Bioinspired nucleic acid-based dynamic networks for signal dynamics
Abstract
Signaling dynamic networks in living systems determine the conversion of environmental information into biological activities. Systems chemistry, focusing on studying complex chemical systems, promotes the connections between chemistry and biology and provides a new way to mimic these signaling dynamic processes by designing artificial networks and understanding their emerging properties and functions that are absent in isolated molecules. Nucleic acids, while relatively simple in their design and synthesis, encode rich structural and functional information in their base sequence, which makes them an ideal building block for constructing complex dynamic networks that can mimic those in living systems. This review briefly introduces nucleic acid-based dynamic networks that can mimic natural signaling dynamic processes. We summarize how the nucleic acid-based dynamic networks are utilized to mimic relatively simple biological transformations, such as feedback and feedforward, which act as sub-networks to produce complex dynamic behaviors upon collective integration. We also emphasize the recent development of far-from-equilibrium networks, which are designed for converting the spatiotemporal signal and coupling with the downstream systems to achieve different functionalities and applications, including temporary nanostructure and patterns, programmed catalysis, and more, using nucleic acid-based dynamic networks. We also address the challenges of developing nucleic acid-based dynamic networks by directed evolution, operating complex networks under confinement conditions, and integrating multiplex networks into cell-like containments aiming to create protocells with living features.
Keywords
INTRODUCTION
Life, which evolved from non-living components, represents the most sophisticated self-organization system in nature[1,2]. Even the simplest unicellular systems consist of multiple components that exhibit spatiotemporal reactions and interactions, giving these systems living properties and functionalities[3]. As a unique natural phenomenon, the prominent features of life entirely differ from those of non-living systems. For example, living systems can sense and process environmental signals to self-organize their structure and self-regulate their functionalities, which enable them to survive change-induced events[4-6]. Such fascinating features have inspired scientists from various disciplines. Recently, chemists have attempted to analyze and comprehend such living features at the molecular level[7]. From a chemical perspective, living characteristics are not only determined by the nature of the components but also by the nonlinear reaction and interaction networks present in living systems[8-10]. When it comes to understanding and manipulating living systems, it is essential to consider the comprehensive study of nonlinear molecule interaction networks at a system level, known as systems chemistry. This includes the organization and dynamics of molecular networks, the emergence of network-guided properties and functions, and the operation of critical biological processes through networks[11-16].
As a critical biological network in living systems, signaling dynamic networks determine the conversion of environmental signals into cellular activities[17]. These networks are essential in adaptation[18], regulation[19], and morphogenesis[20]. By sensing, connecting, and dynamically regulating signaling transducers in networks, external signals such as light[21], temperature[22], pH changes[23], and chemical inputs[24] can be transduced into corresponding biological signals in living systems. Diverse natural transformations in living systems operate through signaling dynamic networks, including feedback[25], feedforward[26], intercommunication[27], signal propagation[28], oscillation[29], steady-state transition[30], and more. By further integrating collective biological transformation processes, organisms could demonstrate adaptivity and autonomy during environmental communication[31,32]. Therefore, from a systems chemistry perspective, living characteristics can be regarded as emerging properties and functionalities that arise from complex dynamic networks.
The rational design of artificial systems that mimic the functions of living systems has been at the forefront of chemistry[14,33,34]. Benefiting from the development of biological understanding, molecular engineering, and supramolecular self-assembly, chemists have started synthesizing artificial dynamic networks to mimic natural signaling dynamic networks[35]. Various chemical networks were reported, including cascaded signaling networks[36], self-replicating[37] and signal-amplifying networks[38], constitutional dynamic networks and libraries[39], etc. However, there is still a long way to go in creating artificial systems that exhibit the major living characteristics of living systems, where multi-network systems can function like molecular intelligence, revealing sufficient reactivity, autonomy, adaptability, sociability, and evolvability.
Although nucleic acids are known as the building blocks of life, they are also modular and versatile motifs that have recently been used to construct sophisticated dynamic networks[35,40,41]. The well-characterized nature of DNA base-pairing makes it easy to control their self-assembly processes while also providing versatile means to reconfigure switchable DNA structures. These include pH-responsive C-G·C+ or T-A·T triplexes[42] and cytosine-rich strands into i-motif forms[43], fuel/anti-fuel strands-driven strand displacement reactions[44], and more. In addition, the nucleic acid sequences encode substantial structural and functional information, such as the sequence-specific recognition of low-molecular-weight ligands[45], macromolecules[46], and even cells (aptamers)[47]. Additionally, nucleic acids possess catalytic properties such as ribozymes[48] and DNAzymes[49]. The programmability of nucleic acid sequences and their functionality provides a rich module toolbox for precisely designing and manipulating defined structures and reaction pathways[50,51]. These are essential for the de novo construction of complex and diverse sets of artificial signaling dynamic networks. The review aims to present recent advances in nucleic acid-based dynamic networks for signal dynamics. First, we discuss nucleic acid-based dynamic networks capable of fundamental signaling dynamics, such as network adaptivity, feedback, feedforward, communication, and more. Then, we introduce nucleic acid-based far-from-equilibrium networks that can convert environmental cues into spatiotemporal variations of output signals, such as pulsed and periodic signals. Potential applications of nucleic acid-based dynamic networks in designing soft materials, pattern dynamics, catalysis, and protocols are addressed. These have expedited different research in various fields, such as systems chemistry, DNA nanotechnology, and materials science.
THE FUNDAMENTAL SIGNALING DYNAMIC PROCESSES
Biological transformations in living systems are often controlled by physically and chemically triggered signaling dynamic networks[52-55]. These networks receive, process, encode, and integrate ever-changing signals for conducting specific biological activities[9,56-57]. Recently, nucleic acids with programmable properties and functionalities have been used as building blocks to construct artificial dynamic networks for mimicking essential signaling dynamic processes[35,58].
Adaptive feature
The adaptive feature is fundamental to these signaling dynamic networks, enabling them to respond nimbly to changes in the environment[18,59]. Substantial research efforts have been directed toward assembling adaptive nucleic acid-based dynamic networks[60-63]. For example, Wang et al. proposed a design to construct an adaptive constitutional dynamic network (CDN) with four interconvertible nucleic acid constituents,
Figure 1. (A) Schematic assembly of a [2 × 2] nucleic acid-based constitutional dynamic network (CDN); (B) The modular construction of the constituents of [2 × 2] CDNs by integrating metal-ion-dependent DNAzyme units as reporter domains and triggering domains as functional units; (C) The orthogonal operation of a [2 × 2] CDN using different triggers and the subsequent recovery of CDN by respective counter-triggers. Figure 1C is quoted with permission from Wang et al.[63].
Under this antagonist-agonist model, the adaptive reconfiguration of CDN is achieved by different triggers, such as fuels/anti-fuels-triggered strand displacement processes[61], fuel strand-induced formation of T-A·T triplexes and their dissociation by anti-fuel strands[64], the light-induced stabilization/destabilization of nucleic acid duplexes by photoisomerizable trans-/cis-azobenzene intercalators[65], and the K+-ion formation of G-quadruplexes and their dissociation by crown ethers[63]. In addition, the programmable building block of the network allows the construction of complex multi-constituent CDNs[62,66]. Constituents with duplex structures can be extended to the constituents of the nucleic acid nanomachines, such as DNA tweezers[64], and 3D nucleic acid nanostructures[66], such as DNA tetrahedra, for the adaptive regulation of DNA nanostructure and nanomachine networks. Scaling a two-dimensional [2 × 2] CDN by increasing the number of constituents enhanced the complexity of the system towards organizing nine constituents in a
Feedback and feedforward
The feedback loop and feedforward loop are the overrepresented network structures in nature[67,68]. Many complex dynamic networks comprise feedback and feedforward loops[17,69-71]. For instance, autonomous oscillations require a delayed negative feedback process that pushes the system back by repressing the autocatalytic species[72]. Nucleic acid-based artificial dynamic networks with feedback and feedforward mechanisms have been well-studied[73-75]. For example, the adaptive features of nucleic acid-based CDNs were applied to assemble feedback-driven CDNs[75]. Figure 2A outlines the schematic diagram of feedback-driven adaptive reconfiguration of nucleic acid-based CDN[75]. The CDN is composed of four constituents, CC′, DD′, CD′, and DC′, each of which is equipped with four different Mg2+-ion-dependent DNAzymes as reporter units. In the presence of hairpin H1, DD′ modified with an additional Mg2+-ion-dependent DNAzyme as a signal activator unit cleaves H1 and yields fragment E1 for signal transmission. E1 stabilizes the signal receptor constituent CC′ through the complementary sequences and the formation of a K+-ion-stabilized G quadruplex (CC′-K+), leading to the upregulation of constituents CC'-K+ and DD′ and concomitantly downregulation of constituents CD′ and DC′. The upregulation of DD' further accelerates the catalytic cleavage of H1 for a positive feedback pathway driving the adaptive reconfiguration of CDN R. Alternatively, a negative feedback-driven adaptive reconfiguration of CDN R was also designed using hairpin H2 as an external trigger. Using experimental and computational means, the feedback-driven adaptive behaviors were illustrated by the time-dependent concentration changes of each constituent of CDNs. The feedback mechanisms provide promising means to construct the signal amplification platform beyond simulating natural networks.
Contrary to the feedback loop, the feedforward loop consists of an input signal (X) that regulates an intermediate signal (Y), while both X and Y regulate an output signal (Z)[72,76]. Haley et al. proposed using internal base-pairing mismatches within DNA duplex substrates to control their strand displacement reaction rates, as shown in Figure 3[77]. The mismatch position-dependent strand displacement reactions are demonstrated through experiment and simulation. During the strand displacement process, the mismatch position of the DNA duplex substrate can be chosen rationally to provide a hidden thermodynamic drive. The strand displacement reaction rates are highest when the mismatch is eliminated early but not immediately. And a mismatch positioned close to the toehold domain of the DNA duplex substrate increases the reaction rate by approximately two orders of magnitude.
