Digitization of flow battery experimental process research and development
Abstract
Rising atmospheric CO2 concentrations urgently call for advanced sustainable energy storage solutions, underlining the pivotal role of renewable energies. This perspective delves into the capabilities of redox flow batteries as potential grid storage contenders, highlighting their benefits over traditional lithium-ion batteries. While all-vanadium flow batteries have established themselves, concerns about vanadium availability have steered interest toward Organic Flow Batteries. The multifaceted nature of organic materials calls for an integrated approach combining artificial intelligence, robotics, and material science to enhance battery efficacy. The union of artificial intelligence and robotics expedites the research and development trajectory, encompassing everything from data assimilation to continuous refinement. With the burgeoning metaverse, a groundbreaking avenue for collaborative research emerges, potentially revolutionizing flow battery research and catalyzing the progression towards sustainable energy resolutions.
Keywords
INTRODUCTION
The escalating concern over atmospheric CO2 concentrations, now at levels unseen in millions of years, underscores the urgency to transition from conventional to sustainable energy sources. Renewable energies, such as solar and wind, are pivotal in this shift, but their inherent intermittency due to diurnal cycles and fluctuating wind conditions necessitates robust energy storage systems[1,2]. Currently, pumped hydroelectric storage dominates, contributing to over 90% of grid energy storage[3]. Yet, despite its efficiency and longevity, its geographical constraints and potential ecological implications limit its ubiquity[4,5]. Hence, the significance of electrochemical energy storage systems, which offer flexibility for urban integration, is increasingly pronounced.
The latest research in energy storage has focused on magnesium-based batteries[6], lithium-ion batteries[7], etc. Magnesium-based batteries show promise in providing high energy density and improved safety compared to traditional lithium-ion batteries. However, they face limitations in terms of cathode materials, electrolyte development, dendrite formation, and commercialization. On the other hand, lithium-ion batteries are renowned for their high energy density and efficiency, making them ideal for portable electronics and electric vehicles. However, they have limitations concerning cycle life, scalability, and safety concerns related to thermal runaway and fires. These two technologies represent different approaches to energy storage, each with its strengths and weaknesses, highlighting the ongoing efforts to address their respective limitations and unlock their full potential in diverse applications.
Flow batteries, also known as redox flow batteries (RFBs), represent this type of electrochemical energy storage technology. Unlike traditional ones, which primarily rely on solid electrodes to store energy, flow batteries employ liquid electrolyte solutions[8]. These solutions are housed in two separate tanks, one positively charged and one negatively charged, and they interact within the core of the flow battery: the cell stack. Here, the actual energy conversion takes place through redox reactions. Due to its distinctive design that separates energy and power[9,10], RFBs enable flexibility based on demand. As such, they do not suffer from the cost wastage of surplus battery stacks and materials typical of conventional batteries[10]. The storage of active reactants in separate containers enhances the safety profile of flow ones in comparison to conventional batteries[9]. Moreover, RFBs usually do not result in significant energy losses and boast a prolonged lifespan, positioning them as the optimal choice for long-duration, large-scale energy storage[11]. However, flow batteries face challenges. Take the most technologically mature and commercialized variant, the Vanadium Redox Flow Battery (VRFB), for instance. Its unique electrolyte ensures system purity and longevity, often surpassing 20,000 cycles[12,13]. Yet, the limited availability and toxicity of vanadium and its higher initial costs have hindered the widespread deployment of VRFB[14-16]. Additionally, their energy density has room for improvement. Organic flow batteries may be the game-changer here. The shift from inorganic to organic electrolytes hints at a higher energy density for flow batteries[17]. Organic molecules, with their varying redox potentials, guarantee greater energy storage capabilities[18]. This contributes to the goals outlined in the International Energy Agency (IEA) roadmap. Furthermore, the design of organic molecules is versatile; solubility, chemical reversibility, and redox potentials can be tailored by modifying specific functional groups on the organic core components, improving the battery performance[19]. Leveraging abundant organic materials might also decrease the costs associated with battery production[20]. Indeed, another intricate hurdle emerges: delving into the vast landscape of organic compounds introduces a myriad of complexities. The expansive array of potential organic candidates mandates a sophisticated and streamlined screening methodology. Moreover, variations in the chemical attributes of these molecules necessitate thorough examination to confirm their stability and operational efficiency within the battery matrix. Key factors, such as solubility, minimal decay in discharge capacity, synergy with ancillary battery elements, and enduring cycling resilience, hold supreme importance. Given the extensive list of materials poised for synthesis and the inherently labor-intensive synthesis procedure, reliance on conventional materials research methodologies may inadvertently impede the evolution of flow batteries.
