Application of radiomics and artificial intelligence in lung cancer immunotherapy: a guide and hurdles from clinical trials
Abstract
Immunotherapy has shown promising results with improved progression-free survival and overall survival in lung cancer. However, novel immunotherapy could generate atypical response patterns, which is a big challenge for traditional imaging criteria. Radiomics, combined with artificial intelligence (AI), represents new quantitative methodologies that could serve as an additional imaging biomarker to predict immunotherapy benefits and assess responses to assist oncologists in decision-making in lung cancer treatment. This paper aims to review the latest advancement of AI-based radiomics applied to lung cancer patients receiving immunotherapy, focusing on the fundamentals of these approaches and commonly used techniques. We also address the hurdles in the AI and radiomic analysis pipeline to guide clinicians in approaching this new concept.
Keywords
INTRODUCTION
Lung cancer is the second most commonly diagnosed cancer and the leading cause of cancer-related death worldwide[1]. Novel treatments such as immunotherapy have greatly changed the landscape of treatment and clinical outcomes for patients with lung cancer[2-4]. However, only a proportion of patients benefit from immunotherapy[5]. Treatment response to immunotherapy is often unpredictable, with significant variability among patients, in the majority of cases unexplainable. Consequently, there is an unmet need for reliable biomarkers to identify the patients likely to respond to immunotherapy, preventing unnecessary exposure to potentially toxic therapies and avoiding wasting financial resources.
PD-L1 expression level is an early biomarker to predict the response of lung cancer to immunotherapy, with a higher level of PD-L1 (> 50% of tumour cells) correlating to a higher response rate[6]. However, there are several disadvantages and challenges in interpreting the PD-L1 expression level. First, the PD-L1 expression level from a biopsy sample can only represent a small static snapshot of the tumour rather than the entire lung cancer burden, including metastatic lesions. It fails to take into account the wide intratumour heterogeneity and rapid evolution of primary or metastatic lung cancer over time[7]. In addition, studies show that the immunotherapy treatment outcomes are heavily impacted by histopathology, tumour microenvironment (TME), immune contexture, and tumour molecular profiles[8]. Furthermore, PD-L1 expression level requires an invasive procedure (surgery or biopsy), which may not be feasible in certain cases due to the high risk of procedure complications relating to tumour location or patients’ co-mobilities. Although other biomarkers, such as Microsatellite Instability (MSI), MisMatch-Repair (MMR) status, Tumour Mutation Burden (TMB), and Tumour Infiltrating Lymphocytes (TIL) have been shown to have predictive value in response to immunotherapy in variable tumour types, most of these biomarkers require invasive procedures and have not been applied to lung cancer management[9]. Liquid biopsy through circulating tumour cells (CTC) and circulating tumour DNA (ctDNA) analyses is becoming a promising tool for predicting PD-L1 status and response to immunotherapy in lung cancer; however, these results are very preliminary[10-12]. Consequently, a more reliable and non-invasive tool that can predict immunotherapy efficacy in lung cancer is needed to assist clinicians to personalize treatment decisions.
In current clinical practice, radiology images including CT, MRI, and PET/CT are standard strategies to monitor treatment responses based on quantitative assessment and dimensional measurements with the benefits of accuracy and reproducibility while minimizing invasiveness. However, immunotherapy could induce atypical response patterns, including pseudoprogression, hyper-progression, and dissociated response, which differ from the response patterns typically observed with cytotoxic chemotherapy and targeted therapies[13-15]. Pseudoprogression is a phenomenon that mimics disease progression, characterised by an initial enlargement of the tumour or the appearance of new lesions, but may not be representative of progression as it is followed by a decrease in tumour burden. It is a challenge to differentiate between pseudoprogression and hyper-progression, as pseudoprogression can be caused by infiltration of active T cells and other immune cells within the lesion, as well as local inflammation[16], while hyper progression is the accelerated tumour progression after immunotherapy, which is caused by upregulation of other immune checkpoints and local inflammation assisting tumour escape and progression[13]. Therefore, more accurate image response criteria, both anatomical and metabolic, are needed to assess immunotherapy benefits when clinicians are confronted with atypical response patterns. Although the RECIST working group developed updated criteria iRECIST[17] to evaluate the tumour response to immunotherapy, prospective studies are still required to fully establish its superiority[18]. As such, non-invasive image biomarkers are required to accurately assess the response of lung cancer to immunotherapy.
Radiomics, in particular, texture analysis, is an innovative tool in radiology with the capability to extract measurable information from images that aim to predict tumour biology, such as EGFR status in lung cancer[19], treatment outcomes and accurately assess treatment response. Consequently, treatment strategy can be personalized by taking into account the specific characteristics of each tumour. Artificial intelligence (AI) is a set of systems that can accurately perform inferences from a large amount of data based on advanced computational algorithms, namely machine learning (ML) and lately prevalent deep learning algorithms. Through AI or machine learning, radiomics features can be integrated with clinicopathological features or other “-mics” information, such as genomics, to further improve the clinical decision process. Here, we review existing studies that explore the application of radiomics and artificial intelligence in immunotherapy response prediction and assessment, particularly focusing on non-small cell lung cancer (NSCLC). We aim to investigate the hurdles at each critical step of radiomic analysis in lung cancer immunotherapy. By comprehensively examining these challenges, we hope to fill the gap in the existing literature and provide valuable insights into how clinicians can effectively address these hurdles and avoid potential errors and mistakes when approaching these new concepts.
DEFINITION OF RADIOMICS AND ARTIFICIAL INTELLIGENCE
Radiomics uses computational methods to quantitate measurable data of images, which is hidden within conventional images and typically imperceptible to the human eyes, to provide a deeper understanding of cancer. Radiomics has been widely investigated in several oncology fields to explore the image biomarkers associated with cancer biology, gene expression, spatial heterogeneity, and TME, such as immune infiltration and neovascularisation[20,21].
Radiomics presents several advantages for oncology. First, feature extraction from medical images is a non-invasive process, which makes it convenient for clinical applications with high patient compliance. Furthermore, radiomics capture the information on the entire three-dimensional tumour and all lesion distributions, which provides a better evaluation of the tumour, including its microenvironment, compared to the conventional image assessment. Finally, radiomics data have the potential for longitudinally tracking changes in tumours over time, enabling identification of treatment-resistant tumours[21].
AI is an umbrella term technology that includes machine learning and deep learning to mimic human intelligence. Deep learning is a subset of machine learning, while machine learning is a subset of AI. Radiomic analysis is based on the application of specific and sophisticated machine learning or deep learning algorithms to analyse the pattern of radiomic features and then make predictions on unseen data. These algorithms have the capability of interpreting complex and multidimensional data compared with traditional statistical methods. AI algorithms are not only able to analyse the numeric radiomic features but also to directly analyse the images to automatically design their radiomic features[22].
The whole process of radiomic analysis can be divided into the following steps [Figure 1]: image acquisition and Volume of Interest (VOI) segmentation, feature extraction and selection, as well as model development and validation[20,23]. However, in each step of developing a reliable radiomic model, there are crucial factors that could impact the ultimate outcomes, which explains why radiomics is still experiencing challenges in application into clinical practice. Here, we listed selected full-text English radiomic literature related to the application of AI-based radiomics in immunotherapy response prediction and assessment in lung cancer
Figure 1. Overview of the radiomics pipeline. The pipeline consisted of image processing, feature processing, radiomics modeling, and validation.