Figure 3. Left panel: Nucleic-acid-based feedforward network driven by mismatch-induced competitive strand displacement reactions; Right panel: The time-dependent relative fluorescence intensity changes of different components in the feedforward network. The right panel of Figure 3 is quoted with permission from Haley et al.[77].
In contrast, a mismatch placed far from the toehold domain of the DNA duplex substrate has a minimal effect. Based on the mismatch position-dependent strand displacement reactions, a feedforward DNA reaction network is designed and constructed as a simple pulse-generating device. The network includes two competitive toehold-mediated strand displacement reactions with an asymmetrically placed mismatch in the duplex substrate OT, 18 bases from the 5' toehold and three from the 3' toehold. The displacement of strand T from OT using 3' invading strand I3 is kinetically favored. The reaction between OT and 5' invading strand I5 is thermodynamically favored as it is more excess than I3, and both eliminate the initially present mismatch. By subjecting a four-fold excess of I5 and a two-fold excess of I3 to a solution of duplex substrate OT, the kinetic-driven reaction of I3 in displacing strand O is activated, as the mismatch position of OT speeds up this process. This reaction is controlled by the competitive invader strand I5, where the product duplex I3T is displaced by the thermodynamically favored strand I5 to control the output through repression. One of the emerging properties of such feedforward networks is their transient response to persistent stimuli, characterized as a pulse generator where the system returns to its original steady state[78].
Communication
Feedback pathways are also constructed between two CDNs to assemble intercommunicating networks[79]. Figure 2B depicts the signal intercommunication between two CDNs, S and T[79]. The equilibrated CDN S and CDN T include four constituents, EE′, EF′, FE′, FF′ and constituents GG′, GH′, HG′, HH′, respectively. FF' in CDN S and GG' in CDN T are modified with two different Mg2+-ion-dependent DNAzymes as signal activator units. EE' in CDN S and HH' in CDN T are functionalized with a double-loop region that, in the presence of an appropriate trigger, can form a triplex structure and act as a signal receptor subunit, respectively. The two independent networks do not share components and lack communication without appropriate triggers. Subjecting CDN S and CDN T to two hairpin triggers, HHH' and HEE', leads to the cleavage of HHH' by FF' and HEE' by GG', respectively. The resulting fragmented strand HHH'-1 from the cleavage of HHH' is inclined to bind the signal receptor HH' of CDN T by forming a T-A·T triplex structure. It leads to the adaptive upregulation of HH' and GG' and downregulation of GH' and HG' in CDN T. Meanwhile, HEE'-1, generated from the GG'-induced cleavage of HEE', can stabilize EE' in CDN S, resulting in the adaptive reconfiguration of CDN S where EE' and FF' are upregulated, and EF' and FE' are downregulated. In short, the molecular signals communicate between two CDNs to regulate each other. The intercommunicating CDNs can act as modules for triggered, network-driven, and biocatalytic cascades and for the intercommunication of network-guided biocatalytic cascades[80,81].
FAR-FROM-EQUILIBRIUM SIGNAL DYNAMICS
As an open system, life is operated in a far-from-equilibrium manner, which requires a continuous inflow of external matter and energy to maintain its living functions[82]. Far-from-equilibrium dynamic behaviors are ubiquitous at all levels of biological processes[83], from molecule-fueled signal transduction[84], dissipative self-assembly of microtubules[85], and the contraction of muscle cells to the propagation of organisms[86]. Substantial efforts were directed at developing far-from-equilibrium networks to achieve spatiotemporal biological transformations[87-89]. This section presents recent advances in designing nucleic acid-based far-from-equilibrium networks for spatiotemporal signaling dynamics. It should be noted that the natural far-from-equilibrium systems are highly complex. Currently, it is far from able to design a network close to the complexity of the natural system.
Dissipative behaviors in networks
The organization of a high-ordered living system from disordered non-living components is accompanied by energy dissipation and entropy production[90]. Such energy dissipation leads to the transient formation of active structures in the living system and plays a vital role in characterizing the emerging system and its resulting properties[91-92]. Substantial research efforts have been directed toward designing an artificial dissipative network leading to adaptable systems with outstanding features[88-90,92-94]. Constructing a dissipative network needs the network to be initiated in an inactive state where reactions or interactions of the network are deactivated. And a fuel-driven network activation mechanism and a following fuel-consuming mechanism need to be encoded in the network. Adding the fuel transforms the network from an initial state to an active state by generating temporal output signals. Meanwhile, the fuel-consuming mechanism in the network starts to deplete the fuel and drive the system back to its original state. At one moment, the activating and deactivating processes of the network balance each other out. If the inflow of the fuel molecule is persistently faster than its depletion, the network is kept in a function-active condition. When fuel addition ceases, the network returns to the inactive state with the dissipation of the fuel molecule. Therefore, regulated by the inflow performance of the fuel, precise spatiotemporal control of the network structure and its output signals can be realized. Thanks to the programmability and predictability of DNA reactions, the rational design of the nucleic acid-based dissipative network is demonstrated[95-109]. In this section, we introduce the recent advances in nucleic acid-based dissipative networks for converting environmental changes to diverse transient signals from the driving model of physically and chemically fueled and enzyme-catalyzed systems.
Transient signals by physical and chemical fuels
The variations of physical and chemical triggers, such as the changes in light, pH, temperature, and chemical states, can be transduced to molecular signals with temporal features to control biological pathways in nature[83,85,110]. The versatile reconfiguration of nucleic acid structures allows us to easily set up artificial reaction networks to sense external changes and transduce them to accessible molecular signals for controlling downstream functions[35,88]. Inspired by nature, where the formation and breaking of disulfide bonds can regulate the structures and functions of proteins to control biological pathways kinetically, Grosso et al. designed a nucleic acid-based dissipative system by coupling allosteric DNA nanodevice and the dynamic sulfur modulator[111]. Figure 4A shows that the ligand-binding allosteric nanodevice is designed to be a stem-loop DNA structure modified with two tails acting as allosteric sites. A disulfide bond is conjugated to the middle of the DNA strand for a redox-responsive allosteric modulator complementary to the two tails of the nanodevice. Upon the addition of the disulfide-linked DNA modulator to the allosteric nanodevice, the interaction between the modulator strand and the allosteric sites of the nanodevice leads to the release of the ligand. Once the disulfide bond in the modulator is reduced to the two thiolated halves, the modulator splits into two short strands and spontaneously de-hybridizes from the nanodevice due to the low bonding affinity. As a result, the DNA allosteric nanodevice restores its ligand binding ability, and the system returns to its original state over time. The transient allosteric process of the nanodevice is highly tolerant. The released ligand can be used as a time-dependent signal to regulate biological activities temporally. The redox-induced disulfide-bond breakage was employed to control the assembly and disassembly of the DNA-based nanotubes kinetically[112]. The inhibitor strand locks the active strand of DNA tiles to block assembling the tubular structure. And the disulfide-modified DNA strand is designed to displace the inhibitor strand to trigger the assembly of the tubular structure. Concurrently, the disulfide-modified DNA strand is reduced into two short DNA fragments in the presence of a reducing agent and dissociates from the inhibitor strand, leading to the disassembly of the DNA nanotube. It is demonstrated that the chemical signals can be transducted using the nucleic acid-based network for transient regulation of DNA self-assembly.
Figure 4. (A) Dissipative operation of a nucleic acid-based nanodevice fueled by sulfur-disulfide reactions; (B) Schematic illustration of the transient DNA system induced by CPA- and NAA-fueled dissipative pH value changes. Figure 4A is quoted with permission from Grosso et al.[111]Figure 4B is quoted with permission from Mariottini et al.[113]. CPA: cyanopropanoic acid; NAA: nitroacetic acid.
In addition, dissipative pH value changes induced by chemical reactions are transduced by pH-responsive C-G·C+ or T-A·T triplex structures[113]. As depicted in Figure 4B, 2-(4-chlorophenyl)-2-cyanopropanoic acid (CPA) and nitroacetic acid (NAA) are selected as chemical fuels for the dissipative operation of two pH-responsive DNA nanodevices. As the typical pH modulators, CPA or NAA allows the time-dependent control of the pH value of the solution. By adding chemical fuel to the solution, the pH value of the solution can be rapidly decreased. Meanwhile, the chemical fuel gradually dissipates through decarboxylation, leading to an increased pH value back to the initial state. In the first case, a DNA-based nanoswitch is designed to be in two switchable conditions that respond to the pH value changes, a duplex form at a high pH value and an intramolecular triplex form at a low pH value. The dissipative CPA pH modulator is employed to induce the transient transformation of the nanoswitch between the duplex form and triplex form. In the second case, a reconfigurable DNA receptor is designed to include a pH-responsive stem-loop domain for the structural transformation and a cargo-strand bonding site for the uptake of the DNA cargo strand. The NAA fuels the release/uptake of the cargo strand. Adding NAA to the system leads to an acidic condition and the transformation of the DNA duplex structure to the triplex form. This structural reconfiguration destabilizes the interaction between the cargo strand and the DNA receptor, releasing the cargo from the nanostructure. With the dissipation of NAA, the pH value returns to the neutral condition, and the triplex structure is dissociated. As a result, the receptor restores its cargo-uptake ability and reloads the cargo strand.
Furthermore, by introducing photo-sensitive molecules, light can be an ideal fuel source for constructing dissipative systems[114-116]. Compared to the above chemical fuels employed in the dissipative operation of DNA-based systems, light presents a series of advantages, including the diverse options in the intensity and wavelength, the convenient control in time and space, and no waste molecules accumulated in the system. For example, Wang et al. utilized an azobenzene-modified DNA strand (F) as a photo-sensitive molecule to operate the dissipative behavior of the DNA-based system[116]. The trans-azobenzene units can intercalate into the DNA duplex structure and stabilize the resulting DNA structure (Ft/G). Upon UV light irradiation (λ = 440 nm), trans-azobenzene units are photoisomerized to the cis-state, which leads to the dissociation of the DNA duplex. The strand-displacement reaction between the released strand G and the Y-shaped structure H/I/J/K leads to the formation of G/J/K and H/I. Under the heat condition, the cis-azobenzene unit is thermally dissipated back to the trans-azobenzene state. Then, the trans-azobenzene-modified strand F displaces strand G from the structure G/J/K to recover the duplex structure (Ft/G) and the Y-shaped structure (H/I/J/K). The repeatable dissipative behavior of the system is presented by the time-dependent concentration changes of intermediate structure I/H.