Here are some possible solutions to address these challenges. The choice of electrolyte is critical in flow battery performance. Research efforts should focus on developing new, high-performance electrolyte materials that are cost-effective, have a wide operating temperature range, and exhibit long-term stability. Innovation in organic and aqueous electrolytes could lead to improved flow battery chemistries.
Increasing the energy density of flow batteries is crucial for reducing their physical footprint and overall cost. Researchers are exploring advanced materials, such as high-energy-density redox couples and nanoparticle-based electrodes, to boost energy storage capacity without increasing the size of the system. They often suffer from lower round-trip efficiency compared to traditional batteries. Enhanced electrode materials and cell designs, along with optimized flow management systems, can help improve energy conversion efficiency. Additionally, advanced catalysts can enhance electrode reactions, reducing energy losses.
Flow battery systems should be designed for easy scalability, allowing them to be tailored to various applications. Modular designs and standardized components can simplify scaling up or down, making them more adaptable to grid-level and remote applications. Finally, extending the lifespan of flow battery systems is essential to reduce maintenance costs and environmental impact. Researchers are working on electrode materials and electrolyte formulations that exhibit improved chemical stability and resilience against degradation over multiple cycles.
CURRENT R&D STATUS OF NEW MATERIALS FOR ORGANIC FLOW BATTERIES
In the realm of flow batteries, the design of organic redox-active materials stands as a cornerstone for achieving enhanced performance. While the inherent complexity of organic materials is undeniable, the research trajectory is not without discernible patterns and guidelines. Central to the design philosophy are several electrochemical characteristics, as demonstrated in Table 1. Firstly, electrochemical stability is paramount. For instance, naphthoquinone demonstrates a stable redox behavior without undergoing decomposition, a trait attributed to its stable aromatic system and extensive conjugation. The structural directive here is to lean towards stable aromatic or conjugated systems that resist degradation during redox processes. Secondly, solubility plays a pivotal role. Ferrocene derivatives, for example, have been tailored to exhibit high solubility in organic solvents. The design cue here is the incorporation of polar or charged functional groups. In aqueous systems, hydrophilic groups are favored, while in non-aqueous systems, hydrophobic groups might be more apt. Reaction kinetics is another crucial aspect. Quinoxaline derivatives, with their facile electron transfer capabilities, exemplify the desired rapid redox kinetics. The molecular design direction in this context is to structure molecules with π orbitals that facilitate easy electron transfer or contain unpaired electrons.
Property targets and potential molecules for ORAMs in organic flow batteries
Property | Example | New material design direction |
Electrochemical stability | Naphthoquinone[21,22] | Utilize stable aromatic systems or extensive conjugation |
High solubility | Ferrocene derivatives in organic solvents[23,24] | Incorporate polar or charged functional groups. Hydrophilic groups for aqueous, hydrophobic groups for non-aqueous |
Low toxicity and environmental impact | Hydroquinone derivatives[25,26] | Derivatives of naturally occurring compounds or molecules that are biodegradable |
Crossover prevention | Organic polymers[27,28] | Molecular design leading to larger sizes/using macromolecular structures |
Compatibility with other battery components | Molecules designed not to react with commonly used porous separators | Avoid groups that can chemically attack/degrade other battery components |
Economic feasibility | Organic molecules synthesized from biomass/waste streams | Require fewer synthesis steps or leverage waste materials |
However, it is imperative to note that achieving perfection across all these parameters is a tall order. Often, enhancing one property might come at the expense of another. In this intricate balancing act, digital technology emerges as a beacon. Computational methods and experimental validation allow for the tailored design of organic redox-active materials. Depending on the specific requirements of a given application or scenario, these digital tools can guide the synthesis of molecules that strike the right balance between the myriad of desired properties, paving the way for next-generation organic flow batteries.
DIGITAL TECHNOLOGY ACCELERATES R&D OF NEW MATERIALS
The synergy between artificial intelligence (AI) and robotics has exhibited a transformative potential in the realm of materials science, particularly in the research and development (R&D) of novel materials[29]. These advanced technologies have catalyzed innovations at multiple stages of the material development pipeline, from data collection and screening to material prediction and experimental synthesis. This is illustrated in Figure 1. In the early stages of any data-driven scientific endeavor, the foundational step involves the meticulous collection and processing of relevant data. This process is critical because the quality and quantity of data gathered can significantly influence the outcomes of subsequent analyses. Once the data is thoroughly gathered and processed, it is strategically divided into two distinct sets: training and testing. These sets play a pivotal role in model development and its evaluation.