Summary of selected immunotherapy-related radiomics studies-general features
Reference | Image | Size | Study design | Treatment | End-point | Radiomics & texture features | Timing |
Tunali et al. 2021[44] | CT PET-CT | 332 | Retrospective | Anti-PD-(L)1+/- Anti-CTLA4 | OS/PFS | GLCM inverse difference | Pre-ICI < 90 days |
Tunali et al. 2019[45] | CT | 228 | Prospective | Anti-PD-(L)1+/- Anti-CTLA4 | Hyperprogression PFS | Border quartile coefficient of dispersion Radial gradient border SD-2D Border 3D Laws E5E5L5 and NGTDM strength | Pre-ICI < 30 days |
Ravanelli et al. 2019 [66] | CT | 104 | Retrospective | Nivolumab | OS/PFS | Kurtosis SSF = 4 mm | Pre-ICI < 2 months |
Trebeschi et al. 2019[67] | CT | 123 | Retrospective | Anti-PD-1 | Response 1 year OS | surfaceVolumeRatio | Pre-ICI |
Jiang et al. 2020[75] | CT PET/CT | 399 | Retrospective | Anti-PD-(L)1 | PD-L1 expression | CT/PETCT wavelet, first-, second features | Pre-surgery |
Sun et al. 2018[46] | CT | 135 | Retrospective | Anti-PD-(L)1 | CD8 expression OS and PFS | GLRLM | Pre-ICI |
Khorrami et al. 2020[34] | CT | 139 | Retrospective | Anti-PD-(L)1 | Response OS | DelRADx | Pre- and Post-ICI |
Dercle et al. 2020[68] | CT | 92 | Retrospective | Nivolumab | BOR | Tumor volume tumor spatial heterogeneity, boundaries irregularity | Pre- and Post ICI |
Castello et al. 2020[30] | CT PETCT | 50 | Prospective | Nivolumab | Hyperprogression | MTB, expressed by MTV and TLG | Pre- and Post ICI |
Polverari et al. 2020[31] | PET/CT | 57 | Retrospective | Anti-PD-(L)1 | OS/PFS Response | SUVmax, MTV and TLG | Pre-ICI |
Liu et al. 2021[76] | CT | 46 | Retrospective | Nivolumab | PFS/OS | Two LoG image and one wavelet feature | Pre-ICI |
Shen et al. 2021[77] | CT | 63 | Retrospective | Anti-PD-(L)1 | Response | 10 features including kurtosis | Pre-ICI |
Liu et al. 2021[42] | CT | 197 | Retrospective | Nivolumab | Response | Delta-radiomics feature with Rad-score | Pre- and Post ICI |
He et al. 2020[49] | CT | 123 | Retrospective | Anti-PD-(L)1 | TMB/response | TMBRB (TMB radiomic biomarker) | Pre-ICI |
Vaidya et al. 2020[47] | CT | 109 | Retrospective | Anti-PD-(L)1 | Hyperprogression | One peritumoral texture feature and two nodule vessel-related tortuosity features | Pre-ICI |
Ladwa et al. 2020[64] | CT | 47 | Retrospective | Nivolumab | TTP, OS, PFS | Skewness, SD, entropy, MPP, mean and kurtosis | Pre-ICI |
Yang et al. 2021[73] | CT | 92 | Retrospective | Anti-PD-(L)1 | DCB, PFS | Rad-score | Pre-ICI |
Tang et al. 2018[78] | CT | 290 | Retrospective | Anti-PD-L1 | OS | Immune pathology features, CD3+ cell density and PD-L1 expression | Pre-ICI |
Yoon et al. 2020[72] | CT | 149 | Retrospective | Anti-PD-L1 | PD-L1 expression | Rad-score | Pre-ICI |
Nardone et al. 2019[69] | CT | 59 | Retrospective | Nivolumab | PFS/OS | GLCM, sphericity and indices from the grey-level histogram | Pre-ICI |
Wu et al. 2020[32] | PETCT | 374 | Retrospective | NR | PD-L1 expression | SUVmax, MTV, TLG | Pre-ICI |
Seban et al. 2019[27] | PETCT | 80 | Retrospective | Anti-PD-(L)1 | PFS/OS | SUVmax and MTV | Pre-ICI |
Mu et al. 2019[65] | PETCT/CT | 194 | Retrospective/prospective | Anti-PD-(L)1 | DCB/PFS/OS | SUVmax | |
Korpics et al. 2020[48] | CT | 68 | Retrospective | Pembrolizumab | PFS/OS | T cell radiomics score post SBRT and IO | Pre-ICI |
Valentinuzzi et al. 2020[24] | PET/CT | 30 | Retrospective | Pembrolizumab | iRADIOMICS | GLCM and GLRLM | Pre- and post-ICI |
Tian et al. 2021[71] | CT | 939 | Retrospective | Pembrolizumab | PD-L1 expression | Wavelet-HL_GLCM_ sum entropy and Wavelet-LL-GLRLM_gray level | Pre-ICI and post ICI |
Summary of selected immunotherapy related radiomics studies-radiomics features
Reference | Software | Segmentation | Non-radiomics features | Images features | Validation | Model |
Tunali et al. 2021[44] | Matlab | Semi-automatic | Albumin metastatic burden | Intra- and peri tumour | External validation | Feature selection: univariable Cox regression Model: CART, multivariable Cox regression |
Tunali et al. 2019[45] | Matlab and c++ | Semi-automatic | Hepatic and/or bone metastases prior therapies, NLR | Intra- and peri tumour | NR | Model: LR |
Ravanelli et al. 2019[66] | TexRAD | Manual | NR | Intra-tumour | Cross-validation | Feature selection: LASSO Model: Cox proportional hazards |
Trebeschi et al. 2019[67] | NR | Manual | Independent gene expression data | NR | Split | Feature selection: wrapper model Model: RF |
Jiang et al. 2020[75] | ITK-SNAP Python | Semi-automatic | NR | NR | Cross-validation | Feature selection: ARD and LASSO Model: LR and RF |
Sun et al. 2018[46] | LIFEx | Semi-automatic | Tumor volume, Prior therapy, Royal marsden prognostic score | Intra- and peri tumour | External | LEN |
Khorrami et al. 2020[34] | 3D-slicer Matlab | Manual | Gender and smoking TIL | Intra and peri-tumor | Split and external | Feature selection: ICC and Wilcoxon rank sum Model: LDA |
Dercle et al. 2020[68] | Matlab | Semi-automatic | NR | NR | Split | Model: RF |
Castello et al. 2020[30] | NR | NR | dNLR Platelet count | NR | NR | Cox proportional hazards regression |
Polverari et al. 2020[31] | LIFEx | Manual | NR | NR | NR | Feature selection: univariate analysis ICC analysis |
Liu et al. 2021[76] | Python | Manual | NR | NR | Cross-validation | Feature selection: L1-based feature selection Model: SVM, LR, GNB |
Shen et al. 2021[77] | Mazda | Manual | NR | NR | NR | Feature selection: Fisher coefficient, MI and POE+ACC Model: LDA, NDA, PCA |
Liu et al. 2021[42] | ITK-SNAP | Manual | PD-L1 expression and distant metastasis | NR | Split | Feature selection: mRMR and LASSO Model: LR |
He et al. 2020[49] | 3D slicer Python | Manual | NR | NR | Split | Deep learning neurol network |
Vaidya et al. 2020[47] | 3D slicer Matlab | Manual | NR | Intra and peri tumour | Split | Feature selection: unsupervised clustering Model: RF, LDA, DLDA, QDA, SVM |
Ladwa et al. 2020[64] | Matlab | NR | NR | NR | Cross validation | General model for combining pairs of texture parameters |
Yang et al. 2021[73] | Python | Semi-automatic | Age and metastases | NR | Cross validation | Clinicopathological model: Rad-score Model: multivariate LR and multivariate Cox proportional hazards regression |
Tang et al. 2018[78] | 3D slicer IBEX | NR | Lesion size, n-status, histology, age at surgery, prior therapy | NR | Split | Feature selection: previously identified features Model: Cox proportional hazards |
Yoon et al. 2020[72] | AVIEW | Semi-automatic | Age, female, smoker status, EGFR+ | NR | Internal bootstrapping | Feature selection: ICC, LASSO Model: multivariate LR |
Nardone et al. 2019[69] | LifeX, X-Tile | NR | Sex, age, histology | NR | Split External | Texture score |
Wu et al. 2020[32] | NR | Age, gender, smoking history, serum tumor markers, tumor location, size, TNM and genetic mutation | NR | NR | Model: LR | |
Seban et al. 2019[27] | NR | NR | dNLR, pathology, LDH, Albumin | NR | NR | LR |
Mu et al. 2019[65] | NR | Semi-automatic | ECOG PS and histology | NR | Split | Feature selection: LASSO Model: multivariable Cox regression |
Korpics et al. 2020[48] | LIFEx | NR | CD 8+ cell Gene mRAN | Intra and peri tumor | NR | Model: LR or Cox proportional hazards model. |
Valentinuzzi et al. 2020[24] | 3D slicer | Semi-automatic | NR | NR | Cross-validation | Model: LR |
Tian et al. 2021[71] | NR | Manual | Age, gender, smoking, and family history | NR | Split | KNN for missing clinical values. Deep convolutional neural network |
CRITICAL POINTS DURING RADIOMIC ANALYSIS
Image acquisition, database creation, and data selection