Beyond the transformation of the physical and chemical changes to the transient DNA signals, nucleic acid-based dynamic networks can generate more complex dynamic behaviors driven by enzymes and DNAzyme[106,116-120].
Transient signals by DNAzyme-catalyzed reactions
Metal-ion-dependent DNAzymes have been applied to drive nucleic acid-based far-from-equilibrium networks for years[116,117]. Recently, Wang et al. reported a gated dissipative network operated by the Pb2+-ion-dependent DNAzyme, as shown in Figure 5[116]. Two gates in the network act as thresholds, enabling the network to decide which transient signal can be reported. The network includes a duplex AB, where A is a Pb2+-ion-dependent DNAzyme sequence, and its catalytic activity is blocked by B; strands C, D, and E are modified with Cy5, BHQ2, and Cy3 and act as reporter units for generating output signals. After adding ribonuclease-containing fuel strand T, duplex AB is displaced to form duplex AT and release single-strand B, which can activate the reporter units by forming duplex structures BCD and BDE. The spatial proximity between Cy5 and BHQ2 in BCD and between Cy3 and BHQ2 in BDE leads to the quenching of Cy5 and Cy3 as competitive output signals due to the two reporter units sharing common strand D. Due to the release of blocker B from AB, the catalytic activity of DNAzyme A is transiently turned on for the cleavage of fuel strand T, leading to the backward strand displacement reaction. Then reporter units BCD and BDE are dissociated, and duplex AB is re-formed, displaying an integrated dissipative reaction cycle. The gated operation of DNAzyme-guided dissipative networks is achieved by controlling the signal pathway between two competitive signal reporter units. Upon the addition of fuel strand T, and in the absence of the inhibitors I1 or I2, two signal reporter units, BCD and BDE, are transiently activated; however, in the presence of I1, the transient gated activation of signal reporter unit BDE occurs, while in the presence of inhibitor I2, the temporary gated operation of signal reporter unit BCD proceeds.
Figure 5. Schematic illustration of gated dissipative networks operated by the Pb2+-ion-dependent DNAzyme. This figure is quoted with permission from Wang et al.[116].
Furthermore, a dissipative three-layer cascaded network is designed based on auto-inhibition processes of Mg2+-ion-dependent DNAzymes[105]. Three coupled sub-networks are constructed to execute a cascaded signal process to control the transient formation and depletion of three different Mg2+-ion-dependent DNAzymes. For example, the sub-network I consists of the toehold-functionalized duplex AA', Mg2+ ions, and corresponding ribonuclease-containing hairpin fuel H1. Subjecting the sub-network I to the fuel T1 results in the formation of Mg2+-ion-dependent DNAzyme AT1. Hairpin fuel H1 acts as the substrate of DNAzyme AT1 to be cleaved into two fragmented strands, H1a and H1b. The resulting strand H1a can displace the strand T1 from AT1 to generate the waste strand H1T1, leading to the disassembly of the Mg2+-ion-dependent DNAzyme AT1 for the re-formation of AA'. And the other fragmented strand, H1b, is used as the cascaded signal to unlock the fuel strand T2 from the coupler ST2 for operating the next-layer sub-network. Two additional dissipative sub-networks, II and III, are designed following a similar design principle. Sub-network II consists of the duplex BB' and hairpin fuel H2. Upon the release of fuel T2, the transient operation of DNAzyme BT2 is activated, and the cleavage of H2 yields functional fragments H2a and H2b. H2a interacts with T2 to dissipate DNAzyme BT2 in sub-network II. H2b is a cascaded signal to trigger the release of fuel strand T3 that activates the transient operation of sub-network II to generate molecular signals H3b and C'. These signals can be used as regulatory signals for the downstream functional system, such as polymerase/nicking machinery and the rolling circle polymerization machinery for complex transient biocatalytic transformations.
Transient signals by enzyme-catalyzed reactions
Nucleic acids are involved in various biochemical reactions, providing infinite possibilities for constructing complex reaction networks[51]. Different enzyme-catalyzed DNA reactions were already used to build dissipative networks for transient signals[32,106-107,109,118-121]. For example, RNA strands are fuel molecules to drive dissipative systems. And RNase H, as the fuel-consuming catalysis, decomposes RNA strands in RNA/DNA heteroduplexes for the dissipation of fuels[106,118-121]. By integrating this dissipative mechanism and DNA-based strand displacement reactions, the variations of RNA strands, such as base sequences and concentrations, are transduced to different transient molecular signals, including DNA strands[106], small bioactive molecules, ATP, cocaine[118], and even the structural information of DNA structures[120,121]. The RNase H-catalyzed dissipative strand displacement reaction also allows us to control the delay time of molecular signals, which is vital to program time-dependent biological activities[122]. Figure 6 illustrates that the nucleic acid-based timing network contains three blocker-inhibited strand displacement reaction pathways[122]. Three-type blocker strands, RNA blocker, DNA blocker containing the modified base 8-oxo-7,8 dihydroguanine (Goxo-blocker), and DNA blocker containing four deoxyuridine mutations (uracil-blocker) are designed to inhibit respective toehold-mediated strand displacement reactions by binding to the toehold domains of the target DNA strands. And RNase H, formamidopyrimidine DNA glycosylase (Fpg), and uracil-DNA glycosylase (UDG) decompose the corresponding blocker strands and unseal strand displacement reactions, respectively. The kinetics of the blocker enzymatic degradation thus determines when the blocker-inhibited strand displacement reactions start. By controlling the concentrations of the blocker strands and the enzymes used in the network, the network enables the orthogonal modulate of the delay times of different signals for potential regulation of downstream biological activities.
Figure 6. Schemes of the nucleic acid-based timing network driven by different enzymatic nucleic acid reactions: (A) RNase H-driven digestion of RNA blocker in RNA-DNA hybrid duplex; (B) Fpg-induced decomposition of DNA blocker containing deoxyuridine mutations; (C) UDG-guided degradation of Uracil-modified DNA blocker. (D) Orthogonal timing operation of strand-displacement reactions by three enzymes. This figure is quoted with permission from Bucci et al.[122].
Adenosine triphosphate (ATP), one of the most important energy materials in living systems, is essential for many enzyme-catalyzed biological reactions[123-125]. In the past few years, Walther et al. introduced ATP as a fuel molecule to design a series of nucleic acid-based dynamic networks through enzymatic DNA reactions[32,107-109,115,126]. For example, they reported T4 DNA ligase and an antagonistic restriction enzyme, BamHI, driving a nucleic acid-based dissipative network[126]. T4 DNA ligase can ligate DNA oligomer substrates encoded with complementary sticky ends at both sides with the consumption of ATP. The chemical energy stored in ATP transfers into the covalent phosphodiester bond in the DNA backbone, allowing for the formation of the transient DNA polymer structures. At the same time, the BamHI cleaves and depolymerizes the DNA polymers at specific sites just ligated, producing the original DNA oligomers and allowing the network to return to its initial state. It should be noted that the ligation reaction must be faster than the restriction reaction, allowing for the transient formation of the DNA polymers. The dynamic behaviors of output signals, such as the intensities and lifetimes, can be regulated by the concentrations of ATP fuels, the variation of the enzyme ratio of T4 DNA ligase and BamHI, and the reaction temperature.
By coupling ATP-fueled DNA ligation and enzyme-controlled restriction, this network holds great potential to program complex dynamic behaviors. By introducing the molecular recognition sites in DNA structures and multi-components into the dissipative network, hierarchical transient behaviors are programmed from the ATP-driven dissipating molecular recognition into cooperative, transient multivalency to transient recognition of colloidal partners to program fuel-driven encapsulation and self-assembly of colloid/DNA hybrids on a system level[109]. In addition, mechanisms of the light control were also integrated into the ATP-fueled dissipative networks[115]. Two different strategies, the light activation of fuel and the light activation of DNA substrates of enzymatic reactions, are applied to realize the light activation, light modulation, and multiple wavelength-dependent dynamic steady states in networks. These networks display the modularity of ATP-fueled dissipative DNA-based networks and the ability of ATP-fueled dissipative networks as regulatory tools to program complex time-dependent signals. More functional complexities are achieved by introducing the functional subunits into dissipative networks. The ATP-fueled ligation of DNA structures with functional subunits results in an intact functional unit, while enzymatic restriction separates them again due to unstable hybridization of the intermediate structures. Relevant functions are exemplified from FRET to multivalent sensing to DNAzymes with catalytic function[107,109,126].
Complex far-from-equilibrium signal dynamic behaviors
Integrating numerous sub-networks with different composites and functionality is a promising approach to constructing complex dynamic networks with enhanced functionalities. The synthetic signaling dynamic network is entering a new stage with the development of the integrating strategy. Walther et al. designed a set of toehold-mediated strand displacement reactions as signal conversion modules[32,108,109,127]. Integrating the ATP-fueled network as a transient signal generator and strand displacement reactions as signal conversion modules assembles the ATP-driven dissipative networks with self-resetting and cascaded behavior[108]. This work paves the way for network-guided regulation of the downstream system. Coupling ATP-fueled dissipative networks to DNA assemblies allows temporary control of DNA nanostructures. ATP-powered DNA ligation induces the transient DNA strand displacement process for activating the self-assembly of DNA nanotubes and restriction-induced backward reactions trigger the degradation of the resulting DNA nanotubes[32]. More interestingly, the reciprocal feedback reaction pathway can be designed to concatenate two sub-networks to form a communicating system for an ATP-driven automaton[127].
