to subsequently target appropriate care. When problems are detected late, poorer patient outcomes tend to be the result.
Using restricted activity in the initial identification of declining health could help to detect those at the highest risk and enable earlier intervention. Before such interventions can be developed, a measure of restricted activity must be established, with an appropriate delivery method for the target users. Developing such an intervention could open the doors to improving MLTC patient care at a crucial time. Ultimately, more collaboration and innovation can align the healthcare system to MLTC patients’ needs.
DECLARATIONS
Authors’ contributions
Wrote the first draft: Henderson IL, McManus RJ
Subsequently edited and finalised the first draft: Henderson IL, Sheppard JP, Barnes RK, McManus RJ
Availability of data and materials
Not applicable.
Financial support and sponsorship
Henderson I, Barnes R, and McManus RJ receive funding from the National Institute for Health and Care Research (NIHR) Applied Research Collaboration (ARC) Oxford and Thames Valley (OxTV). McManus RJ is an NIHR Senior Investigator. Sheppard JP receives funding from the Wellcome Trust/Royal Society via a Sir Henry Dale Fellowship (Ref: 211182/Z/18/Z). This research was funded, in part, by the Wellcome Trust. For the purpose of open access, the author has applied a CC BY public copyright licence to any Author Accepted Manuscript version arising from this submission.
Conflicts of interest
McManus RJ has worked with Omron and Sensyne to develop telemonitoring systems for which the University of Oxford has received consultancy and /or licencing payments. He receives occasional expenses and honoraria payments for talks at conferences and the latter are paid to Green Templeton College Oxford. The other authors have no conflicts to declare.
Ethical approval and consent to participate
Not applicable.
Consent for publication
Not applicable.
Copyright
© The Author(s) 2023.
REFERENCES
1. Barnett K, Mercer SW, Norbury M, Watt G, Wyke S, Guthrie B. Epidemiology of multimorbidity and implications for health care, research, and medical education: a cross-sectional study. Lancet 2012;380:37-43.
2. The Academy of Medical Sciences. Multimorbidity: a priority for global health research; 2018. Available from: https://acmedsci.ac.uk/file-download/82222577 [Last accessed on 10 Apr 2023].
3. Office for National Statistics (ONS). Overview of the UK population: January 2021. Available from: https://www.ons.gov.uk/peoplepopulationandcommunity/populationandmigration/populationestimates/articles/overviewoftheukpopulation/january2021 [Last accessed on 10 Apr 2023].
4. Hughes LD, McMurdo ME, Guthrie B. Guidelines for people not for diseases: the challenges of applying UK clinical guidelines to people with multimorbidity. Age Ageing 2013;42:62-9.
5. Kingston A, Robinson L, Booth H, Knapp M, Jagger C. MODEM project. Projections of multi-morbidity in the older population in England to 2035: estimates from the Population Ageing and Care Simulation (PACSim) model. Age Ageing 2018;47:374-80.
6. Office for National Statistics (ONS). Health state life expectancies, UK: 2016 to 2018. Available from: https://www.ons.gov.uk/peoplepopulationandcommunity/healthandsocialcare/healthandlifeexpectancies/bulletins/healthstatelifeexpectanciesuk/2016to2018 [Last accessed on 10 Apr 2023].
7. Fortin M, Lapointe L, Hudon C, Vanasse A, Ntetu AL, Maltais D. Multimorbidity and quality of life in primary care: a systematic review. Health Qual Life Outcomes 2004;2:51.
8. Gunn JM, Ayton DR, Densley K, et al. The association between chronic illness, multimorbidity and depressive symptoms in an Australian primary care cohort. Soc Psychiatry Psychiatr Epidemiol 2012;47:175-84.
9. Keesara S, Jonas A, Schulman K. Covid-19 and health care’s digital revolution. N Engl J Med 2020;382:e82.
10. Lim SA, Khorrami A, Wassersug RJ. Twenty years on - has patient-centered care been equally well integrated among medical specialties? Postgrad Med 2022;134:20-5.
11. Caulfield BM, Donnelly SC. What is connected health and why will it change your practice? QJM 2013;106:703-7.
13. Kraef C, van der Meirschen M, Free C. Digital telemedicine interventions for patients with multimorbidity: a systematic review and meta-analysis. BMJ Open 2020;10:e036904.
14. Villacampa-Fernández P, Navarro-Pardo E, Tarín JJ, Cano A. Frailty and multimorbidity: two related yet different concepts. Maturitas 2017;95:31-5.
15. Gill TM, Gahbauer EA, Leo-Summers L, Murphy TE. Taking to bed at the end of life. J Am Geriatr Soc 2019;67:1248-52.
16. Carey EC, Walter LC, Lindquist K, Covinsky KE. Development and validation of a functional morbidity index to predict mortality in community-dwelling elders. J Gen Intern Med 2004;19:1027-33.
17. Carey EC, Covinsky KE, Lui LY, Eng C, Sands LP, Walter LC. Prediction of mortality in community-living frail elderly people with long-term care needs. J Am Geriatr Soc 2008;56:68-75.
18. Zhao L, Tatara K, Kuroda K, Takayama Y. Mortality of frail elderly people living at home in relation to housing conditions. J Epidemiol Community Health 1993;47:298-302.