Nearly all clinical images, including MRI, CT, PET/CT, and ultrasound, can be analysed by radiomics tools. In lung cancer, PET/CT and CT are the most commonly used imaging modalities for radiomic investigation[24,25]. Overall, associations of PET features were consistent across studies both within specific cancer types and across different types of cancers[26,27]; they use F-fluorodeoxyglucose (FDG) uptake features such as Maximum Standard Unit Value (SUVmax), which is positively correlated with expression of PD-L1 and durable clinical response[26]. Moreover, there is an increasing interest in using new PET features including Tumour Metabolic Volume (TMV), Total Lesion Glycolysis (TLG), and metabolic activity of the gut microbiome as potential nuclear-medicine immunotherapy biomarkers[26,28-32]. However, lung cancer patients commonly have CT for staging and response assessment during the course of immunotherapy, considering the high radiation exposure of PET/CT. In that case, CT images are more accessible for longitudinal radiomic analysis of tumour, especially for resistance investigation, thereby providing complementary information and improving response assessment performances. In addition, the optimal timing for imaging in radiomic analysis continues to be a subject of debate. Valentinuzzi et al. discovered that certain features demonstrated a high predictive value at baseline but showed a significant decrease at months 1 and 4 following treatment, which suggests that intrinsic tumour characteristics likely have an impact on response[24]. On the other hand, some studies suggested that longitudinal changes in radiomic features could be linked with treatment response and may serve as prognostic biomarkers[33,34].
Lack of standardization in imaging acquisition is another limitation during radiomic analysis. Although the wide variations in imaging acquisition and reconstruction protocols across manufacturers and hospitals rarely generate an issue in the regular extraction of radiomic features, the variations could introduce biases during the numerical AI-based radiomic analysis since the reconstructed parameters may generate alternations unrelated to underlying biologic effects[35]. To overcome this issue, either standardized acquisition parameters or normalizing voxel intensities of images are applied to extract more accurate and reproducible radiomic features in different studies[36].
In addition, the size of radiomic studies is critical to create correct radiomic models. In a radiomic study, a minimum of 100 patients ideally should be included, while larger cohorts could offer improved statistical power. Selected studies in this review have samples ranging from 30 to 939, with more than half of the studies recruited over 100 patients. Small-size studies could potentially increase the risk that reported correlations are either false positives or applicable only to the specific group of patients, which indicates a lack of generalizability[21]. The challenges associated with sufficient data acquisition may be alleviated over a period of time through enhanced access to shared resources such as the Imaging Data Commons (IDC), which is a cloud-based publicly available cancer imaging database from The Cancer Imaging Archive (TCIA) and other major imaging repositories. Another approach of data augmentation is to artificially inflate the training data set size by rotating or inverting the images, or a more recently developed machine learning algorithmic architecture, generative adversarial networks (GANs) that create new data by generating images with a different modality[37]. Multi-centre collaborations can also facilitate a diverse and representative dataset to minimize the potential biases and confounding factors that can impact the accuracy of predictions and conclusions.
Identification and segmentation of volume of interest
Identification and segmentation of the volume of interest (VOI) is one of the most critical and challenging steps in radiomic analysis because the subsequent feature data are generated from the segmented volumes. The segmentation includes the acquisition of the lesions by performing manual image delineation or utilising an automated AI technique known as auto-segmentation to define the VOI. While manual segmentations are frequently conducted by experienced radiologists and are considered the gold standards for radiomic analysis, there is unavoidable inter- and intra-observer variability. A large source of radiomic feature bias is caused by manual tumour contouring, which raises the issue of reproducibility. Moreover, contouring tumours can be particularly challenging due to their indistinct borders, which is time-consuming for the clinician and may impact reproducibility[38]. Consequently, the presence of bias should be taken into consideration when interpreting radiomic features. In recent years, there has been an increasing trend in utilizing AI approaches to perform segmentation tasks without any need for human intervention that can relieve bias and reproducibility issues, thus can handle less well-defined VOI or entire image datasets, especially in non-homogeneous lesions[39]. This very popular and advanced subset of AI is called deep learning, especially convolutional neural networks (CNN), which has a unique architecture and uses convolution operations to analyse pixel-level data from images[40]. A consensus is emerging that optimum reproducible segmentation could be achieved by computer-aided edge detection followed by manual curation.
Feature identification in radiomics
The heart of radiomics is the extraction of imaging features, which involves specialised software to perform high-throughput extraction of a large number of quantitative imaging data of VOI. Considering the variability of features extracted with different software, the image biomarker standardisation initiative (IBSI) has proposed guidelines to standardize feature extraction protocols with the aim of unifying feature extraction[41]. These features can be broadly classified into three groups: shape, first-order (image intensity), and second-order (texture) features. Shape-based features are the collection of quantitative data regarding the geometric features of the VOI, including 2D and 3D levels. These shape-based features are independent of the voxel intensity distribution in the VOI. First-order features are generally histogram-based and describe the distribution of voxel intensities within the VOI. Second-order features analyse the relationships among voxels with similar intensity values and provide insights into the heterogeneity within the VOI. These features are expressed in terms of matrices, indicating that statistics are derived from voxel relationships rather than the values themselves. Examples of second-order features are represented by Gray Level Co-occurrence Matrix (GLCM), Gray Level Size Zone Matrix (GLSZM), Gray Level Run Length Matrix (GLRLM), Neighbouring Gray Tone Difference Matrix (NGTDM), and Gray Level Dependence Matrix (GLDM) features [Table 3][41]. Many feature extraction software did not adhere to IBSI criteria, such as earlier studies with in-house code generated in Matlab. Some studies did not give any information regarding the feature extraction tools. The lack of transparency and interpretability of the extracted radiomic features limits their clinical use. In order to enhance the design of studies and foster the transparency of data reporting, there is a growing inclination to pre-register studies in databases such as the Open Science Framework (OSF). This facilitates the integrity of data use and encourages the adoption of standard practices. Furthermore, the utilization of publicly available repositories with open-source software and datasets, such as NCI cancer research data commons, will contribute to the continued advancement of transparency and reproducibility in radiomics studies.