Recently, Sun et al. reported a signal communication and cross-regulation system based on dissipative DNA reaction networks, as depicted in Figure 7[127]. The system includes one signal-sender network by fuel-driven RNA transcription and two signal-receiver networks by ligation-digestion strand displacement reaction networks. In the signal-sender network, A transcriptional template is designed to generate an RNA signal, where the activation and deactivation of RNA transcription are controlled by the fuel-driven ligation/digestion reaction guided by the formation and dissociation of the T7 promoter domain. Instead of the T4 DNA ligase, E. coli DNA ligase is used to ligate DNA fragments with 3'-OH and 5'-phosphate ends with β-nicotinamide adenine dinucleotide (NAD+) fuel. At the same time, BsaI allows the enzymatic digestion of the resulting DNA template. The signal-receiver network includes two NAD+-fueled dissipative sub-networks undergoing similar ligation-digestion reactions. The transient RNA signal from the sender network enters the receiver network for signal regulation. The signal systems display four types of signal output patterns, repression, promotion, repression-recovery, and promotion-stop, regulated by the variations of different DNA sequence designs and enzymes involved in the system. For example, in the repression process, RNA is designed to interact with the reactive monomer via toehold-mediated strand-displacement reactions, which inhibits the ligation of two reactive monomers into dimer structures, partially shutting down the receiver network and repressing signal outputs. By adding RNase H to degrade the RNA simultaneously, RNA in the form of a non-reactive monomer is digesting to reactivate the NAD+-fueled dissipative sub-network in the receiver network. Thus, the signal pattern transforms from a repression form into a repression-recovery form.
Figure 7. Schematic design of communication and cross-regulation system integrating NAD+-fueled dissipative RNA transcription sub-network and toehold-mediated strand displacement reactions sub-networks. This figure is quoted with permission from Sun et al.[127].
As analogous to the signal dynamic in cellular gene regulatory networks, a synthetic RNA transcription machinery based on T7 RNA polymerase and RNase H, genelet, was designed to produce and degrade RNA signals for establishing a signal turnover process. It provides a powerful tool for assembling in vitro transcriptional regulatory networks, such as oscillating and bistable networks, for complex signaling dynamics[128-132]. For example, the delay-negative feedback oscillator and the three-repressor ring oscillator were constructed based on modular RNA transcription machines[128]. These networks consist of 2 or 3 switchable transcriptional templates. Each transcriptional template contains an incomplete promoter site for T7 RNA polymerase. When a DNA activator strand completes the promoter site, nucleotide triphosphates (NTPs) fuel the transcription of an RNA signal that can regulate the transcription of the target genelet by controlling the availability of the corresponding DNA activator through the strand displacement process. Therefore, regulatory models, such as negative feedback loops, can be programmed by designing the nucleic acid sequences of transcriptional templates. In addition to RNA signal production by activated transcription, RNA signals are also degraded by RNase H to maintain the continuous dynamic of the system. The resulting oscillating network can generate periodic RNA signals for regulating downstream functional systems. Relevant applications include a synthetic biochemical clock in a cell-like environment, dynamic patterns, molecular motion, production timer, and more[129,133]. In addition, Schaffter et al. reported bistable networks using genelets as building blocks[131]. The standard bistable network consists of two reciprocally-inhibited genelets. Two DNA activators are designed to control the transcription of the genelet, respectively. When one DNA activator binds to its corresponding promoter domain to activate the transcription of the genelet, the newly transcribed RNA signal represses the transcription of another genelet by replacing its DNA activator from the promoter domain of the second genelet. Moreover, RNA bound to DNA is degraded by RNase H, freeing DNA activators. It displaces typical bistable dynamic behaviors, which means only one genelet can be activated at a time. The resulting bistable network can change its states in response to different environmental signals and coordinates the temporal regulation of state-specific downstream signal dynamics.
Furthermore, Schaffter et al. develop a hierarchical engineering strategy for building more complex network systems, as shown in Figure 8[87]. At the fundamental level of reactive nodes, they created the hairpin clamp (HPC5) genelet toolbox with three states (OFF/ON/BLK) to improve the performance of genelet nodes. When the T7 RNAP promoter sequence of a genelet is incomplete, transcription is almost non-existent (defined as OFF state). When a DNA activator completes the promoter domain, transcription is switched to ON. Transcription disappears when a DNA blocker replaces the DNA activator (BLK). Genelets can also regulate one another through upstream transcription, either by activating or blocking downstream genelets to trigger state transitions. A series of modularized, interchangeable nodes with similar performance and minimum crosstalk form a standardized library. Engineering these standard nodes into feedforward and feedback loops creates functional modules. For example, these nodes are used to design three incoherent feedforward loop modules that produce pulses of genelet activation via upstream transcription. Finally, multiple incoherent feedforward loop modules are coordinated into a composite network that generates sequential pulsed signals with tunable pulse shapes and delay time. Beyond that, these elements are also used to engineer a switchable tristable network, which includes three mutually repressive bistable sub-networks. And integrating incoherent feedforward loop modules into a switchable multistable network leads to more extensive composite networks that produce state-specific pulsed signals. For instance, two different states of a bistable module each had a distinctive IFFL pulse. These networks with complex signaling dynamic behaviors demonstrate that the standardized far-from-equilibrium genelet toolbox broadened the prospect of autonomous chemical systems by constructing large-scale networks with enhanced complexity and functionality.
Figure 8. A scalable engineering strategy for building far-from-equilibrium chemical networks. (A) Schematic illustration of (A) a node in OFF/ON/BLK states; (B) State-transitions of a node; (C) Overview of the modular assembly from standard nodes, functional modules to multimodule networks. This figure is quoted with permission from Schaffter et al.[87]
At the same time, Rondelez et al. developed a modular DNA Polymerase/Exonuclease/Nickase (PEN) tool for assembling complex dynamic systems[134-137]. The PEN tool executes three cascaded reactions catalyzed by the respective enzyme. First, a DNA strand acts as a primer to bind a template strand, forming the primer/template structure. Using deoxynucleotide triphosphate (dNTP) fuels, the activated template can be recognized by a polymerase and elongated to a stable full duplex. Second, the template is designed with the recognition sequence of the nicking enzyme. The nicking enzyme binds a specific recognition site positioned in the polymerized DNA duplex leading to the cleavage of only the polymerized side of the DNA duplex, thereby releasing a short DNA output strand. Third, except for the template, the exonuclease recognizes and degrades the single-strand DNA strands involved in the PEN toolbox. Therefore, these enzyme-catalyzed reactions constitute a dissipative reaction network for the single-strand DNA signal production and decay cycle. Programming the sequences of DNA templates allows PEN to regulate each other by interacting with activators or inhibitors. These are basic signaling dynamic processes to build complex dynamic systems. Diverse in vitro DNA networks, such as oscillating and bistable networks, have been reported using the PEN toolbox[134-139]. The previous review effectively summarized their dynamic behaviors and provided detailed design principles[140].
Recently, Dehne et al. reported that PEN-based reaction networks could generate periodic signals for the dynamic control of colloidal self-organization, as shown in Figure 9[139]. To program the complex dynamic behaviors on a mesoscopic scale, two different fluorescent-labeled colloidal particles with a size of 1 µm are functionalized with two different DNA single strands. The dynamic aggregation of these particles can be controlled using complementary linker strands. And a dNTP-driven predator-prey oscillating network is designed to guide the periodic aggregation of colloidal particles. The system includes four different functional modules. An autocatalytic reaction module involves a DNA polymerizing process and the following sequence-specific nicking reactions that lead to the primer-activated production of itself for amplifying its concentration. The primer strand can initiate two downstream reactions: the production of the complementary linker strand for triggering the aggregation of colloidal particles and the negative-feedback polymerizing reaction to block the primer strand by forming a stable DNA duplex. The exonuclease degrades the primer, the blocked primer, and the complementary linker in their single-strand state. With this design, the periodic concentration change of the complementary linker strand is realized to drive the autonomous oscillatory colloidal structure formation and dissociation without the addition of external stimuli.
Figure 9. Schemes of enzymatic reaction network based on the PEN toolbox to control colloidal periodic self-organization, where the network consists of three functional modules, linker production, activation, and inhibition. This figure is quoted with permission from Dehne et al.[139].
Nucleic acids have been used to store and process information for a long time[51]. DNA circuits that act as the central processing unit (CPU) execute diverse analog computing operations[141]. As artificial neural networks (ANN) are revolutionizing electronic computing, DNA computing researchers turn their gaze to mimic biological information processes[141-143]. Biomimetic networks were used to imitate massively parallel and recurrent architectures, analog and asynchronous operation, and fault-tolerant and redundant computations. However, biological information processes, such as genetic information encoding and decoding and signaling dynamic processes, typically operate nonlinearly, allowing the biological system to manipulate molecular information precisely[57]. This is essential to transduce biological information to complex natural behaviors, such as self-organization[144], cell differentiation[145], and migration[146]. Recently, Okumura et al. used PEN tools to build neuron networks with tunable weights and biases, including positive weight, negative weight, weight adjustment, amplification, bias, and reporting units[143]. Specifically, the inputs are divided into positive and negative weights. The positive weight inputs will bind to the converter templates and produce an output strand with DNA polymerase and nickase. The negative weight operation produces the complementary strand, which deactivates the strand generated by the positive weight process. At the same time, the weight adjustment template competes with the above two weight templates to adjust the weights. When the weighted sum exceeds a given tunable threshold, the neuron is transformed into an ON state by the autocatalytic exponential replication of the output signal. Throughout this process, the exonuclease continuously degrades all single-stranded DNA to maintain nonlinear dynamic behaviors. The neuron is powered by these far-from-equilibrium DNA weighting reactions for discriminating tiny differences in the input concentration to generate an output signal. In addition, the output signal can then regulate a downstream, connected neuron. Designed neurons are assembled in multilayer architectures to classify nonlinearly separable regions and perform nonlinear decisions with higher sensitivity than simple DNA networks. The modular design of the PEN-based neuron network highlights the potential to expand and enhance the nucleic acid-based dynamic network to make it “smarter”.