The training dataset serves as the primary resource during the model selection phase. It provides the necessary data points for the selected model to learn and adapt. Through this learning process, the model endeavors to discern patterns, correlations, or any underlying relationships present in the dataset. On the other hand, the testing dataset plays a critical role in ensuring the model reliability. It acts as a benchmark, facilitating rigorous evaluation by presenting unseen data to the model. This step ensures that the model is not just memorizing the training data but is genuinely learning from it, which is crucial for its effective application in real-world scenarios. Once confident in the model capabilities, it can be applied to predict prospective materials. This is where the theoretical meets the practical. Leveraging the power of modern technology, these predicted materials can be synthesized through automated experiments. However, predicting and synthesizing these materials is just the beginning. It is essential to scrutinize their actual properties and subject them to performance testing in real-world conditions.
The results from these performance tests are invaluable. They serve a dual purpose. Firstly, they provide a feedback loop for the data-driven model. If there are discrepancies between predicted outcomes and experimental results, the model can be refined and retrained, improving its predictive capabilities over time. Secondly, these tested and validated data points can be incorporated back into the original database. This enhances the richness and diversity of the database, ensuring it remains updated with the latest findings. Such iterative processes not only foster continuous improvement in the predictive accuracy of the model but also catalyze advancements in the broader realm of materials R&D. Over time, this synergy between data-driven modeling, automated synthesis, and rigorous testing can revolutionize the way materials are discovered, designed, and deployed in various applications.
In the realm of data collection and processing, traditional methods have often been found to be cumbersome, time-consuming, and prone to errors. As illustrated in Figure 2, the advent of digitization offers a transformative solution.
Figure 2. Digital technology accelerates the collection and processing of information in the FB development[33,39]. Data Extraction & New Data Generation: Reproduced with permission from ref.[39]. Copyright 2021 Royal Society of Chemistry. Feature selection & engineering: (A) Molecular weight distribution for the D3TaLES database; (B) Computed values for oxidation potential for sample molecules; (C) Scatter plot with histograms of D3TaLES molecules with calculated oxidation potential and radical stability score. Reproduced with permission from ref.[33]. Copyright 2023 Royal Society of Chemistry.
In the face of vast literature and unstructured data, AI tools have emerged as invaluable assets in identifying and sifting through this profusion to extract salient information. For instance, platforms such as IBM DeepSearch[30], encompassing the Corpus Conversion Service (CCS) and Corpus Processing Service (CPS), have become pivotal in gleaning meaningful insights from intricate formats such as PDFs. Parallelly, ChemDataExtractor can extract information from 229,061 battery research papers, thereby creating an extensive database of battery materials and their associated properties[31]. Beyond literature, new data acquisition avenues have been paved through innovative high-throughput experimentation processes. A prime example is the methodology fashioned by Liang et al., merging robotic automation with high-throughput techniques to amass extensive, quality-controlled solubility data[32].
After the collection phase, data processing emerges as a pivotal component, dictating the quality and efficacy of subsequent analyses. Using databases such as D3TaLES, SOMAS, etc.[33,34], the significance of feature engineering and feature selection becomes evident. D3TaLES employs density functional theory (DFT) computations to perform intricate feature engineering for redox-active small molecules, meticulously determining their critical physical and chemical attributes[33]. Through high-throughput screening, only the paramount oxidation properties are retained, epitomizing a streamlined feature selection process. Conversely, SOMAS zeroes in on the aqueous solubility of neutral organic molecules. Each molecule in this database is adorned with quantum descriptors derived from DFT and traditional molecular descriptors, a testament to its rigorous feature engineering. Moreover, the meticulous choice of molecule types and descriptors underscores the importance of feature selection. Both databases underscore the granularity and structured nature of data processing, laying a robust foundation for ensuing machine learning models and analytical pursuits.
Upon receiving data formatted for machine learning comprehension, the initial step encompasses model selection. The nature of the problem at hand dictates the most suitable model. For regression-oriented challenges, options such as linear regression, decision tree regression, random forests, and neural networks emerge as fitting candidates[29]. Conversely, classification problems might lean more toward logistic regression, support vector machines, or neural networks. Model initialization follows, wherein parameters are set. Models such as neural networks necessitate random parameter initialization, while others such as linear regression do not demand such a step.