Radiomics features
Shape features | ||||||
Shape feature (3D) | Descriptors of the three-dimensional size and shape of the VOI | Mesh volume Voxel volume Surface area Surface area to volume ratio Sphericity Compactness1 Compactness 2 Spherical disproportion Maximum 3D diameter | Maximum 2D diameter (slice) Maximum 2D diameter (Column) Maximum 2D diameter (Row) Major Axis length Minor Axis length Least Axis length Elongation Flatness | |||
Shape feature (2D) | Features descriptors of the two-dimensional size and shape of VOI. | Mesh surface Pixel Surface Perimeter Perimeter to Surface ratio Sphericity | Spherical disproportion Maximum 2D diameter Major Axis Length Minor Axis Length Elongation | |||
First order features | ||||||
Descriptor of the distribution of voxel intensities within the image region defined by the mask through commonly used and basic metric | Energy Total Energy Entropy Minimum 10th percentile 90th percentile Maximum Mean Median Interquartile range Variance | Range Mean Absolute Deviation (MAD) Robust mean Absolute Deviation (rMAD) Root Mean Squared (RMS) Standard Deviation Skewness Kurtosis Uniformity | ||||
Second order features | ||||||
Gray Level Co-occurrence Matrix (GLCM) | Autocorrelation Joint Average Cluster Prominence Cluster Shade Cluster Tendency Contrast Correlation Difference Average Difference Entropy Difference Variance Joint Energy Joint Entropy Informational Measure of Correlation (IMC) 1 Informational Measure of Correlation (IMC) 2 | Inverse Difference Moment (IDM) Maximal Correlation Coefficient (MCC) Inverse Difference Moment Normalized (IDMN) Inverse Difference (ID) Inverse Difference Normalized (IDN) Inverse Variance Maximum Probability Sum Average Sum Entropy Sum of Squares | ||||
Gray Level Size Zone Matrix (GLSZM) | Small Area Emphasis (SAE) Large Area Emphasis (LAE) Gray Level Non-Uniformity (GLN) Gray Level Non-Uniformity Normalized (GLNN) Size-Zone Non-Uniformity (SZN)Zone Non-Uniformity Normalized (SZNN) Zone Percentage (ZP) Gray Level Variance (GLV) Zone Variance (ZV) Zone Entropy (ZE) Low Gray Level Zone Emphasis (LGLZE) High Gray Level Zone Emphasis (HGLZE) Small Area Low Gray Level Emphasis (SALGLE) Small Area High Gray Level Emphasis (SAHGLE) Large Area Low Gray Level Emphasis (LALGLE) Large Area High Gray Level Emphasis (LAHGLE) | |||||
Gray Level Run length Matrix (GLRLM) | Short Run Emphasis (SRE) Long Run Emphasis (LRE) Gray Level Non-uniformity (GLN) Gray Level Non-Uniformity Normalized (GLNN) Run Length Non-Uniformity (RLN) Run Length Non-Uniformity Normalized (RLNN) Run Percentage (RP) Gray Level Variance (GLV) Run Variance (RV) Run Entropy (RE) Low Gray Level Run Emphasis (LGLRE) High Gray Level Run Emphasis (HGLRE) Short Run Low Gray Level Emphasis (SRLGLE) Short Run High Gray Level Emphasis (SRHGLE) Long Run Low Gray Level Emphasis (LRLGLE) Long Run High Gray Level Emphasis (LRHGLE) | |||||
Neighboring Gray Tone Difference Matrix (NGTDM) | Coarseness Contrast Busyness Complexity Strength | |||||
Gray Level Dependence Matrix (GLDM) | Small Dependence Emphasis (SDE) Large Dependence Emphasis (LDE) Gray Level Non-Uniformity (GLN) Dependence Non-Uniformity (DN) Dependence Non-Uniformity Normalized (DNN) Gray Level Variance (GLV) Dependence Variance (DV) Dependence Entropy (DE) Low Gray Level Emphasis (LGLE) High Gray Level Emphasis (HGLE) Small Dependence Low Gray Level Emphasis (SDLGLE) Small Dependence High Gray Level Emphasis (SDHGLE) Large Dependence Low Gray Level Emphasis (LDLGLE) Large Dependence High Gray Level Emphasis (LDHGLE) |
Furthermore, special radiomics features have been widely investigated in recent research. “Delta-radiomics”, referred to as the evolution of radiomic features over time during treatment, can assess each lesion at multiple time points, rather than information derived from a single lesion at a single time point. Consequently, delta radiomics can overcome the reproducibility issue and sometimes the overfitting issue. Liu et al. reported that the unique delta radiomics could provide a consistent framework for immunotherapy response assessment and overcome the overfitting issues and confusion caused by mixed response patterns of immunotherapy[42]; similar results have been reported by the Cousin group[43]. The Korrami group reported that the most discriminating texture features for prediction of immunotherapy response were DelRADx Haralick, Gabor and Laws features[34]. In addition, peritumoral radiomic features rather than the features extracted from the solid tumour itself are the other popular features for investigation recently as the immunotherapy treatment outcomes are also largely influenced by the TME. When performing segmentation of VOI, good to excellent performance of peri-tumoral radiomic features was reported in predicting immunotherapy response[34,44-48]. He et al. reported that the TME contributed no less to the accuracy of prediction than did the tumour region[49]. However, there is little consensus within the literature as to how much peri-tumoral region should be used in the VOI, which should be carefully considered in any radiomic analysis to avoid false-positive associations.
Feature selection also poses a significant challenge in model generation, considering all the possible features in the model could lead to overfitting and decreasing the model’s utility when applied to new data[50]. Therefore, proper dimensional reduction techniques should be employed to reduce non-informative or redundant predictors in the model to select the representative features. There are two main types of feature selection techniques: supervised and unsupervised; supervised methods can be further divided into filter, wrapper, and intrinsic methods. The filter methods are using the characteristics of given data to select features with higher scores, which are then employed in the model. The wrapper methods involve multiple models to decide which features should be removed or added to achieve the best-performing combination through a ranking approach. The intrinsic methods use the classifier for decision-making with features selected within the training process[51,52]. Commonly used feature selection methods are listed in Table 4, such as Principal Component Analysis (PCA), Clustering, Least Absolute Shrinkage, Selection Operator (LASSO), and Automatic Relevance Determination (ARD)[53]. The feature selection step is difficult to standardize as there is no universal best feature selection method. The best combination is the one that performs better in solving the specific problem under consideration. Consequently, the transparency of feature selection by accurately describing the process and possibly sharing the data would enable the researchers to repeat and implement the results in new studies to achieve better results.