SIGNALING DYNAMICS IN CELL-LIKE ENVIRONMENTS
A cell is the smallest unit of life, in which tens of thousands of genes and countless biomolecules coordinate to perform cellular functions through complex dynamic networks[147]. Despite the progress of nucleic acid-based dynamic networks operated in the test tube, it is still challenging to integrate nucleic acid-based dynamic networks into cell-like containments to design and construct “artificial cells” for mimicking cellular functions. In the following sections, we exemplify the operation of nucleic acid-based dynamic networks in cell-like containments to mimic the properties and functionalities of living cells, including cellular adaptive behavior, signal amplification, information processing, intercommunications, and more.
Cellular signaling dynamic systems involve interwoven molecular reaction networks to transduce information from external environmental stimuli[148-149]. Constructing artificial cells that receive and process signals is of great significance. Peng et al. designed a DNA-based artificial molecular signaling system capable of receiving, transducing, and responding to calls from an outside stimulus[150]. Figure 10 depicts a schematic diagram of synthetic signaling dynamic system based on a cell-mimicking giant membrane vesicle (GMV). The system consists of internal dynamic networks and artificial membrane structures with nanogatekeepers. The nanogatekeeper is a switchable artificial ion nanochannel to control the ion permeability of membrane structures in response to external stimuli, opening in the presence of ATP and closing by generating the blocker strand. Without ATP, the locker strand binding the top region of the mimetic ion nanochannel plugs the DNA nanogatekeeper. The tight membrane structure of GMV isolates internal signaling dynamic networks from the environmental Zn2+ ions and Sr2+ ions, preventing them from triggering the network. Subjecting the system to ATP leads to the switch of the nanogatekeeper from the closed state to the open state due to the high binding affinity of the locker strand with ATP. Then, metal ions outside the GMV, such as Zn2+ ion and Sr2+ ion, diffuse from opened ion nanochannels inside the GMVs, triggering artificial signaling dynamic systems consisting of signal amplification, signal transduction, and signal reporting and feedback process. In the presence of Zn2+ ions, the signal amplification process is activated by Zn2+-ion-dependent DNAzyme, which cleaves the substrate Sub-I to produce the DNA fragment strand S-I. Strand S-I acts as additional catalysis to initiate the downstream toehold-mediated strand displacement reactions, which makes strand S-II. Then, S-II binds to the target strand T, forming a duplex T/S-II recognized explicitly by endonuclease Pvu II. This leads to the degradation of T into multiple fragments, inducing rolling circle amplification (RCA) with SYBR Green I, a fluorescent dye used to detect the final degradation products and characterize the whole system. Besides the above signal transduction process, a feedback pathway is designed to regulate the state of the nanogatekeeper embedded in the membrane of GMV. With Sr2+ ions diffusing into the GMVs, the Sr2+-ion-dependent G-quadruplex-driven DNA strand displacement reaction also occurs, thereby releasing the single-strand blocker, which blocks the bottom region of the DNA nanogatekeeper to the closed state.
Figure 10. Schematic illustration of DNA-based artificial molecular signaling system based on a cell-mimicking giant membrane vesicle. This figure is quoted with permission from Peng et al.[150].
Natural signaling dynamic networks can distinguish meaningful stimuli from weak noise. To mimic this process, Wang et al. designed an adaptive synthetic cell based on a nucleic acid-based network termed molecular central processing unit, which can sense and process continuous dynamic fluctuation of environmental stimuli[151]. The DNA nanopores embedded in the membrane of the artificial cell allow for K+ ions diffusion driven by osmotic pressure. The network system within the synthetic cell includes three functional modules: a signal converter, a fluctuation filter, and a signal-intensity accumulator. The signal converter senses the environmental concentration fluctuation of K+ ions and converts them to the intracellular concentration fluctuation of DNA analog. And the fluctuation filter includes four incoherent feedforward loops and a threshold gate for the concentration filtration of the DNA analog. When the fluctuation filter determines that a concentration of DNA analog is similar to weak noise (below the concentrations threshold), it does not conduct the downstream pathway; however, if the concentration is above the threshold, the filter delivers a signal to the signal-intensity accumulator. Then the signal-intensity accumulator records the dynamic signals transmitted from the fluctuation filter through molecular catalysis. Through the construction of a mixed-cell community, the synthetic molecular system can sense, analyze, and process meaningful stimuli from the environment or the release of natural cells rather than weak noise. This study contributes to constructing artificial molecular biological systems with more bionic functions and even specific living activities and provides a new way to understand and regulate the natural biological reaction networks.
Beyond intracellular signaling dynamics, the communication between the synthetic cells that represent the sociability of artificial cells is critical to developing complex living systems[152-156]. Joesaar et al. proposed a general approach to engineer intercommunication networks between the populations of protocells, as shown in Figure 11[155]. Each of the protocells in the populations is made of two parts, a protein-based microcapsule mimicking the semi-permeable membrane of the natural cell and a nucleic acid-based reaction network module acting as the information-processing unit. The protein-based microcapsule, named proteinosome, is permeable to short (< 100 bases), singlestranded nucleic acids and is highly suitable for developing a protocellular communication platform. The nucleic acid-based reaction network module is designed to encode and decode molecular information through enzyme-free DNA strand-displacement reactions. It is modified with streptavidin for encapsulation in the proteinosome. Individual protocells can be engineered to perform various tasks, such as signal detection, signal transduction, signal cascading, signal amplification, feedback operation, and Boolean logic operations. The programmability and orthogonality of DNA reactions make DNA strands ideal molecular information to minimize unintended cross-interactions in protocellular communities. Several populations of protocells can exchange information simultaneously on crisscrossed pathways, and one population can efficiently transfer messages to several other populations. Therefore, compartmentalized nucleic acid-based network modules allow collective signaling dynamics in the protocell populations, such as multiplex sensing, cascaded amplification, bidirectional communication, and distributed logic operations. In addition, transcriptional genelet and CRISPR/Cas-based DNA machinery were also applied to develop protocellular signaling networks for mimicking cellular features[156].
Figure 11. (A) A general scheme for protocellular communication of DNA-encapsulating protocells; (B) The collective signaling behaviors involved in protocellular communication; (C) The preparation of the protocellular system. This figure is quoted with permission from Joesaar et al.[155]
Similarly, the transcriptional genelet module is established and encapsulated in the protocells. The encapsulation and activation of DNA templates were demonstrated by generating RNA aptamer signals in the presence of T7 RNAP and external DNA trigger strands. Then, the functional transcription genelet module encapsulated in the protocell generates diffusive RNA signals, which could be sensed by the neighboring protocells and trigger the signal cascade or the localization of Cas nucleases in the neighboring protocells. These findings highlight the opportunities for combining the programmability of DNA nanotechnology with the capabilities of semi-permeable proteinosome in protocellular signaling networks and provide a further step towards complex signaling dynamic behaviors in protocells.
The above examples show how the information encoded in the sequence of nucleic acids regulates chemical reactions and inter-molecular interactions to produce nucleic acid-based dynamic networks for information processing. Combining the nucleic acid-based dynamic network and compartmentalized systems represents a new way to construct protocells with living features. These understandings are crucial in laying the groundwork for building complexity in multi-component systems and highlighting the potential of nucleic acid-based dynamic networks for signal transduction in artificial cells.
CONCLUSION AND OUTLOOK
Living systems illustrate the importance of the organization of interacting components in complex dynamic networks[9-10]. DNA, RNA, proteins, and other components organized in the manner of equilibrium, near equilibrium, far-from-equilibrium, or collaborative patterns form diverse biological networks[57,83]. These networks provide interactive platforms to program biological response patterns by affecting information transformation processes, such as feedback, feedforward, communication, adaptation, bistability, and oscillation mechanisms. The networks discussed here show the recent advances in using nucleic acids as building blocks to synthesize bioinspired networks that mimic natural processes. We summarized the chemical principles and the dynamic behaviors of fundamental dynamic networks, such as feedback, feedforward, communicating, and network adaption. Then we categorized artificial transient-signal conversion networks from the perspective of fuel types. Numerous dissipative far-from-equilibrium networks are proposed as signaling transducers that convert environmental inputs into temporal output signals. In addition, we discuss the recent advances in complex signaling dynamic behaviors by integrating different functional networks. Furthermore, nucleic acid-based dynamic networks linking with downstream functional systems are introduced to highlight the network-guided emerging properties and functions from nanostructure and pattern dynamics to information processes in protocells.
Despite the considerable progress achieved with nucleic acid-based dynamic networks, the complexity and functionality of natural networks have surpassed that of artificial networks. Therefore, the construction of nucleic acid-based dynamic networks with enhanced complexity and functionality remains challenging due to the limitations of existing approaches. Living systems operate under far-from-equilibrium conditions with complex spatiotemporal behaviors, and forming such far-from-equilibrium systems follows a spatiotemporal evolutionary pathway under environmental stress[82]. This approach allows the system to self-regulate the interactions and reactions of its components and adapt itself to be in tune with the ever-changing environment[157-160]. To address this challenge, an evolutionary approach is highly desired. Integrating numerous known far-from-equilibrium networks and adapting to environmental changes through the training-learning process holds great promise for triggering the evolutionary process of the artificial network. With this understanding, it is possible to imagine spatiotemporal networks with life-like complexity and capabilities.