The heart of the process is model training. Employing the training set, the model endeavors to learn the relationship between input data (descriptors) and target outputs, including conductivity. This phase is typically iterative, with each cycle refining the model parameters based on prediction discrepancies. In the context of neural networks, this encompasses forward propagation (for predictions), loss computation (e.g., mean squared error), backpropagation (for gradient calculations), and parameter updating mechanisms such as gradient descent. Introducing algorithms such as GRYFFIN[35] to this workflow heralds a transformative moment in material prediction. GRYFFIN, as an adept Bayesian optimization method, rapidly pinpoints candidate molecules boasting desired properties. Its efficacy becomes particularly evident when contrasted with conventional strategies, bestowing unmatched precision and efficiency during the prediction phase.
Despite the extensive screening of promising compounds, the sheer quantity of these compounds remains substantial, leading to a considerable backlog of materials awaiting experimentation. Consequently, the implementation of high-throughput experimentation through automation technologies holds significant potential for breakthroughs in this context. Foremost among these is the progression in robotics, enabling precise and rapid execution of complex experimental operations by robotic arms. Concurrently, developments in sensors and data acquisition technologies ensure real-time monitoring and recording of all critical parameters during experimental processes. The integration of cloud computing and big data technologies guarantees effective storage, processing, and analysis of the massive experimental data generated. Finally, the application of AI and machine learning algorithms opens avenues for extracting valuable information and patterns from this data. Collectively, these technological synergies not only enhance the efficiency of experiments but also ensure the accuracy and reliability of results, thus profoundly advancing the study of material science. A quintessential example of the prowess of automated experimentation hails from the research team at the Lawrence Berkeley National Laboratory in the United States. They pioneered a software platform named "ChemOS" that, in tandem with automated laboratory equipment, facilitates unattended chemical synthesis experiments[36]. In the realm of material development, leveraging this system enabled automated high-throughput screening, culminating in the successful synthesis and evaluation of several hundred distinct materials. This markedly expedited the discovery process of new materials, conserving considerable manpower and resources while propelling material R&D at an unprecedented pace. Similarly, researchers in the UK have instituted an automated experimental system capable of autonomously planning, executing, and analyzing electrochemical experiments[37]. In the quest for novel battery materials, this system, through its automated screening and testing, offers invaluable data and insights to researchers.
In the performance assessment phase for new materials, AI-driven experimental analysis tools are ushering in a new era[38]. During the critical phase of performance assessment for novel materials, there is a palpable buzz surrounding the integration of AI into experimental analysis tools. This revolution is poised to redefine the landscape of material science and its associated experimental methodologies. At the heart of these tools are advanced machine learning algorithms that can delve deep into vast amounts of experimental data. With an ability to decipher complex patterns, they precisely identify the optimal performance parameters, which might often elude traditional analysis techniques. Such granular insights hold monumental importance. On the one hand, they amplify the application potential of the material by providing clear guidelines on how to harness its maximum capability. On the other hand, they endow researchers with a treasure trove of data, which can be pivotal for refining and optimizing the material properties in subsequent iterations. Now, imagine coupling these AI-driven tools with the precision and efficiency of robotics. This union represents a watershed moment in the realm of material research. Robots, with their ability to consistently and accurately execute experiments, when guided by analytical prowess of AI, bring forth an unprecedented continuous feedback loop. This means that every single piece of data extracted from an experiment does not just stop at providing insights; it actively contributes to the ongoing training and refinement of machine learning models. Such a dynamic and iterative framework is a game changer. Not only does it exponentially increase the efficiency of each experiment by rapidly converging on desired outcomes, but it also instills a culture of perpetual learning. Every experiment, success or failure, becomes a lesson, a stepping stone toward perfection. This ensures that the entire R&D trajectory is not static but is a vibrant journey of continuous enhancements. As we stand at the cusp of this technological evolution, the promise is clear: a more streamlined, insightful, and perpetually improving landscape of material R&D. This is described in Figure 2[33,39].
A specific study on quinone electrolytes for RFBs highlights how machine learning and theoretical calculations can be combined to evaluate quinone molecules[40]. This process involves constructing a library of quinone molecules and using DFT and molecular dynamics (MD) simulations to calculate their properties. The study uses models such as eXtreme Gradient Boosting (Xgboost)[41] and Attentive Fingerprints[42] for the predictive analysis of quinone molecules. These models help in predicting properties such as solvation free energies and HOMO-LUMO gaps, which are essential for understanding the redox potential and solubility of the electrolytes in RFBs.
Researchers used active learning coupled with quantum mechanical simulations to explore RFBs. This approach enabled the efficient screening of a large number of redox-active polymers from a dataset, enhancing the discovery process. The team focused on designing redox-active polymers that could be electrically cleaved at a specific voltage to prevent the formation of an inactive film during battery operation. They used machine learning to identify molecules with the desired properties from a large dataset, showing a significant improvement over traditional methods.