Summary of feature selection and classification methods with the Acronyms and full names
Acronym | Feature selection method name | Acronym | Classification method name |
CHSQ | Chi-square score | GNB | Gaussion naïve bayes |
TSQ | T-test score | MNB | Multinomial naïve bayes |
WLCX | Wilcoxon | BNB | Bemoulli naïve bayes |
VAR | Variance | KNN | K-nearest neighborhood |
RELF | Relief | RF | Random forest |
MI | Mutual information | BAG | Bagging |
MRMRe | Minimum redundancy maximum relevance ensemble | DT | Decision tree |
RF | Random forest | GBDT | Gradient boosting decision tree |
ETE | Extra tree ensemble | Adaboost | Adaptive boosting |
GBDT | Gradient boosting decision tree | XGB | Xgboost |
XGB | Xgboost | LDA | Linear discriminant analysis |
Automatic relevance determination | LGR | Logistic regression | |
L-SVM | L1-based linear support vector machine | Linear-SVM | Linear support vector machine |
LASSO | Least absolute shrinkage and selection operator | RBF-SVM | Radial basis function support vector machine classification |
EN | Elastic net | MLPC | Multi-layer perceptorn |
PCA | Principal component analysis |
Modelling techniques
The final stage of radiomics involves constructing mathematical models to provide predictive information and to evaluate the current research objectives through artificial intelligence or machine-learning techniques using algorithms to uncover intricate relationships from the data. There are three subgroups in machine-learning techniques: supervised, unsupervised, and reinforcement learning. Supervised learning techniques are widely utilized in radiomic analysis, which is analysed alongside outputs to establish a mathematical representation linking input characteristics and the target variables, such as Support Vector Machine (SVM), Decision Tree (DT), Random Forest (RF), and Multivariate Cox Proportional Hazard Regression models. Unsupervised learning models could be an alternative, especially in cases of poorly labelled data, to uncover hidden structures and learn unknown patterns within the data. Commonly employed algorithms include K-means clustering, Hierarchical clustering, and Linear discriminant analysis. Reinforcement learning techniques utilize labelled input data to acquire a sequence based on the outcome of the interactions with an environment[54]. Like feature selection methods, no single model appears to outperform others across different tasks. Hence, considering the combination of multiple models may prove to be an effective approach to enhancing model performance. Moreover, deep learning techniques are assuming an increasingly crucial role in the medical field and offer a promising direction for radiomics. Deep learning models can be further categorized into two types: convolutional neural networks (CNN), where input data are structured in two-dimensional or three-dimensional formats, and typical models, where input data are in vector form (nonstructured, one-dimensional)[55].
To measure the quality of the model’s prediction, the parameter of the Receiver Operating Characteristic (ROC) curve and Area Under Curve (AUC) are used. ROC curve illustrates the relationship between the True Positive Rate (TPR) vs. False Positive Rate (FPR) at various classification thresholds. Decreasing the classification threshold classifies more items as positive, resulting in an increase in both FPR and TPR. AUC then further offers a comprehensive measure of performance across all potential classification thresholds with values ranging from 0 to 1 (a value of 1 indicates a complete separation between classes, and a value of 0 signifies the classifier’s complete failure)[56]. However, biased training samples, improper utilization of training datasets, excessively strong adjustments, and data leakage from training to testing datasets all contribute to over-fitting results in numerous studies with high ROC and AUC values. Consequently, to enhance the generalizability of the model, it is crucial to incorporate validation datasets, particularly those that are independent of the training set and ideally sourced from multi-institutions.
Validation of the model and evaluation of its performance
In order to facilitate the translation of radiomics into clinical implementation, it is imperative to ensure the reproducibility of a machine learning model performance. Validation techniques serve as valuable tools for assessing the generalizability of the model. A valid model should demonstrate statistical consistency between the training and testing datasets, which can be evaluated through internal validation or external validation. Internal validation methods, such as K-fold cross-validation and bootstrapping, are commonly employed for initial evaluations or fine-tuning of the developing model to estimate its performance. Theoretically, radiomic studies must be validated through external validation, such as temporal or geographic validation, against completely independent data, preferably with data from a different institution[57]. An externally validated model carries greater reliability compared to an internally validated model since internal validation can introduce bias during the process of feature selection, as the feature selection algorithm can leak into the entire dataset and cause overly optimistic results. External validation plays a critical role in validating the generalizability of the models, which avoids any deliberate selection bias and maintains the balance in patient demographic features. For example, geographic validations, which involve multiple technical parameters, such as imaging acquisition scanners and protocols, are expected to be more representative of real-world practice[57].
Furthermore, some criteria have been proposed to assess the characteristics and ultimately the quality of a radiomic study, including its reporting. For example, the Radiomic Quality Score (RQS) comprises 16 items and allows for a maximum of 36 potential points. The ROS encompasses various aspects, including acquisition parameters and data sharing, with the aim of enhancing the quality of radiomic research[58,59]. A recent study proposed a classification framework for image mining studies, drawing inspiration from the phases of drug development ranging from phase I to IV. The phase categorisation based on the sample size, type of study (retrospective/prospective), validation approach, and the development stage highlighted the scarcity of phase III and IV studies[60]. With some specific adaptations, adhering to these criteria could potentially establish radiomics as the standardized tool in healthcare.
RADIOMICS AND AI APPLICATION OF IMMUNOTHERAPY IN LUNG CANCER
In the context of assessing immunotherapy-related prediction and response, the studies on the application of radiomics in lung cancer can be roughly divided into three categories.
The first is to predict the occurrence of hyperprogression. The exact pathophysiological mechanism of this phenomenon remains unclear; however, the upregulation of other immune checkpoints, activation of oncogenic signalling including MDM2 amplification or EGFR alterations, and the modification of TME or local inflammation could help in tumour escape and progression[61]. According to the available reports, among patients receiving immunotherapy, the incidence of hyperprogression fluctuated from 8% to 25.7%[61,62]. Therefore, if this unique immunotherapy response can be identified, especially from pseudoprogression, the worse situation may be avoided. Castello et al. reported that hyperprogression was significantly associated with a high metabolic tumour burden, expressed by MTV and TLG[30]. They also demonstrated that clinical variables, derived Neutrophil-to-Lymphocyte Ratio (dNLR), were found to be associated with hyperprogression status[30]. Similarly, the Tunali group reported that the elevated NLR could imply that inflammatory cells are present in TME and lead to immune evasion or escape from the immune intervention. They identified three radiomics features (Radial gradient border SD-2d, Border quartile coefficient of dispersion, Border 3D Laws E5E5L5), which were extracted from the border regions of the tumours and may be capturing the data related to the TME. Another border feature, NGTDM strength, can quantify the similarity of pixel intensities within a neighbourhood to derive heterogeneity and the texture of the TME[45]. The Vaidya group reported similar results with one peritumoral feature from 5 to 10 mm outside the tumour and two nodule vessel-related tortuosity features relating to hyperprogression status[47].
The second category is to predict the response to immunotherapy, which has been a topic of intense discussion among radiomic applications in oncology. The Tunali group reported that the high GLCM inverse difference is associated with hypoxic tumours, which contribute to angiogenesis, tumour growth, invasiveness, and metastasis[44]. They also found that the clinical co-variable features with the presence of hepatic and/or bone metastases are associated with poor outcomes of immunotherapy in lung cancer[45]. The resident and infiltrated stromal cells in the bone marrow and their molecular mediators create a unique bone metastatic TME that leads to persistent immunosuppression[63]. The liver is a known site of immune tolerance, which may be the cause of resistant liver metastasis lesions to immunotherapy[45]. Furthermore, the Valentinuzzi group reported that most responders with NSCLC treated with pembrolizumab have finer and more homogeneous metabolic structures, as reflected by higher Small Run Emphasis (SRE) and lower Entropy-GLCM, respectively[24]. Similar findings from the Ladwa group showed that patients who have a treatment response to nivolumab had a combination of positive skewness in CT texture along with either low entropy or low standard deviation (SD), which indicated a hyperdense yet homogenous tumour[64]. On the contrary, Mu et al. found that texture features (PET_SRLGE, KLD_SZE), indicating more heterogeneous and more convex (smaller CHDensity) tumours and lower mean SUV, were positively correlated with durable clinical benefit (DCB)[65]. They explained that PD-L1 high expression cells can form a more rounded mass with the ability to evade immune attack. In addition, the highly metabolically active tumours will produce more lactic acid, which is a potent inhibitor of effector T-cell function[65]. The Ravanelli group reported similar results and claimed that lesions with more homogeneous enhancement are less responsible for immunotherapy based on the feature kurtosis on coarse texture[66]. This result was confirmed in a further study by the Trebeschi group, which found that lesions with more heterogeneous morphological profiles with non-uniform density patterns and compact borders are more likely to respond to immunotherapy[67]. Dercle et al. demonstrated that the temporal changes in intratumoral spatial heterogeneity were associated with sensitivity to nivolumab as well as gefitinib[68]. One possible explanation for these contradictory results is that morphological heterogeneity does not necessarily align with genetic heterogeneity. Factors such as infiltration, neovascularization, inflammation, and necrosis can also contribute to the morphological irregularities, which are commonly presented in pseudoprogression. In addition, the Nardone group reported that GLCM-entropy, GLCM-dissimilarity, and GLCM-correlation are correlated with a worse prognosis[69]. Recently, the Zhu group reported that the weighted radiomics approach exhibited superior performance compared to conventional approaches to predict response to immunotherapy[70]. Despite the promising outcomes, current radiomic studies have not yielded definitive conclusions. Further large cohort and standardized studies are needed to explore the association between radiomics features and immunotherapy responses.