The next challenge is how to use the nucleic acid-based dynamic network as the regulatory tool for downstream functional systems, such as nanomachines, nanomaterials, and protocells. Nucleic acid-based dynamic networks in a test tube rely on a dilute solution in which the network components are dispersed throughout the solution. These are not conducive to the functionalization of the network. We need to start developing nucleic acid-based dynamic networks that operate in semi-solvent or solvent-free bulk for materials sciences, such as hydrogels, and integrate networks into confined environments, such as DNA frameworks, DNA origami, synthetic condensates, for sophisticated nanomachines, switches, and motors. In addition, nucleic acid-based dynamic networks are more valuable tools for manipulating and controlling biological information than traditional approaches in a protocellular environment. Nucleic acid-based dynamic networks, in particular, have successfully transitioned from a test tube to a cell-like environment. But complex temporal control and various nonlinear regulatory networks in living cells are still missing in artificial systems. We anticipate that further integration of artificial complex spatiotemporal networks, systems chemistry, nanotechnology, and synthetic biology will be of great relevance to laying the foundation for the de novo construction of artificial cells with living features.
DECLARATIONS
Authors’ contributionsPrepared and revised the manuscript: Yue L, Zhong R, Yi L, Wang X, Shu W
Revised the manuscript: Yue L
Designed and revised the manuscript: Yue L
All authors contributed to the discussion and preparation of the manuscript.
Availability of data and materialsNot applicable.
Financial support and sponsorshipThis work was supported by the National Key Research and Development Project (2021YFA0909400) and the National Natural Science Foundation of China (NSFC 22101080).
Conflicts of interestAll authors declared that there are no conflicts of interest.
Ethical approval and consent to participateNot applicable.
Consent for publicationNot applicable.
Copyright© The Author(s) 2023.
REFERENCES
1. Wedlich-Söldner R, Betz T. Self-organization: the fundament of cell biology. Philos Trans R Soc Lond B Biol Sci 2018;373:20170103.
3. Kolisko M, Boscaro V, Burki F, Lynn DH, Keeling PJ. Single-cell transcriptomics for microbial eukaryotes. Curr Biol 2014;24:R1081-2.
4. Ma'ayan A, Jenkins SL, Neves S, et al. Formation of regulatory patterns during signal propagation in a Mammalian cellular network. Science 2005;309:1078-83.
5. White FM, Wolf-Yadlin A. Methods for the analysis of protein phosphorylation-mediated cellular signaling networks. Annu Rev Anal Chem 2016;9:295-315.
6. Vignes H, Vagena-Pantoula C, Vermot J. Mechanical control of tissue shape: Cell-extrinsic and -intrinsic mechanisms join forces to regulate morphogenesis. Semin Cell Dev Biol 2022;130:45-55.
8. Lehn JM. Perspectives in chemistry-steps towards complex matter. Angew Chem Int Ed Engl 2013;52:2836-50.
10. Gartner ZJ, Prescher JA, Lavis LD. Unraveling cell-to-cell signaling networks with chemical biology. Nat Chem Biol 2017;13:564-8.
13. Peyralans JJ, Otto S. Recent highlights in systems chemistry. Curr Opin Chem Biol 2009;13:705-13.
14. Corbett PT, Leclaire J, Vial L, et al. Dynamic combinatorial chemistry. Chem Rev 2006;106:3652-711.
15. Li J, Nowak P, Otto S. Dynamic combinatorial libraries: from exploring molecular recognition to systems chemistry. J Am Chem Soc 2013;135:9222-39.
18. Helwig B, van Sluijs B, Pogodaev AA, Postma SGJ, Huck WTS. Bottom-up construction of an adaptive enzymatic reaction network. Angew Chem Int Ed Engl 2018;57:14065-9.
19. Tyson JJ, Chen KC, Novak B. Sniffers, buzzers, toggles and blinkers: dynamics of regulatory and signaling pathways in the cell. Curr Opin Cell Biol 2003;15:221-31.
20. Moustakas A, Heldin CH. Signaling networks guiding epithelial-mesenchymal transitions during embryogenesis and cancer progression. Cancer Sci 2007;98:1512-20.
22. Almblad H, Randall TE, Liu F, et al. Bacterial cyclic diguanylate signaling networks sense temperature. Nat Commun 2021;12:1986.
23. Orij R, Brul S, Smits GJ. Intracellular pH is a tightly controlled signal in yeast. Biochim Biophys Acta 2011;1810:933-44.
24. Marchenko V, Sapru HN. Cardiovascular responses to chemical stimulation of the lateral tegmental field and adjacent medullary reticular formation in the rat. Brain Res 2003;977:247-60.
25. Angeli D, Ferrell JE Jr, Sontag ED. Detection of multistability, bifurcations, and hysteresis in a large class of biological positive-feedback systems. Proc Natl Acad Sci U S A 2004;101:1822-7.
26. Chong SJF, Lai JXH, Qu J, et al. A feedforward relationship between active Rac1 and phosphorylated Bcl-2 is critical for sustaining Bcl-2 phosphorylation and promoting cancer progression. Cancer Lett 2019;457:151-67.
27. Eppig JJ. Intercommunication between mammalian oocytes and companion somatic cells. Bioessays 1991;13:569-74.
28. Szego CM. Mechanisms of hormone action: parallels in receptor-mediated signal propagation for steroid and peptide effectors. Life Sci 1984;35:2383-96.
29. Krosigk M, Bal T, McCormick DA. Cellular mechanisms of a synchronized oscillation in the thalamus. Science 1993;261:361-4.
30. Pardridge WM, Landaw EM. Steady state model of 3,5,3'-triiodothyronine transport in liver predicts high cellular exchangeable hormone concentration relative to in vitro free hormone concentration. Endocrinology 1987;120:1059-68.
31. Samanta A, Hörner M, Liu W, Weber W, Walther A. Signal-processing and adaptive prototissue formation in metabolic DNA protocells. Nat Commun 2022;13:3968.
32. Deng J, Walther A. Autonomous DNA nanostructures instructed by hierarchically concatenated chemical reaction networks. Nat Commun 2021;12:5132.
33. Lehn JM. From supramolecular chemistry towards constitutional dynamic chemistry and adaptive chemistry. Chem Soc Rev 2007;36:151-60.
34. Herrmann A. Dynamic combinatorial/covalent chemistry: a tool to read, generate and modulate the bioactivity of compounds and compound mixtures. Chem Soc Rev 2014;43:1899-933.
35. Yue L, Wang S, Zhou Z, Willner I. Nucleic acid based constitutional dynamic networks: From basic principles to applications. J Am Chem Soc 2020;142:21577-94.
36. Wang S, Yue L, Willner I. Enzyme-guided selection and cascaded emergence of nanostructured constitutional dynamic networks. Nano Lett 2020;20:5451-7.
37. Zhou Z, Wang J, Willner I. Dictated emergence of nucleic acid-based constitutional dynamic networks by DNA replication machineries. J Am Chem Soc 2021;143:241-51.
38. Zhang Q, Bhattacharya S, Andersen ME. Ultrasensitive response motifs: basic amplifiers in molecular signalling networks. Open Biol 2013;3:130031.
39. He M, Lehn JM. Time-dependent switching of constitutional dynamic libraries and networks from kinetic to thermodynamic distributions. J Am Chem Soc 2019;141:18560-9.
40. Zhang DY, Seelig G. Dynamic DNA nanotechnology using strand-displacement reactions. Nat Chem 2011;3:103-13.
41. Zhang DY, Turberfield AJ, Yurke B, Winfree E. Engineering entropy-driven reactions and networks catalyzed by DNA. Science 2007;318:1121-5.
42. Hu Y, Cecconello A, Idili A, Ricci F, Willner I. Triplex DNA nanostructures: From basic properties to applications. Angew Chem Int Ed Engl 2017;56:15210-33.
43. Gehring K, Leroy JL, Guéron M. A tetrameric DNA structure with protonated cytosine.cytosine base pairs. Nature 1993;363:561-5.
44. Simmel FC, Yurke B, Singh HR. Principles and applications of nucleic acid strand displacement reactions. Chem Rev 2019;119:6326-69.
45. Walker MJ, Varani G. An allosteric switch primes sequence-specific DNA recognition. Cell 2019;176:4-6.
46. Yang H, Kim K, Li S, Pacheco J, Chen XS. Structural basis of sequence-specific RNA recognition by the antiviral factor APOBEC3G. Nat Commun 2022;13:7498.
47. Osborne SE, Matsumura I, Ellington AD. Aptamers as therapeutic and diagnostic reagents: problems and prospects. Curr Opin Chem Biol 1997;1:5-9.
49. McConnell EM, Cozma I, Mou Q, Brennan JD, Lu Y, Li Y. Biosensing with DNAzymes. Chem Soc Rev 2021;50:8954-94.
52. Barabási AL, Oltvai ZN. Network biology: understanding the cell’s functional organization. Nat Rev Genet 2004;5:101-13.
54. Jaenisch R, Bird A. Epigenetic regulation of gene expression: how the genome integrates intrinsic and environmental signals. Nat Genet 2003;33 Suppl:245-54.
55. Vogel C, Marcotte EM. Insights into the regulation of protein abundance from proteomic and transcriptomic analyses. Nat Rev Genet 2012;13:227-32.
56. Emmert-Streib F, Glazko GV. Network biology: a direct approach to study biological function. Wiley Interdiscip Rev Syst Biol Med 2011;3:379-91.
57. Purvis JE, Lahav G. Encoding and decoding cellular information through signaling dynamics. Cell 2013;152:945-56.
58. Wang F, Lu CH, Willner I. From cascaded catalytic nucleic acids to enzyme-DNA nanostructures: controlling reactivity, sensing, logic operations, and assembly of complex structures. Chem Rev 2014;114:2881-941.
59. Lee TI, Rinaldi NJ, Robert F, et al. Transcriptional regulatory networks in Saccharomyces cerevisiae. Science 2002;298:799-804.
60. Yue L, Wulf V, Wang S, Willner I. Evolution of nucleic-acid-based constitutional dynamic networks revealing adaptive and emergent functions. Angew Chem Int Ed Engl 2019;58:12238-45.
61. Yue L, Wang S, Willner I. Triggered reversible substitution of adaptive constitutional dynamic networks dictates programmed catalytic functions. Sci Adv 2019;5:eaav5564.
62. Zhou Z, Yue L, Wang S, Lehn JM, Willner I. DNA-based multiconstituent dynamic networks: hierarchical adaptive control over the composition and cooperative catalytic functions of the systems. J Am Chem Soc 2018;140:12077-89.
