Special Issue
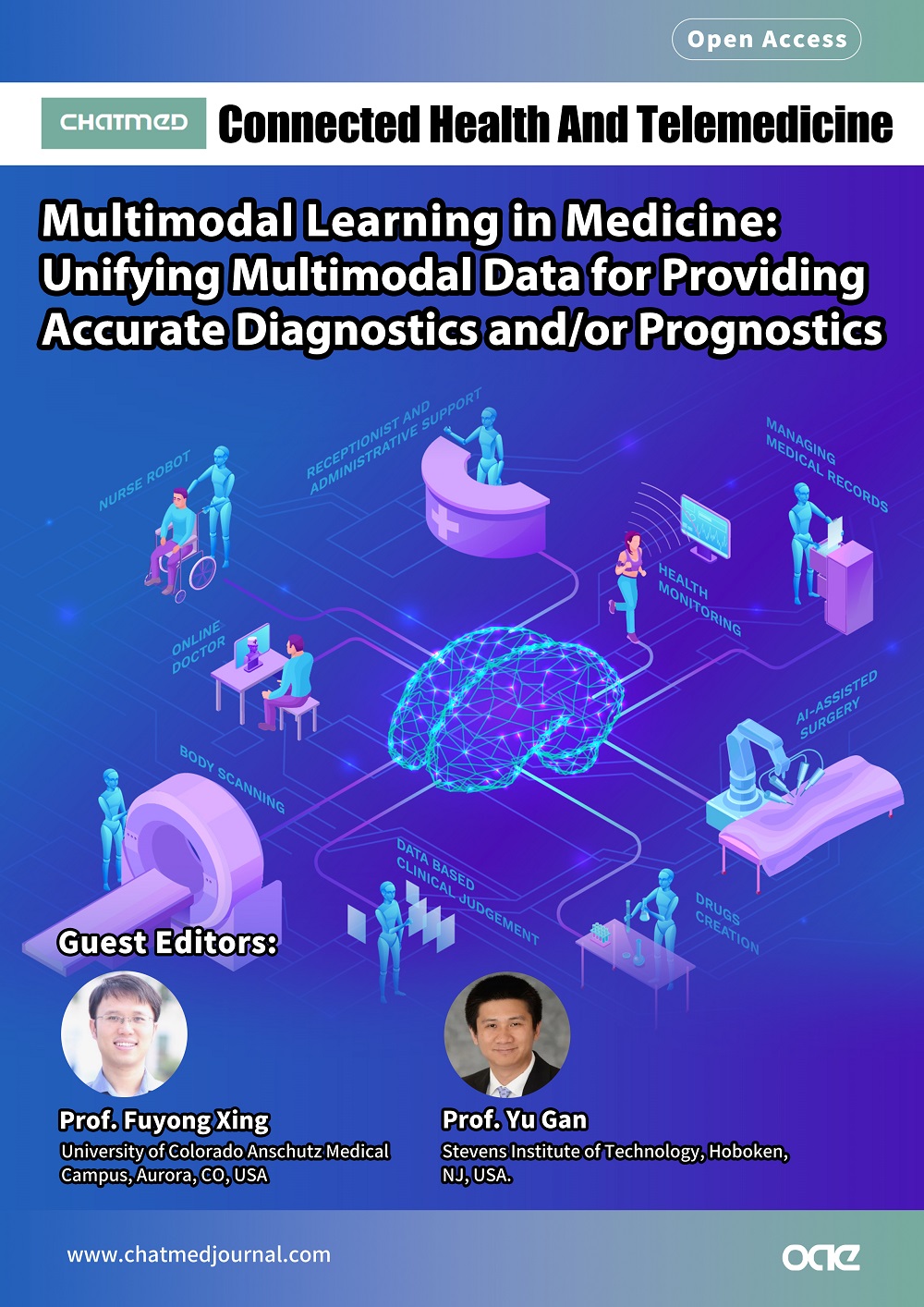
Topic: Multimodal Learning in Medicine: Unifying Multimodal Data for Providing Accurate Diagnostics and/or Prognostics
A Special Issue of Connected Health And Telemedicine
ISSN 2993-2920 (Online)
Submission deadline: 31 Jan 2024
Guest Editor(s)
Special Issue Introduction
Despite the promising results of recent deep multimodal learning in medicine, several significant challenges need to be addressed in real-world applications. These challenges include, but are not limited to, representation learning from heterogeneous multimodal data, information fusion of different modalities, and co-learning to transfer knowledge between modalities. The challenges have significantly impeded the application of deep multimodal learning in real clinical research and practice.
The goal of this Special Issue is to provide a comprehensive platform for researchers and practitioners to showcase their innovative approaches, methodologies, and case studies that highlight the power of integrating multimodal data for accurate diagnosis and/or prognosis. By bringing together a diverse range of perspectives and expertise, we aim to foster collaborations and facilitate transformative advancements in medical diagnosis and patient care.
Scope of the Special Issue
This Special Issue aims to provide a snapshot of recent research related to multimodal machine/deep learning in medicine, benefiting medical AI researchers, clinicians and physicians. The topics of interest will include, but not be limited to:
• Novel algorithms of multimodal learning in medicine;
• Self-supervised multimodal learning;
• Multimodal learning with Transformers and/or other neural networks;
• Vision-language models;
• Multimodal models to integrate medical images (e.g., ultrasound, computed tomography, magnetic resonance imaging, and/or digital pathology) with other modalities, such as electronic health records, genomic, proteomic and/or metabolomic data;
• Applications of state-of-the-art multimodal learning algorithms in medicine;
• Datasets that support multimodal learning.
Key Dates
• Submission deadline: Jan 31, 2024
• Notification of the first review: Mar 1, 2024
• Submission of revised manuscript: Apr 1, 2024
• Notification of final decision: May 1, 2024
Submission Deadline
Submission Information
For Author Instructions, please refer to https://www.oaepublish.com/comengsys/author_instructions
For Online Submission, please login at https://oaemesas.com/login?JournalId=chatmed&IssueId=chatmed230720
Submission Deadline: 1 Nov 2023
Contacts: Ruobing Tong, Assistant Editor, assistant_editor@chatmedjournal.com