19. Clark LP, Dion DM, Barker WH. Taking to bed. Rapid functional decline in an independently mobile older population living in an intermediate-care facility. J Am Geriatr Soc 1990;38:967-72.
20. Ginsberg GM, Hammerman-Rozenberg R, Cohen A, Stessman J. Independence in instrumental activities of daily living and its effect on mortality. Aging (Milano) 1999;11:161-8.
21. Jylha M, Hervonen A. Functional status and need of help among people aged 90 or over: a mailed survey with a total home-dwelling population. Scand J Public Health 1999;27:106-11.
22. Gill TM, Desai MM, Gahbauer EA, Holford TR, Williams CS. Restricted activity among community-living older persons: incidence, precipitants, and health care utilization. Ann Intern Med 2001;135:313-21.
23. Portenhauser AA, Terhorst Y, Schultchen D, et al. Mobile apps for older adults: systematic search and evaluation within online stores. JMIR Aging 2021;4:e23313.
24. Martínez-Pérez B, de la Torre-Díez I, López-Coronado M. Mobile health applications for the most prevalent conditions by the World Health Organization: review and analysis. J Med Internet Res 2013;15:e120.
25. Derawi M, Bours P. Gait and activity recognition using commercial phones. Comput Secur 2013;39:137-44.
26. Hakim A, Huq MS, Shanta S, Ibrahim B. Smartphone based data mining for fall detection: analysis and design. Procedia Comput Sci 2017;105:46-51.
27. Natale V, Drejak M, Erbacci A, Tonetti L, Fabbri M, Martoni M. Monitoring sleep with a smartphone accelerometer. Sleep Biol Rhythms 2012;10:287-92.
28. Menni C, Valdes AM, Freidin MB, et al. Real-time tracking of self-reported symptoms to predict potential COVID-19. Nat Med 2020;26:1037-40.
29. Pandit JA, Radin JM, Quer G, Topol EJ. Smartphone apps in the COVID-19 pandemic. Nat Biotechnol 2022;40:1013-22.
30. Apple App Store. Available from:https://www.apple.com/app-store/ [Last accessed on 10 Apr 2023].
31. Google Play Store. Available from:https://google.fandom.com/wiki/Google_Play_Store [Last accessed on 10 Apr 2023].
32. Goodday SM, Atkinson L, Goodwin G, et al. The true colours remote symptom monitoring system: a decade of evolution. J Med Internet Res 2020;22:e15188.
33. McKnight RF, Bilderbeck AC, Miklowitz DJ, Hinds C, Goodwin GM, Geddes JR. Longitudinal mood monitoring in bipolar disorder: course of illness as revealed through a short messaging service. J Affect Disord 2017;223:139-45.
34. Walsh A, Matini L, Hinds C, et al. Real-time data monitoring for ulcerative colitis: patient perception and qualitative analysis. Intest Res 2019;17:365-74.
35. Kaye JA, Maxwell SA, Mattek N, et al. Intelligent systems for assessing aging changes: home-based, unobtrusive, and continuous assessment of aging. J Gerontol B Psychol Sci Soc Sci 2011;66:i180-i90.
36. NICE. Multimorbidity 2018. Available from: https://cks.nice.org.uk/topics/multimorbidity/ [Last accessed on 10 Apr 2023].
37. Perera R, McFadden E, McLellan J, et al. Optimal strategies for monitoring lipid levels in patients at risk or with cardiovascular disease: a systematic review with statistical and cost-effectiveness modelling. Health Technol Assess 2015;19:1-401, vii-viii.
38. Farmer AJ, Stevens R, Hirst J, et al. Optimal strategies for identifying kidney disease in diabetes: properties of screening tests, progression of renal dysfunction and impact of treatment - systematic review and modelling of progression and cost-effectiveness. Health Technol Assess 2014;18:1-128.
39. Hermes S, Riasanow T, Clemons EK, Böhm M, Krcmar H. The digital transformation of the healthcare industry: exploring the rise of emerging platform ecosystems and their influence on the role of patients. Bus Res 2020;13:1033-69.
40. Wang Y, Wu T, Chen Z. Active usage of mobile health applications: cross-sectional study. J Med Internet Res 2021;23:e25330.
41. Ceci L. Mobile app usage - Statistics & Facts: Statista; 2022. Available from: https://www.statista.com/topics/1002/mobile-app-usage/#topicOverview [Last accessed on 10 Apr 2023].
Cite This Article
How to Cite
Download Citation
Export Citation File:
Type of Import
Tips on Downloading Citation
Citation Manager File Format
Type of Import
Direct Import: When the Direct Import option is selected (the default state), a dialogue box will give you the option to Save or Open the downloaded citation data. Choosing Open will either launch your citation manager or give you a choice of applications with which to use the metadata. The Save option saves the file locally for later use.
Indirect Import: When the Indirect Import option is selected, the metadata is displayed and may be copied and pasted as needed.
About This Article
Copyright
Data & Comments
Data
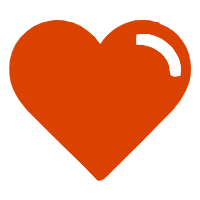
Comments
Comments must be written in English. Spam, offensive content, impersonation, and private information will not be permitted. If any comment is reported and identified as inappropriate content by OAE staff, the comment will be removed without notice. If you have any queries or need any help, please contact us at [email protected].