63. Wang S, Yue L, Shpilt Z, et al. Controlling the catalytic functions of DNAzymes within constitutional dynamic networks of DNA nanostructures. J Am Chem Soc 2017;139:9662-71.
64. Yue L, Wang S, Cecconello A, Lehn JM, Willner I. Orthogonal operation of constitutional dynamic networks consisting of DNA-tweezer machines. ACS Nano 2017;11:12027-36.
65. Wang S, Yue L, Li ZY, Zhang J, Tian H, Willner I. Light-induced reversible reconfiguration of DNA-based constitutional dynamic networks: Application to switchable catalysis. Angew Chem Int Ed Engl 2018;57:8105-9.
66. Yue L, Wang S, Willner I. Three-dimensional nucleic-acid-based constitutional dynamic networks: Enhancing diversity through complexity of the systems. J Am Chem Soc 2019;141:16461-70.
67. Jiang Y, Hao N. Memorizing environmental signals through feedback and feedforward loops. Curr Opin Cell Biol 2021;69:96-102.
68. Reeves GT. The engineering principles of combining a transcriptional incoherent feedforward loop with negative feedback. J Biol Eng 2019;13:62.
69. Patel A, Murray RM, Sen S. Assessment of robustness to temperature in a negative feedback loop and a feedforward loop. ACS Synth Biol 2020;9:1581-90.
70. Gao Y, Chen Y, Shang J, et al. Enzyme-free autocatalysis-driven feedback DNA circuits for amplified aptasensing of living cells. ACS Appl Mater Interfaces 2022;14:5080-9.
71. Shi K, Xie S, Tian R, et al. A CRISPR-Cas autocatalysis-driven feedback amplification network for supersensitive DNA diagnostics. Sci Adv 2021:7.
72. Novák B, Tyson JJ. Design principles of biochemical oscillators. Nat Rev Mol Cell Biol 2008;9:981-91.
73. Liu H, Yang Q, Peng R, et al. Artificial signal feedback network mimicking cellular adaptivity. J Am Chem Soc 2019;141:6458-61.
74. Zhu S, Zhang Q. Implementing feedforward neural network using DNA strand displacement reactions. NANO 2021;16:2150001.
75. Yue L, Wang S, Wulf V, et al. Consecutive feedback-driven constitutional dynamic networks. Proc Natl Acad Sci U S A 2019;116:2843-8.
76. Bleris L, Xie Z, Glass D, Adadey A, Sontag E, Benenson Y. Synthetic incoherent feedforward circuits show adaptation to the amount of their genetic template. Mol Syst Biol 2011;7:519.
77. Haley NEC, Ouldridge TE, Mullor Ruiz I, et al. Design of hidden thermodynamic driving for non-equilibrium systems via mismatch elimination during DNA strand displacement. Nat Commun 2020;11:2562.
78. Basu S, Mehreja R, Thiberge S, Chen MT, Weiss R. Spatiotemporal control of gene expression with pulse-generating networks. Proc Natl Acad Sci U S A 2004;101:6355-60.
79. Yue L, Wang S, Lilienthal S, et al. Intercommunication of DNA-based constitutional dynamic networks. J Am Chem Soc 2018;140:8721-31.
80. Wang C, Yue L, Willner I. Controlling biocatalytic cascades with enzyme-DNA dynamic networks. Nat Catal 2020;3:941-50.
81. Wang C, O'Hagan MP, Neumann E, Nechushtai R, Willner I. Integration of photocatalytic and dark-operating catalytic biomimetic transformations through DNA-based constitutional dynamic networks. Nat Commun 2021;12:4224.
83. Goldbeter A. Dissipative structures in biological systems: bistability, oscillations, spatial patterns and waves. Philos Trans A Math Phys Eng Sci 2018;376:20170376.
85. David-Pfeuty T, Erickson HP, Pantaloni D. Guanosinetriphosphatase activity of tubulin associated with microtubule assembly. Proc Natl Acad Sci U S A 1977;74:5372-6.
86. Murrell M, Oakes PW, Lenz M, Gardel ML. Forcing cells into shape: the mechanics of actomyosin contractility. Nat Rev Mol Cell Biol 2015;16:486-98.
87. Schaffter SW, Chen KL, O’Brien J, Noble M, Murugan A, Schulman R. Standardized excitable elements for scalable engineering of far-from-equilibrium chemical networks. Nat Chem 2022;14:1224-32.
88. Li Z, Wang J, Willner I. Transient out-of-equilibrium nucleic acid-based dissipative networks and their applications. Adv Funct Materials 2022;32:2200799.
90. De S, Klajn R. Dissipative self-assembly driven by the consumption of chemical fuels. Adv Mater 2018;30:e1706750.
91. Te Brinke E, Groen J, Herrmann A, et al. Dissipative adaptation in driven self-assembly leading to self-dividing fibrils. Nat Nanotechnol 2018;13:849-55.
92. Liu Q, Li H, Yu B, et al. DNA-based dissipative assembly toward nanoarchitectonics. Adv Funct Materials 2022;32:2201196.
93. Sorrenti A, Leira-Iglesias J, Sato A, Hermans TM. Non-equilibrium steady states in supramolecular polymerization. Nat Commun 2017;8:15899.
94. Busiello DM, Liang S, Piazza F, De Los Rios P. Dissipation-driven selection of states in non-equilibrium chemical networks. Commun Chem 2021;4:16.
95. Wang S, Yue L, Wulf V, Lilienthal S, Willner I. Dissipative constitutional dynamic networks for tunable transient responses and catalytic functions. J Am Chem Soc 2020;142:17480-8.
96. Zhou Z, Ouyang Y, Wang J, Willner I. Dissipative gated and cascaded DNA networks. J Am Chem Soc 2021;143:5071-9.
97. Wang C, Zhou Z, Ouyang Y, et al. Gated dissipative dynamic artificial photosynthetic model systems. J Am Chem Soc 2021;143:12120-8.
98. Dehne H, Reitenbach A, Bausch AR. Transient self-organisation of DNA coated colloids directed by enzymatic reactions. Sci Rep 2019;9:7350.
99. Li N, Zhao Y, Liu Y, et al. Self-resetting molecular probes for nucleic acids detection enabled by fuel dissipative systems. Nano Today 2021;41:101308.
100. Ouyang Y, Zhang P, Willner I. Dissipative biocatalytic cascades and gated transient biocatalytic cascades driven by nucleic acid networks. Sci Adv 2022;8:eabn3534.
101. Dong J, Ouyang Y, Wang J, O'Hagan MP, Willner I. Assembly of dynamic gated and cascaded transient DNAzyme networks. ACS Nano 2022;16:6153-64.
102. Luo M, Xuan M, Huo S, et al. Four-dimensional deoxyribonucleic acid-gold nanoparticle assemblies. Angew Chem Int Ed Engl 2020;59:17250-5.
103. Ouyang Y, Zhang P, Manis-Levy H, Paltiel Y, Willner I. Transient dissipative optical properties of aggregated Au nanoparticles, CdSe/ZnS quantum dots, and supramolecular nucleic acid-stabilized Ag nanoclusters. J Am Chem Soc 2021;143:17622-32.
104. Li Z, Wang J, Zhou Z, O'Hagan MP, Willner I. Gated transient dissipative dimerization of DNA tetrahedra nanostructures for programmed DNAzymes catalysis. ACS Nano 2022;16:3625-36.
105. Wang J, Li Z, Willner I. Cascaded dissipative DNAzyme-driven layered networks guide transient replication of coded-strands as gene models. Nat Commun 2022;13:4414.
106. Grosso E, Amodio A, Ragazzon G, Prins LJ, Ricci F. Dissipative synthetic DNA-based receptors for the transient loading and release of molecular cargo. Angew Chem Int Ed Engl 2018;57:10489-93.
107. Deng J, Liu W, Sun M, Walther A. Dissipative organization of DNA oligomers for transient catalytic function. Angew Chem Int Ed Engl 2022;61:e202113477.
108. Deng J, Walther A. Fuel-driven transient DNA strand displacement circuitry with self-resetting function. J Am Chem Soc 2020;142:21102-9.
109. Deng J, Walther A. ATP-powered molecular recognition to engineer transient multivalency and self-sorting 4D hierarchical systems. Nat Commun 2020;11:3658.
110. Reisler E, Egelman EH. Actin structure and function: what we still do not understand. J Biol Chem 2007;282:36133-7.
111. Grosso E, Ponzo I, Ragazzon G, Prins LJ, Ricci F. Disulfide-linked allosteric modulators for multi-cycle kinetic control of DNA-based nanodevices. Angew Chem Int Ed Engl 2020;59:21058-63.
112. Grosso E, Prins LJ, Ricci F. Transient DNA-based nanostructures controlled by redox inputs. Angew Chem Int Ed Engl 2020;59:13238-45.
113. Mariottini D, Del Giudice D, Ercolani G, Di Stefano S, Ricci F. Dissipative operation of pH-responsive DNA-based nanodevices. Chem Sci 2021;12:11735-9.
114. Chen XM, Hou XF, Bisoyi HK, et al. Light-fueled transient supramolecular assemblies in water as fluorescence modulators. Nat Commun 2021;12:4993.
115. Deng J, Bezold D, Jessen HJ, Walther A. Multiple light control mechanisms in ATP-fueled non-equilibrium DNA systems. Angew Chem Int Ed Engl 2020;59:12084-92.
116. Wang J, Li Z, Zhou Z, et al. DNAzyme- and light-induced dissipative and gated DNA networks. Chem Sci 2021;12:11204-12.
117. Chen Y, Wang M, Mao C. An autonomous DNA nanomotor powered by a DNA enzyme. Angew Chem Int Ed Engl 2004;43:3554-7.
118. Grosso E, Ragazzon G, Prins LJ, Ricci F. Fuel-responsive allosteric DNA-based aptamers for the transient release of ATP and cocaine. Angew Chem Int Ed Engl 2019;58:5582-6.