The third application of radiomics in immunotherapy is to predict the PD-L1 expression level or other biomarkers in lung cancer patients. The Tian group used the deep learning model to investigate the lesion, especially the marginal zone of lesions with texture analysis, and reported that two features, Wavelet-HL_GLCM_ sum entropy and Wavelet-LL-GLRLM_gray level, are highly predictive for the PD-L1expression[71]. The Yoon group reported that a trend of larger Texture_GLRLM_RV, Texture_GLCM_ASM, and Texture_GLRLM-SRHGE with smaller Texture GLRLM-RE are associated with high PD-L1 expression. They also claimed that the lesion with homogenous and high CT attenuating large voxel values could be more likely to be PD-L1 positive[72]. Valentinuzzi et al. studied the radiomic feature of PET/CT in predicting outcomes of PD-L1 status[24]. Their results found that radiomic signatures had better predictive power than both PD-L1 status and iRECIST criteria[24]. Sun et al. built a radiomics-based predictor of the CD8 cell expression signature by using the machine learning model as such to predict the immunotherapy response[46]. The imaging biomarker can provide a promising way to predict the immune phenotype of lung cancers and infer the clinical outcomes after immunotherapy treatment.
RADIOGENOMICS AND “RADIO-…OMICS”
Although radiomic models have shown predictive and prognostic value in lung cancer, the most effective models for predicting prognosis and responses to immunotherapy are likely to be obtained through the integration of radiomic features with clinicopathological variables, including next-generation genomic and translational data. Certain efforts have been directed to the combination of multi-omic data, where radiomic features alone are not sufficient to capture tumour biology, to provide better insight. Yang et al. built up two nomogram models, combing radiomic data, and Rad-score, age, and M stage, to predict the immunotherapy response in lung cancer through multivariate logistic regression analysis. Their models achieved accuracy with AUC (0.902) for durable response and longer progression-free survival (PFS)[73]. More recently, the Bouhamama group combined radiomic features with transcriptomics to predict response to immunotherapy for advanced NSCLC patients[74]. However, composite models utilizing image data and co-variables are limited by data completeness. To overcome this issue, the establishment of public data repositories can potentially enhance over time by providing improved access to data through shared resources. Furthermore, the integration of these extensive and diverse data sources will require enhanced software and workflow management. Platforms such as MultiAssay Experiment have been specifically generated to facilitate interactive analysis by providing data objects and structures tailored for this purpose.
CONCLUSION
Despite the promising results in the field of lung cancer immunotherapy, these radiomic studies coupled with AI were too premature and lacked sound methodical quality to draw definitive conclusions for clinical implementation and broad adoption. Further studies with large cohorts are warranted to enhance the generalizability of these findings. In addition, the majority of these studies are observational (either prospective or retrospective) rather than interventional. More efforts, including rigorous testing, validation, and methodological improvements, are needed to translate these innovative radiomics tools into clinical practice to assist clinicians in the selection of lung cancer patients for immunotherapy.
DECLARATIONS
Authors’ contributionsDesigning and writing the manuscripts: Wu X, Polychronis A
Availability of data and materialsNot applicable.
Financial support and sponsorshipNone.
Conflicts of interestBoth authors declared that there are no conflicts of interest.
Ethical approval and consent to participateNot applicable.
Consent for publicationNot applicable.
Copyright© The Author(s) 2023.
REFERENCES
1. Sung H, Ferlay J, Siegel RL, et al. Global cancer statistics 2020: GLOBOCAN estimates of incidence and mortality worldwide for 36 cancers in 185 countries. CA Cancer J Clin 2021;71:209-49.
2. Reck M, Rodríguez-Abreu D, Robinson AG, et al. Pembrolizumab versus chemotherapy for
3. Mok TSK, Wu YL, Kudaba I, et al. Pembrolizumab
4. Antonia SJ, Villegas A, Daniel D, et al. Durvalumab after chemoradiotherapy in stage III non-small-cell lung cancer. N Engl J Med 2017;377:1919-29.
5. Sharma P, Hu-Lieskovan S, Wargo JA, Ribas A. Primary, adaptive, and acquired resistance to cancer immunotherapy. Cell 2017;168:707-23.
6. Davis AA, Patel VG. The role of PD-L1 expression as a predictive biomarker: an analysis of all US Food and Drug Administration (FDA) approvals of immune checkpoint inhibitors. J Immunother Cancer 2019;7:278.
7. Boothman AM, Scott M, Ratcliffe M, et al. Impact of patient characteristics, prior therapy, and sample type on tumor cell programmed cell death ligand 1 expression in patients with advanced NSCLC screened for the ATLANTIC study. J Thorac Oncol 2019;14:1390-9.
8. Binnewies M, Roberts EW, Kersten K, et al. Understanding the tumor immune microenvironment (TIME) for effective therapy. Nat Med 2018;24:541-50.
9. Giustini N, Bazhenova L. Recognizing prognostic and predictive biomarkers in the treatment of non-small cell lung cancer (NSCLC) with immune checkpoint inhibitors (ICIs). Lung Cancer 2021;12:21-34.
10. Brozos-Vázquez EM, Díaz-Peña R, García-González J, et al. Immunotherapy in nonsmall-cell lung cancer: current status and future prospects for liquid biopsy. Cancer Immunol Immunother 2021;70:1177-88.
11. Yang Y, Liu H, Chen Y, et al. Liquid biopsy on the horizon in immunotherapy of non-small cell lung cancer: current status, challenges, and perspectives. Cell Death Dis 2023;14:230.
12. de Miguel-Perez D, Russo A, Arrieta O, et al. Extracellular vesicle PD-L1 dynamics predict durable response to immune-checkpoint inhibitors and survival in patients with non-small cell lung cancer. J Exp Clin Cancer Res 2022;41:186.
13. Champiat S, Dercle L, Ammari S, et al. Hyperprogressive disease is a new pattern of progression in cancer patients treated by anti-PD-1/PD-L1. Clin Cancer Res 2017;23:1920-8.
14. Kim HK, Heo MH, Lee HS, et al. Comparison of RECIST to immune-related response criteria in patients with non-small cell lung cancer treated with immune-checkpoint inhibitors. Cancer Chemother Pharmacol 2017;80:591-8.