Machine learning can rapidly screen and predict the best combinations of alkaline quinoxaline and acidic benzoquinone for optimal battery performance. For material property prediction, AI can predict key properties such as solubility, stability, and redox potential of these materials, leading to better design and faster development. The integration of machine learning in RFB research opens new avenues for innovative designs and improved functionality of alkaline quinoxaline-acidic benzoquinone flow batteries.
REVOLUTION IN DEVELOPMENT OF FLOW BATTERIES WITH METAVERSE
In a world dominated by rapidly advancing research methods, AI and robotics have left an indelible mark in the laboratory, ushering in an era of accelerated scientific discovery. AI has simplified data analysis, enhanced predictive capabilities, and provided researchers with profound insights, which makes various breakthroughs in academic society. Recently, researchers have used AI to predict the composition and properties of 2.2 million new materials, potentially revolutionizing fields such as electronics and solar cells[43]. Utilizing data from the Materials Project database, the AI, named GNoME, identified stable crystal structures and novel battery materials, with a success rate of 80% in predictions. This AI-driven approach promises to accelerate the discovery and development of new materials, marking a significant advancement in materials science.
At the same time, robotics technology has paved the way for the mechanization and optimization of many laboratory processes. However, despite their revolutionary impact, certain challenges remain evident: the most advanced robotic systems remain constrained by their physical environment, unable to adapt instantly to various experimental setups or be immediately available wherever needed. While AI and robotics technologies have introduced efficiency, real-time collaboration in physical laboratories often faces logistical challenges. Concurrently, executing multiple experimental scenarios in a physical environment is frequently impractical, thereby extending research timelines. Emerging from this backdrop is the metaverse: an immersive and persistent 3D environment that combines augmented reality (AR), virtual reality (VR), and the broader digital universe. At its core, the metaverse is a confluence of interconnected digital spaces, fostering real-time interactions that blur the lines between the physical and the virtual. Unlike a mere digital enclave, the metaverse unfolds as an expansive and interactive continuum where traditional distinctions are redefined, constraints are challenged, and opportunities continually emerge.
When the metaverse aligns with materials science, the landscape of new material development undergoes a profound transformation. Envision a realm where geographical boundaries become obsolete, where researchers from across the globe can congregate virtually, sharing their latest experimental findings without the conventional restrictions of location and time. Within this digital expanse, scientists can immerse themselves in vivid, real-time visualizations of ongoing or past chemical experiments. Such an interactive experience facilitates an intuitive understanding of complex processes, as researchers can manipulate virtual apparatus, observe reactions at any scale - be it atomic or macroscopic - and analyze data in unprecedented detail. Furthermore, the vast repository of shared knowledge and experiences within the metaverse can be harnessed to fuel the evolution of AI algorithms. As researchers input their expertise, AI systems become increasingly adept not only in predicting material properties but also in recommending innovative experimental pathways. Before initiating real-world automated synthesis, the virtual laboratories of metaverse offer a sandbox environment for preliminary synthesis trials. These virtual trials generate data that can refine and optimize the design of real-world automated experiments. Conversely, real-world experimental outcomes can be fed back into the metaverse, perpetuating a cycle of iterative improvement between the virtual and tangible realms.
Addressing foundational challenges, digitizing an entire experiment in the metaverse requires a comprehensive mapping of physical processes to virtual analogs. Advanced sensors and Internet of Things (IoT) devices can capture real-world lab data, which can then be rendered in the metaverse using high-fidelity simulations. Secure and efficient data transfer protocols, coupled with robust cybersecurity measures, ensure the integrity and confidentiality of shared research. The research paradigm with metaverse can be described in Figure 3. All the research modules can be integrated into the metaverse. The machine learning and various structural databases can be accessed through AI-Generated Content (AIGC) with proper prompts. The theoretical calculation tools, such as DFT and MD, can be delicately arranged as the backend computation scheme through cloud computing. In recent years, numerous autonomous and standardized experiment hardware and software have been developed. The hardware can generate high throughput experiment data for 24 h, which can be immediately collected in the metaverse surface through the hardware digital twin, which is a virtual model designed to accurately reflect a physical system. With all the modules interactable in the metaverse, the scientists can finish the whole research process during the continuous interaction with the computer. Through queries to the existing database, scientists can pick some candidates that they wish to study and synthesize. Then, they can design, operate, and observe the experiment through the experiment hardware digital twin, where the reaction information is synchronously available. During a long chemical reaction, scientists can analyze the intermediate state of reaction to judge whether the experiment parameters are appropriate through theoretical calculations, which provides explanations for experiment phenomena. Then, they can immediately revise or rerun the experiment instead of wasting time for the end of the current experiment. This is where human-in-loop optimization accelerates the experiment optimization process. After the optimization, the product state information and unexpected product can be added to the previous database.