119. Grosso E, Irmisch P, Gentile S, Prins LJ, Seidel R, Ricci F. Dissipative Control over the toehold-mediated DNA strand displacement reaction. Angew Chem Int Ed Engl 2022;61:e202201929.
120. Agarwal S, Franco E. Enzyme-driven assembly and disassembly of hybrid DNA-RNA nanotubes. J Am Chem Soc 2019;141:7831-41.
121. Gentile S, Del Grosso E, Pungchai PE, Franco E, Prins LJ, Ricci F. Spontaneous reorganization of DNA-based polymers in higher ordered structures fueled by RNA. J Am Chem Soc 2021;143:20296-301.
122. Bucci J, Irmisch P, Del Grosso E, Seidel R, Ricci F. Orthogonal enzyme-driven timers for DNA strand displacement reactions. J Am Chem Soc 2022;144:19791-8.
123. Abraham EH, Okunieff P, Scala S, et al. Cystic fibrosis transmembrane conductance regulator and adenosine triphosphate. Science 1997;275:1324-6.
124. Finger TE, Danilova V, Barrows J, et al. ATP signaling is crucial for communication from taste buds to gustatory nerves. Science 2005;310:1495-9.
125. Shi Y, Tang M, Sun C, et al. ATP mimics pH-dependent dual peroxidase-catalase activities driving H2O2 decomposition. CCS Chem 2019;1:373-83.
126. Heinen L, Walther A. Programmable dynamic steady states in ATP-driven nonequilibrium DNA systems. Sci Adv 2019;5:eaaw0590.
127. Sun M, Deng J, Walther A. Communication and cross-regulation between chemically fueled sender and receiver reaction networks. Angew Chem Int Ed Engl 2023;62:e202214499.
129. Weitz M, Kim J, Kapsner K, Winfree E, Franco E, Simmel FC. Diversity in the dynamical behaviour of a compartmentalized programmable biochemical oscillator. Nat Chem 2014;6:295-302.
130. Kim J, White KS, Winfree E. Construction of an in vitro bistable circuit from synthetic transcriptional switches. Mol Syst Biol 2006;2:68.
131. Schaffter SW, Schulman R. Building in vitro transcriptional regulatory networks by successively integrating multiple functional circuit modules. Nat Chem 2019;11:829-38.
132. Subsoontorn P, Kim J, Winfree E. Ensemble Bayesian analysis of bistability in a synthetic transcriptional switch. ACS Synth Biol 2012;1:299-316.
133. Franco E, Friedrichs E, Kim J, et al. Timing molecular motion and production with a synthetic transcriptional clock. Proc Natl Acad Sci U S A 2011;108:E784-93.
135. Baccouche A, Montagne K, Padirac A, Fujii T, Rondelez Y. Dynamic DNA-toolbox reaction circuits: a walkthrough. Methods 2014;67:234-49.
136. Montagne K, Plasson R, Sakai Y, Fujii T, Rondelez Y. Programming an in vitro DNA oscillator using a molecular networking strategy. Mol Syst Biol 2011;7:466.
137. Padirac A, Fujii T, Rondelez Y. Bottom-up construction of in vitro switchable memories. Proc Natl Acad Sci U S A 2012;109:E3212-20.
138. Zadorin AS, Rondelez Y, Gines G, et al. Synthesis and materialization of a reaction-diffusion French flag pattern. Nat Chem 2017;9:990-6.
139. Dehne H, Reitenbach A, Bausch AR. Reversible and spatiotemporal control of colloidal structure formation. Nat Commun 2021;12:6811.
140. Padirac A, Fujii T, Rondelez Y. Nucleic acids for the rational design of reaction circuits. Curr Opin Biotechnol 2013;24:575-80.
141. Xiong X, Zhu T, Zhu Y, et al. Molecular convolutional neural networks with DNA regulatory circuits. Nat Mach Intell 2022;4:625-35.
142. Qian L, Winfree E, Bruck J. Neural network computation with DNA strand displacement cascades. Nature 2011;475:368-72.
143. Okumura S, Gines G, Lobato-Dauzier N, et al. Nonlinear decision-making with enzymatic neural networks. Nature 2022;610:496-501.
144. Nimiritsky P, Novoseletskaya E, Eremichev R, et al. Self-organization provides cell fate commitment in MSC sheet condensed areas via ROCK-dependent mechanism. Biomedicines 2021;9:1192.
145. Davidson EH, Rast JP, Oliveri P, et al. A genomic regulatory network for development. Science 2002;295:1669-78.
146. Parsons JT, Horwitz AR, Schwartz MA. Cell adhesion: integrating cytoskeletal dynamics and cellular tension. Nat Rev Mol Cell Biol 2010;11:633-43.
147. Omidvar M, Zdarta J, Sigurdardóttir SB, Pinelo M. Mimicking natural strategies to create multi-environment enzymatic reactors: From natural cell compartments to artificial polyelectrolyte reactors. Biotechnol Adv 2022;54:107798.
148. Monteith GR, Prevarskaya N, Roberts-Thomson SJ. The calcium-cancer signalling nexus. Nat Rev Cancer 2017;17:367-80.
149. Buchakjian MR, Kornbluth S. The engine driving the ship: metabolic steering of cell proliferation and death. Nat Rev Mol Cell Biol 2010;11:715-27.
150. Peng R, Xu L, Wang H, et al. DNA-based artificial molecular signaling system that mimics basic elements of reception and response. Nat Commun 2020;11:978.
151. Wang D, Yang Y, Chen F, Lyu Y, Tan W. Network topology-directed design of molecular CPU for cell-like dynamic information processing. Sci Adv 2022;8:eabq0917.
152. Buddingh’ BC, Elzinga J, van Hest JCM. Intercellular communication between artificial cells by allosteric amplification of a molecular signal. Nat Commun 2020;11:1652.
153. Mason AF, Buddingh’ BC, Williams DS, van Hest JCM. Hierarchical self-assembly of a copolymer-stabilized coacervate protocell. J Am Chem Soc 2017;139:17309-12.
154. Elani Y, Law RV, Ces O. Vesicle-based artificial cells as chemical microreactors with spatially segregated reaction pathways. Nat Commun 2014;5:5305.
155. Joesaar A, Yang S, Bögels B, et al. DNA-based communication in populations of synthetic protocells. Nat Nanotechnol 2019;14:369-78.
156. Yang S, Joesaar A, Bögels BWA, Mann S, de Greef TFA. Protocellular CRISPR/Cas-based diffusive communication using transcriptional RNA signaling. Angew Chem Int Ed Engl 2022;61:e202202436.
157. Pawson T. Specificity in signal transduction: from phosphotyrosine-SH2 domain interactions to complex cellular systems. Cell 2004;116:191-203.
158. Pawson T, Nash P. Assembly of cell regulatory systems through protein interaction domains. Science 2003;300:445-52.
159. You L, Cox RS 3rd, Weiss R, Arnold FH. Programmed population control by cell-cell communication and regulated killing. Nature 2004;428:868-71.
Cite This Article
Export citation file: BibTeX | RIS
OAE Style
Zhong R, Yi L, Wang X, Shu W, Yue L. Bioinspired nucleic acid-based dynamic networks for signal dynamics. Chem Synth 2023;3:27. http://dx.doi.org/10.20517/cs.2023.15
AMA Style
Zhong R, Yi L, Wang X, Shu W, Yue L. Bioinspired nucleic acid-based dynamic networks for signal dynamics. Chemical Synthesis. 2023; 3(3): 27. http://dx.doi.org/10.20517/cs.2023.15
Chicago/Turabian Style
Zhong, Rui, Lin Yi, Xiarui Wang, Weijun Shu, Liang Yue. 2023. "Bioinspired nucleic acid-based dynamic networks for signal dynamics" Chemical Synthesis. 3, no.3: 27. http://dx.doi.org/10.20517/cs.2023.15
ACS Style
Zhong, R.; Yi L.; Wang X.; Shu W.; Yue L. Bioinspired nucleic acid-based dynamic networks for signal dynamics. Chem. Synth. 2023, 3, 27. http://dx.doi.org/10.20517/cs.2023.15
About This Article
Copyright
Author Biographies
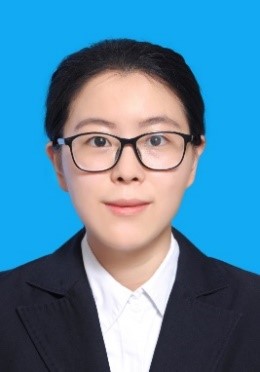
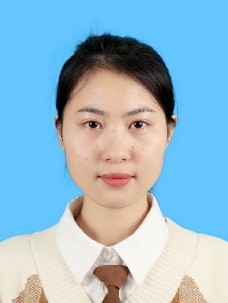
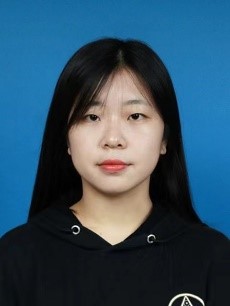
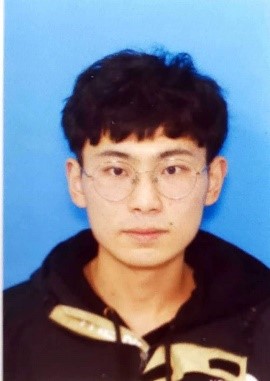
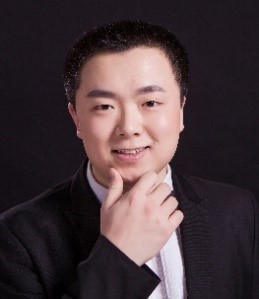
Data & Comments
Data

Comments
Comments must be written in English. Spam, offensive content, impersonation, and private information will not be permitted. If any comment is reported and identified as inappropriate content by OAE staff, the comment will be removed without notice. If you have any queries or need any help, please contact us at support@oaepublish.com.