15. Tazdait M, Mezquita L, Lahmar J, et al. Patterns of responses in metastatic NSCLC during PD-1 or PDL-1 inhibitor therapy: comparison of RECIST 1.1, irRECIST and iRECIST criteria. Eur J Cancer 2018;88:38-47.
16. Soria F, Beleni AI, D’Andrea D, et al. Pseudoprogression and hyperprogression during immune checkpoint inhibitor therapy for urothelial and kidney cancer. World J Urol 2018;36:1703-9.
17. Seymour L, Bogaerts J, Perrone A, et al. iRECIST: guidelines for response criteria for use in trials testing immunotherapeutics. Lancet Oncol 2017;18:e143-52.
18. Mulkey F, Theoret MR, Keegan P, Pazdur R, Sridhara R. Comparison of iRECIST versus RECIST V.1.1 in patients treated with an anti-PD-1 or PD-L1 antibody: pooled FDA analysis. J Immunother Cancer 2020;8:e000146.
19. Nguyen HS, Ho DKN, Nguyen NN, Tran HM, Tam KW, Le NQK. Predicting EGFR mutation status in non-small cell lung cancer using artificial intelligence: a systematic review and meta-analysis. Acad Radiol 2023:S1076-6332(23)00179-4.
21. Liu Z, Wang S, Dong D, et al. The applications of radiomics in precision diagnosis and treatment of oncology: opportunities and challenges. Theranostics 2019;9:1303-22.
22. Dercle L, McGale J, Sun S, et al. Artificial intelligence and radiomics: fundamentals, applications, and challenges in immunotherapy. J Immunother Cancer 2022;10:e005292.
23. Shur JD, Doran SJ, Kumar S, et al. Radiomics in oncology: a practical guide. Radiographics 2021;41:1717-32.
24. Valentinuzzi D, Vrankar M, Boc N, et al. [18F]FDG PET immunotherapy radiomics signature (iRADIOMICS) predicts response of non-small-cell lung cancer patients treated with pembrolizumab. Radiol Oncol 2020;54:285-94.
25. Léger MA, Routy B, Juneau D. FDG PET/CT for evaluation of immunotherapy response in lung cancer patients. Semin Nucl Med 2022;52:707-19.
26. Kaira K, Higuchi T, Naruse I, et al. Metabolic activity by (18)F-FDG-PET/CT is predictive of early response after nivolumab in previously treated NSCLC. Eur J Nucl Med Mol Imaging 2018;45:56-66.
27. Seban RD, Rouzier R, Latouche A, et al. Total metabolic tumor volume and spleen metabolism on baseline [18F]-FDG PET/CT as independent prognostic biomarkers of recurrence in resected breast cancer. Eur J Nucl Med Mol Imaging 2021;48:3560-70.
28. Pellegrino S, Fonti R, Mazziotti E, et al. Total metabolic tumor volume by 18F-FDG PET/CT for the prediction of outcome in patients with non-small cell lung cancer. Ann Nucl Med 2019;33:937-44.
29. Routy B, Le Chatelier E, Derosa L, et al. Gut microbiome influences efficacy of PD-1-based immunotherapy against epithelial tumors. Science 2018;359:91-7.
30. Castello A, Rossi S, Mazziotti E, Toschi L, Lopci E. Hyperprogressive Disease in patients with non-small cell lung cancer treated with checkpoint inhibitors: the role of (18)F-FDG PET/CT. J Nucl Med 2020;61:821-6.
31. Polverari G, Ceci F, Bertaglia V, et al. (18)F-FDG pet parameters and radiomics features analysis in advanced nsclc treated with immunotherapy as predictors of therapy response and survival. Cancers 2020;12:1163.
32. Wu X, Huang Y, Zhao Q, et al. PD-L1 expression correlation with metabolic parameters of FDG PET/CT and clinicopathological characteristics in non-small cell lung cancer. EJNMMI Res 2020;10:51.
33. Bak SH, Park H, Sohn I, Lee SH, Ahn MJ, Lee HY. Prognostic impact of longitudinal monitoring of radiomic features in patients with advanced non-small cell lung cancer. Sci Rep 2019;9:8730.
34. Khorrami M, Prasanna P, Gupta A, et al. Changes in CT radiomic features associated with lymphocyte distribution predict overall survival and response to immunotherapy in non-small cell lung cancer. Cancer Immunol Res 2020;8:108-19.
35. Gillies RJ, Kinahan PE, Hricak H. Radiomics: images are more than pictures, they are data. Radiology 2016;278:563-77.
36. Clarke LP, Nordstrom RJ, Zhang H, et al. The quantitative imaging network: NCI’s historical perspective and planned goals. Transl Oncol 2014;7:1-4.
37. Han T, Wu J, Luo W, Wang H, Jin Z, Qu L. Review of generative adversarial networks in mono- and cross-modal biomedical image registration. Front Neuroinform 2022;16:933230.
38. Rios Velazquez E, Aerts HJ, Gu Y, et al. A semiautomatic CT-based ensemble segmentation of lung tumors: comparison with oncologists’ delineations and with the surgical specimen. Radiother Oncol 2012;105:167-73.
39. Hosny A, Aerts HJ, Mak RH. Handcrafted versus deep learning radiomics for prediction of cancer therapy response. Lancet Digit Health 2019;1:e106-7.
40. Zhao P, Li C, Rahaman MM, et al. A comparative study of deep learning classification methods on a small environmental microorganism image dataset (EMDS-6): from convolutional neural networks to visual transformers. Front Microbiol 2022;13:792166.
41. Zwanenburg A, Vallières M, Abdalah MA, et al. The image biomarker standardization initiative: standardized quantitative radiomics for high-throughput image-based phenotyping. Radiology 2020;295:328-38.
42. Liu Y, Wu M, Zhang Y, et al. Imaging biomarkers to predict and evaluate the effectiveness of immunotherapy in advanced non-small-cell lung cancer. Front Oncol 2021;11:657615.
43. Cousin F, Louis T, Dheur S, et al. Radiomics and delta-radiomics signatures to predict response and survival in patients with non-small-cell lung cancer treated with immune checkpoint inhibitors. Cancers 2023;15:1968.
44. Tunali I, Tan Y, Gray JE, et al. Hypoxia-related radiomics and immunotherapy response: a multicohort study of non-small cell lung cancer. JNCI Cancer Spectr 2021:5.
45. Tunali I, Gray JE, Qi J, et al. Novel clinical and radiomic predictors of rapid disease progression phenotypes among lung cancer patients treated with immunotherapy: an early report. Lung Cancer 2019;129:75-9.
46. Sun R, Limkin EJ, Vakalopoulou M, et al. A radiomics approach to assess tumour-infiltrating CD8 cells and response to anti-PD-1 or anti-PD-L1 immunotherapy: an imaging biomarker, retrospective multicohort study. Lancet Oncol 2018;19:1180-91.
47. Vaidya P, Bera K, Patil PD, et al. Novel, non-invasive imaging approach to identify patients with advanced non-small cell lung cancer at risk of hyperprogressive disease with immune checkpoint blockade. J Immunother Cancer 2020;8:e001343.
48. Korpics MC, Polley MY, Bhave SR, et al. A validated T cell radiomics score is associated with clinical outcomes following multisite SBRT and pembrolizumab. Int J Radiat Oncol Biol Phys 2020;108:189-95.
49. He B, Dong D, She Y, et al. Predicting response to immunotherapy in advanced non-small-cell lung cancer using tumor mutational burden radiomic biomarker. J Immunother Cancer 2020;8:e000550.