To realize the above process, acquiring and analyzing in-situ experiment data is crucial, such as chromatography, Raman spectrum, etc. In the context of fluid batteries, Electrochemical Impedance Spectroscopy (EIS) and mass spectrometry are necessary. EIS is extensively used in analyzing internal resistances, charge transfer resistances and diffusion processes, which is both non-destructive and sensible to the studied system. Real-time mass spectrometry provides a comprehensive understanding of electrolyte composition changes, offering massive in-situ data for battery performance changes.
Virtual testing environments, while beneficial, have limitations in replicating real-world battery behavior. They may not fully capture complex physical and chemical interactions, environmental variations, and aging effects under different usage scenarios. This can lead to discrepancies between virtual predictions and actual battery performance. Additionally, ensuring the security of these environments is challenging. They are susceptible to cyber threats, data breaches, and unauthorized access, which can compromise the integrity of simulations and the confidentiality of sensitive data. Robust cybersecurity measures are essential to protect these environments from such risks. However, we believe these problems will be addressed in the future.
The marriage of the metaverse with the realm of flow battery research signifies a revolutionary leap forward. The metaverse facilitates rapid prototyping, enabling swifter simulations that herald unprecedented advancements in flow battery designs. Moreover, by preempting tangible tests with virtual ones, a marked conservation of precious resources ensures rare materials are utilized only when the chances of success are optimal. This virtual realm becomes the converging point for global researchers, knitting together a tapestry of diverse expertise and novel ideas in pursuit of refining flow battery technology. In this digital dominion, the boundaries of safety are expanded, permitting the risk-free exploration of potentially hazardous flow battery chemistries. Moreover, the perennial challenges of scaling - transitioning from lab-scale cells to expansive full-scale systems - are tackled proactively, with the metaverse offering high-precision simulations to iron out potential kinks. In essence, the integration of the metaverse with digital laboratories transcends mere technological advancement; it represents a transformative recalibration in the very ethos of scientific exploration. For disciplines such as flow battery research, this metamorphic shift has the potential to hasten landmark discoveries, bringing sustainable energy solutions tantalizingly closer to fruition.
CONCLUSION
The rapid technological evolution has ushered the R&D of flow batteries into a groundbreaking digital age. By integrating AI, robotics, and the expansive realm of the metaverse, we are not just transcending the confines of the physical world but also achieving unprecedented real-time global collaboration. The digital laboratory offers a limitless research space, enabling intricate battery chemistries to be simulated and tested in a virtual environment with speed and safety. This innovative approach not only conserves vital research resources but also amplifies the efficiency and security of R&D endeavors. Crucially, it provides a unique platform where scientists, engineers, and researchers can collaborate beyond geographical and cultural boundaries, united in their pursuit of sustainable energy solutions. This paradigm shift, particularly in flow batteries and other advanced technological fields, heralds a greener, more efficient, and interconnected future.
DECLARATIONS
Authors’ contributions
Made substantial contributions to the conception and design of the study and performed data analysis and interpretation: Zhu X, Zhao Y
Provided experimental, technical, and material support: Chen C, Dai G, Gao Y, Xu P, Feng S, He W
Availability of data and materials
Not applicable.
Financial support and sponsorship
This work was supported by the National Natural Science Foundation of China (No. 22179031; No. 22075240; No. 22109111); the Natural Science Foundation of Zhejiang Province (Grant No. LY22B030008; No. LQ22B030006); the Shenzhen Fundamental Research Foundation (No. JCYJ20210324142213036).
Conflicts of interest
All authors declared that there are no conflicts of interest.
Ethical approval and consent to participate
Not applicable.
Consent for publication
Not applicable.
Copyright
© The Author(s) 2024.
REFERENCES
1. Zakeri B, Syri S. Electrical energy storage systems: a comparative life cycle cost analysis. Renew Sustain Energy Rev 2015;42:569-96.
2. Aneke M, Wang M. Energy storage technologies and real life applications - a state of the art review. Appl Energy 2016;179:350-77.
3. Renewable energy policy network for the 21 century. 2017. Available from: https://www.ren21.net/wp-content/uploads/2019/05/GSR2017_Full-Report_English.pdf [Last accessed on 11 Mar 2023].
4. Bullough C, Gatzen C, Jakiel C, Koller M, Nowi A, Zunft S. Advanced adiabatic compressed air energy storage for the integration of wind energy. In Proceedings of the european wind energy conference; 22-25 Nov 2004, London, UK. Available from: https://www.nrc.gov/docs/ML1202/ML12026A783.pdf [Last accessed on 11 Mar 2023].