50. Incoronato M, Aiello M, Infante T, et al. Radiogenomic analysis of oncological data: a technical survey. Int J Mol Sci 2017;18:805.
51. Stanzione A, Cuocolo R, Ugga L, et al. Oncologic imaging and radiomics: a walkthrough review of methodological challenges. Cancers 2022;14:4871.
52. Jović A, Brkić K, Bogunović N. A review of feature selection methods with applications. In: Proceedings of the 38th International Convention on Information and Communication Technology, Electronics and Microelectronics (MIPRO): IEEE; 2015 May 25-29; Opatija, Croatia. pp. 1200-5.
53. Nguyen LH, Holmes S. Ten quick tips for effective dimensionality reduction. PLoS Comput Biol 2019;15:e1006907.
54. Avanzo M, Wei L, Stancanello J, et al. Machine and deep learning methods for radiomics. Med Phys 2020;47:e185-202.
55. Choy G, Khalilzadeh O, Michalski M, et al. Current applications and future impact of machine learning in radiology. Radiology 2018;288:318-28.
56. Hajian-Tilaki K. Receiver operating characteristic (ROC) curve analysis for medical diagnostic test evaluation. Caspian J Intern Med 2013;4:627-35.
57. Park SH, Han K. Methodologic guide for evaluating clinical performance and effect of artificial intelligence technology for medical diagnosis and prediction. Radiology 2018;286:800-9.
58. Lambin P, Leijenaar RTH, Deist TM, et al. Radiomics: the bridge between medical imaging and personalized medicine. Nat Rev Clin Oncol 2017;14:749-62.
59. Park JE, Kim D, Kim HS, et al. Quality of science and reporting of radiomics in oncologic studies: room for improvement according to radiomics quality score and TRIPOD statement. Eur Radiol 2020;30:523-36.
60. Sollini M, Antunovic L, Chiti A, Kirienko M. Towards clinical application of image mining: a systematic review on artificial intelligence and radiomics. Eur J Nucl Med Mol Imaging 2019;46:2656-72.
61. MO, Kirca O, Gunduz S, Ozdogan M. Hyperprogression after immunotherapy: a comprehensive review. J BUON 2019;24:2232-41. Available from: https://napier-repository.worktribe.com/output/2452554/hyperprogression-after-immunotherapy-a-comprehensive-review [Last accessed on 3 Aug 2023].
62. Sehgal K. Hyperprogression in patients with cancer receiving immune checkpoint inhibitors. JAMA Netw Open 2021;4:e211839.
63. Reinstein ZZ, Pamarthy S, Sagar V, et al. Overcoming immunosuppression in bone metastases. Crit Rev Oncol Hematol 2017;117:114-27.
64. Ladwa R, Roberts KE, O’Leary C, Maggacis N, O’Byrne KJ, Miles K. Computed tomography texture analysis of response to second-line nivolumab in metastatic non-small cell lung cancer. Lung Cancer Manag 2020;9:LMT38.
65. Mu W, Tunali I, Gray JE, Qi J, Schabath MB, Gillies RJ. Radiomics of (18)F-FDG PET/CT images predicts clinical benefit of advanced NSCLC patients to checkpoint blockade immunotherapy. Eur J Nucl Med Mol Imaging 2020;47:1168-82.
66. Ravanelli M, Agazzi GM, Milanese G, et al. Prognostic and predictive value of histogram analysis in patients with non-small cell lung cancer refractory to platinum treated by nivolumab: a multicentre retrospective study. Eur J Radiol 2019;118:251-6.
67. Trebeschi S, Drago SG, Birkbak NJ, et al. Predicting response to cancer immunotherapy using noninvasive radiomic biomarkers. Ann Oncol 2019;30:998-1004.
68. Dercle L, Fronheiser M, Lu L, et al. Identification of non-small cell lung cancer sensitive to systemic cancer therapies using radiomics. Clin Cancer Res 2020;26:2151-62.
69. Nardone V, Tini P, Pastina P, et al. Radiomics predicts survival of patients with advanced non-small cell lung cancer undergoing PD-1 blockade using Nivolumab. Oncol Lett 2020;19:1559-66.
70. Zhu Z, Chen M, Hu G, et al. A pre-treatment CT-based weighted radiomic approach combined with clinical characteristics to predict durable clinical benefits of immunotherapy in advanced lung cancer. Eur Radiol 2023;33:3918-30.
71. Tian P, He B, Mu W, et al. Assessing PD-L1 expression in non-small cell lung cancer and predicting responses to immune checkpoint inhibitors using deep learning on computed tomography images. Theranostics 2021;11:2098-107.
72. Yoon J, Suh YJ, Han K, et al. Utility of CT radiomics for prediction of PD-L1 expression in advanced lung adenocarcinomas. Thorac Cancer 2020;11:993-1004.
73. Yang B, Zhou L, Zhong J, et al. Combination of computed tomography imaging-based radiomics and clinicopathological characteristics for predicting the clinical benefits of immune checkpoint inhibitors in lung cancer. Respir Res 2021;22:189.
74. Bouhamama A, Leporq B, Faraz K, et al. Radiomics combined with transcriptomics to predict response to immunotherapy from patients treated with PD-1/PD-L1 inhibitors for advanced NSCLC. Fron Radiol 2023;3:1168448.
75. Jiang M, Sun D, Guo Y, et al. Assessing PD-L1 expression level by radiomic features from PET/CT in nonsmall cell lung cancer patients: an initial result. Acad Radiol 2020;27:171-9.
76. Liu C, Gong J, Yu H, Liu Q, Wang S, Wang J. A CT-Based radiomics approach to predict nivolumab response in advanced non-small-cell lung cancer. Front Oncol 2021;11:544339.
77. Shen L, Fu H, Tao G, Liu X, Yuan Z, Ye X. Pre-immunotherapy contrast-enhanced CT texture-based classification: a useful approach to non-small cell lung cancer immunotherapy efficacy prediction. Front Oncol 2021;11:591106.
Cite This Article
Export citation file: BibTeX | RIS
OAE Style
Wu X, Polychronis A. Application of radiomics and artificial intelligence in lung cancer immunotherapy: a guide and hurdles from clinical trials. J Cancer Metastasis Treat 2023;9:29. http://dx.doi.org/10.20517/2394-4722.2023.10
AMA Style
Wu X, Polychronis A. Application of radiomics and artificial intelligence in lung cancer immunotherapy: a guide and hurdles from clinical trials. Journal of Cancer Metastasis and Treatment. 2023; 9: 29. http://dx.doi.org/10.20517/2394-4722.2023.10
Chicago/Turabian Style
Wu, Xiaorong, Andreas Polychronis. 2023. "Application of radiomics and artificial intelligence in lung cancer immunotherapy: a guide and hurdles from clinical trials" Journal of Cancer Metastasis and Treatment. 9: 29. http://dx.doi.org/10.20517/2394-4722.2023.10
ACS Style
Wu, X.; Polychronis A. Application of radiomics and artificial intelligence in lung cancer immunotherapy: a guide and hurdles from clinical trials. J. Cancer. Metastasis. Treat. 2023, 9, 29. http://dx.doi.org/10.20517/2394-4722.2023.10
About This Article
Copyright
Data & Comments
Data
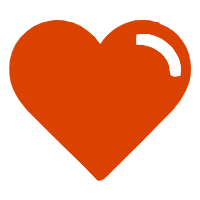

Comments
Comments must be written in English. Spam, offensive content, impersonation, and private information will not be permitted. If any comment is reported and identified as inappropriate content by OAE staff, the comment will be removed without notice. If you have any queries or need any help, please contact us at support@oaepublish.com.