5. Harby A, Sauterleute J, Korpås M, Killingtveit Å, Solvang E, Nielsen T. Pumped storage hydropower. In: Transition to renewable energy systems; 2013, p. 597.
6. Huang H, Li D, Hou L, et al. Advanced protective layer design on the surface of Mg-based metal and application in batteries: challenges and progress. J Power Sources 2022;542:231755.
7. Wu J, Zhou T, Zhong B, Wang Q, Liu W, Zhou H. Designing anion-derived solid electrolyte interphase in a siloxane-based electrolyte for lithium-metal batteries. ACS Appl Mater Interfaces 2022;14:27873-81.
8. Wang W, Luo Q, Li B, Wei X, Li L, Yang Z. Recent progress in redox flow battery research and development. Adv Funct Mater 2013;23:970-86.
9. Chalamala BR, Soundappan T, Fisher GR, Anstey MR, Viswanathan VV, Perry ML. Redox flow batteries: an engineering perspective. Proc IEEE 2014;102:976-99.
10. Goldstein A. Federal policy to accelerate innovation in long-duration energy storage: the case for flow batteries. 2021. Available from: https://itif.org/publications/2021/04/07/federal-policy-accelerate-innovation-long-duration-energy-storage-case-flow/ [Last accessed on 11 Mar 2023].
11. Gür TM. Review of electrical energy storage technologies, materials and systems: challenges and prospects for large-scale grid storage. Energy Environ Sci 2018;11:2696-767.
12. Iwakiri I, Antunes T, Almeida H, Sousa JP, Figueira RB, Mendes A. Redox flow batteries: materials, design and prospects. Energies 2021;14:5643.
13. Yuan X, Song C, Platt A, et al. A review of all-vanadium redox flow battery durability: degradation mechanisms and mitigation strategies. Int J Energy Res 2019;43:6599.
14. Lu W, Yuan Z, Zhao Y, Li X, Zhang H, Vankelecom IFJ. High-performance porous uncharged membranes for vanadium flow battery applications created by tuning cohesive and swelling forces. Energy Environ Sci 2016;9:2319-25.
15. Jia C, Liu J, Yan C. A significantly improved membrane for vanadium redox flow battery. J Power Sources 2010;195:4380-3.
16. Lou X, Lu B, He M, et al. Functionalized carbon black modified sulfonated polyether ether ketone membrane for highly stable vanadium redox flow battery. J Membr Sci 2022;643:120015.
17. Wei X, Pan W, Duan W, et al. Materials and systems for organic redox flow batteries: status and challenges. ACS Energy Lett 2017;2:2187-204.
18. Larcher D, Tarascon JM. Towards greener and more sustainable batteries for electrical energy storage. Nat Chem 2015;7:19-29.
19. Ding Y, Zhang C, Zhang L, Zhou Y, Yu G. Molecular engineering of organic electroactive materials for redox flow batteries. Chem Soc Rev 2018;47:69-103.
20. Tabor DP, Roch LM, Saikin SK, et al. Accelerating the discovery of materials for clean energy in the era of smart automation. Nat Rev Mater 2018;3:5-20.
21. Wang C, Yang Z, Wang Y, et al. High-performance alkaline organic redox flow batteries based on 2-hydroxy-3-carboxy-1,4-naphthoquinone. ACS Energy Lett 2018;3:2404-9.
22. Tong L, Goulet M, Tabor DP, et al. Molecular engineering of an alkaline naphthoquinone flow battery. ACS Energy Lett 2019;4:1880-7.
23. Yu J, Salla M, Zhang H, et al. A robust anionic sulfonated ferrocene derivative for pH-neutral aqueous flow battery. Energy Stor Mater 2020;29:216-22.
24. Hwang B, Park MS, Kim K. Ferrocene and cobaltocene derivatives for non-aqueous redox flow batteries. ChemSusChem 2015;8:310-4.
25. Gerhardt MR, Tong L, Chen Q, Gordon RG, Aziz MJ. Anthraquinone derivatives in aqueous flow batteries. Meet Abstr 2016;MA2016-01:382.
26. Yang X, Garcia SN, Janoschka T, Kónya D, Hager MD, Schubert US. Novel, stable catholyte for aqueous organic redox flow batteries: symmetric cell study of hydroquinones with high accessible capacity. Molecules 2021;26:3823.
27. Lai YY, Li X, Zhu Y. Polymeric active materials for redox flow battery application. ACS Appl Polym Mater 2020;2:113-28.
28. Janoschka T, Martin N, Martin U, et al. An aqueous, polymer-based redox-flow battery using non-corrosive, safe, and low-cost materials. Nature 2015;527:78-81.
29. Li T, Zhang C, Li X. Machine learning for flow batteries: opportunities and challenges. Chem Sci 2022;13:4740-52.
30. Pyzer-knapp EO, Pitera JW, Staar PWJ, et al. Accelerating materials discovery using artificial intelligence, high performance computing and robotics. NPJ Comput Mater 2022;8:84.
31. Huang S, Cole JM. A database of battery materials auto-generated using ChemDataExtractor. Sci Data 2020;7:260.
32. Liang Y, Job H, Feng R, et al. High-throughput solubility determination for data-driven materials design and discovery in redox flow battery research. ChemRxiv 2023.
33. Duke R, Bhat V, Sornberger P, Odom SA, Risko C. Towards a comprehensive data infrastructure for redox-active organic molecules targeting non-aqueous redox flow batteries. Dig Discov 2023;2:1152-62.
34. Gao P, Andersen A, Sepulveda J, et al. SOMAS: a platform for data-driven material discovery in redox flow battery development. Sci Data 2022;9:740.
35. Häse F, Aldeghi M, Hickman RJ, Roch LM, Aspuru-guzik A. Gryffin: an algorithm for bayesian optimization of categorical variables informed by expert knowledge. Appl Phys Rev 2021;8:031406.
36. Roch LM, Häse F, Kreisbeck C, et al. ChemOS: orchestrating autonomous experimentation. Sci Robot 2018;3:eaat5559.
37. Boyce BL, Uchic MD. Progress toward autonomous experimental systems for alloy development. MRS Bull 2019;44:273-80.
38. Zhu X. Toward the uniform of chemical theory, simulation, and experiments in metaverse technology. Precis Chem 2023;1:192-8.
39. Li T, Lu W, Yuan Z, Zhang H, Li X. A data-driven and DFT assisted theoretic guide for membrane design in flow batteries. J Mater Chem A 2021;9:14545-52.
40. Wang F, Li J, Liu Z, Qiu T, Wu J, Lu D. Computational design of quinone electrolytes for redox flow batteries using high-throughput machine learning and theoretical calculations. Front Chem Eng 2023;4:1086412.
41. Chen Q, Gerhardt MR, Hartle L, Aziz MJ. A quinone-bromide flow battery with 1 W/cm2 power density. J Electrochem Soc 2016;163:A5010-3.
42. Xiong Z, Wang D, Liu X, et al. Pushing the boundaries of molecular representation for drug discovery with the graph attention mechanism. J Med Chem 2020;63:8749-60.
43. Merchant A, Batzner S, Schoenholz SS, Aykol M, Cheon G, Cubuk ED. Scaling deep learning for materials discovery. Nature 2023;624:80-5.
Cite This Article
Export citation file: BibTeX | RIS
OAE Style
Chen C, Dai G, Gao Y, Xu P, He W, Feng S, Zhu X, Zhao Y. Digitization of flow battery experimental process research and development. Energy Mater 2024;4:400019. http://dx.doi.org/10.20517/energymater.2023.91
AMA Style
Chen C, Dai G, Gao Y, Xu P, He W, Feng S, Zhu X, Zhao Y. Digitization of flow battery experimental process research and development. Energy Materials. 2024; 4(2): 400019. http://dx.doi.org/10.20517/energymater.2023.91
Chicago/Turabian Style
Chen, Changyu, Gaole Dai, Yuechen Gao, Peizhe Xu, Wei He, Shunan Feng, Xi Zhu, Yu Zhao. 2024. "Digitization of flow battery experimental process research and development" Energy Materials. 4, no.2: 400019. http://dx.doi.org/10.20517/energymater.2023.91
ACS Style
Chen, C.; Dai G.; Gao Y.; Xu P.; He W.; Feng S.; Zhu X.; Zhao Y. Digitization of flow battery experimental process research and development. Energy Mater. 2024, 4, 400019. http://dx.doi.org/10.20517/energymater.2023.91
About This Article
Special Issue
Copyright
Data & Comments
Data
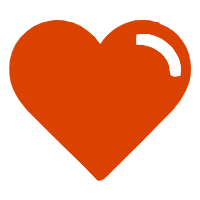

Comments
Comments must be written in English. Spam, offensive content, impersonation, and private information will not be permitted. If any comment is reported and identified as inappropriate content by OAE staff, the comment will be removed without notice. If you have any queries or need any help, please contact us at support@oaepublish.com.